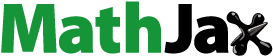
Abstract
Water inrush is a serious geological disaster in tunnel. Due to the complexity of geological conditions, large-scale water inrush is prone to occur in tunnel through water-rich fault during construction. Based on the comprehensive analysis of influencing factors, 8 evaluation indexes and the corresponding grading criteria are put forward to assess the risk of water inrush, and the risk of water inrush is divided into 4 levels. Synthesizing the standardization process and the analytic hierarchy process (AHP), a novel Cloud model is established to assess the risk of tunnel water inrush. The model is applied to the Longjinxi Tunnel to assess the risk level of water inrush. It is proved that the assessment results of the tunnel through water-rich fault is consistent with the actual situation, which verifies the reliability of evaluation model.
1. Introduction
Tunnel excavation leads to the damage to underground rock structures and the change of underground water transport network or storage conditions. Large-scale water inrush has become a serious geological hazard for tunnel construction in water-rich area. Tunnel water inrush is the dynamic process accompanied by sudden released energy at a high speed. Therefore, when tunnel water inrush occurs, groundwater will suddenly gush into the tunnel, which poses a great threat to the construction personnel and machinery (Zhang et al. Citation2014; Li, Liu, et al. Citation2015; Wu et al. Citation2017).
There are three basic conditions for the occurrence of tunnel water inrush. 1. Specific geological structure, such as karst, fault etc. Xu (Citation2011) summarized the mechanisms of the water inrush induced by the fault reactivation and analyzed the coupling interaction between the seepage field of the aquifer and the stresses field. Wu et al. (Citation2019) studied the failure modes of the rock stratum between the tunnel face and the filled karst cave (with water and with water-mud mixture) under earthquake action. 2. Abundant groundwater and its replenishment. Groundwater feeding will exert a direct influence on the development of adverse geology (Shakhin Citation2010). 3. Excavation and disturbance. The disturbance of rock stratum and rich-water structure in construction process directly induce tunnel water inrush (Verma et al. Citation2013; Li et al. Citation2016).
In order to accurately evaluate the risk of water inrush, a series of theories and methods have been applied to assess the risk of water inrush in tunnel construction. Li, Zhou, et al. (Citation2015) and Li, Lei, et al. (Citation2015) put forward an attribute synthetic evaluation system and fuzzy mathematics combined with AHP method to predict the water inrush in tunnel. Chu et al. (Citation2017) proposed the two-class fuzzy comprehensive evaluation to assess the risk of water inrush in karst tunnels. Wang, Chen, et al. Citation2019) adopted the intuitionistic fuzzy analysis to assess the risk of water inrush, which considered the subjective and objective weights of influencing factors. The above risk assessments of water inrush disaster mainly focused on karst tunnels. Fault is a universal adverse geological structure on the construction process, and its formation environment and geological structure are quite different from the karst. The large-scale water inrush disaster occurs frequently in tunnels through the water-rich fault. Hua et al. (Citation2011) established the risk assessment method of water inrush based on catastrophe progression to analyze water-inrush risk induced by fault activation. Wang et al. (Citation2012) evaluated the risk of water inrush in coal mines with a secondary fuzzy comprehensive evaluation system. Xue et al. (Citation2017) analyzed the mechanism of water and mud inrush from extensional faults, shear faults and compressive faults on the basis of the cusp catastrophe model.
The Cloud model, proposed by Li based on fuzzy set theory and probability statistics, is a mathematical tool for the study of uncertain problems (Li et al. Citation1995). It implements the transformation between a qualitative concept and its quantitative instantiations, and also embodies the fuzziness and randomness of the research object. The Cloud model has been widely used in numerous different engineering fields, such as tunnel gas outburst (Zhang et al. Citation2019), rock slope (Liu et al. Citation2014), rock burst (Liu et al. Citation2013; Zhou et al. Citation2016) etc. In this paper, synthesizing the standardization process and the analytic hierarchy process (AHP), a novel Cloud model is established. And taking 8 evaluation indexes into account, especially fault scale and fault feature, the risk assessment system of water inrush is proposed. The above model is successfully applied to assess the risk of water inrush in tunnel through water-rich fault, which provides an effective approach for risk assessment and offers a good reference for similar engineering.
