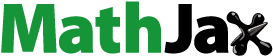
Abstract
Tropical cyclones wreak havoc on the lives of coastal populations, especially in marginalised communities in developing countries where cyclone impacts are disproportionately lethal compared to developed countries. These populations must implement measures that enable them to prepare for, respond to, and recover from cyclone impacts. Using empirical evidence, this study presents a spatio-temporally explicit, index-based assessment technique to assess social resilience to tropical cyclones. The key components of social resilience were extracted by Principal Component Analysis; distinct resilience indices were generated and mapped for the three disaster phases. The most influential components were then aggregated into an Effective Social Resilience Index (ESoRI), which was mapped across the study area. The technique was applied in a coastal sub-district of Bangladesh to demonstrate the approach. Basic preparedness training, emergency response, social bonding, and reconstruction and rehabilitation strongly influenced resilience in ways that corresponded closely to the phases of the disaster-management cycle: preparedness, response, and recovery. The spatial pattern of resilience revealed that social resilience varies significantly in time and space within a circumscribed locale. Importantly, areas close to the sea and rivers were found to be more resilient despite their high exposure to cyclones and lack of external support. This study will guide policymakers concerned with resilience building on a priority basis such as those working in resource-scarce conditions of developing countries.
1. Introduction
Social resilience is an intrinsic feature of a society that stems from human perceptions, behaviors and characteristics, and provides people with the capacity to withstand a disaster and recover from its long-enduring impacts, thus maintaining the functioning of the social system (Adger Citation2000). This concept has gained increasing importance among the scientific community and policymakers, who are often seeking a holistic strategy to alleviate adverse impacts of frequently occurring natural disasters (Aldunce et al. Citation2015; Cox and Hamlen Citation2015). Social scientists see social resilience as a ‘naturally emergent' response to disasters (Pelling Citation2003). Disaster scholars are of the view that management strategies for potential disasters must recognize and build on the underlying capacities of communities (Lorenz Citation2013). It is therefore imperative to measure social resilience within a community in order to develop plans that build on these social capacities to mitigate current and future disaster risks to which the community is susceptible (Aitsi-Selmi et al. Citation2015).
Tropical cyclones, a devastating and regularly occurring natural phenomenon, are known to be worsened by climate change, and have caused significant damage to the environment and human society in many locations (IPCC Citation2014). A total of 1,712 hazardous cyclones were recorded worldwide during the last three decades, causing substantial economic losses worth ∼1.15 trillion $USD along with ∼1.35 million fatalities (CRED Citation2020). The threats associated with cyclones and their possible impacts differ greatly depending on the geographical location, degree of population concentration, and the socio-economic status of the area they strike and, most importantly, the intensity and magnitude of the hazard itself (Torresan et al. Citation2008). Cyclone impacts are greatest in low-lying coastal regions (Adger et al. Citation2005). A common impact is the destruction of livelihoods and the depletion of coastal resources (Akter and Mallick Citation2013). Communities that are hit repeatedly by cyclones become socially isolated and their internal cohesion is often disrupted (Islam and Walkerden Citation2014). Post-disaster traumatic stress is often reported among cyclone-affected communities (Hasan et al. Citation2020). Nonetheless, despite knowing that disaster risk exists, in many developing countries large populations seek to live in coastal regions because of their proximity to coastal resources that support their livelihoods (Ahsan et al. Citation2017; Huq et al. Citation2020).
Bangladesh, a South Asian developing country, is hit frequently by several hazards, including tropical cyclones. Cyclones jeopardize the large population (approximately 44 million) residing in the coastal districts of the country (Paul et al. Citation2010). Over the last three decades, coastal Bangladesh has witnessed a total of 49 tropical cyclones, the most severe of which were Gorky (1991), Sidr (2007), Aila (2013), and Mahasen (2014); they each profoundly compromised human wellbeing. In addition, Cyclones like Odisha (1999), Nargis (2008), Hudhud (2014), and Bulbul (2019), which passed over the coasts of India and Myanmar, also severely impacted the Bangladeshi coast. Global disaster statistics show that these cyclones were responsible for killing ∼0.2 million people and caused economic losses approaching ∼44 billion $USD (CRED Citation2020) in the areas surrounding the Bay of Bengal. Recently, in 2020, an extra-severe tropical cyclone named Amphan struck West Bengal in India and touched the western portion of the Bangladeshi coast near the Sundarbans.
Although it has a high level of exposure to tropical cyclones, Bangladesh has achieved significant success in minimizing deaths from cyclonic hazards through substantial improvements in hazard forecasting and evacuation systems (Paul Citation2009, Citation2012). For instance, in 2007, the category 4 Cyclone Sidr took 3,406 human lives, far fewer than the number of fatalities (140,000) caused by the similarly sized Cyclone Gorky, which struck Bangladesh’s coast in 1991 (Paul Citation2009). Despite the country’s success in reducing fatalities, cyclonic impacts have the potential to disrupt the normal functioning of societies, impede ongoing development activities, and greatly slow economic activities in the country (Akter and Mallick Citation2013). One estimate predicts that poverty would increase up to 15% by 2030 in the coastal region of Bangladesh due to climate change and associated hazards (IPCC Citation2014). Cyclonic impacts also pose major challenges for other sectors of growth like livelihoods, health, and food security (Carabine et al. Citation2014).
Realizing the importance of community capacity for mitigating the inevitable shocks from disasters before, during, and after they strike, the Sendai Framework for Disaster Risk Reduction (2015–2030) was established, and resilience building features front and center in this strategy (Kelman Citation2015; Murphy et al. Citation2018). This framework sets ambitious targets of concurrently solving humanitarian crises and advancing development activities among the world’s most vulnerable communities, who were already grappling with multiple, persistent stresses. The framework also claims that community engagement can lead to more equitable and sustainable disaster management decisions, because communities are best informed about their own needs and aspirations and are the first responders to disaster events. With the increasing risk of disasters in developing countries like Bangladesh, disaster resilience at the community level is more important than ever (Habiba et al. Citation2013). Although measures for community-based disaster risk reduction are practiced in Bangladesh, a holistic framework for assessing social resilience is yet to be developed, which may enhance the effectiveness of prevailing disaster risk reduction strategies.
Research directed to understanding tropical cyclones and their impacts is wide ranging. Many studies have been devoted to gaining a clear understanding of the processes of surge intensification and wind movement associated with tropical cyclones using spatial modeling and simulation techniques (Murty et al. Citation2016; Singh et al. Citation2019; Sahoo and Bhaskaran Citation2019a; Citation2019b). Other studies have variously focused on risk and vulnerability assessment related to tropical cyclones using geospatial techniques (Quader et al. Citation2017; Hoque et al. Citation2018, Citation2019; Mullick et al. Citation2019; Alam et al. Citation2020), cyclone resilience (Islam et al. Citation2020), relationships between meteorological parameters and cyclone genesis (Kundu et al. Citation2001), and physical changes on land and in oceans during cyclones (Chauhan et al. Citation2018).
Much work has also been done on social resilience. Due to its unseen nature and the complexity of measuring it, certain challenges remain in formulating a robust measurement technique for social resilience. The way in which the concept of social resilience is understood and applied across various coastal settings is related to how it is assessed, and there is no widely agreed, clear definition of the concept (Saja et al. Citation2019).
Nevertheless, to date, a substantial number of studies have attempted to assess social resilience in coastal regions using both quantitative and qualitative methods. Quantitative studies generally combine several indicators from empirical data into an index with numerical values to measure social resilience. For instance, Orencio and Fujii (Citation2013) introduced an index of community resilience at the local scale in the Philippines, drawing on stakeholders’ responses to questions about various social factors (e.g., livelihoods, social protection, and disaster planning). Kafle (Citation2012) developed an index to measure community resilience for multiple communities in Indonesia using both process-based indicators (community-based interventions to mitigate risk) and outcome-based indicators (achievement of the priorities outlined in the Hyogo Framework for Action (HFA)). Lam et al. (Citation2015) proposed a Resilience Inference Measurement (RIM) model at the regional scale that integrated vulnerability (exposure and damage) and resilience (adaptive capacity). They used socio-economic characteristics as proxies, such as per-capita damage and population growth, representing vulnerability and resilience/recovery, respectively. In contrast, qualitative assessment techniques have focused mostly on a particular dimension of community resilience, intending to obtain a deep understanding of resilience for a specific site or a specific group of people. When proceeding with qualitative approaches, researchers have considered responses from at-risk populations such as risk perceptions and learning experiences (Matin and Taylor Citation2015; Adelekan and Asiyanbi Citation2016; Frausto et al. Citation2016); human interventions like coping and adaptation practices (Paton et al. Citation2008; Uy et al. Citation2011; Bennett et al. Citation2014; Kokorsch and Benediktsson Citation2018); social networks/cohesion (Islam and Walkerden Citation2014) and vulnerability reduction strategies (Schwarz et al. Citation2011; Flood and Schechtman Citation2014); institutional roles and responsibilities, such as perception of risk by diverse stakeholders (Singh and Chudasama Citation2017) and drivers of resilience (Kwok et al. Citation2016); formulating plans to integrate resilience into mainstream policies (Amuzu et al. Citation2018); disaster governance in the lens of resilience (Jozaei and Mitchell Citation2018); and social networks (Forgette et al. Citation2009).
