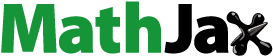
Abstract
Rockburst hazard is one of the most outstanding problems in deep rock mechanics, which has become the bottleneck for deep underground engineering in China. Rockburst occurs with some evident precursors, however, the rockburst mechanism is not clearly revealed. The acoustic emissions (microcracks) occur before the failure of rockmaterial, and rockburst is the sudden failure of rock mass caused by the development of microcracks. Therefore, it is effective to study the microcrack precursors for the surrounding rock of deep-buried tunnels by the microseismic monitoring system, and to warn of the rockburst. A method for monitoring and warning of rockburst in deep underground engineering is proposed based on the study of rockburst mechanism and in situ experience for many years. Main points of the proposed method are numerical simulation based on the monitoring, warning of rockburst based on the numerical simulation, and control of rockburst based on the warning. The proposed method was applied and improved successively in the deep-buried tunnels of the Jinping II Hydropower Station and the Hanjiang-to-Weihe River Diversion Project, which may prevent the occurrence of rockburst or decrease the incidence of rockburst. The proposed method is fast, accurate and intelligent by integrating the microseismic monitoring, artificial intelligence, big data and large-scale numerical analysis, and may be promoted to other deep underground engineering.
1. Introduction
Rockburst is a kind of geological hazards in stressed hard rocks, plenty of rockburst disasters happened in the mines and tunnels in the world (). Earlier researcher (Blake Citation1967, Russenes Citation1974, Linkov Citation1992, Kaiser et al. Citation1996, Ortlepp Citation2005) think that there is no generally accepted definition for rockburst. In the past decades, some rockburst definitions proposed by researchers worldwide is reviewed in the following text. He et al. (Citation2007) held that rockburst is the phenomenon of nonlinear dynamics with the instantaneous release of energy along the free surface of rock excavation. Zhang and Fu (Citation2008) noted that rockburst occurs in hard and brittle rocks under a high initial stress condition induced by the sudden release of strain energy during excavation, which causes ejection of rock fragments. Solak (Citation2009) considered the rockburst as the sudden and violent failure of the rock mass, caused by highly stressed brittle rocks and the rapid release of accumulated strain energy. Qian (Citation2012) defined the rockburst as a rock failure phenomenon caused by the excavation unloading in underground engineering of high stress regime. Ma et al. (Citation2014) described the rockburst as a sudden energy release phenomenon in highly stressed rocks caused by many factors such as rock fracture, rock ejection, and earthquakes. Dietz et al. (Citation2018) defined the rockburst is a sudden and violent movement and collapse of rock in underground caves under high stress conditions. In China National Standards, the rockburst in Technical Terms in Water Resources and Hydropower Project is defined as the release of strain energy in rocks causing the phenomenon of a sudden rock burst in cave sidewall or foundation pit. The Code for Engineering Geological Investigation of Water Resources and Hydropower pointed out that rockburst tend to occur in hard, brittle and intact rock sections without underground water under a high in-situ stress condition. It can be concluded that there are two ways to define rockburst, one is based on the rock failure phenomenon in combination with geomechanical analysis, and the other is based on the occurrence of seismic events in rock volume during the evolution of rockburst. Though the complex rockburst mechanism is not well and clearly understood by scholars, the high strength rock mass and the sudden release of elastic energy are generally accepted as two key factors for rockburst. The high strength characteristic is an intrinsic property of rocks, while the energy release is a representation for progressive failure of rocks.
Table 1. Statistics of rockburst disasters happened in the mines and tunnels in the world.
