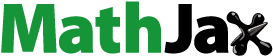
Abstract
The special planning for shelters is an integral part of overall city planning and is a key issue in solving the urban security problem. During the planning of earthquake emergency shelters, it is usually necessary to prioritize the designated shelters to avoid wasting resources. However, in previous research initiatives, special attention has been given to the general location-allocation model without prior consideration of the designated shelters. This article presents a multi-objective supplemental location-allocation optimization model for earthquake emergency shelters that addresses the concerns related to the designated shelters. This model is believed to remain more consistent with the knowledge of shelter planners and policy makers by incorporating the uncertainties of the temporal dynamics of population distribution and the spatially heterogeneous distribution of evacuees under various earthquake damage scenarios by using the elliptical attenuation model for seismic intensity into the location-allocation problem. A case study of the central area of Beijing, China is presented. A modified particle swarm optimization algorithm is applied to solve the supplemental location-allocation model and the general location-allocation model, and the solutions obtained from the two models are then compared. The results show that the solutions of the supplemental location-allocation model significantly minimized the shelter area by prioritizing the designated shelters. By contrast, without prior consideration of the designated shelters, the shelter areas significantly increased, which slightly decreased the evacuation distance in the general location-allocation model. The policy makers could select solutions from the obtained minimal-area scheme using the supplemental location-allocation model that ensures acceptable evacuation distances and minimal budgets by prioritizing the designated shelters during the phased planning of shelters in Beijing.
1. Introduction
Most cities in China have dramatically expanded over the last several decades, and the immeasurable value losses in light of frequent disasters may be enormous (Ding et al. Citation2005). Construction of emergency shelters has proven to be one of the most efficient ways to reduce human casualties and property losses during a disaster. For example, after the Great East Japan Earthquake on March 11, 2011, emergency shelters played an extremely important role, with approximately 250,000 people taking temporary refuge (BBC Citation2011). Typhoon Haiyan struck the Philippines in 2013, devastating communities and claiming more than 6,300 lives. These local communities are in need of emergency shelters that can withstand severe storm conditions. Therefore, GlobalMedic initiated a project rooted in disaster preparedness in 2015, and four evacuation shelters were constructed in highly vulnerable communities (GlobalMedic Citation2016). Powerful Typhoon Mangkhut slammed into the Philippines in 2018; however, there were no major damages or casualties in the region (CBS Citation2018), where residents poured into emergency shelters some of which were already filled to capacity (RNZ 2018). As an integral part of the overall urban planning, urban emergency shelters as safe spaces in a city, their detailed and special planning is necessary to make evacuation as efficient as possible, which has been a key issue to solve the urban security problem. Hence, the planning of emergency shelters has to be established, implemented, monitored, maintained and constantly updated by local city decision makers (Scheer et al. Citation2012).
The planning of disaster emergency shelters in cities must consider the interests of both planners and residents. Consequently, the multi-objective location-allocation model has received increasing attention for the planning of disaster shelters. In addition, several objectives are generally selected to minimize evacuation distance or time, facility area, construction costs and disaster risks in the shelter location-allocation problem. For instance, Alçada Almeida et al. (Citation2009) selected four objectives to minimize the total distance from all of the population to shelters, the total risk of the primary path being impassable, the fire risks at shelters and the total time from the shelters to the university hospital; then, the authors proposed a multi-objective p-median model for evacuation during major fires and solved it by using a web-based decision support system. Tzeng et al. (Citation2007) developed a deterministic multi-objective model for earthquake relief distribution with regard to minimizing the total costs and total travel time and maximizing the satisfaction of demand points in a planning period and solved it using a fuzzy multi-objective programming method. Doerner et al. (Citation2009) presented a multi-objective optimization model for hurricane disaster shelters with the objectives of minimizing the weighted average of the minimum and maximum distances, the risk of tsunami events and costs and proposed a heuristic approach based on the non-dominated sorting genetic algorithm-II (NSGA-II) to provide optimal solutions. Yushimito et al. (Citation2012) looked to maximize coverage of affected regions while minimizing human suffering through the use of a social cost function to create an uncapacitated nonlinear location optimization model that was solved by a Voronoi-based heuristic algorithm, and they presented an application to a real-world problem with data from Hurricane Katrina. Bozorgi-Amiri et al. (Citation2012) proposed a robust multi-objective location-allocation optimization model in a stochastic environment by using discrete scenarios, which yielded more flexibility and practical aspects in real-world cases. The model was formulated as a mixed-integer nonlinear programming to minimize the sum of the expected total cost (which includes costs of location, procurement, transportation, holding, and shortage) and the variance of the total cost. Furthermore, a modified particle swarm optimization (PSO) algorithm was introduced to solve the model that exhibited successful performance in any problem size. In a subsequent study, the model added an objective of maximizing the satisfaction levels of the affected areas by minimizing the sum of the maximum shortages in the affected areas using a case study for disaster planning for earthquake scenarios in the region of Iran (Bozorgi-Amiri et al. Citation2013). Zhang et al. (Citation2013) proposed a bottleneck Steiner tree based multi-objective optimization model to find the location of emergency facilities by minimizing the generalized cost of the system and minimizing the maximal distance between facilities and demand points. The model is solved using a population-based pattern-matching algorithm called cellular stochastic diffusion search. Barzinpour and Esmaeili (Citation2014) developed a multi-objective mixed-integer linear programming model, with the objectives of maximizing the population coverage and minimizing the construction costs and traffic costs by using a virtual zoning approach to achieve humanitarian and financial goals. Hu et al. (Citation2014) developed a bi-objective model for earthquake emergency shelter location and allocation to minimize the total evacuation distance and the total cost, subject to capacity and contiguity constraints, and the model was solved with a non-dominated sorting genetic algorithm based on NSGA-II was developed to solve the bi-objective model. Based on the scenarios, Rodríguez-Espíndola and Gaytán (Citation2015) checked the flood areas by using GIS and proposed a model with the objective of minimizing distance and costs, and solved it using CPLEX 11.0 with the weighted-sum method. Zhao et al. (Citation2015) introduced a multi-objective allocation model for earthquake emergency shelters, with the objectives of minimizing the total weighted evacuation time and the total area of shelters, and solved it using a modified PSO algorithm combined with the simulated annealing (SA) algorithm. In the follow-up work, the relationships between evacuation population size, earthquake emergency shelter capacity, and evacuation time were explored (Zhao et al. Citation2017), and the solutions of the model with four objective groups were given and compared using safety, capacity and investment evaluation index (Xu et al. Citation2018). Trivedi and Singh (Citation2017) developed a hybrid multi-objective location-allocation model for emergency shelters based on analytic hierarchy process (AHP), fuzzy set theory and goal programming approach. It was solved by converting all objectives into a single objective function, and the results were validated by considering two real case studies of Nepal earthquakes in 2015. Zhao et al. (Citation2017) proposed a location optimization model for emergency rescue facilities that consisted of three objectives representing service capacity, global efficiency and equity in the case of large-scale urban environmental disasters. Appropriate spatial representation and encoding strategy were designed and coupled with NSGA-II to solve the model. Based on a hypothetical disaster scenario, a case study of the Chaoyang district in Beijing was presented to demonstrate the methodology and explore the conflicting objectives. Ma et al. (Citation2018) developed a multi-objective location-allocation model for earthquake emergency shelters using Wenchang of Hainan province, China as a case study. The two objectives were to minimize the total area of all shelters and to minimize the total evacuation distance, with the constraints of capacity and distance, and the model was solved by the modified PSO algorithm.