In the Bangladeshi context, most research on social resilience has been qualitative in nature, with a focus on a single dimension of resilience (Ray-Bennett et al. Citation2010; Rahman Citation2012; Akter and Mallick Citation2013; Islam and Walkerden Citation2014; Ahmed et al. Citation2016; Ayeb-Karlsson et al. Citation2016; Islam et al. Citation2017; Sikder and Higgins Citation2017). Among the available studies, an inductive approach using secondary data is common, while the use of primary empirical data is uncommon. There is a distinct trend in resilience assessments towards using a multi-hazard approach instead of focusing on a single hazard. In addition, resilience assessment frameworks do not usually consider the temporal patterning of resilience.
To address these incongruities and to understand the temporal and spatial patterning of resilience, the objective of this research was to develop a comprehensive approach to assessing social resilience and test it in a coastal sub-district of Bangladesh that has been impacted frequently by tropical cyclones. In this study resilience is understood to be the ability of coastal residents to prepare for, respond to, and recover from a cyclone disaster using their own knowledge, skills, and resources. We used Cyclone Sidr as the basis of our resilience assessment because it was the most recent devastating cyclone to hit the study area.
To achieve the objective of the research, the following research questions were formulated:
How does the social structure of a coastal environment and behavior of the coastal residents contribute to their resilience against tropical cyclones in the three distinct phases of the disaster event (pre, syn and post); and
How does resilience vary spatially within a local area?
Our approach represents an advancement over traditional approaches for assessing social resilience and offers great benefit to coastal administrators for building resilient communities through the best and most comprehensive utilization of limited resources.
The remainder of this manuscript is organized in the following sections. In the Materials and methods section we present a description of the study area in terms of its socioeconomic characteristics. A rationale is given for choosing this area prior to a brief methodological overview and explanation of data collection processes. The analysis and analytical techniques adopted for index calculation are explained. A detailed results section follows, including the identification of factors that influenced resilience during the three disaster phases and their spatial distribution across the study area. We provide a detailed explanation of these results in the discussion section and consider the practical implications of our study while flagging some limitations and future directions. Finally, we briefly summarise our study in a concluding section.
2 Materials and methodology
2.1. Study site
The study area, Kalapara, is located between 21°48'N to 22°05'N latitude and 90°05'E to 90°20'E longitude. It is a sub-district (upazila) of Patuakhali District, situated in the southwestern coastal region of Bangladesh. Kalapara is bounded by the Bay of Bengal in the south and two major rivers, the Rabnabad River in the east and the Andharmanik River in the west (). The upazila consists of eleven unions (4th administrative tier) and 239 villages. Of the unions, Lata Chapli, Dhulasar, Lalua, Baliatali, Dhankhali, and Mohipur are located in the vicinity of the Bay of Bengal and are also close to the two big rivers passing through either side of the study area; these are the unions most exposed to tropical cyclones, with Lata Chapli and Dhulashar being highly exposed because of their long coastlines along the Bay of Bengal. This region is inhabited mostly by fishing communities. Dalbuganj, Nilganj, Mithaganj, Tiakhali, and Chakamaiya unions are less exposed to cyclones because of their distance from both the sea and the rivers. Tiakhali and Nilganj are the administrative centers where most of the social elites reside. The total population of Kalapara in 2017 was around 238,000, with a density of 484 persons per km2 (BBS Citation2017). The rationale behind selecting this area for testing our approach was that tropical cyclones frequently hit this region and cause massive devastation due to its geographic location and fragile socio-economic condition. Two of the worst tropical cyclones to have hit Bangladesh in recent times, Sidr (2007) and Aila (2009), heavily affected the region. Cyclone Sidr struck on 15 November 2007, with wind speeds of 220–240 km/h and a storm surge of 6–8 m; it caused severe damage and claimed 3,406 lives (Paul et al. Citation2010).
Figure 1. Location map of the study area. Source: GIS shapefiles (BBS Citation2017) and SRTM DEM30 (https://earthexplorer.usgs.gov/).
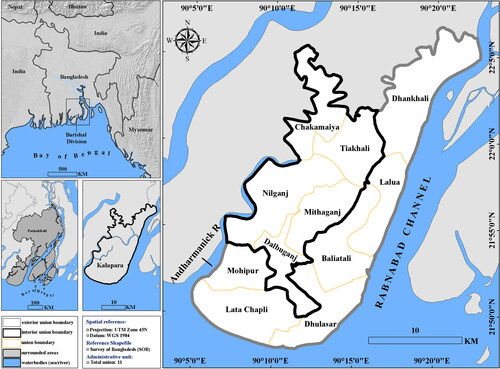
2.2. Overview of the assessment method
is a generalized flowchart showing the methodological approach adopted for this study. The primary aim was to produce distinct social resilience indices for each of the three different phases (pre, syn and post) of a cyclone disaster, then generate a composite index by combining the most influential variables from the three phases. Social resilience was quantified using Principal Component Analysis (PCA) using a subset of variables derived from questionnaire surveys. In order to represent the indices in a spatially explicit way, they were mapped using ArcGIS (version 10.4).
2.3. Questionnaire design and rationale for selecting variables
Various social characteristics of coastal communities were used to measure resilience against a cyclone disaster. Guided by existing literature (Adger et al. Citation2005; Mayunga Citation2007; Cutter et al. Citation2008; Norris et al. Citation2008; Longstaff et al. Citation2010; Islam and Walkerden Citation2014; Cutter Citation2016; Yoon et al. Citation2016), together with prior knowledge of the study area, a structured questionnaire was prepared (Supplementary material A), tested, and subsequently approved by the University of New South Wales (UNSW) Ethics Panel (ID: HC180105). Variables that captured the typical lifestyles of community members and disaster-management interventions within the community were included in the questionnaire under five dimensions, demography, livelihood, social cohesion, institutional efficacy, and economy. These essential dimensions are frequently used by researchers to determine the intrinsic social capacities of communities to cope with disasters in both local and global contexts. The rationale for our choice of specific variables is presented in Supplementary material B.
Demography refers to human characteristics such as age, education, health, and housing. Demographic variables can be used to infer self-efficacy for dealing with disaster impacts and are thus indicative of resilience. For example, education instills awareness of disasters and augments a person’s understanding of disaster risk, by virtue of which s/he can act intelligently in times of disaster and save other community members (Hoffmann and Muttarak Citation2017).
Livelihood typically refers to the means through which people earn wages to provide for their basic necessities (Chambers and Conway Citation1992). The availability of diverse livelihood options within a community generally signifies resilience. For example, in places with diverse livelihoods, households can achieve uninterrupted earnings throughout the year even if one occupation is impaired by natural hazards. Moreover, additional technical skills, if present amongst community members, may contribute significantly to meeting their daily needs during the post-disaster recovery phase and thus have a positive impact on their well-being.
Social cohesion refers to various aspects of relationships (such as bonding and bridging, norms, and trust) that facilitate self-organization within a community through coordination and cooperation (Putnam Citation1995). Societies having such relationships among their members are believed to be more resilient (Mayunga Citation2007).
Institutional efficacy refers to the involvement of various formal (government, NGOs, and private) and informal (farmers’, fishermen’s, and businessmen’s associations) organizations in building the capacity of social systems to deal with disaster impacts (Norris et al. Citation2008). For instance, people who are marginalized in everyday life are usually unable to cope with the adverse effects of hazards such as tropical cyclones, and they rely heavily on these organizations’ response-oriented support, like evacuation and rescue, and for provision of relief, shelter, and medical support for survival.
Economic status greatly influences human decision-making processes and that also applies to disaster management activities (Mayunga Citation2007; Yoon et al. Citation2016). Generally, the greater the economic capacity of a household, the more resilient it is. For example, financially solvent households can spend money on various measures to safeguard their homes from disasters such as building the house on elevated land or making the house more disaster resilient by using durable construction materials.
2.4. Data collection
This study relied on primary data acquired through an extensive household survey. The required sample size was estimated to be 435 from a total of 57,286 households in the study area, based on the WHO (Citation2005) sample-size calculation tool. A field team consisting of ten persons, all having prior experience with survey administration, conducted the survey during April to July 2018. All of them were acquainted explicitly with the research context. Prior to the actual survey, a pilot study was undertaken to test the applicability of the questionnaire. During the survey, the sampling technique of probability proportional to size (PPS) was employed, considering the livelihood categories in each union. Around 35 households were targeted for each union and respondents were selected proportionally based on the percentage of existing livelihoods in that union. For example, if 50% of the population in a union practiced a particular livelihood, 50% of the households to be surveyed were targeted from this occupational group. Participation in the survey was voluntary. Each interview included 150 questions and took around 1.5–2 hours. Survey administrators provided explanations in cases where participants were unable to comprehend a question. The household heads were interviewed as they were believed to be the primary spokesperson for their households.