2. Theoretical methods
2.1. AHP method
Analytic hierarchy process (AHP) is a method that classifies the factors related to decision making into levels of objectives, criteria and schemes (Saaty Citation1977, Citation1979, Citation2008). According to the goal of the evaluation system, it analyzes the underlying logical relationship of the study objects, and establishes the multi-level analysis model (Ohnishi and Yamanoi Citation2014).
Firstly, set up the hierarchy system containing many influencing factors. Then, the hierarchical judgment matrix can be established by the binary comparison method, as shown in EquationEquation (1)(1)
(1) .
(1)
(1)
The approximate value of the maximum eigenvalue (λmax) and the eigenvectors (ω) can be solved via EquationEquations (2)–(4).
(2)
(2)
(3)
(3)
(4)
(4)
where ωij is the weight of each factor, A is the eigenvector, λmax is the largest eigenvalue.
To avoid abnormal results, the consistency of judgment matrix should be verified with EquationEquation (5)(5)
(5) .
(5)
(5)
where n is the order number, R.I. is the random consistency index. The standard values are depicted in .
Table 1. The random consistency index.
Based on the above calculation, as C.R.<0.1, the consistency of the judgment matrix will meet the requirements. Thus, the index weights of the judgment matrix can be determined.
2.2. Cloud model
2.2.1. Definition of cloud
U(x) is presented as a quantitative universe of discourse and C is the qualitative expression in the universe of discourse. Each x in the universe of discourse U(x) can be regarded as a random realization of the qualitative expression in the universe of discourse. For each random element x, there is a random value with stabilization tendency, called the certainty degree μ(x). The variable x obeys the Gaussian distribution x∼N(Ex, En’2) and En’ obeys the Gaussian distribution En’∼N(En, He2). The calculation equation μ(x) is shown in EquationEquation (6)(6)
(6) . In the universe of discourse U(x), the set of certainty degree μ(x) with the corresponding qualitative expression C is the normal cloud and each certainty degree x is the cloud drop.
(6)
(6)
The cloud numerical characteristics can be described as the expectation (Ex), entropy (En) and hyper entropy (He). is the normal cloud diagram. Expectation (Ex) of the universe of discourse U(x) is the typical case of the qualitative concept. Entropy (En) reflects the value range of the universe of discourse corresponding to the qualitative concept. The smaller the value of entropy is, the easier the quantitative description is. The dispersion degree of cloud drops can be indicated by Hyper entropy (He), which is the uncertainty degree of En. The three characteristic numbers can accurately describe the qualitative concept and the quantitative instantiations of the study object. Thus, the conversion model between qualitative concepts and quantitative values is established.
2.2.2. Cloud generator
In Cloud model theory, the tools to implement the conversion between qualitative index and quantitative index is cloud generator, which contains the forward cloud generator and the backward one. The forward cloud generator can convert the qualitative characteristics of indexes into quantitative values. The algorithm steps are shown in (Wang et al. Citation2016).
2.3. AHP-Cloud model procedure
2.3.1. Determining index weight
The weight of evaluation index poses significant influence on the result of risk assessment, which is generally embodied in multi-factor comprehensive evaluation. The weight of each evaluation index ωij is obtained by AHP method and expert scoring, and its consistency is to be checked.
2.3.2. Standardization of the evaluation index
In order to eliminate the influence of index dimension, the value of each evaluation index should be standardized. The evaluation index can be divided into the profitability index and the attrition index. For profitability index, the larger the value is, the higher the risk is, while the smaller the attrition index is. The calculation formula is as follow (Liu et al. Citation2014).
For profitability index,
(7)
(7)
For attrition index,
(8)
(8)
where
is the standardized value of evaluation index.
2.3.3. Generating the cloud of evaluation index
Using the standardized values of indexes, the cloud numerical characteristics (Ex, En, He) of different risk levels for each index can be calculated by EquationEquation (9)(9)
(9) . In the case of unilateral constraint [
+∞) or (-∞,
), the value of (Ex, En, He) can be obtained by the determination of default parameters or expected values.