In recent years, there has been a growing number of studies that have focused on the multi-objective location-allocation problem for disaster emergency shelters. Nevertheless, uncertainty is an inseparable part of the location-allocation problem, which should be fully considered. Therefore, a multi-objective, multi-mode, multi-commodity, and multi-period stochastic model was presented by Najafi et al. (Citation2013) that was capable of handling real-life emergency relief conditions under capacity and demand uncertainties. Jalali et al. (Citation2016) developed a reliable bi-objective facility location model with provider-side uncertainty of facilities that was employed by a set of scenarios. In addition, a meta-heuristic algorithm called multi-objective biogeography-based optimization was employed to find a near-optimal Pareto solution, which was used to verify the solution that was compared with a non-dominated ranking genetic algorithm (NRGA) and a multi-objective SA algorithm. To incorporate uncertainty into a multi-objective model, Haghi et al. (Citation2017) developed a robust multi-objective optimization model for logistics planning by defining a set of scenarios that accounted for the uncertainty parameters. The ε-constraint method was utilized, and the MOGASA and NSGA-II algorithms were proposed for the large-size instances to solve the model.
On the one hand, research has focused on considering the multi-objective location-allocation problem as purely static processes, whereas there has been mild interest in formulating and solving dynamic multi-objective optimization problems, especially for disaster emergency shelters. Because, most real-world optimization problems involve objectives, constraints, and parameters that constantly change with time (Deb and Karthik Citation2007). For example, the dynamic changes, quick responses and unpredictable events are the main features of the emergency location-allocation problem, which make them quite different from the traditional location-allocation systems (Zhang et al. Citation2013). In addition, the standard for urban planning on earthquake resistance and hazardous prevention (GB50413-2007) has clearly stated that it is advisable to consider the dynamic diurnal variations of citizens when estimating the number and distribution of evacuees (PRC Ministry of Construction Citation2007). Therefore, the uncertainties in this article incorporate the temporal dynamics of the population distribution and the spatial heterogeneity of evacuee distribution under various earthquake damage scenarios by using the elliptical attenuation model for seismic intensity to build a dynamic multi-objective optimization model. On the other hand, the previous studies on the shelter location-allocation problem have mainly dealt with the question of how the evacuees can be evacuated as fast as possible and where the candidate shelters can be located as efficiently as possible during an emergency, which can be summarized as the general location-allocation problem. However, a handful of designated shelters exist that have been planned and equipped with relief supplies. Therefore, the main aims for the future planning of disaster emergency shelters should be to supplement the candidate shelters by prioritizing the designated shelters due to the insufficiency of these shelters, which is consistent with the concept of sustainable development. For example, Ma et al. (Citation2019) presented a hierarchical supplement location-allocation optimization model for disaster supply warehouses to achieve resource integration with the demonstration of the Beijing-Tianjin-Hebei region in China. To the best of our knowledge, no study has focused on the application of dynamic multi-objective supplemental location-allocation techniques for the planning of earthquake emergency shelters, which both makes up the research gaps of the uncertainties and designated shelters.
The estimation of spatially and temporally dynamic evacuees, the locations of designated shelters and candidate shelters, and the selection of evacuation routes for the evacuees have a great influence on the emergency transfer and resettlement in disaster relief practice. The number of evacuees is the most important input parameter for location-allocation models of earthquake emergency shelters, and the research on this parameter needs to be further refined. Therefore, in this study, we improved the spatially heterogeneous distribution model of evacuees under different earthquake scenarios by using the elliptical attenuation model for seismic intensity. In addition, the dynamic spatial-temporal variations of population exposure were fully considered to develop a conceptual model of the dynamic spatial-temporal distribution of evacuees based on subway smart card data and building type data. Finally, a scenario-based multi-objective supplemental location-allocation optimization model was developed by considering the designated shelters and the uncertainties of spatial-temporal dynamics with a real-world large-scale case study in the central area of Beijing. Notably, two contributions regarding uncertainties and the designated shelters were considered in this study.
The remainder of this article is organized as follows: Section 2 introduces the research methods of this study. Section 3 presents an application of the central area of Beijing as a case study and analyzes the results. In Section 4, the advantages and disadvantages of the research methods are discussed and the reasons are analyzed. And then, the future research direction is pointed out. Finally, the conclusions are given in section 5.