2.5. Inspection of survey responses and categorization of variables
All survey sheets were checked carefully to exclude those with incomplete responses (n = 27). For the purpose of analysis, a total of 408 responses were utilized. The responses were transferred to statistical software, The Statistical Package for the Social Sciences (SPSS) version 24 (IBM Citation2016). The majority of the questions were converted to and stored in binary format (1 = yes, 0 = no), except those variables having numeric values (e.g., total number of educated household members, monthly income, number of dependent persons). Of the variables, 49 were retained for the final analysis after a multicollinearity check and the combination of variables into meaningful social indicators (e.g., dependency ratio, education status). Variables were further categorized into the three different phases of the cyclone disaster, before (pre-disaster), in the short term after (syn-disaster), and in the long term after (post-disaster), based on existing literature and field experience ().
Table 1. Selected variables for PCA analysis representing pre-, syn-, and post-disaster phases.
2.6. Computing social resilience indices (SoRIs)
Index-based assessment is a commonly used method to measure a social construct (e.g., resilience or vulnerability). Holistic measures that capture multiple social aspects linked to the three distinct phases of a disaster using indices can offer clear insights into the most important factors determining community resilience at different points in time. An index-based assessment not only provides insight about the constructs but can also be used to display the outcomes in a spatially explicit way. Moreover, it minimizes the interpretational complexity of individual variables and allows multiple attributes to be aggregated, compared, and the outcome ranked for a better understanding of a particular concept (Reckien Citation2018). Generally, an index value is created by summing variables with appropriate weights. There is no universally accepted method to determine the weights, with researchers employing diverse techniques. In order to create weighted aggregated SoRIs for the three disaster phases, we relied on Principal Component Analysis (PCA) both to identify the most influential components and also to calculate component variance as the basis for weighting (Solangaarachchi et al. Citation2012).
2.6.1. Principal component analysis (PCA)
Principal Component Analysis is a multivariate data analysis technique that reduces the dimensionality of a large dataset and improves data interpretability. The primary task of PCA is to reduce a dataset with many variables to a minimum number by decomposing the original variables into groups or clusters, thereby obtaining the latent components that identify patterns and reveal variations in the dataset (Field Citation2013). PCA displays variation in data through a number of principal components (PC), which are the outcome of a linear orthogonal transformation process in N-dimensional space. This computing process runs continuously until the dataset variability is determined. This iteration process attempts to extract maximum variability within a large dataset and finally develop a set of components, with the 1st PC having maximum variance followed by 2nd PC exhibiting the next highest variance, and so on.
2.6.2. PC extraction
Prior to applying PCA, a few conditions needed to be met and checked. Firstly, we examined the internal correlations within the dataset in order to eliminate redundant variables. The Pearson pairwise correlation matrix was applied, using r = 0.25 as a cut-off. None of the remaining variables in the three different categories showed high correlations. The second task was to standardize the variables, as bias can occur in model outcomes due to the inclusion of data with different scales and ranges. This was done in the correlation matrix when running PCA, as the PCA standardizes the data prior to component extraction. Since PCA assembles variables according to their variance into a number of PCs, the varimax rotation was employed to extract the PC with the highest variance. The Kaiser Criterion was used to select the number of PCs (i.e., PCs with an eigenvalue greater than 1). Subsequently, the component extraction was assessed quantitatively by two separate tests: the Kaiser-Meyer-Olkin (KMO) test for sampling adequacy and Bartlett’s test of sphericity to check the internal correlation among the variables. The criteria set by these two tests were met for all three PCAs used in this analysis (i.e., pre-, syn- and post-disaster analyses). Communality, representing the variance of variables in the corresponding component’s score, was considered to be ≥ 0.4 for this analysis, which meant that the extracted PCs represented the variables adequately (Field Citation2013).
PCs are a broad representation of a particular trait of social resilience; they have directional influence, whether contributing positively, which indicates increased resilience, or negatively, which indicates decreased resilience (Wood et al. Citation2010). This directionality is determined from how the variables loaded on corresponding PCs. In our study, all the variables except one loaded positively on their respective components and none of the components had both positively and negatively loading variables. All PCs extracted in this study had positive directionality, thus implying individual PCs represented a specific community capacity that increased community resilience. Each PC is composed of the sum of variables that it contained, which is mathematically represented by the following equation:
(1)
(1)
where, Xi is the standardized value of variable i, and βi is the corresponding loading.
As noted previously, the SoRI for each disaster phase was calculated using the weighted aggregation technique; the PCs were weighted using the percentage of variance they explained in the PCA, implying that they do not contribute equally to social resilience. Finally, the SoRI was calculated using the formula:
(2)
(2)
where, Wi is the weight of PCi, the percentage of variance explained.
Once each individual SoRI was computed, an Effective Social Resilience Index (ESoRI) was calculated using the variables that proved to be most influential to social resilience in each of the three disaster phases. The percentage values of these variables were converted into z-scores and summed to produce the final ESoRI index.
2.7. Mapping social resilience
After calculating the SoRI for the pre-, syn- and post-disaster phases and the composite ESoRI, four separate maps were generated using ArcGIS (version 10.4) to reveal the spatial patterning of social resilience across all the unions of the study area. In order to obtain the phase-specific index value (i.e., SoRI and ESoRI) for each union, we first calculated individual SoRI and ESoRI values for each household that was surveyed and then aggregated those values for each union to map them using the GIS. We used the Shapiro-Wilk test (p < 0.05) in SPSS to test the distribution of index values for normality before calculating the average index value from the household index values grouped under a particular phase. If the value in a union followed a normal distribution, the mean value was taken as the representative index value for that union; otherwise, the median value was used. For ease of further calculation, all union index values were then normalized to a range of 0 to 1 using the min-max formula. We joined the values to the union shapefile and each union was classified into one of three categories: low, medium or high resilience, using quantiles as class breaks. The study area is small, with fairly similar measures of readiness, response, and recovery by local residents and disaster managers across the region. However, due to differences in their geographical locations, we defined the boundary of highly exposed unions (exterior) and less exposed unions (interior) based on their position from the sea and rivers. Accordingly, the mapping technique focused on highlighting the differences in social resilience in these two different geographic settings within the study area.
2.8. Validation
Generally, if a community has already initiated or has an adequate preparedness program for future disasters, they can adjust to an emergency with minimal loss, and subsequently manage a quick recovery. To test this hypothesis, household recovery, an indicator of resilience, was compared with the ESoRI values. The final composite ESoRI map was validated against household responses regarding recovery after Cyclone Sidr measured using a Likert scale (1–3, where 1 indicates barely, 2 indicates moderately, and 3 indicates mostly recovered). This study anticipated a straightforward and positive relationship between recovery and social resilience, which meant that the higher the resilience of a union, the higher the percentage of mostly recovered households within that administrative boundary. Therefore, the percentage of households that were mostly recovered was determined for each union and was compared with its respective ESoRI value in order to validate the outcome of the study.
3. Results
3.1. General description of the households and their disaster management capacity
The key findings regarding the demography, socio-economy, and disaster-management strategies of the study area at the household level are presented in this section. 77% of the surveyed households were nuclear families, with a mean of five persons per household. The mean age of the household head, usually a male, was 46.5 years, with the range being 22 to 80 years; the mean length of their residence in this area was 43 years. The average proportion of dependents was 65%. Only 3% of households had disabled members. 98% of the households lived in their own house, with the remaining 2% either in a rental house, a relative's house, or government housing. 71% of the houses in the survey sample were kutcha (made from low-quality materials like mud and thatch), 24% semi-pucca (roofs made of corrugated iron sheets and floor and exterior walls made of high-quality materials), and 4% pucca (floor, roof, and exterior walls all made of high-quality materials). The literacy rate of the surveyed households was 69%. Regarding primary occupation, 47% lived on agriculture, 17% on fishing, 11% were day laborers, 15% were engaged in business, 4% in government and private jobs, and 5% in miscellaneous activities (school/college teacher, medical practice, and tourism businesses). Around 59% of the households lived below the poverty line (monthly per capita income ∼$USD 25) (BBS Citation2016).
Many of the households in this area had participated in basic disaster-management training and awareness programs, corresponding to 39% and 38% of the total surveyed households, respectively. 45% of households stocked dry food, medicines, and other necessary items as a part of their contingency planning. Prior to Cyclone Sidr’s landfall in 2007, 86% of households received early warning of the storm; 70% managed to evacuate successfully and seek shelter well before the hazard struck. In the post-disaster period, 66% of households received house repairs, 31% received cash, and 6% received health support to help them recover from the impact of the disaster. 48% of households believed that relevant organizations, i.e., local government and NGOs, had collaborated on disaster-management activities in the study area. In the recovery context, 48% of households believed that they had only partially recovered, 28% stated that they were moderately recovered, and only 16% of the households believed they had fully recovered after Cyclone Sidr.