2. Monitoring and prediction of rockburst
Rockburst mechanism is a complex and challenging problem because the occurrence time, location, source area is characterized by strong randomness and diversity. The rockburst mechanism is not clear, which makes it difficult to predict. Prediction and warning of rockburst is classified into rockburst prediction in engineering survey stage and rockburst warning in engineering construction stage. In engineering investigation stage, the geological conditions and in-situ stresses are first explored and measured by drilling, geophysical method and mechanical experiments. Rockburst risk is evaluated based on rockburst criteria to provide reference for engineering design. The rockburst criteria mainly consist of impact energy index Wcf (Zhang et al. Citation2017), Russense criterion σθ/σc (ratio of tangential stress to rock strength) (Zhang and Fu Citation2008), strain energy storage index η (Li et al. Citation2001), elastic strain energy index Wet (Zhang et al. Citation2017), and Taozhenyu criterion σc/σmax (Ratio of rock strength to the maximum in-situ stress) (Zhang and Fu Citation2008). In order to predict rockburst tendency of rock masses, some mathematical methods, e.g., intelligent algorithm, fractal theory, and nonlinear chaos theory are used to analyze the key influence factors of rockburst such as stress regime, rock properties, geological structures, and construction method. However, prediction of rockburst tendency in underground engineering is limited by the precision of geological survey data. In engineering construction stage, accuracy and successful rate of rockburst prediction increase with an increase in the precision of stress information and geological conditions. However, prediction of rockburst tendency based on rockburst criteria is static, which cannot realize dynamic warning of rockburst during the excavation process. Prediction of rockburst tendency is difficult to meet the requirements of rockburst control in underground engineering with high risk of intense rockburst. Therefore, many techniques, e.g., pressure method, photoelastic method, resistivity method, electromagnetic radiation method, and microseismic monitoring method, are developed to monitoring rockburst in-situ. The microseismic monitoring technique is widely used to monitor and predict rockburst in mining engineering and water conservancy and hydropower engineering.
Rockburst is a typical irreversible energy dissipation process from the perspective of energy accumulation and dissipation, and there is no obvious macroscopic precursors to rockburst in general. The elastic strain energy gradually accumulates within rocks and reaches to a limit equilibrium state prior to rockburst, and a rockburst may be induced when the equilibrium breaks by the external disturbance. The evolution and occurrence of rockburst are company with energy dissipation and acoustic emission signal. Therefore, the rockburst may be predicted effective by monitoring the acoustic emission signals in rocks.
The microseismic monitoring technique is widely used to ensure the safety of underground excavation engineering in recent years. In 1940s, the United States Bureau of Mines began to apply the microseismic method to monitoring rockburst which seriously threaten the safety of underground mines, but few successful cases have been reported so far (Jenkins et al. Citation1990). The first rockburst recorded in Canada was occurred at the Ontario mine in 1928, and the rockburst research report finished by Morriosn (Citation1942) is still regarded as a classic report on rockburst. Kasier and Maloney (Citation1997) carried out the study on the relation between rockburst mechanism and microseismic events during 1990 to 1995, and they put forwards the design standard and parameters for dynamic bearing capacity of roadways in underground mines with rockburst risk. Beck and Brady (Citation2002) proposed a method for quantitative assessment and interpretation of the seismic hazard of planned excavation in a hard-rock mine, and the method is based on the three-dimensional, elastic, boundary element modelling of the host rock mass and historical observations of seismicity in the mine. Cai et al. (Citation2001) proposed a rock degradation model to quantitatively estimate the location, counts and propagation of cracks based on the microseismic data and associated source model. Urbancic and Trifu (Citation2000) reviewed the progress of microseismic monitoring in mines of Canada, which mainly focused on characterize the types of source model by the S-wave energy to P-wave energy and rockburst prediction by vibration velocity and acceleration, and b value. In Australia, the microseismic monitoring application began from 1994, which was well used in 13 mines until 2000 (Luo et al. Citation2000). Shen et al. (Citation2008) compared the stress, displacement and microseismic monitoring result during the caving of long wall working face in coal mine, Australia, and they found that microseismic indicators are more effective and sensitive than the stress and displacement information to reveal the caving process of roof. Scholars in South Africa Milev and Spottisswoode (Citation2002) used the accumulative seismic moment and volume of convergence to characterize the 300000 seismic events occurred at Deelkraal, Elandsrand, East Driefontein, Kloof and Leeudoorn mines in South Africa. Senfaute et al. (Citation1997) characterized and analyzed the 2114 induced microseismic events during the mining operations in the Provence colliery, France to identify or establish criteria for the zones likely to generate rockburst. Gibowicz (Citation1990) interpreted the research progress in seismicity induced by mining, which consists of improvement of microseismic monitoring system, understanding for induced seismicity mechanism and possibility of prediction and control of seismic hazards.