2. Methods
In this article, we consider the impacts of various objective and subjective factors on the spatial distribution of evacuees at the micro level. The objective factors include the destruction of community buildings at various intensity ranges caused by different earthquake scenarios at specific earthquake magnitudes and epicentre locations. The subjective factors include the willingness of residents to take refuge. The dynamic diurnal changes in the population at the macro level are also considered, and a conceptual model of the dynamic spatial-temporal distribution of evacuees is constructed (). Thus, our methods contain two parts: (1) developing the temporally dynamic population distribution model, and (2) modelling the spatially heterogeneous distribution of evacuees.
Figure 1. A conceptual model of the dynamic spatial-temporal distribution of evacuees. Source: the authors.
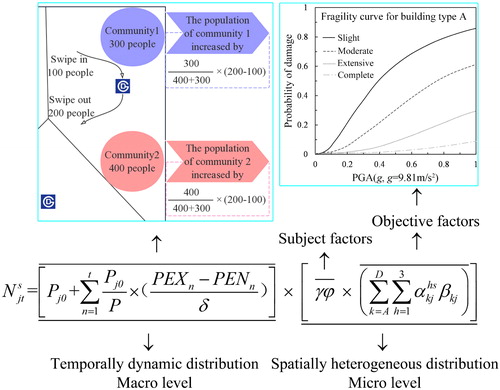
At the macro level, the total population in a region directly affects the dynamic diurnal variations of the population. In a day, the population shows an inverted U-shaped fluctuation. Similarly, evacuees will also show the characteristics of temporally dynamic distributions.
At the micro level, by setting different earthquake strike scenarios, the exceeding probabilities of different types of buildings reaching different damage states are obtained by using the associated fragility curves. The proportions of different building types are combined to calculate the rate of building damage in each community due to objective factors under different earthquake damage scenarios. Simultaneously, we should fully consider the subjective factors, such as the willingness of the evacuees to remain in their houses under different damage states and the willingness of the evacuees to choose the designated shelters, to simulate the spatially heterogeneous distribution of evacuees.
2.1. Temporally dynamic population distribution model
In research of the dynamic diurnal variations of population in large cities, scholars have found that the population shows an inverted U-shaped fluctuation in a single day. In the same way, similar fluctuations would occur in evacuees. The method proposed by Ma et al. (Citation2017) was used to calculate the dynamic temporal distribution of the population, as shown in EquationEquation (1)(1)
(1) . The population parameter in the deterministic location-allocation problem remained constant by deciding on a single period of time. However, the real-world location-allocation problem for earthquake emergency shelters is a decision that has long-term effects; so, the parameters of the system, such as the number of evacuees, construction costs, management costs, and environmental factors, may vary over time, and facility additions can occur at different times. Consequently, a critical decision in the dynamic location-allocation model is not only where but also when to build a facility (Boonmee et al. Citation2017).
(1)
(1)
where Pjt is the population of community j during period t. For further insight, see Ma et al. (Citation2017) for detailed information on the other variables.
2.2. Spatially heterogeneous distribution model of evacuees
The occurrence of an earthquake is random and uncertain. The epicentral intensities and isoseismal lines vary even for earthquakes with the same magnitude. The damages to community buildings may also differ due to their different structure types and locations. Consequently, the associated number of evacuees is characterized by spatial heterogeneity.
The isoseismic statistics in China indicate that the elliptical attenuation model is more reasonable but more complex than the point source attenuation model for describing the distribution of seismic intensity, especially for large-scale earthquakes (Shi et al. Citation2011). According to the previous research on the elliptical attenuation relationship, there are many empirical attenuation models at home and abroad. Based on the comparison of multiple attenuation models, an elliptical attenuation model in North China proposed by Cui (Citation2010) is used in this study. Based on the strikes of the faults and the orientations of the maximum principal stress of the earthquake scenarios in the region, the isoseismal lines can be drawn to determine the intensity zones in different communities. In this study, we improved the spatially heterogeneous distribution model of evacuees proposed by Xu et al. (Citation2018) by using the elliptical attenuation model.
The spatiotemporally dynamic evacuation needs in relation to the location-allocation problem for earthquake emergency shelters in this study had a spatiotemporal dynamic change () characteristic, which was calculated using EquationEquation (2)
(2)
(2) . The evacuation rates for each district at the different intensities of the two earthquake scenarios were calculated using EquationEquation (3)
(3)
(3) based on the building types, fragility curve (Fang et al. Citation2011) and the empirical equation (Ding et al. Citation2014) that related seismic intensity and peak ground acceleration.
(2)
(2)
(3)
(3)
where
represents the number of evacuees in community j during period t in scenario s, Rs represents the total evacuation rate due to multiple subjective and objective factors in scenario s, different
values represent the proportions of people who are willing to evacuate at different house damage states, and
represents the proportion of people who are willing to evacuate to designated shelters (shelter evacuees). We do not consider the non-shelter evacuees who evacuate to hotels or the places of their friends and relatives. For further insight, see Xu et al. (Citation2018) for detailed information on the other variables.
2.3. Supplemental location-allocation optimization model (SLA model)
The probabilities of earthquake occurrence are unknown, so the uncertain parameters are identified using discrete scenarios in our scenario-based location-allocation optimization problem for earthquake emergency shelters. Our scenario-based multi-objective SLA model prioritizes the designated shelters to make full use of them. The model will supplement the surrounding candidate shelters, which can be planned as new earthquake emergency shelters for the future when a designated shelter capacity is insufficient (can no longer serve any other community).