3.2. PCA outcomes: Pre-, syn- and post-disaster phases
The following sections report the outcomes of the PCA, conducted to identify the most influential social factors contributing to household resilience in the three phases of Cyclone Sidr, and present the maps generated to reveal the spatial patterning of social resilience across the study area.
3.2.1. Pre-disaster PCA
The purpose of the pre-disaster PCA was to discover key factors contributing to the resilience of a household, including preparedness measures to safeguard lives and property during the cyclone and the subsequent recovery phases. After testing for statistical significance using the KMO (> 0.5) and Bartlett's Test of Sphericity (p < 0.001), eight PCs including 21 variables, were retained in the pre-disaster PCA, explaining 63% of the total variance (). PC1 denoted non-structural disaster preparedness measures and explained 18.0% of the total variance with four variables: basic preparedness training, awareness program, active volunteers, and disaster studies (school curriculum). These actions are conventional in cyclone-prone coastal communities to ensure safety during a disaster. Their successful implementation increases resilience at the household level. PC2 represented structural measures for disaster mitigation and explained 8.6% of the total variance with three variables: maintenance of cyclone shelters, maintenance of other infrastructure (roads, bridges, hospitals), and installation of new cyclone shelters. Critical infrastructure plays a pivotal role in community functioning, not only in normal times but also in crisis situations where they help to protect lives and property. PC3, representing household contingency planning, explained 7.2% of the total variance and included three variables: sustenance for emergency, savings, and first-aid knowledge. These three things support basic life needs immediately for people who have them available and help them to recover from the initial shock of a disaster. PC4 represented household-level mitigation measures and explained 6.7% of the total variance with two variables: house-protection measures like tying the house to trees or raising its plinth level, and livestock-protection measures like vaccinating animals or rearing them on higher ground. PC5 represented education and knowledge sharing and explained 6.3% of the total variance with three variables: education status, spending on education, and sharing knowledge. Education facilitates understanding of disaster risk, hence people can implement mitigation measures well in advance (Hoffmann and Muttarak Citation2017). Sharing knowledge with others enhances awareness among communities, which may help reduce disaster risks. PC6 represented protecting social and physical assets and explained 6.0% of the total variance with three variables: residency, house ownership, and training for fishermen. Length of residence and property ownership are two vital socio-demographic characteristics that give households experience in dealing with disasters and prompt them to take risk-reduction measures. Families living in their own house generally make investments for protecting their property from disaster impacts, which is less likely in the case of renters (Bichard and Kazmierczak Citation2012). Certain occupational groups can be provided with specialized training prior to a disaster for protecting their livelihoods. For example, training for people involved in deep-sea fishing can explain how to safeguard their lives and fishing equipment. PC7 represented household financial solvency and explained 5.3% of the total variance with two variables: household earnings (above the poverty line) and additional income source. Financially solvent households are relatively more resilient, as they are obviously more capable of ensuring preparedness (spending money on disaster risk-reduction measures) than poorer households. PC8 represented housing quality and explained 4.9% of the total variance with only a single variable: type of house. Houses made of robust building materials and with higher plinth levels provide protection from the impact of hazards like wind pressure or water surge during a cyclone and are thus more resilient.
Table 2. PCA results based on pre-disaster variables.
3.2.2. Syn-disaster PCA
The purpose of the syn-disaster PCA was to ascertain the key factors contributing to resilience in terms of the skills and support that the community utilized to protect their lives and property during the crisis (immediately before, during, and immediately after the cyclone). It resulted in seven PCs with 13 variables, explaining 68% of the total variance and satisfying the KMO (> 0.5) and Bartlett's Test of Sphericity (p < 0.001) for statistical significance (). PC1, representing the emergency response, explained 19% of the total variance. Households receiving early warning of disasters can safely evacuate and receive timely relief for survival during an emergency situation and thus are more resilient than households that do not. The variables evacuation and rescue, food and water supply, post-disaster relief, and early warning interventions were included in this factor. All are essential during an emergency period. PC2 and PC3 explained 12% and 8.7% of the total variance, respectively, and represented social bonding with three variables, namely emotional, food, and shelter support from family and relatives, and social bridging with two variables, namely shelter and food support from neighbors and community members. Households with strong social bonding and bridging are generally more resilient as they can cope better with the initial shock of disasters by receiving support from relatives, neighbors or other community members after a disaster, when external support is often insufficient or not yet available. PC4 represented specialist support and explained 8.1% of the total variance, with two variables: support to fishermen and medical support. For example, fishermen out at deep-sea fishing may often fail to receive hazard warnings; therefore, they require specialized training for protecting their lives and fishing gear upon arrival of an unexpected environmental disaster. Similarly, post-disaster medical support is critically important for severely injured people to return to their pre-disaster condition. PC5 represented social connectivity, which explained 7.2% of the total variance and included two variables: connection to the local administration and relation to political/social elites. Often, households who have positive relationships with social elites can overcome disaster impacts more easily by getting support from external sources (food, shelter, and relief) during an emergency. PC6 represented family structure with two variables, family size and non-dependency, and explained 6.9% of the total variance. Small families are more resilient in terms of evacuation and rescue than larger families. Families with non-dependent members are also less vulnerable during an emergency, as they do not require extra care or assistance during evacuation and rescue. PC7 represented household wealth in terms of household commodities (TV/radio/vehicle) and explained 6.5% of the total variance. Households with a TV/radio have the privilege to receive hazard warnings quickly, especially in remote communities, where other means of information dissemination are relatively scarce and slow.
Table 3. PCA results based on syn-disaster variables.
3.2.3. Post-disaster PCA
The purpose of the post-disaster PCA was to reveal the factors that demonstrated a household’s capacity to recover from post-disaster adversities and revive its well-being in the course of time. Seven PCs were extracted by the PCA, with 15 variables, which explained 60% of the total variance and satisfied the KMO (> 0.5) and Bartlett's Test of Sphericity (p < 0.001) for statistical significance (). PC1 represented reconstruction and rehabilitation, and explained 14% of the total variance with five variables: stakeholders' progress meeting, water and sanitation support, reconstruction of critical infrastructure, house-repair support and revised financial loans. Recovery measures, essential for a disaster-impacted community, generally begin with the rebuilding of destroyed houses and essential infrastructure such as roads, bridges, schools, and shelters. Nutritional supplements, adequate healthcare services, and interest-free loans are also crucial for victims to get over the initial shock and recover. PC2, representing social bonding, explained 12% of the total variance with three variables: financial, livelihood, and physical-labor support from family and relatives. This indicates the importance of a household’s internal cohesion for post-disaster recovery. PC3 denoted long-term livelihood recovery, explained 7.8% of the total variance with two variables: diversified livelihood and migration. Households that are engaged in multiple earning activities can recover faster than households with a single income source and hence are more resilient. Migration (emigration) is another strategy disaster-affected households use to resume earning activities and return to normal life. PC4, post-disaster financial solvency, explained 7.3% of the total variance with two variables: house repair support from the government or NGOs and non-agricultural occupation. Recovery after a disaster might be prolonged for impacted households with limited economic resources; however, cash support from local authorities can lessen their suffering during this time period. Households having non-agricultural jobs and jobs with fixed salaries were found to be better off after a disaster than households that relied solely on agriculture, because of the loss of crop production and added expense of planting new crops. PC5, which denoted social bridging, explained 6.6% of the total variance and involved only one variable: physical-labor support (e.g., with house repairs or livelihoods) from neighbors and other community members (other than immediate family members). This demonstrates the importance of solidarity within a community, which expedites recovery of disaster-affected households. PC6 represented short-term livelihood recovery, and explained 6.4% of the total variance with two variables: livelihood support from local government and NGOs, and additional livelihood skills. After being impacted by disasters, households can resume their normal livelihood activities in the shortest possible time by receiving either external or internal support. People possessing diverse skills can manage living expenses for their families from alternative sources during the post-disaster period prior to the restoration of their primary livelihood sectors like agriculture and fisheries. PC7, named post-disaster well-being support, explained 6.0% of the total variance with two variables: health coverage and financial support from neighbors and other community members. Households with health insurance are eligible for free medical treatment for affected family members, which lessens the financial burden of these expenses after a disaster. Financial support from neighbors and community members is extremely beneficial for underprivileged households to survive and bounce back to normal life post-disaster.
Table 4. PCA results based on post-disaster variables.
In summary, the most influential components for social resilience across all the phases of the disaster, as revealed by the three PCA results, were basic preparedness training, emergency response, social bonding, and reconstruction and rehabilitation. Therefore, all the variables of these components were aggregated to generate the ESoRI.