(9)
(9)
where
respectively represent the maximum standardized value and the minimum standardized value for each risk level. k is a constant which is to reflect the fuzzy threshold of assessment. Normally, k is one hundredth of the maximum value of evaluation index. Therefore, for the standardization value of the evaluation index, k is 0.01.
In the AHP-Cloud model without standardization process, the second step can be ignored and the maximum and minimum value for each risk level Imax & Imin can substitute the corresponding variables in EquationEquation (9)(9)
(9) .
2.3.4. Generating the comprehensive cloud
Synthesizing the cloud numerical characteristics and the weight of each index, the cloud numerical characteristics of different risk levels can be calculated by EquationEquation (10)(10)
(10) .
(10)
(10)
2.3.5. Application assessment
To analyze a specific project, several typical samples for the objects should be selected. The certainty degree of different risk levels in the sample can be calculated by EquationEquations (6)(6)
(6) and Equation(11)
(11)
(11) . Meanwhile, the cloud numerical characteristics of each evaluation index for all samples can be obtained. Combined with the weight, the numerical characteristics of the comprehensive Cloud model and the cloud diagram are obtained by EquationEquation (12)
(12)
(12) (Zhou et al. Citation2016).
(11)
(11)
(12)
(12)
3. Risk level and factors of water inrush
3.1. Risk level of water inrush
Due to the complex geological conditions, there are many uncertain factors to induce high risks in tunnel construction. Three purposes to establish risk level of water inrush are shown as follows.
Enhance the safety awareness to prevent disaster, strengthen the construction supervision and ensure the safety of construction.
Enhance the monitoring measurement during the construction period and adjust the construction scheme in time.
Reduce the risk of tunnel construction and improve the ability to deal with emergencies.
In order to guide the construction effectively, it’s unfavorable to set overmuch risk levels. Thus, it’s deemed that the risk level of water inrush in tunnel through water-rich fault is consisted of 4 levels, green, yellow, orange and red. In Green level, there is no risk of tunnel water inrush, and the construction can be carried out according to the established tunnel design and construction scheme. The yellow level is for low risk of water inrush in tunnel. In this situation, tunnel construction can proceed normally, while the frequency of monitoring measurement needs to be strengthened. The orange level is on behalf of moderate chance for water inrush in tunnel. Grouting & blocking water and real-time monitoring measurement are essential during construction. The red level means high-risk. A large scale of water inrush in tunnel may occur and the adequate measures must be taken to ensure tunnel stability.
3.2. Evaluation index
Tunnel water inrush is a dynamic phenomenon formed by changing the transport network or storage conditions of underground water under external interference during the tunnel construction. The occurrence of water inrush is affected by many factors, and the main factors can be divided into engineering geological condition (I1), hydrologic geological conditions (I2) and tunneling conditions (I3).
3.2.1. Engineering geological condition
The stratum lithologies (I11) on both sides of faults determine the lithology & structure of fault fillings, the fault width and fragmentation degree. And it also affects the water conductivity and water abundance of fault. Ma et al. (Citation2009) studied the classification and characteristics of the stratum lithologies on both sides of faults and put forward the quantification classification standard.
Strata occurrence (I12) is an important factor affecting the groundwater flow. The permeability coefficient along the rock layer is much higher than that in the vertical layer. Different strata inclinations lead to the change of catchment area, which directly affects the seepage of groundwater (Li et al. Citation2013). Zhou et al. (Citation2013) adopted the modified strata inclination as the representative evaluation index of strata occurrence and raised the corresponding classification standard.
The fault scale (I13) and fault feature (I14) are direct influencing factors for water inrush in tunnel through water-rich fault. The fault width and fault dip are respectively important indexes to reflect the scale and feature of faults. Zhu et al. (Citation2018) studied the influence of fault width and fault dip on seepage velocity of rock mass. Ma et al. (Citation2009) proposed the grading standard of fault width.