(4)
(4)
(5)
(5)
subject to
(6)
(6)
(7)
(7)
(8)
(8)
(9)
(9)
(10)
(10)
(11)
(11)
(12)
(12)
where
I is the set of candidate shelters: I = {1, 2, … i, … N}
J is the set of communities: J = {1,2, … j, … M}
J’ is the remaining communities that cannot be accommodated by the designated shelter
is the set of designated shelters:
S is the set of scenarios: S = {A, B, … s, … Z}
Ai is the area of candidate shelter i
dji is the length of the shortest path between community j and shelter i
Dj is the maximal evacuation distance for evacuees in community j
L is the smallest refuge area per capita (1 m2/person)
Our scenario-based multi-objective SLA model first allocates the evacuees into the designated shelters, and then, the model denoted in EquationEquations (4)(4)
(4) and Equation(5)
(5)
(5) selects new shelters and allocates the rest of the evacuees to make location-allocation decisions such that the total shelter area and evacuation distance are minimized, which are both subject to the capacity constraints expressed in EquationEquation (6)
(6)
(6) and the distance constraints expressed in EquationEquation (7)
(7)
(7) . The capacity constraints require that the areas of the shelters satisfy the daytime and nighttime demands of the evacuees. The distance constraint requires that the distance from community j to a potential shelter i should be less than the distance of the longest evacuation route returned by the algorithm. EquationEquation (8)
(8)
(8) ensures that each community will select only one shelter. EquationEquation (9)
(9)
(9) ensures that the designated shelters are selected. EquationEquation (10)
(10)
(10) ensures that the designated shelters can be fully utilized within their service area. EquationEquations (11)
(11)
(11) and Equation(12)
(12)
(12) define the decision variables in the model.
2.4. General multi-objective location-allocation optimization model (GLA model)
Most researchers have developed multi-objective models that are GLA models. The GLA model considers all of the designated shelters, parks, green spaces and playgrounds as candidate shelters to accommodate evacuees to have the same priority. Thus, the designated shelters are not the prior shelters to ensure that EquationEquations (9–10) are satisfied, which would be unrealistic and wasteful. In this study, we also developed a GLA model using the same objectives (4-5) and constraints (6-8, 11-12) to compare the solutions obtained by the SLA and GLA models.
The PSO algorithm has been successfully applied to facility location-allocation problems (Bozorgi-Amiri et al. Citation2012). To effectively solve complex and discrete optimization problems, Zhao et al. (Citation2015) modified the PSO algorithm for the location-allocation problem by applying a von Neumann structure in the former generations, adding global structure in the later generations, and combining with the SA algorithm to help obtain the global optimum. The modified PSO algorithm was used to solve the GLA and SLA models in this study.
3. A case study
3.1. City context and earthquake scenario hypothesis
Beijing is the capital of China and is located in the central part of the Yinshan-Yanshan seismic belt in the major earthquake area of North China, and a number of fault zones are distributed in the surrounding areas. According to historical earthquake situations, it is likely that a moderate or strong earthquake will occur in Beijing. The upper magnitude limit in the latent focal region in Beijing is 8.0, and most of the central area of Beijing is within the upper magnitude limit of 7.0 () (Zhou Citation2016). Therefore, four scenarios based on the historical earthquake data with increasing numbers of evacuees were considered: an M7.0 earthquake in South Beijing during the nighttime (Bn) and daytime (Bd) and an M8.0 earthquake in Sanhe-Pinggu during the nighttime (An) and daytime (Ad). In addition, the number of evacuees in community j during the daytime and nighttime in scenario s are set to the calculated maximum number of daytime evacuees and the number of evacuees from the total resident population, respectively. In this study, it is assumed that the intensity of the isoseismic trend of scenario A is consistent with the strike of the fault zone because the M8.0 earthquake in Sanhe-Pinggu is the latest surface rupture event in the fault, and that of scenario B is consistent with the direction of the maximum principal stress because there is no information about typical faults and their activities.
Figure 2. The latent focal region and upper magnitude limits in Beijing. Source: Figure (a) is from the GB 18306-2015 Seismic ground motion parameters zonation map of China, modified by the authors. Figure (b): the authors.
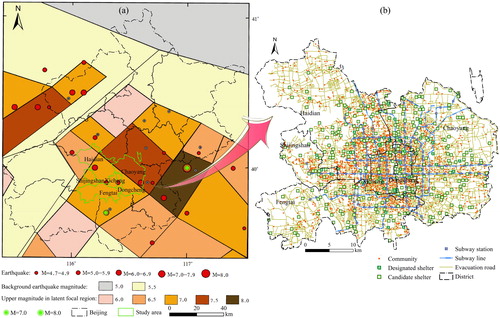
This research considers a study area consisting of the six central districts of Beijing: Dongcheng, Xicheng, Chaoyang, Haidian, Shijingshan and Fengtai. This densely populated region covers an area of 1369 km2, and the 6th National Census Data Bulletin recorded a resident population of 11.72 million in 2010. This region accounts for 8% of the area of Beijing, but approximately 61% of the total resident population is concentrated in this region, and population density is approximately seven times the average density of Beijing. The region covers 2039 communities and contains 39 designated earthquake shelters with the capacity to accommodate 1.51 million evacuees (China Earthquake Administration Citation2015) and has 17 subway lines with 173 subway stations (excluding the airport area) ().
3.2. Data
The data used in this study can be divided into three categories: temporally dynamic population distribution data (including the community population data and the subway smart card data), spatially heterogeneous distribution data of evacuees (including earthquake data, seismic fragility curves, building type data and evacuation willingness), and geospatial data. The proportions of the population who lived in different types of buildings as reported in the 6th National Census in 2010 for the various districts are used to represent the proportions of building type. See for details.
Table 1. Data used in this study.
High spatiotemporal resolution evacuees are critical for emergency preparedness and response, including rapid risk assessment, modified evacuation planning and relief delivery so as to improve the regional security. However, population data are usually organized by coarse administrative units or census blocks such as streets, which are too large for identifying evacuation units. Spatially finer data at the community level are more valuable for better planning in disaster risk management. In addition, the dynamics of evacuees on a detailed time scale could serve as a valuable information source (Miyazaki et al. Citation2015) for disaster risk assessments. Our dynamic hourly population data are then used to estimate the spatially heterogeneous distribution of evacuees under earthquake scenarios at different times to create better preparedness and mitigation plans based on subway smart card data.