3.3. Spatial pattern of social resilience index
and demonstrate clearly that no single category of resilience was dominant in interior or exterior unions of the study area. In the pre-disaster phase the interior unions ranged from high to moderate in terms of resilience (). The higher resilience of the interior unions was apparently due to the component disaster preparedness; however, education and knowledge and protecting social and physical assets components were also highly influential (). In contrast, the exterior unions showed low-to-moderate resilience. However, these unions showed a higher resilience component score for mitigation measures (both institutional and household).
Figure 3. Spatial distribution of the disaster-phase composite SoRIs (low, moderate and high resilience categories) based on a quantile classification scheme: (a) pre-disaster; (b) syn-disaster; and (c) post-disaster. Source: Author.
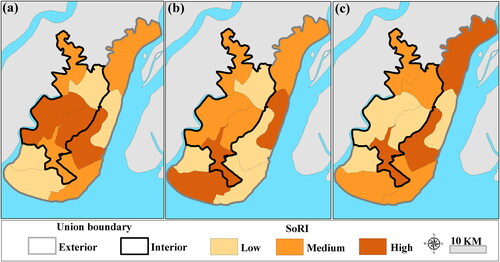
Table 5. Resilience categories (high (H), moderate (M) and low (L)) at the individual principal component level in each disaster phase.
In the syn-disaster phase, the interior unions predominantly exhibited moderate resilience (3 unions out of 5) whereas the exterior unions (3 unions out of 6 unions) showed low resilience (). In the interior unions, high resilience scores were associated with emergency response from local institutions, specialist support, and social connectivity, whereas in the exterior unions, higher scores were attributable to social bonding, social bridging, and family structure ().
In the post-disaster phase, there were similar numbers for low or moderate resilience in the interior unions. The exterior unions had the same proportion of unions in all three resilience classes (). Higher resilience in the exterior unions was attributable to higher component scores from social bonding, social bridging, and short- and long-term livelihood recovery. However, the interior unions showed higher component scores in terms of reconstruction and rehabilitation, and household well-being support ().
In the ESoRI map (), out of eleven unions, three exhibited high resilience, of which two were interior unions (Dalbuganj and Mithganj) and one was an exterior union (Dhankhali). In these unions, higher resilience was predominantly related to high levels of syn- and post-disaster resilience. Three exterior unions showed moderate resilience in comparison to one interior union. This pattern can be attributed to higher syn-SoRI values. Both interior and exterior unions had two low-resilience unions, which can be attributed to low-to-moderate resilience levels across all three individual phase indices.
3.4. Validation
The scatterplot shows a relatively strong and positive association between the percentage of households with the highest recovery and the ESoRI values (). The data do not appear to contain any outliers. A positive relationship (R = 0.75) implies that the composite index is effective in reflecting the level of social resilience in the study area. Some dispersion around the trend line is apparent in the scatterplot, which might be due to the small number of sample points (i.e., unions) in the plot or ambiguous responses by the participants in cases where either they misunderstood the meaning of recovery or wanted to hide their post-disaster well-being status.
4. Discussion
For effective disaster management (i.e., preparedness, emergency response, and recovery measures) at the community level, it is essential to draw upon the social capacities of a community that can increase their resilience to disasters (Berkes and Ross Citation2013). For this reason, this study explored coastal resilience by examining social behaviors of the coastal population in Bangladesh that support disaster mitigation and restoration of well-being after a catastrophic cyclone. To our knowledge, this is the first study to employ a comprehensive approach that considers the temporal phases of a cyclone disaster when assessing spatial patterning of resilience. The current study revealed variation in resilience at spatial and temporal scales that might provide local decision-makers with baseline information about at-risk communities while supporting planning of interventions for effective disaster risk reduction strategies (Frazier et al. Citation2013).
Quantitative methods for assessing resilience via indices are used widely because they can highlight links between key variables that might not be evident if examined individually (Cutter et al. Citation2014). The wide range of variables used in the current study yielded a robust outcome from each PCA. The phase-based PCA yielded a total of twenty-two components that were used to create the SoRIs for the three distinct phases (eight for the pre-disaster, seven for the syn-disaster, and seven for the post-disaster phase).
In the pre-disaster PCA, the highest variance was explained by non-structural disaster preparedness measures (). This outcome is consistent with the findings of the study by Khan (Citation2008), which claimed that pre-disaster readiness can significantly reduce the effect of a disaster on vulnerable communities, and thereby prevent the loss of assets. Numerous other researchers noted that disaster preparedness is essential for building community resilience, especially for the poorer communities on the Bangladeshi coast (Paul and Routray Citation2011; Mahmud and Prowse Citation2012; Orencio and Fujii Citation2013; Dasgupta and Shaw Citation2015).
In the syn-disaster PCA, the factor emergency response from local institutions was found to be dominant, which implies that acting intelligently during a crisis can minimize loss of life and property (). Our finding is consistent with several other studies, e.g., Paul Citation2009; Paul and Dutt Citation2010, in which emergency response has been found to be vital in terms of protecting life and property. Bangladesh has been recognized as a role model for reducing mortality during cyclone disasters through improvement of evacuation routes and cyclone shelters (Mallick et al. Citation2017). Another factor, social bonding, including the variables emotional support, and sharing food and shelter, was found to be important as well. This particular finding is consistent with a study in the coastal areas of Bangladesh by Islam and Walkerden (Citation2014), which revealed that communities that have good relationships among their members are more capable of reducing disaster impacts through mutual understanding and co-operation.
In the post-disaster PCA, two components, namely reconstruction and rehabilitation and social bonding, were found to be most influential (). In acknowledgement of the importance of post-disaster interventions, several studies (Mallick et al. Citation2011; Chhotray and Few Citation2012; Nadiruzzaman and Paul Citation2013; Sultana and Mallick Citation2015; Islam et al. Citation2017; Sadik et al. Citation2017) have contended that recovery requires long-term planning and monitoring to restore the well-being of the affected communities and prepare for future disasters, thereby building community resilience. The post-disaster PCA further revealed that social bonding also promoted resilience during the recovery phases of a cyclone. This finding is consistent with the study by Islam and Walkerden (Citation2014), in which they argued that bonding and bridging networks contributed significantly to community resilience during a disaster event in Bangladesh.
It is noteworthy that the four factors, basic preparedness training, emergency response, social bonding, and reconstruction and rehabilitation that proved to be highly influential to social resilience in the current study (>10% variance explained, ) capture standard practices undertaken by the coastal residents in Bangladesh and correspond closely to the conventional disaster-management cycle: preparedness, response, and recovery. However, the demographic characteristics of a community such as education and knowledge, residency and ownership, and household income, which explained less variance in the current analysis, have been highlighted in several other studies assessing social resilience from the vulnerability point of view, assuming an inverse relationship between vulnerability and resilience (Akter and Mallick Citation2013; Ahmed et al. Citation2016; Choudhury and Haque Citation2016; Kais Citation2019).
The ESoRI results provide a clear insight into the overall social resilience of the surveyed area. However, the phase-based SoRIs (pre, syn and post) revealed considerable spatial variability in resilience (classified as low, medium or high) across the different unions (). It is apparent from the maps that both the interior unions (less exposed to cyclones) and exterior unions (highly exposed to cyclones) showed variations in resilience. The map of pre-disaster SoRI showed that the interior unions were more resilient (better prepared for disaster) than the exterior unions (). Conversely, the study revealed that in the syn- and post-disaster phases, the number of highly exposed exterior unions with high resilience was greater than the number of interior unions with high resilience ( and ). But it is surprising that the highest variance-explaining-components, emergency response in the syn-disaster phase and rehabilitation and reconstruction in the post-disaster phase, had slightly higher scores (high to moderate resilience categories) in the interior unions (). This might be explained by the fact that, in this study area, the majority of the disaster-management entities were located in the interior unions, so that people in these unions could easily access relevant services during an emergency because of their social standing and closer contact with the administrators. This finding is consistent with other studies (Mallick and Vogt Citation2011; Islam et al. Citation2017) that claimed that more advantaged households in Bangladesh can procure social services with less effort than disadvantaged households. Despite such incongruities, marginalized people residing in at-risk regions prepared themselves well in advance in terms of securing their livelihoods. They have sound social cohesion with each other and also try to maintain good relationships with social elites. Consequently, they were able to receive the support and services they needed to help overcome the stress of the cyclone. Akter and Mallick (Citation2013) have also argued that the poor do not have low resilience because they show a greater ability to withstand shocks than the rich.