In China, the Mentougou coal mine first used the No.581 microseismograph developed by Institute of Geophysics, Chinese Academy of Sciences to monitor rockburst hazards in 1959 (Xu Citation1959). In 1970s, Changsha Institute of Mining Research developed the DYF-1 and DYF-2 portable geophony analytic instrument and STL-1 and STL-12 multi-channel acoustic emission monitoring system to study rockburst in mines (Sang et al. Citation1992). The Mentougou coal mine, Fangshan coal mine, Taozhuang coal mine, Beipiao coal mine, and Longfeng coal mine employed the microseismic monitoring system (SAK-SYLOK) from Poland successively from 1984. The Fankou lead zinc mine first employed the microseismic monitoring system from Canada for rockburst monitoring and control (Li et al. Citation2005). The Dongguashan copper mine used the ISS microseismic monitoring system from South Africa to explore the rockburst problems (Tang et al. Citation2006). Shirengou iron mine integrated the ESG microseismic monitoring system form Canada and numerical simulation to study the stability of slope during variation of mining method from open pit to underground mining. Lu et al. (Citation2013) used the TDS-6 microseismic test system to study the frequency characteristics of microseismic signal during the deformation and fracture of coal-rock samples obtained from the Sanhejian coal mine. He et al. (Citation2010) studied the characteristics of acoustic emissions during the fracture of rock mass, and proposed the catastrophe theory for rockburst based on acoustic emission monitoring. Chen et al. (Citation2012) estimated the rockburst risk of surrounding rocks near the faults in deep-buried working face.
Cao et al. (Citation2016) investigated the microseismic multidimensional information for the identification of rock bursts and spatial-temporal pre-warning in a specific coalface which suffered high rock burst risk in a mining area near a large residual coal pillar. In recent years, the microseismic monitoring system is widely used for rockburst prediction in hydraulic tunnels. Tang et al. (Citation2010) first reported the successful application of microseismic monitoring to predict rockburst in deep-buried tunnel of the Jinping II hydropower station. Ma et al. (Citation2015) studied the rockburst characteristics in tunnels of the Jinping II hydropower station and proposed 4 rockburst criteria based on microseismic monitoring. Feng et al. (Citation2016) proposed a fractal calculation method to study the self-similarity of microseismic energy during the evolution of immediate rockburst. Xiao et al. (Citation2016) studied rock failure mechanisms during the evolution of strain-structure slip rockbursts in deep-buried tunnel using moment tensor analysis of microseismic events.
3. Main points for warning of rockburst
Excavation unloading induces microcracks and associated energy release in the form of stress waves, and the triaxial compression stress regime of surrounding rock (σ1>σ2>σ3) is changed to biaxial compression stress regime (σ1≠0, σ2≠0, σ3≈0), where the σ1, σ2, and σ3 are the maximum, intermediate and minimum principal stresses, respectively. The surrounding rock is heavily damaged with the increase in number and scale of microcracks, which may finally induce the rockburst hazard. Rockburst is a progressive failure of rock mass, which is strongly nonlinear influenced by many factors, e.g., in-situ stress, rock properties, geological structures, excavation size, excavation method and support parameters. Rockburst mechanism is extremely complex, and it is difficult to predict the occurrence and intensity of rockburst by a general theory or monitoring technique. Therefore, a method for monitoring and warning of rockburst in deep underground engineering is proposed to prevent rockburst or reduce the rockburst risk. Note that main points of the proposed method are numerical simulation based on the monitoring, warning of rockburst based on the numerical simulation, and control of rockburst based on the warning, as shown in .