There are 39 designated earthquake emergency shelters in the study area. In which, Relic Parks of Yuan Dynasty City and Relic Parks of Imperial City are two banded green spaces and divided into several shelters according to the natural street boundaries. These shelters cannot accommodate the total numbers of evacuees in scenarios B and A, which exceed 1 and 2 million individuals, respectively. Therefore, in accordance with the earthquake emergency shelter (outdoor) programme guidelines (OPG) for the central area of Beijing, 317 open spaces, including parks, green spaces and playgrounds with areas greater than 1000 m2 in the Dongcheng and Xicheng districts and 2000 m2 in the other districts, were also considered as candidate shelters (). Both types of shelters were treated as temporary shelters, and the shelter capacities that were used in the models were 60% of the total areas excluding surface water areas, as recommended by the OPG.
The best evacuation route for each community to reach its assigned shelter (namely, dji) was calculated using the Dijkstra algorithm with the objective of minimizing the total route length. The longest evacuation route distances (Dj) returned by the algorithm for each district are as follows: 6600 m in Fengtai and 7082 m in Haidian, with 6000 m used for all other districts in the GLA model. The Dj values returned by the algorithm for the GLA model are the same with the SLA model when supplementing new candidate shelters. In addition, the Dj values are set to 6000 m in the SLA model for the designated shelters to ensure arrival after 1 hour of walking. Earthquake-induced road damage was ignored because it was assumed that people would evacuate on foot according to the temporary evacuation plan for the city.
3.3. Results
3.3.1. Evacuation rate at the district level
The exceeding probabilities of complete damage, heavy damage and medium damage to buildings can be calculated based on the fragility curve (Fang et al. Citation2011) and the empirical equation (Ding et al. Citation2014) that relates seismic intensity and peak ground acceleration. Simultaneously, as shown in , the forecast results of the average earthquake damage rates for the different intensities of the two earthquake scenarios were derived from the product of exceeding probabilities and the proportions of the four building types. Finally, the evacuation rates for each district at the different intensities of the two earthquake scenarios were calculated using EquationEquation (3)(3)
(3) . shows the evacuation rates calculated for the different scenarios. In addition, shows the spatial distribution of the evacuation rates.
Figure 3. Spatial distribution of evacuation rate for each district at the different intensities of the two earthquake scenarios. Source: the authors.
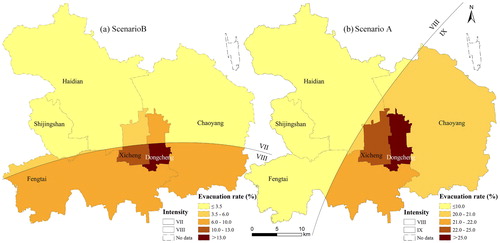
Table 2. Forecast results of average earthquake damage rates (%) for the six districts in central Beijing, corresponding to the product of α and β.
Table 3. Evacuation rates (Rs) calculated using EquationEquation (3)(3)
(3) .
shows that the earthquake damage rates under the different intensities in the different districts have the following consistency rules: With the increase in earthquake strike strength (intensity), the proportions of basically intact and slightly damaged buildings in different districts decreases, while the proportions of moderately, extensively and collapsed buildings increases. Under the conditions of low earthquake strike strength (such as VII intensity), the buildings in each district were mostly intact, followed by slightly damaged, and the proportions of reaching moderately and extensively damaged were both below 10%, while the proportion of collapsed buildings was approximately zero. In contrast, in the case of high earthquake strike strength (such as IX intensity), the differences in the proportions of buildings with different states of damage were small for each district.
As seen from and , the evacuation rates were lower in scenario B than scenario A, and the average evacuation rate of the communities was 5.95%. South Dongcheng and Shijingshan had the highest and lowest rates of 15.00 and 2.64%, respectively. The evacuation rates were higher in scenario A than scenario B with an average evacuation rate of the communities of 17.35%, and the highest rate was 27.92% in Dongcheng and the lowest was 8.09% in Shijingshan. Compared with the other four districts, the evacuation rates in Dongcheng and Xicheng remained high even in the same intensity zone. The reasons for this result lie in the fact that the proportions of the population within building type A (mainly reinforced concrete structures) and type B (mainly brick-concrete structures) were relatively low, while the proportion in type C (mainly brick-wood structures) buildings was relatively high. Shijingshan had the lowest evacuation rate because it was located in low-intensity zones in both scenarios. On the other hand, type A and B buildings had relatively high proportions with relatively lower exceeding probabilities, while type C had lower proportions with higher exceeding probabilities. Type D buildings (mainly raw-soil and stone structures) were extremely rare in the six districts ().
Table 4. Proportions of building types in the six districts in central Beijing.
3.3.2. Temporal and spatial structures of the dynamic distribution of evacuees
The estimates of the number of evacuees in most location-allocation problems to date have focused on the total population recorded in census data, and consequently, the conventional shelter planning must allocate all of the population of the study area to shelters, leading to the need to build new and redundant shelters. In shelter planning for earthquake emergencies, however, it is usually unnecessary and unrealistic to build many earthquake emergency shelters because many people are either unwilling to evacuate or their buildings are basically intact or only slightly damaged. Consequently, research on the spatially heterogeneous distribution of evacuees under uncertainty is thus imperative for understanding actual evacuation activities. It is also critical to consider the dynamic diurnal variations of the urban population, especially for municipalities due to the frequent movements of the population. Thus, we will be able to begin monitoring and analyzing where and when evacuees allocate to each shelters and which evacuation route they choose based on the spatial-temporal dynamics of evacuees.
The study area can be divided into two intensities (VII and VIII in scenario B, VIII and IX in scenario A) based on the seismic intensity elliptical attenuation model. shows the population density maps of the study area for the two scenarios during nighttime and daytime. On the one hand, the distribution of evacuee density in the six central districts also shows a significant downtown-suburban-outer suburban pattern that is consistent with the associated population densities from the centre to the outer areas, especially during the daytime. On the other hand, there is a marked discrepancy in the evacuee density between the different intensity zones (VII and VIII, VIII and IX), which could be attributed to the spatial heterogeneity of both earthquake intensity and building types. Once the occurrence time, location and magnitude of an earthquake disaster are determined, the spatial-temporal dynamics of evacuees can be rapidly assessed based on our temporally dynamic population distribution model and spatially heterogeneous distribution model of evacuees.