Although the current study was comprehensive and systematically executed, like most other studies, it too has some limitations. For example, instead of using equal weighting, the relative contributions of individual variables to the overall SoRI were calculated using the amount of variance they explained in the statistical analysis of the dataset. Although there is no general consensus on whether using equal or varying weights for the components will yield the best result when computing an additive index, this method might be inappropriate for areas with pre-identified key drivers of resilience. Primary data were used to overcome the limitations associated with secondary data (e.g., lack of availability of essential variables and lack of recent census data) and for validating the calculated SoRIs. Nevertheless, bias in the responses related to personal values, beliefs, and backgrounds of the respondents, lack of adequate knowledge, and fading memories of past disaster experience might have impacted the SoRI construction and validation. We chose Cyclone Sidr for the current research because it is considered to be the deadliest and most devastating cyclone on the Bangladesh coast in the last 13 years in terms of human deaths, ecosystem damage, and economic loss. Targeting long-term impacts and recovery requires studying disasters that occurred at least ten years ago (Chhotray and Few Citation2012), and Cyclone Sidr provided an outstanding case study for these purposes. Our developed framework is applicable for other cyclones making landfall on the Bangladesh coast and its adjoining areas. Characterizing a union as either more or less resilient in this study was based solely on the responses from the surveyed households in that particular union. Using a limited number of sample points to represent the overall social resilience of a community is, however, reasonable for traditional rural villages in Bangladesh because they have more-or-less homogeneous socio-demographic conditions. However, using such a small sample size might not be appropriate even for other parts of Bangladesh, let alone in other countries where households may differ substantially on every conceivable measure of well-being.
Recognizing the potential impacts of climate-induced disasters on development gains, the government of Bangladesh is keen to establish constitutional frameworks for promoting disaster risk reduction at the national level. The National Disaster Management Council (NDMC), the highest entity for disaster management, and other affiliated government departments and committees, are working efficiently to manage disasters in the country from national to local levels (district, upazila, and union level). Emphasizing the importance of disaster preparedness for effective response and recovery, the Sendai Framework for Disaster Risk Reduction (SFDRR) 2015–2030 has sought more investment for increasing the capacity of at-risk communities to achieve resilience. Inspired by this framework, more effort has been directed towards developing resilient communities in different parts of the world (Shaw and Okazaki Citation2003; Twigg Citation2009). To translate the priorities of the global framework, Bangladesh has undertaken strategies at the national level for the management of climate-induced natural disasters, addressing the three distinct phases, pre-disaster, syn-disaster, and post-disaster. Bangladesh has experienced a paradigm shift in disaster management from response/relief-oriented to proactive approaches in which building resilience has received major attention. The findings of the current study provide evidence that places that undertook effective pre-disaster preparation activities were more resilient.
The pressing issue of disaster impacts on coastal communities, particularly in developing countries, has turned resilience assessment into the main precursor of local policy formulation (Khalili et al. Citation2015; Kwok et al. 2016). In this regard, the importance of social resilience has been acknowledged widely (Habiba et al. Citation2013). Integrating the concept of social resilience into local disaster-management frameworks allows social resilience deficits to be addressed, thus making disaster management interventions more useful. To begin to adopt effective measures and formulate policies for responding to cyclone impacts, it is important to have a comprehensive understanding of existing community capacities. The current research has sought to provide meaningful insights into the dynamic nature of social systems in the context of cyclone hazard management.
With the help of spatial techniques and social responses, this study has developed ways of measuring the resilience status of a vulnerable coastal setting. Resilience indices like the one generated in this study have the potential to weight the temporal components differently. The pre-disaster SoRI devised in this study can be used as a proactive tool for designing disaster-prevention measures and mitigation planning at the local level, thereby increasing the resilience of vulnerable households prior to a disaster; the syn-disaster and post-disaster SoRIs can be used as reactive tools in planning to enable effective responses and recovery of the communities. Knowledge of the spatial patterning of resilience across the study area may facilitate effective disaster-management planning and subsequent implementation wherever required by directing support where it is most needed. Assessments like the one demonstrated in the current study are particularly beneficial for the countries like Bangladesh where interventions are to be designed with dual objectives including disaster management and improving community well-being and economic development due to an inadequate resource-base. The study could, therefore, contribute significantly to the disaster-management policies of Bangladesh, directly at the local level and additionally at the national level. Outside of Bangladesh, this study could benefit other areas with similar geographical settings and socioeconomic characteristics, and where coastal communities have faith in their own knowledge and skills for dealing with the impacts of a cyclone disaster. Outcomes of such studies may guide coastal planners while formulating strategies for integrated coastal zone management in many developing countries like India, Myanmar, Indonesia, or the Philippines, which are constantly combating recurrent cyclones. This simple but effective spatio-temporal approach can be applied in these countries and other parts of the globe by incorporating context-specific variables.
5. Conclusion
Disaster resilience has been defined as the ability of a social system to withstand and recover from the impacts of environmental hazards in an efficient and timely manner. Notably, the success of disaster management within a community depends on how well people can overcome immediate threats by utilizing their available resources, adjusting their lives and livelihoods to deal with the anticipated risks, and crafting institutions that both improve their capacity to survive disasters and their individual welfare. This highlights the significance of social resilience for effective disaster management. With this motivation, the present study used household responses in a cyclone-affected sub-district of the Bangladeshi coast to assess social resilience. A conceptual framework was developed and tested to highlight the fundamental aspects of social resilience within a community. Unlike traditional resilience-assessment tools based on secondary data, this study used empirical evidence of social resilience describing traditional disaster-management measures and external support of coastal residents before, during, and after a disaster. These findings have great importance for all areas of the Bangladeshi coast where marginalized populations are vulnerable to cyclone impacts and this approach could be implemented in similar settings in other nations with modifications of the socio-demographic attributes that, in this study, were typical for the study area.
The factors contributing to resilience in the pre-, syn-, and post-disaster phases of a tropical cyclone were extracted through Principal Component Analysis (PCA) and three spatially explicit indices (SoRIs) were constructed for the three distinct phases. An integrated Effective Social Resilience Index (ESoRI) was also generated to provide a holistic overview of social resilience across the study area. The dominant factors, as revealed by PCA, were basic preparedness training, emergency response, social bonding, and reconstruction and rehabilitation, which match the conventional disaster-management cycle: preparedness, response, and recovery. Spatial variations in the influential components in the different phases of the disaster and the composite SoRI at the union level showed that social resilience varied across the study area. The interior unions showed greater resilience than the exposed exterior unions in the pre-disaster phase, whereas households in the exposed exterior unions showed relatively higher resilience in the syn- and post-disaster phases. The ESoRI, however, showed that most of the highly resilient unions were situated inland (far from rivers and the sea) and the moderately resilient unions in the exterior region (close to rivers and the sea). Nonetheless, equal numbers of less resilient unions were found in both the interior and exterior areas. In a nutshell, our findings argue that even within a small geographical location, the interventions needed for disaster management vary significantly, hinting at the inconsistencies of existing disaster frameworks and policies.
While developed countries around the world struggle to cope with disasters (e.g., bushfires in Australia, earthquakes/tsunamis in Japan, hurricanes in the USA), it is far more challenging for the least-developed countries to deal with the escalating level of risk related to prevailing climatic changes. Nevertheless, irrespective of their ranking in the human development index, it is now highly recommended that every nation builds disaster resilience specifically in the social context for disaster risk reduction and to safeguard development gains. The current research therefore has the potential to contribute effectively towards reshaping societal resilience against disasters and achieving sustainable development goals in developing countries of the world.
Supplemental Material
Download MS Word (144.9 KB)Acknowledgment
This research was supported by a University International Postgraduate Award (UIPA) scholarship awarded to the first author. We are indebted to the local administration of the study site for their valuable logistical support during the field investigation and also the local residents who actively participated in the household survey. We are grateful to our research assistants, especially Mahmud Al Noor Tushar, Mehedi Hasan, Mahmudul Hasan and Mahfuzur Rahman, for their great support during the field investigation as well as for assisting with data entry. The authors are grateful to Dr Peter McIntyre, UNSW Canberra, for his English proofreading.
Data availability
This article is part of doctoral research, and the data are subject to the approval of the UNSW ethics panel. All the additional information is given in the supplementary section. The data are available from the authors upon request but not before the PhD degree is approved.
Disclosure statement
No potential conflict of interest was reported by the authors.
References
- Alam A, Sammonds P, Ahmed B. 2020. Cyclone risk assessment of the Cox’s Bazar district and Rohingya refugee camps in southeast Bangladesh. Sci Total Environ. 704:135360.
- Adelekan IO, Asiyanbi AP. 2016. Flood risk perception in flood-affected communities in Lagos. Nat Hazards. 80(1):445–469.
- Adger WN. 2000. Social and ecological resilience: are they related? Prog Hum Geogr. 24(3):347–364.
- Adger WN, Hughes TP, Folke C, Carpenter SR, Rockström J. 2005. Social-ecological resilience to coastal disasters. Science. 309(5737):1036–1039.
- Ahmed B, Kelman I, Fehr HK, Saha M. 2016. Community resilience to cyclone disasters in coastal Bangladesh. Sustainability. 8(8):805.
- Ahsan MN, Vink K, Takeuchi K. 2017. Livelihood strategies and resource dependency nexus in the Sundarbans. In: Dasgupta R, Rajib S (Eds.)., Participatory mangrove management in a changing climate. Springer, Tokyo, p 137-160 .