Numerical simulation based on the monitoring. Excavation unloading induces microcracks within surrounding rocks and radiate detectable seismic waves. The seismic waves can be captured by sensors to a limited extent, and source parameters of the microcracks, e.g., occurrence time, location, source radius and energy, can be calculated by the supported software efficiently and in real time. Failure of rock mass is caused by the energy dissipation and energy release from the viewpoint of energy conversion. The energy dissipation leads to the damage and strength degradation of rock mass, and the energy release results in the sudden failure of rock mass. Xie et al. (Citation2005) proposed the calculation formula for energy release per rock element based on the rock uniaxial compression test under the cycle loading and unloading conditions, expressed as:
(1)
(1)
where, Ue is the releasable strain energy per rock element, E0 is the initial elastic modulus of rock, ν is the Poisson’s ratio of rock, and σ1, σ2 and σ3 are the maximum, intermediate and minimum principal stresses, respectively. The location, source radius and energy are employed to invert the rock damage quantitatively at different regions based on the criterion of energy release and dissipation, and then, a three-dimensional numerical model considering the effect of microseismic damage can be constructed to evaluate the rock stability dynamically. The damage variable of rock element is defined as the ratio of the assigned energy ΔU of the rock element based on the seismic radiation energy to the total releasable elastic strain energy Ue. The elastic modulus and uniaxial compression strength are degraded linearly according to the damage variable, and the modified rock parameters are used for large-scale numerical simulation. Therefore, the stress regime, stability variation and failure of surrounding rock can be simulated dynamically, which may provide important information for warning of rockburst.
Warning of rockburst based on the numerical simulation. The in-situ stress and rock properties are of great importance for rockburst evolution. The ratio of rock uniaxial compression strength to maximum in-situ stress (Rc/σmax) is used as the rockburst criterion in the Code for Design of Railway Tunnel and Rc/σmax equals to 4 ∼ 7, 2 ∼ 4, and 1 ∼ 2 correspond to the slight rockburst, moderate rockburst, and intense rockburst, respectively. The in-situ stress is often measured by the expensive stress relief method and hydraulic fracturing method, and the stress regime distribution in the engineering area is cannot accurately described by few in-situ test data. Therefore, a sophisticated numerical model including the rock properties, geological structures and measured in-situ stress data should be constructed to back analysis the global in-situ stress regime, and to provide reference for design of engineering layout, excavation method, excavation shape/size and support. Microcracks are initiated in stressed rocks due to excavation unloading, and they may be propagated and nucleated to large-scale fractures, which may induce heavy damage, progressive failure of surrounding rocks and then rockburst hazard. The microseismic monitoring system is able to capture the source information of microseismic events efficiently, and the damage variable of rock element within the source radius can thus be calculated by the energy of microseismic events and the releasable strain energy of rock element. The rock mechanical parameters are modified using damage variable based on the proposed deterioration criterion, and then feedback to the three-dimensional finite model for large-scale numerical calculation. Variation of stress regime at the interest zone, i.e., stress buildup, stress transfer and stress release, can be quantitatively calculated by numerical simulation, additionally, excavation damage zone and stability of surrounding rocks can be dynamically evaluated, which is valuable for early warning of rockburst.