3.3.3. Comparison of the GLA model and SLA model
Consisting with the current shelter planning of Beijing, all of the modelling results were based on the integrity of the administrative divisions. Consequently, the evacuees cannot evacuate to other districts outside their own. The associated non-inferior solutions of the multi-objective GLA model and SLA model for the six districts in central Beijing are shown in . It is not possible to improve each objective value in the alternative non-inferior solution set; consequently, the scatter plots for each scheme shown in maintain a descending order by distance and an ascending order by area. Notably, the solutions significantly differ between scenarios A and B and slightly differ between the daytime and nighttime due to the different distributions of the evacuees. A range of alternative location-allocation schemes exist that demonstrate the inherent trade-off between the conflicting objectives, which could be provided to city planners through the sets of non-inferior solutions based on the multi-objective technique. Decision makers can then apply their judgements to determine the desired balance between the conflicting objectives (Current et al. Citation1990) while simultaneously considering the differences between the different scenarios. Decision makers with sufficient budgets could select schemes with shorter total evacuation distances (at the bottom right of the plots in ) and larger shelter areas in the GLA model required in scenario A. Conversely, decision makers with limited budgets could select schemes with smaller total shelter areas (at the top left of the plots) in the SLA model, which would be a more suitable option for realistic emergency shelter planning by preferentially considering the designated shelters.
Figure 5. Non-inferior solutions for all schemes obtained using the GLA model (left) and SLA model (right) under various scenarios for the six districts in central Beijing. Each feasible scheme is represented by one point in the figure and corresponds to a particular spatial allocation result. Source: the authors.
The minimal-area and minimal-distance schemes corresponds to the conflicting objectives between the managers and the evacuees; namely, the minimal total shelter area and the minimal total evacuation distance, are usually used to demonstrate the quality of the solutions for various scenarios. and compare the spatial allocation results, and and present a further comparison of the solutions corresponding to the two schemes obtained using the two models.
Figure 6. Spatial allocation results corresponding to the minimal-area solutions obtained using the GLA model (left) and SLA model (right) for the various scenarios. The red lines are allocation lines for the designated shelters that can accommodate part of the communities for the different scenarios in the SLA model. Source: the authors.
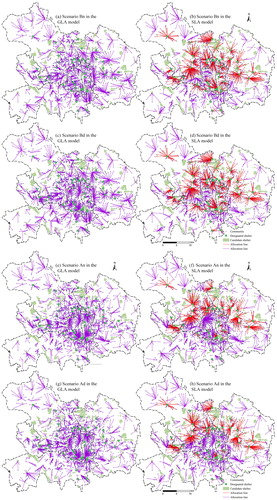
Figure 7. Spatial allocation results corresponding to the minimal-distance solutions obtained using the GLA model (the left) and SLA model (the right) for the various scenarios. The red lines are the same as Figure 6 for different scenarios. Source: the authors.
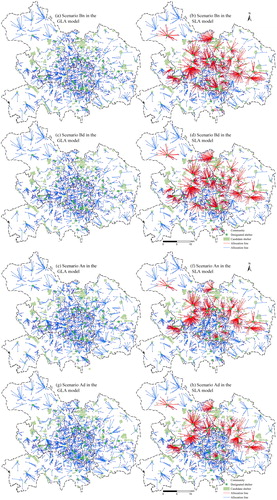
Table 5. Comparison of solutions of minimal-area scheme for the two models.
Table 6. Comparison of solutions of minimal-distance scheme for the two models.
As shown in , and , for the minimal-area scheme, the average shelter area (ASA) and the number of selected shelters (NSS) obtained using the SLA model are superior to those obtained using the GLA model, except for scenario Bn in Shijingshan and scenario Ad in Xicheng, as indicated by the dark grey entries. However, the actual ASA in the SLA model is also superior (scenario Bn in Shijingshan) or equal (scenario Ad in Xicheng) to the GLA model when the designated shelter area is added to the selected shelter area. Therefore, the solutions of the SLA model can significantly minimize the shelter area compared with the solutions of the GLA model by prioritizing the designated shelters. In addition, managers can prioritize the designated shelters to make full use of them.
As shown in , and , for the minimal-distance scheme, the average evacuation distance per person (AEDP) achieved using the SLA model is inferior to that achieved using the GLA model. The SLA model generated longer distances when the evacuees first allocated and fully utilized the designated shelters, which inevitably led to the disappearance of the distance advantage in the SLA model compared with the GLA model. Nevertheless, the solutions of the SLA model are slightly inferior to those obtained using the GLA model (scenarios Bn and Bd in Fengtai), even slightly superior to the GLA model (scenarios An and Ad of Fengtai) as indicated by the dark grey entries; because, there are only five designated shelters in the relatively large district of Fengtai. In addition, the five designated shelters with small areas play a small role in the evacuation activities, which leads to the similar solutions in the two models. To sum up, solutions of the GLA model can slightly minimize the evacuation distance compared with the solutions of the SLA model without prior consideration of the designated shelters. In addition, the ASA and NSS significantly increased to slightly decrease the AEDP in the minimal-distance scheme. Therefore, managers can select solutions for the minimal-area scheme with acceptable AEDP and minimal budgets.
4. Discussion
Complex decision problems are frequently encountered in urban planning when focusing on the location-allocation problem for earthquake emergency shelters, and these problems typically involve the consideration of a wide range of incommensurable and conflicting objectives (Coutinho-Rodrigues et al. Citation2012). Most multi-objective location-allocation models to date do not consider the currently designated shelters as a priority. During the planning for earthquake emergency shelters, however, it is usually necessary to prioritize the designated shelters to avoid wasting resources. The key features that distinguish this research from previous works consist of the supplemental location-allocation optimization model that considers the real-world spatial-temporal dynamic distribution of evacuees and its implementation in the large-scale location-allocation problem of earthquake emergency shelters. As shown in and of the spatial allocation results obtained using the SLA model, the first part evacuees evacuated to the designated shelters, and the rest of the evacuees that could not be accommodated evacuated to other candidate shelters based on the supplemental location-allocation optimization method. However, the post-selected candidate shelters may be better choices for the first part evacuees who were accommodated by the designated shelters. Consequently, the final spatial allocation results of the SLA model remained slightly irrational, as shown in and , and the minimal-distance solutions were inferior to those obtained using the GLA model, as shown in . These results were directly related to the priorities of the designated shelters.