3.2.2. Hydrologic geological conditions
The storage condition of groundwater determines the material source of water inrush (Shi et al. Citation2017; Xu et al. Citation2018). As faults and karsts are filled with water, water inrush disaster is likely to occur in tunnels through water-rich fault.
The extent of water inrush in tunnel through water-rich fault is mainly affected by the richness and supply of fault water. Therefore, the main influencing factors are groundwater level (I21) and groundwater replenishment (I22). The corresponding evaluation index for groundwater level is the altitude difference between the groundwater level and the tunnel floor. There are many parameters can reflect the degree of groundwater recharge. Among which, the monitoring of water pressure in tunnel construction is relatively simple, so water pressure is selected as the evaluation parameter to reflect groundwater recharge, which is beneficial to the engineering application of the evaluation standard system (Peng et al. Citation2016; Wang, Li, et al. Citation2019).
3.2.3. Tunneling conditions
The disturbance of rock stratum and rich-water structure in construction is the direct cause of tunnel water inrush (Verma and Singh Citation2010). The main influencing factors determined by tunneling conditions are tunnel excavation (I31) and advance geological forecast (I32). In the risk assessment of tunnel water inrush, the excavation span generally acts as an evaluation index of tunneling conditions (Li and Wu Citation2019). The advanced geological forecast methods for groundwater in tunnel construction include advanced drilling, infrared water exploration, TSP and so on. Among which, advanced drilling is the most intuitive way to reveal water inrush in tunnel. In order to obtain the quantitative criteria, the water jet distance of advancing hole is also selected as an evaluation index (Peng et al. Citation2016).
Combined with water inrush classification of tunnel, the evaluation indexes and the corresponding grading criterion can be acquired, as shown in .
Table 2. Evaluation indexes and grading criterion of water inrush.
4. Risk assessment model of water inrush
4.1. Risk evaluation index and weight
Based on the above analysis, the evaluation index system of water inrush can be established. By evaluating the significances of the indexes, the judgment matrixes of indexes weights can be acquired with expert scoring method. Results show that C.R. of the matrixes are less than 0.10, which illuminates that the matrixes have favorable consistencies. Thus, the comprehensive weights of the indexes can be sorted as .
Table 3. Comprehensive weights of the indexes.
4.2. Cloud model of water inrush
The evaluation indexes in are all profitability indexes. Therefore, the standardized values of the indexes can be calculated by EquationEquation (7)(7)
(7) . Then the cloud numerical characteristics (Ex, En, He) of different risk levels for the above indexes can be calculated by EquationEquation (9)
(9)
(9) , and the results are shown in .
Table 4. Cloud numerical characteristics for each evaluation index.
4.3. Comprehensive risk assessment model
Risk level of water inrush is affected by the above influencing factors and evaluation indexes. According to the cloud numerical characteristics and the weight of each index, the numerical characteristics of the comprehensive Cloud model corresponding to different levels can be obtained by EquationEquation (10)(10)
(10) , and the results are as follows, Green level (Ex=0.160, En=0.035, He=0.01), Yellow level (Ex=0.389, En=0.042, He=0.01), Orange level (Ex=0.623, En=0.062, He=0.01), Red level (Ex=0.925, En=0.067, He=0.01). The comprehensive evaluation cloud diagram is shown in .
5. Engineering applications
5.1. Project overview
The Longjinxi Tunnel is one of the dominant project of Zhangzhou diversion project, which is located in Fujian Province, China. The diversion tunnel is in aggregate 13.8 km in length. The maximum buried depth is about 312 m. The strata exposed along the tunnel are mainly Yanshanian coarse granite, granodiorite and Triassic quartz fine sandstone with argillaceous siltstone. In this paper, the coarse granites invaded in the late Yanshanian period are mainly distributed in the study area, and the distribution range is more than 5 km. The buried depth of the groundwater level is generally 5 ∼ 15 m and the main part of the tunnel is below the underground water level. Due to the influence of the regional geological structure, along with the diversion tunnel, the fault tectonics is well developed. The long fault cut the rock mass from the deep to the surface, and trenches and streams are formed on the surface, which leads to the rich water terrain near the fault.