- Aitsi-Selmi A, Egawa S, Sasaki H, Wannous C, Murray V. 2015. The Sendai framework for disaster risk reduction: renewing the global commitment to people’s resilience, health, and well-being. Int J Disaster Risk Sci. 6(2):164–176.
- Akter S, Mallick B. 2013. The poverty-vulnerability-resilience nexus: evidence from Bangladesh. Ecol Econ. 96:114–124.
- Aldunce P, Beilin R, Howden M, Handmer J. 2015. Resilience for disaster risk management in a changing climate: practitioners’ frames and practices. Global Environ Change. 30:1–11.
- Amuzu J, Jallow BP, Kabo-Bah AT, Yaffa S. 2018. The climate change vulnerability and risk management matrix for the coastal zone of The Gambia. Hydrology. 5(1):14.
- Ayeb-Karlsson S, VAN DER Geest K, Ahmed I, Huq S, Warner K. 2016. A people-centred perspective on climate change, environmental stress, and livelihood resilience in Bangladesh . Sustain Sci. 11(4):679–694.
- BBS. 2016. Preliminary report on houshold income and expendtiture survey. Bangladesh Bureau of Statistics (BBS), Ministry of Planning, Government of the People’s Republic of Bangladesh, Dhaka, Bangladesh.
- BBS. 2017. Small area atlas of Bangladesh, Patuakhali district. Bangladesh Bureau of Statistics (BBS), Ministry of Planning, Government of the People’s Republic of Bangladesh, Dhaka, Bangladesh.
- Bennett NJ, Dearden P, Murray G, Kadfak A. 2014. The capacity to adapt?: Communities in a changing climate, environment, and economy on the northern Andaman coast of Thailand. Ecol Soc. 19(2), Article no: 5
- Berkes F, Ross H. 2013. Community resilience: toward an integrated approach. Soc Nat Resour. 26:5–20.
- Bichard E, Kazmierczak A. 2012. Are homeowners willing to adapt to and mitigate the effects of climate change? Clim Change. 112(3–4):633–654.
- Carabine E, Lemma A, Dupar M, Jones L, Mulugetta Y, Ranger N, VAN Aalst M. 2014. The IPCC’s fifth assessment Report: what is in it for South Asia. London: Overseas Development Institute and Climate Development and Knowledge Network.
- Centre for Research on Epidemiology of Disasters (CRED). 2020. EM‐DAT: the international disaster database. EM-DAT, CRED / UCLouvain, Brussels, Belgium. [accessed 2020 Apr 15]. https://www.emdat.be/.
- Chambers R, Conway G. 1992. Sustainable rural livelihoods: practical concepts for the 21st century. Institute of Development Studies (UK), Brighton, UK.
- Chauhan A, Kumar R, Singh RP. 2018. Coupling between Land–Ocean–atmosphere and pronounced changes in atmospheric/meteorological parameters associated with the Hudhud Cyclone of October 2014. Int J Environ Res Public Health. 15(12):2759.
- Chhotray V, Few R. 2012. Post-disaster recovery and ongoing vulnerability: Ten years after the super-cyclone of 1999 in Orissa, India. Global Environ Change. 22(3):695–702.
- Cox RS, Hamlen M. 2015. Community disaster resilience and the rural resilience index. Amer Behav Sci. 59(2):220–237.
- Cutter SL. 2016. The landscape of disaster resilience indicators in the USA. Nat Hazards. 80(2):741–758.
- Cutter SL, Ash KD, Emrich CT. 2014. The geographies of community disaster resilience. Global Environ Change. 29:65–77.
- Cutter SL, Barnes L, Berry M, Burton C, Evans E, Tate E, Webb J. 2008. A place-based model for understanding community resilience to natural disasters. Global Environ Change. 18(4):598–606.
- Dasgupta R, Shaw R. 2015. An indicator based approach to assess coastal communities' resilience against climate related disasters in Indian Sundarbans. J Coast Conserv. 19(1):85–101.
- Field A. 2013. Discovering statistics using IBM SPSS statistics. Sage. London
- Flood S, Schechtman J. 2014. The rise of resilience: evolution of a new concept in coastal planning in Ireland and the US. Ocean Coastal Manage. 102:19–31.
- Forgette R, Dettrey B, VAN Boening M, Swanson DA. 2009. Before, now, and after: Assessing Hurricane Katrina relief. Popul Res Policy Rev. 28(1):31–44.
- Frausto O, Vazquez A, Arroyo L, Castillo L, Hernández M. 2016. Hurricane resilience indicators in Mexican Caribbean coastal cities. Int J Safe. 6(4):755–763.
- Frazier TG, Thompson CM, Dezzani RJ, Butsick D. 2013. Spatial and temporal quantification of resilience at the community scale. Appl Geogr. 42:95–107.
- Habiba U, Shaw R, Abedin MA. 2013. Community-based disaster risk reduction approaches in Bangladesh. In: Shaw R, Mallick F, Islam A (Eds.), Disaster risk reduction approaches in Bangladesh. Springer., p.259-279, Tokyo
- M-U-I, Haque CE. 2016. We are more scared of the power elites than the floods”: Adaptive capacity and resilience of wetland community to flash flood disasters in Bangladesh. Int J Disaster Risk Reduct. 19:145–158.
- Hasan MT, Adhikary G, Mahmood S, Papri N, Shihab HM, Kasujja R, Ahmed HU, Azad AK, Nasreen M. 2020. Exploring mental health needs and services among affected population in a cyclone affected area in costal Bangladesh: a qualitative case study. Int J Mental Health Syst. 14:1–9.
- Hoffmann R, Muttarak R. 2017. Learn from the past, prepare for the future: Impacts of education and experience on disaster preparedness in the Philippines and Thailand. World Dev. 96:32–51.
- Hoque MA-A, Phinn S, Roelfsema C, Childs I. 2018. Assessing tropical cyclone risks using geospatial techniques. Appl Geogr. 98:22–33.
- Hoque MA-A, Pradhan B, Ahmed N, Roy S. 2019. Tropical cyclone risk assessment using geospatial techniques for the eastern coastal region of Bangladesh. Sci Total Environ. 692:10–22.
- Huq N, Pedroso R, Bruns A, Ribbe L, Huq S. 2020. Changing dynamics of livelihood dependence on ecosystem services at temporal and spatial scales: an assessment in the southern wetland areas of Bangladesh. Ecol Indic. 110:105855.
- IBM. 2016. IBM SPSS statistics for windows, version 24.0. Armonk, NY.
- IPCC. 2014. Technical summary. In: Field CB, Barros VR, Mach KJ, Mastrandrea MD, Van Aalst M, Adger W, Arent DJ, Barnett J, Betts R, Bilir TE, editors. Climate change 2014: Impacts, adaptation, and vulnerability. Part A: Global and sectoral aspects. Contribution of working group II to the fifth assessment report of the intergovernmental panel on climate change. Cambridge University Press, Cambridge, United Kingdom and New York, NY, USA.
- Islam MA, Paull DJ, Griffin AL, Murshed S. 2020. Assessing ecosystem resilience to a tropical cyclone based on ecosystem service supply proficiency using geospatial techniques and social responses in coastal Bangladesh. Int J Disaster Risk Reduct. 49:101667.
- Islam R, Walkerden G. 2014. How bonding and bridging networks contribute to disaster resilience and recovery on the Bangladeshi coast. Int J Disaster Risk Reduct. 10:281–291.
- Islam R, Walkerden G, Amati M. 2017. Households’ experience of local government during recovery from cyclones in coastal Bangladesh: resilience, equity, and corruption. Nat Hazards. 85(1):361–378.
- Jozaei J, Mitchell M. 2018. An assessment for developing resilience capacity of Tasmanian coastal governance. Ocean Coastal Manage. 163:130–140.
- Kafle SK. 2012. Measuring disaster-resilient communities: a case study of coastal communities in Indonesia. J Bus Contin Emer Plan. 5(4):316–326.
- Kais SM. 2019. Climate change: vulnerability and resilience in commercial shrimp aquaculture in Bangladesh. In Ganpat W, Isaac W.A. (Eds.), Oceanography and coastal informatics: breakthroughs in research and practice. IGI Global, p. 152–177, Hershey, PA.
- Kelman I. 2015. Climate change and the Sendai framework for disaster risk reduction. Int J Disaster Risk Sci. 6(2):117–127.
- Khalili S, Harre M, Morley P. 2015. A temporal framework of social resilience indicators of communities to flood, case studies: Wagga and Kempsey, NSW, Australia. Int J Disaster Risk Reduct. 13:248–254.
- Khan MSA. 2008. Disaster preparedness for sustainable development in Bangladesh. Disaster Prev Manage: Int J. 17(5):662-671.
- Kokorsch M, Benediktsson K. 2018. Where have all the people gone? The limits of resilience in coastal communities. Norsk Geografisk Tidsskrift. 72(2):97–114.
- Kundu S, Sahoo A, Mohapatra S, Singh R. 2001. Change analysis using IRS-P4 OCM data after the Orissa super cyclone. Int J Remote Sens. 22(7):1383–1389.