In future studies, in addition to considering the dynamic diurnal variations of the evacuees due to building damage, the shortage of essential services (e.g., water, power) (Li et al. Citation2017) could further influence the dynamic temporal changes of the evacuees during a longer period after disasters (1 week, 1 month, etc.). Consequently, earthquake shelters could be classified as immediate, short-term, and long-term, which satisfy the different demands during the associated evacuation phases (Chen et al. Citation2013). Finally, a dynamic hierarchical supplement location-allocation optimization model could be developed to provide a basis for the phased planning of different levels of shelters in Beijing. Nevertheless, the location-allocation problem for earthquake emergency shelters is still a multidisciplinary and complicated systematic project.
5. Conclusion
This article presents a dynamic multi-objective supplemental location-allocation optimization model for earthquake emergency shelters in the case of evacuation via walking that addresses the concerns related to the designated shelters, and this model is believed to be more consistent with the knowledge of shelter planners and managers. This article also incorporates the uncertainties of the temporal dynamics of the population distribution and the spatial heterogeneity of evacuee distribution under various earthquake damage scenarios by using the elliptical attenuation model for seismic intensity into the location-allocation problem. Solutions were derived by applying a modified PSO algorithm to both the SLA model and the conventional GLA model, and the comparison of the results revealed the advantages of the SLA model over the GLA model. A case study in the central area of Beijing was presented to demonstrate the proposed methodology. The solutions of the minimal-area scheme in the SLA model distinctly minimized the shelter area compared with the GLA model by prioritizing the designated shelters. By contrast, the solutions of the minimal-distance scheme in the GLA model, without prior consideration of the designated shelters, slightly minimized the evacuation distance compared with the SLA model. Meanwhile, the ASA and NSS significantly increased to slightly decrease the AEDP in the minimal-distance scheme. Therefore, managers could select the solutions obtained for the minimal-area scheme using the SLA model with acceptable AEDP and minimal budgets by prioritizing the designated shelters. This study aims to make up for the lack of research on supplemental location-allocation planning throughout the world, substantially improve the efficiency and professionalism of disaster reduction and emergency response in China, and provide effective planning strategies and enlightenment for urban planning with respect to public safety in Beijing.
Acknowledgments
The subway fare smart card data were provided by Beihang Interest Group on SmartCity, and we are grateful for their permission to use it in this paper. We also thank the Beijing Municipal Bureau of Civil Affairs for providing access to community population data.
Disclosure statement
No potential conflict of interest was reported by the authors.
Additional information
Funding
References
- Alçada Almeida L, Tralhao L, Santos L, Coutinho Rodrigues J. 2009. A multiobjective approach to locate emergency shelters and identify evacuation routes in urban areas. Geogr Anal. 41(1):9–29.
- Barzinpour F, Esmaeili V. 2014. A multi-objective relief chain location distribution model for urban disaster management. Int J Adv Manuf Technol. 70(5–8):1291–1302.
- BBC News. Japan tsunami: Toll tops 10,000 two weeks after quake, 2011 [online]. Available from: http://www.bbc.co.uk/news/world-asia-pacific-12857544 [Accessed 2 January 2019].
- Boonmee C, Arimura M, Asada T. 2017. Facility location optimization model for emergency humanitarian logistics. Int J Disaster Risk Reduction. 24:485–498.
- Bozorgi-Amiri A, Jabalameli MS, Al-e-Hashem SM. 2013. A multi-objective robust stochastic programming model for disaster relief logistics under uncertainty. Or Spectr. 35(4):905–933.
- Bozorgi-Amiri A, Jabalameli MS, Alinaghian M, Heydari M. 2012. A modified particle swarm optimization for disaster relief logistics under uncertain environment. Int J Adv Manuf Technol. 60(1–4):357–371.
- CBS News. Powerful Typhoon Mangkhut slams into Philippines [online], 2018. Available from: https://www.cbsnews.com/news/typhoon-mangkhut-slams-into-philippines-today-2018-09-14/ [Accessed 7 January 2019].
- Chen Z, Chen X, Li Q, Chen J. 2013. The temporal hierarchy of shelters: a hierarchical location model for earthquake-shelter planning. Int J Geogr Inf Sci. 27(8):1612–1630.
- China Earthquake Administration 2015. Earthquake emergency shelters planning summary report of Beijing in 2014 [online]. Available from: http://www.cea.gov.cn/publish/dizhenj/466/499/20150714105650474725721/index.html [Accessed 2 January 2019].
- Coutinho-Rodrigues J, Tralhão L, Alçada-Almeida L. 2012. Solving a location-routing problem with a multiobjective approach: the design of urban evacuation plans. J Transp Geogr. 22:206–218.
- Cui X. 2010. The study of earthquake intensity attenuation model and earthquake shakemap theory (In Chinese). Hefei: University of Science and Technology of China.
- Current J, Min H, Schilling D. 1990. Multiobjective analysis of facility location decisions. Eur J Oper Res. 49(3):295–307.
- Deb K, Karthik S. 2007. Dynamic multi-objective optimization and decision-making using modified NSGA-II: a case study on hydro-thermal power scheduling. Paper presented at the International conference on evolutionary multi-criterion optimization, Berlin, Heidelberg.
- Ding C, Song Y, Knaap G, Huang Y. 2005. Urban planning and spatial structure: urban sustainable development strategy (In Chinese). Beijing: China Architecture & Building Press.
- Ding B, Sun J, Li X, Liu Z, Du J. 2014. Research progress and discussion of the correlation between seismic intensity and ground motion parameters (In Chinese). Earthquake Eng Eng Dyn. 34(5):7–20.