There are many faults in the tunnel, and the region of tunnel through the faults can be divided into 4 sections. The K4 + 646∼K5 + 515 section, the K5 + 515∼K6 + 490 section, the K6 + 490∼K7 + 336 section and the K6 + 336∼K7 + 910 section are respectively in the influence region of the No. F46 fault, the No. F45 fault, the No. F63 fault, and the No. F56 fault. The monitoring or experimental data of the 4 sections are collected in .
Table 5. Average measured value of evaluation index.
5.2. Risk level assessment
The data of evaluation index in each section are standardized. The forward cloud generator is employed to calculate the certainty degree of each evaluation index in different risk levels, as shown in . Combining with the weight, the synthetic certainty degree is obtained by EquationEquation (11)(11)
(11) . The numerical characteristics of comprehensive Cloud model for Longjinxi Tunnel is (Ex=0.581, En=0.096, He=0.01) and the comprehensive evaluation cloud diagram is shown in .
Table 6. Risk level of water inrush in Longjinxi Tunnel.
According to the principle of maximum certainty degree, the risk levels for the sections of K4 + 646∼K5 + 515 and K6 + 490∼K7 + 336 are orange, and that for the section of K5 + 515∼K6 + 490 is yellow, while the value of μ(Orange) is relatively large, which indicates that it has a tendency to develop into a higher risk level. The cloud drops concentrate mainly on the orange level, and some of the cloud drops are in the region of red level, as shown in . It indicates that water inrush in Longjinxi Tunnel has reached a relatively high risk level, and some sections are at risk of the occurrence of water inrush on a large scale.
5.3. Field verification
According to the statistics of the geological hazards occurring during tunnel construction, the specific situations of water inrush in Longjinxi Tunnel at the research section are shown in , and the scenes in field are shown in and . It is apparent that Longjinxi Tunnel is at a high risk and the assessment results of each section comply with the actual situations.
Table 7. Geological disaster statistics of Longjinxi Tunnel.
6. Discussion
The standardization process and the AHP method are employed to establish the novel Cloud model for evaluating water inrush risk in tunnel through water-rich fault. In the researches of risk assessment based on Cloud model, the standardization of evaluation index is not a necessary process, and these researches have also obtained well evaluation and prediction results (Liu et al. Citation2013; Wang, Li, et al. Citation2019). To analyze the validity of the standardization process on the assessment results, the AHP-Cloud method without standardization process, namely without standardization process of evaluation index in section3.2, is adopted to evaluate the water inrush risk in Longjinxi Tunnel.
The assessment results of the AHP-Cloud model with & without standardization process are shown in , and the comprehensive evaluation cloud of AHP-Cloud model without standardization process is shown in .
Table 8. Assessment results of the AHP-Cloud model with & without standardization process.
In sections of K4 + 646∼K5 + 515, K5 + 515∼K6 + 490 and K6 + 490∼K7 + 336, the risk levels of water inrush respectively assessed by the AHP-Cloud model with & without standardization process are consistent, while the results for the section of K7 + 336∼K8 + 110 are not the same. For the section of K7 + 336∼K8 + 110, the risk level derived from the AHP-Cloud model without standardization process is Orange, and the risk level with standardization process is Red. In actual construction, water inrush on a large scale appears in this section, which indicates the accuracy of the result with standardization process. As seen from and , the cloud drops of the above two methods are concentrated around the orange level. The cloud drops of the two methods concentrate mainly on the Orange level, and some of the cloud drops with standardization process are in the high risk region, which indicates that these sections are at risk of the occurrence of water inrush on a large scale. Compared with the standardization process, the cloud drops without standardization process in these sections doesn’t reveal the risk of large-scale water inrush.
Comparing the assessment results of the two methods, the results without standardization process might be inaccurate in some cases. Therefore, the standardization process is an essential component for the AHP-Cloud model, which can effectively improve the accuracy of the assessment results.
7. Conclusion
Water inrush has become a serious geological hazard in tunnel through water-rich fault. To comprehensively take the complex and unpredictable engineering geology into consideration, several influencing factors are selected to assess the risk level of water inrush. And the evaluation index system and the corresponding grading criterion are established.