Control of rockburst based on the warning. Evolution of rockburst in deep underground engineering is extremely complex influenced by many factors, e.g., in-situ stress, geological structures, excavation disturbance and support, and it is difficult to analysis a large number of microseismic data and warning of rockburst manually. Therefore, the artificial intelligence is employed to efficiently analysis the spatial-temporal distribution and source parameters of microseismic events in real time. The occurrence possibility, intensity and location of rockburst may be predicted accurately based on the in-depth analysis of microseismic data and the feedback numerical simulation. Some active preconditioning measures, e.g., stress-relief blast and stress-relief boreholes, should be taken to effectively reduce the stress concentration and release the strain energy of surrounding rock at areas with high rockburst risk. Additionally, the energy release measures can be optimized based on the occurrence possibility and intense of rockburst, e.g., the layout of boreholes and charge quantity in each borehole for stress-relief blast, and the number, diameter and depth of the stress-relief boreholes. Some active supports should be added to control of rockburst when the occurrence possibility and intensity of rockburst cannot be reduced only by the preconditioning measures. The anchor bolts of high energy absorption capability, e.g., the new anchor bolt with constant resistance and large deformation and cone bolt, in combination with the steel arch are used to absorb the energy released by rock fractures and prevent the violent failure of shallow surrounding rocks. Besides, the opening surface should be sprayed with fiber concrete as soon as possible to improve the integrity of support system.
4. Prediction principle and intelligent criterion of rockburst
4.1. Feasibility of rockburst prediction
Monitoring and prediction of rockburst is a worldwide problem, which attracts attention of many scholars in geotechnical engineering. It is generally accepted that the rockburst source is dominant by shear fracture mechanism like earthquake, and rockburst follows scale invariant. Therefore, many scholars think that rockburst is similar to earthquake with no essential difference, and the low success rate of earthquake monitoring and prediction indicates the dim future of rockburst monitoring and prediction. The confidence of technicians in rockburst monitoring and prediction is severely affected by the low success rate of earthquake prediction, additionally, some large earthquakes were not successfully predicted in recent years, which makes many scholars in geotechnical engineering more confused about whether the rockburst can be predicted. The authors in this study hold that rockburst can be predicted to some extent, and the viewpoint is supported and validated by many successful rockburst prediction cases in mining and tunneling engineering.
Most rockbursts are the results of human activities and engineering constructions, though they are similar to natural earthquake in the view of mechanical mechanism. The in-situ stress regime of tunnel surrounding rocks is disturbed by excavation resulting in stress redistribution, high stress and energy concentration, which may drive the deformation localization, fractures and progressive failure of surrounding rocks, and finally induce rockburst hazard. The natural earthquakes are characterized by a long evolution period, low frequency and, large source depth, by contrast, the rockbursts are characterized by a short evolution period, high frequency, and source at shallow surrounding rock. Furthermore, occurrence and intensity of rockburst are closely related to the geological structures, which are surveyed before tunnel excavation. Therefore, the rockburst is more likely to be predicted than the natural earthquake, and the reasons are listed as follows:
Source area. The occurrence of natural earthquake is closely related to the stratum structure and geological conditions of crustal medium, and it is difficult to gain insight into the crustal structure in large scale, which results in the low success rate of earthquake prediction. The geological conditions in the construction area of tunneling engineering have been surveyed in detail prior to excavation, and the technicians are familiar with the geological structures and rock properties, which is of great importance for analyzing the relation between rockburst and geological structures, and studying rockburst mechanism.
Evolution period. The natural earthquakes have a long evolution period, and most of them are more than 100 years. Therefore, it is difficult for researchers to study the whole evolution process of an earthquake in their lives. The underground engineering such as tunnel is constructed in sequence according to the design, and rockburst has a close spatial-temporal relation to the known excavation process. Hundreds of rockbursts occurred in the headrace tunnels during the construction period of 5 years, and a number of rockbursts have repeatability to some extent, which is beneficial for studying rockburst characteristics and increasing the success rate of rockburst prediction.
Research scale. The large-scale geological model is difficult to construct in earthquake forecasting limited by the limited by the current development level of computer. However, for underground engineering, it is possible to construct a sophisticated geological model. The stress regime and stability variation of tunnel structure can be simulated by the three-dimensional mechanical model. Additionally, the geological structures in underground engineering can be surveyed clearly by geophysical instruments such as ground-penetrating radar and land sonar, which effectively promotes the study on monitoring and prediction of rockburst.