- Doerner KF, Gutjahr WJ, Nolz PC. 2009. Multi-criteria location planning for public facilities in tsunami-prone coastal areas. Or Spectr. 31(3):651–678.
- Fang W, Wang J, Shi P. 2011. Integrated risk governance: database, risk map and network platform (In Chinese). Beijing: Science Press.
- GlobalMedic. Philippines disaster preparedness storm shelter project 2015-2016 [online], 2016. Available from: https://globalmedic.ca/responses/typhoon/philippines-disaster-preparedness-storm-shelter-project-2016/ [Accessed 7 January 2019].
- Haghi M, Ghomi SMTF, Jolai F. 2017. Developing a robust multi-objective model for pre/post disaster times under uncertainty in demand and resource. J Clean Prod. 154:188–202.
- Hu F, Yang S, Xu W. 2014. A non-dominated sorting genetic algorithm for the location and districting planning of earthquake shelters. Int J Geogr Inf Sci. 28(7):1482–1501.
- Jalali S, Seifbarghy M, Sadeghi J, Ahmadi S. 2016. Optimizing a bi-objective reliable facility location problem with adapted stochastic measures using tuned-parameter multi-objective algorithms. Knowl Based Syst. 95:45–57.
- Li H, Zhao L, Huang R, Hu Q. 2017. Hierarchical earthquake shelter planning in urban areas: A case for Shanghai in China. Int J Disaster Risk Reduct. 22:431–446.
- Ma Y, Xu W, Qin L, Zhao X, Du J. 2019. Hierarchical supplement location-allocation optimization for disaster supply warehouses in the Beijing–Tianjin–Hebei region of China. Geom Nat Hazards Risk. 10(1):102–117.
- Ma Y, Xu W, Zhao X, Li Y. 2017. Modeling the hourly distribution of population at a high spatiotemporal resolution using subway smart card data: a case study in the central area of Beijing. IJGI. 6(5):128.
- Ma Y, Zhao X, Qin L, Liang P, Zhou H, Yuan Y, Xu W. 2018. Multi-objective location-allocation model for earthquake emergency shelters with multiple constraints: a case study in Wenchang of Hainan Province. J Catastrophol. 33(1):218–224. In Chinese
- Miyazaki H, Nagai M, Shibasaki R. 2015. Reviews of geospatial information technology and collaborative data delivery for disaster risk management. IJGI. 4(4):1936–1964.
- Najafi M, Eshghi K, Dullaert W. 2013. A multi-objective robust optimization model for logistics planning in the earthquake response phase. Trans Res Part E: Logist Trans Rev. 49(1):217–249.
- PRC Ministry of Construction. 2007. GB 50413-2007 Standard for urban planning on earthquake resistance and hazardous prevention (In Chinese). Beijing: China Architecture & Building Press.
- Radio New Zealand (RNZ). CNMI typhoon shelters filling up as Mangkhut approaches [online]. Available from: https://www.radionz.co.nz/international/pacific-news/366104/cnmi-typhoon-shelters-filling-up-as-mangkhut-approaches [Accessed 7 January 2019].
- Rodríguez-Espíndola O, Gaytán J. 2015. Scenario-based preparedness plan for floods. Nat Hazards. 76(2):1241–1262.
- Scheer SJ, Varela V, Eftychidis G. 2012. A generic framework for tsunami evacuation planning. Phys Chem Earth. 49:79–91.
- Shi J, Yan Q, Ge Q. 2011. An algorithm for arbitrary engineering site earthquake intensity or motion parameter using ellipsoid attenuation model (In Chinese). Inland Earthquake. 25(1):21–28.
- Trivedi A, Singh A. 2017. A hybrid multi-objective decision model for emergency shelter location-relocation projects using fuzzy analytic hierarchy process and goal programming approach. Int J Proj Manage. 35(5):827–840.
- Tzeng G, Cheng H, Huang TD. 2007. Multi-objective optimal planning for designing relief delivery systems. Trans Res Part E: Logist Trans Rev. 43(6):673–686.
- Xu W, Ma Y, Zhao X, Li Y, Qin L, Du J. 2018. A comparison of scenario-based hybrid bilevel and multi-objective location-allocation models for earthquake emergency shelters: a case study in the central area of Beijing, China. Int J Geogr Inf Sci. 32(2):236–256.
- Xu W, Zhao X, Ma Y, Li Y, Qin L, Wang Y, Du J. 2018. A multi-objective optimization based method for evaluating earthquake shelter location–allocation. Geom Nat Hazards Risk. 9(1):662–677.
- Yushimito WF, Jaller M, Ukkusuri S. 2012. A Voronoi-based heuristic algorithm for locating distribution centers in disasters. Netw Spat Econ. 12(1):21–39.
- Zhang J, Dong M, Chen FF. 2013. A bottleneck Steiner tree based multi-objective location model and intelligent optimization of emergency logistics systems. Robot Comput Integr Manuf. 29(3):48–55.
- Zhao M, Chen QW, Ma J, Cai D. 2017. Optimizing temporary rescue facility locations for large-scale urban environmental emergencies to improve public safety. J. Environ. Inf. 29(1):61–73.
- Zhao L, Wang K, Wang J. 2014. Theory and method for unban emergency shelter planning (In Chinese). Beijing, China: Science Press.
- Zhao X, Xu W, Ma Y, Hu F. 2015. Scenario-based multi-objective optimum allocation model for earthquake emergency shelters using a modified particle swarm optimization algorithm: a case study in Chaoyang district, Beijing, China. PLoS One. 10(12):e144455.
- Zhao X, Xu W, Ma Y, Qin L, Zhang J, Wang Y. 2017. Relationships between evacuation population size, earthquake emergency shelter capacity, and evacuation time. Int J Disaster Risk Sci. 8(4):457–470.
- Zhou B. 2016. Technical Progress for the division of latent focal region in the new seismic ground motion parameters zonation map of China (In Chinese). City Disaster Reduct. 3:18–23.