- Kwok AH, Doyle EE, Becker J, Johnston D, Paton D. 2016. What is ‘social resilience’? Perspectives of disaster researchers, emergency management practitioners, and policymakers in New Zealand. Int J Disaster Risk Reduct. 19:197–211.
- Lam NS-N, Qiang Y, Arenas H, Brito P, Liu K-B. 2015. Mapping and assessing coastal resilience in the Caribbean region. Cartogr Geogr Inform Sci. 42(4):315–322.
- Longstaff PH, Armstrong NJ, Perrin K, Parker WM, Hidek MA. 2010. Building resilient communities: a preliminary framework for assessment. Homeland Secur Aff. article number: 6.
- Lorenz DF. 2013. The diversity of resilience: contributions from a social science perspective. Nat Hazards. 67(1):7–24.
- Mahmud T, Prowse M. 2012. Corruption in cyclone preparedness and relief efforts in coastal Bangladesh: Lessons for climate adaptation? Global Environ Change. 22(4):933–943.
- Mallick B, Ahmed B, Vogt J. 2017. Living with the risks of cyclone disasters in the south-western coastal region of Bangladesh. Environments. 4(1):13.
- Mallick B, Rahaman KR, Vogt J. 2011. Coastal livelihood and physical infrastructure in Bangladesh after cyclone Aila. Mitig Adapt Strateg Glob Change. 16(6):629–648.
- Mallick B, Vogt J. 2011. Social supremacy and its role in local level disaster mitigation planning in Bangladesh. Disaster Prev Manage. 20(5):543–556.
- Matin N, Taylor R. 2015. Emergence of human resilience in coastal ecosystems under environmental change. Ecol Soc. 20(2)., article number:43
- Mayunga JS. 2007. Understanding and applying the concept of community disaster resilience: a capital-based approach. Summer Acad Soc Vulnerability Resilience Build. 1:16.
- Mullick MRA, Tanim A, Islam SS. 2019. Coastal vulnerability analysis of Bangladesh coast using fuzzy logic based geospatial techniques. Ocean Coastal Manage. 174:154–169.
- Murphy R, Pelling M, Adams H, Di Vicenz S, Visman E. 2018. Operationalise building back better. Int J Disaster Risk Reduct. 31:135–142. https://doi.org/https://doi.org/10.1016/j.ijdrr.2018.04.009.
- Murty P, Bhaskaran PK, Gayathri R, Sahoo B, Kumar TS, Subbareddy B. 2016. Numerical study of coastal hydrodynamics using a coupled model for Hudhud cyclone in the Bay of Bengal. Estuarine Coastal Shelf Sci. 183:13–27.
- Nadiruzzaman M, Paul BK. 2013. Post-Sidr public housing assistance in Bangladesh: a case study. Environ Hazard. 12(2):166–179.
- Norris FH, Stevens SP, Pfefferbaum B, Wyche KF, Pfefferbaum R. L. 2008. Community resilience as a metaphor, theory, set of capacities, and strategy for disaster readiness. Am J Community Psychol. 41(1-2):127–150.
- Orencio PM, Fujii M. 2013. A localized disaster-resilience index to assess coastal communities based on an analytic hierarchy process (AHP). Int J Disaster Risk Reduct. 3:62–75.
- Paton D, Gregg CE, Houghton BF, Lachman R, Lachman J, Johnston DM, Wongbusarakum S. 2008. The impact of the 2004 tsunami on coastal Thai communities: assessing adaptive capacity. Disasters. 32(1):106–119.
- Paul BK. 2009. Why relatively fewer people died? The case of Bangladesh’s Cyclone Sidr. Nat Hazards. 50(2):289–304.
- Paul BK. 2012. Factors affecting evacuation behavior: the case of 2007 Cyclone Sidr, Bangladesh. The Prof Geogr. 64(3):401–414.
- Paul BK, Dutt S. 2010. Hazard warnings and responses to evacuation orders: the case of Bangladesh's cyclone Sidr. Geog Rev. 100(3):336–355.
- Paul BK, Rashid H, Islam MS, Hunt LM. 2010. Cyclone evacuation in Bangladesh: tropical cyclones Gorky (1991) vs. Sidr (2007). Environ Hazards. 9(1):89–101.
- Paul SK, Routray JK. 2011. Household response to cyclone and induced surge in coastal Bangladesh: coping strategies and explanatory variables. Nat Hazards. 57(2):477–499.
- Pelling M. 2003. The vulnerability of cities: natural disasters and social resilience. Earthscan, Sterling, VA.
- Putnam RD. 1995. Tuning in, tuning out: The strange disappearance of social capital in America. PS: Political Sci Politics, 28, 664–664.
- Quader MA, Khan AU, Kervyn M. 2017. Assessing risks from cyclones for human lives and livelihoods in the coastal region of Bangladesh. Inter J of Envi Rese Publ Heal. 14(8):831.
- Rahman M. 2012. Enhancement of resilience of coastal community in Bangladesh through crop diversification in adaptation to climate change impacts. BRAC University, BRAC University, Dhaka, Bangladesh, MSc Thesis.
- Ray-Bennett NS, Collins A, Bhuiya A, Edgeworth R, Nahar P, Alamgir F. 2010. Exploring the meaning of health security for disaster resilience through people's perspectives in Bangladesh. Health Place. 16:581–589.
- Reckien D. 2018. What is in an index? Construction method, data metric, and weighting scheme determine the outcome of composite social vulnerability indices in New York City. Reg Environ Change. 18(5):1439–1451.
- Sadik MS, Nakagawa H, Rahman MR, Shaw R, Kawaike K, Fujita K, Islam ST. 2017. Systematic study of Cyclone Aila recovery efforts in Koyra, Bangladesh highlighting the possible contribution to vulnerability reduction. J Jpn Soc Nat Disaster Sci. 36:107–119.
- Sahoo B, Bhaskaran PK. 2019a. Prediction of storm surge and coastal inundation using Artificial Neural Network–a case study for 1999 Odisha Super Cyclone. Weather Clim Extremes. 23:100196.
- Sahoo B, Bhaskaran PK. 2019b. Prediction of storm surge and inundation using climatological datasets for the Indian coast using soft computing techniques. Soft Comput. 23(23):12363–12383.
- Saja AA, Goonetilleke A, Teo M, Ziyath AM. 2019. A critical review of social resilience assessment frameworks in disaster management. Int J Disaster Risk Reduct. 35:101096.
- Schwarz AM, Béné C, Bennett G, Boso D, Hilly Z, Paul C, Posala R, Sibiti S, Andrew N. 2011. Vulnerability and resilience of remote rural communities to shocks and global changes: Empirical analysis from Solomon Islands. Global Environ Change. 21(3):1128–1140.
- Shaw R, Okazaki K. 2003. Sustainability in grass-roots initiatives: focus on community based disaster management. Kobe: UNCRD.
- Sikder MJU, Higgins V. 2017. Remittances and social resilience of migrant households in rural Bangladesh. Migr Dev. 6(2):253–275.
- Singh PK, Chudasama H. 2017. Assessing impacts and community preparedness to cyclones: a fuzzy cognitive mapping approach. Clim Change. 143(3–4):337–354.
- Singh K, Mandal M, Bhaskaran PK. 2019. Impact of radiance data assimilation on the prediction performance of cyclonic storm SIDR using WRF-3DVAR modelling system. Meteorol Atmos Phys. 131(1):11–28.
- Solangaarachchi D, Griffin AL, Doherty MD. 2012. Social vulnerability in the context of bushfire risk at the urban-bush interface in Sydney: a case study of the Blue Mountains and Ku-ring-gai local council areas. Nat Hazards. 64(2):1873–1898.
- Sultana Z, Mallick B. 2015. Adaptation strategies after cyclone in southwest coastal Bangladesh–pro poor policy choices. Am J Rural Dev. 3(2):24–33.
- Torresan S, Critto A, Dalla Valle M, Harvey N, Marcomini A. 2008. Assessing coastal vulnerability to climate change: comparing segmentation at global and regional scales. Sustain Sci. 3(1):45–65.
- Twigg J. 2009. Characteristics of a disaster-resilient community: a guidance note (version 2), London: Benfield UCL Hazard Research Centre.
- Uy N, Takeuchi Y, Shaw R. 2011. Local adaptation for livelihood resilience in Albay, Philippines. Environ Hazards. 10(2):139–153.
- WHO. 2005. Immunization Coverage Cluster Survey—Reference Manual WHO Document Production Services, Geneva, Switzerland.
- Wood NJ, Burton CG, Cutter SL. 2010. Community variations in social vulnerability to Cascadia-related tsunamis in the U.S. Pacific Northwest. Nat Hazards. 52(2):369–389.
- Yoon DK, Kang JE, Brody SD. 2016. A measurement of community disaster resilience in Korea. J Environ Plann Manage. 59(3):436–460.