The standardization process and the AHP method are employed to establish the novel Cloud model for evaluating water inrush risk in tunnel through water-rich fault. The AHP method effectively improves the accuracy of weight calculation, and the standardization process makes it possible to make a comparison among the evaluation indexes with different units.
In order to verify the credibility of AHP-Cloud method, it is applied to assess the risk of water inrush in Longjinxi Tunnel. It is proved that the assessment results of the tunnel through water-rich fault is consistent with the actual situation.
Comparing the assessment results respectively derives from the AHP-Cloud method with and without standardization process, the results without standardization process might be inaccurate in some cases. Therefore, the standardization process is an essential component for the AHP-Cloud model, which can effectively improve the accuracy of the assessment results.
Supplemental Material
Download MS Word (734 KB)Disclosure statement
No potential conflict of interest was reported by the authors.
Additional information
Funding
References
- Chu H, Xu G, Yasufuku N, Yu Z, Liu P, Wang J. 2017. Risk assessment of water inrush in karst tunnels based on two-class fuzzy comprehensive evaluation method. Arab J Geosci. 10(7):179.
- Hua XG, Zhang WQ, Jiao DZ. 2011. Assessment method of water-inrush risk induced by fault activation and its application research. Procedia Engineering. 26:441–448
- Li DY, Meng HJ, Shi XM. 1995. Membership clouds and membership cloud generators. J Comput Res Dev. 32(6):15–20.
- Li LP, Zhou ZQ, Li SC, Xue YG, Xu ZH, Shi SS. 2015. An attribute synthetic evaluation system for risk assessment of floor water inrush in coal mines. Mine Water Environ. 34(3):288–294.
- Li LP, Lei T, Li S, Zhang Q, Xu Z, Shi S, Zhou Z. 2015. Risk assessment of water inrush in karst tunnels and software development. Arab J Geosci. 8(4):1843–1854.
- Li LP, Tu WF, Shi SS, Chen JX, Zhang YH. 2016. Mechanism of water inrush in tunnel construction in karst area. Geomat Nat Haz Risk. 7(sup1):35–46.
- Li SC, Zhou ZQ, Li LP, Xu ZH, Zhang QQ, Shi SS. 2013. Risk assessment of water inrush in karst tunnels based on attribute synthetic evaluation system. Tunn Undergr Sp Tech. 38:50–58.
- Li SC, Liu B, Nie LC, Liu ZY, Tian MZ, Wang SR, Su MX, Guo Q. 2015. Detecting and monitoring of water inrush in tunnels and coal mines using direct current resistivity method: a review. J Rock Mech Geotechn Eng. 7(4):469–478.
- Li SC, Wu J. 2019. A multi-factor comprehensive risk assessment method of karst tunnels and its engineering application. Bull Eng Geol Environ. 78:1761–1776.
- Liu ZB, Shao JF, Xu WY, Meng YD. 2013. Prediction of rock burst classification using the technique of cloud models with attribution weight. Nat Hazards. 68(2):549–568.
- Liu ZB, Shao JF, Xu WY, Xu F. 2014. Comprehensive stability evaluation of rock slope using the cloud model-based approach. Rock Mech Rock Eng. 47(6):2239–2252.
- Ma SW, Mei ZR, Zhang JW. 2009. Geologic structural quantitative evaluation of abrupt geologic hazards for long and large tunnels. Mod Tunnelling Technol. 46(2):99–104 (in Chinese).
- Ohnishi SI, Yamanoi T. 2014. On fuzzy priority weights of AHP for double inner dependence structure. Proc Comput Sci. 35:1003–1012.
- Peng YX, Wu L, Su Y, Zhou RF. 2016. Risk prediction of tunnel water or mud inrush based on disaster forewarning grading. Geotech Geol Eng. 34(6):1923–1932.
- Saaty TL. 1977. A scaling method for priorities in hierarchical structures. J Math Psychol. 15(3):234–281.
- Saaty TL. 1979. Applications of analytical hierarchies. Math Comput Simuin. 21(1):1–20.
- Saaty TL. 2008. Decision making for leaders: the analytic hierarchy process for decisions in a complex world. Pittsburgh (PA): RWS Publications. ISBN 0-9620317-8-X.
- Shakhin RS. 2010. Hydrodynamic conditions and karst formation in the northwest part of Syria. Moscow Univ Geol Bull. 65(4):263–264.
- Shi SS, Bu L, Li SC, Xiong ZM, Xie XK, Li LP, Zhou ZQ, Xu ZH, Ma D. 2017. Application of comprehensive prediction method of water inrush hazards induced by unfavourable geological body in high risk karst tunnel: a case study. Geomat Nat Haz Risk. 8(2):1407–1423.
- Verma AK, Roy S, Gautam PK. 2013. Estimation of groundwater seepage rate into Maneri-Uttarkashi power tunnel: an analytical approach. Int. J Earth Sci Eng. 6:1429–1433
- Verma AK, Singh TN. 2010. Assessment of tunnel instability - a numerical approach. Arab J Geosci. 3(2):181–192.
- Wang XT, Li SC, Xu ZH, Hu J, Pan DD, Xue YG. 2019. Risk assessment of water inrush in karst tunnels excavation based on normal cloud model. Bull Eng Geol Environ. 78(5):3783–3798.
- Wang Y, Yang WF, Li M, Liu X. 2012. Risk assessment of floor water inrush in coal mines based on secondary fuzzy comprehensive evaluation. Int J Rock Mech Min. 52:50–55.
- Wang YC, Yin X, Jing HW, Liu RC. Su HJ. 2016. A novel cloud model for risk analysis of water inrush in karst tunnels. Environ Earth Sci. 75:1450.
- Wang YC, Chen F, Yin X, Geng F. 2019. Study on the risk assessment of water inrush in karst tunnels based on intuitionistic fuzzy theory. Geomat Nat Haz Risk. 10(1):1070–1083.
- Wu J, Li S. C, Xu Z. H, Huang X, Xue Y. G, Wang Z. C, Li L. P. 2017. Flow characteristics and escape-route optimization afterwater inrush in a backward-excavated Karst Tunnel. Int J Geomech. 17(4): 4016096.
- Wu J, Li SC, Xu ZH, Zhao J. 2019. Determination of required rock thickness to resist water and mud inrush from karst caves under earthquake action. Tunn Undergr Sp Tech. 85:43–55.
- Xu DJ. 2011. Advances in the research on mechanisms of the groundwater inrush caused by the fault reactivation in coalmines. Procedia Eng. 26:824–831.
- Xu ZH, Wu J, Li SC, Zhang B, Huang X. 2018. Semianalytical solution to determine minimum safety thickness of rock resisting water inrush from filling-type karst caves. Int J Geomech. 18(2):4017152.
- Xue Y, Wang D, Li S, Qiu D, Li Z, Zhu J. 2017. A risk prediction method for water or mud inrush from water-bearing faults in subsea tunnel based on cusp catastrophe model. KSCE J Civ Eng. 21(7):2607–2614.
- Zhang D, Fang Q, Lou H. 2014. Grouting techniques for the unfavorable geological conditions of Xiang’an subsea tunnel in China. J Rock Mech Geotechn Eng. 6(5):438–446.
- Zhang K, Zheng WB, Xu C, Chen SG. 2019. Risk assessment of gas outburst in tunnels in non-coal formation based on the attribute mathematical theory. Geomat Nat Haz Risk. 10(1):483–504.
- Zhu BB, Wu L, Peng YX, Zhou WW, Chen CH. 2018. Risk assessment of water inrush in tunnel through water-rich fault. Geotech Geol Eng. 36(1):317–326.
- Zhou KP, Lin Y, Deng HW, Li JL, Liu CJ. 2016. Prediction of rock burst classification using cloud model with entropy weight. T Nonferr Metal Soc. 26(7):1995–2002.
- Zhou ZQ, Li SC, Li LP, Shi SS, Song SG, Wang K. 2013. Attribute recognition model of fatalness assessment of water inrush in karst tunnels and its application. Rock Soil Mech. 34(3):818–826 (in Chinese).