Rockburst is a macroscopic and progressive failure of rocks developed by the initiation, propagation and nucleation of microcracks, and the microcrack precursors to rockburst makes it possible to monitor and predict. The microcracks induced by excavation unloading can be captured by sensors to a limited extent, and the evolution of microcracks can thus be evaluated by analyzing the spatial-temporal distribution and source parameters of microseismic events, which lays the foundation for monitoring and prediction of rockburst.
4.2. Prediction principle of rockburst by microseismic monitoring
Cai et al. (Citation2007) defines the acoustic emissions and microseismic events as low-energy seismic events associated with a sudden inelastic deformation, e.g., the sudden movement of existing fractures and the generation of new fractures or the propagation of fractures. These seismic events happen within a given rock volume and radiate detectable seismic waves. The main difference between acoustic emissions and microseismic events is that the seismic motion frequencies of acoustic emission signals are higher than those of microseismic signals, as shown in . The seismic waves radiated by rock microcracks can be captured by sensors to a limited extent, and then processed by the supported software to calculate the source parameters of the microseismic events, e.g., occurrence time, location, energy, magnitude, and apparent stress. Each microseismic event contains abundant stress variation information of the interest rock volume, and the damage and stress regime variation of rocks can be evaluated by analysis on the source parameters of a large number of microseismic events, therefore, the occurrence possibility, location and intensity of rockburst may be predicted based on the in-depth analysis on microseismic data.
Figure 2. Seismic wave frequency spectrum and application of acoustic emission/microseismic techniques.
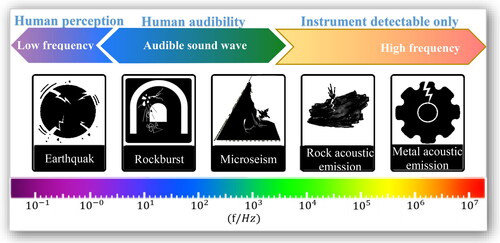
Rockburst is considered as the interaction result of surrounding rock and nucleation zone, and a two-body interaction mechanism for rockburst evolution was proposed by Ma et al. (Citation2018). The fractured or softened rock mass denoted by Body II is simplified as a spring, and the elastic intact rock mass interacting with Body II denoted as Body I is simplified as a rock specimen, as shown in . The potential function for the whole system is:
(2)
(2)
where, V is the potential energy of the two-body interaction system, λ is the initial stiffness of rock specimen, u is the deformation of rock specimen, u0 is the deformation corresponding to the peak load, k is the stiffness of spring and a is the deformation of spring. Then the instability criterion of the system is as follows:
(3)
(3)
where, λ1 is the gradient of the constitutive curve at the inflection point for weakened Body II.
According to Equationequation (3)(3)
(3) , the variation of stability for the two-body interaction system undergoes an external force can be can be divided into four stages:
Stage 1: Body I and Body II reach Point A1 and A2 along the curves in , respectively. In this stage, both Body I and Body II are in an elastic energy storage stage, and few microseismic events happen.
Figure 4. Constitutive curves of Body I and Body II in the two-body interaction (Ma et al., Citation2018).
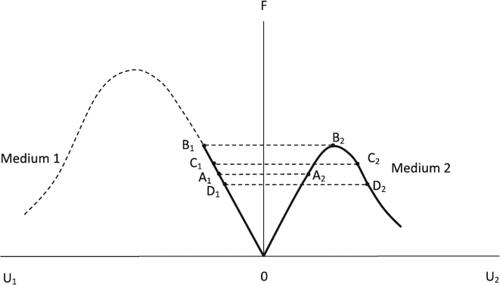
Stage 2: Body II suffers the inelastic deformation and reaches the peak point B2 in . In this stage, λ1 gradually decreases to 0 at the peak point. However, Body I is still in an elastic stage or has slightly inelastic deformation. The two-body interaction system is stable in this stage because A number of high-magnitude microseismic events happen in this stage due to the microcracks within nucleation zone develops to large-scale cracks, therefore, stage II This stage corresponds to the pre-rockburst period.
Stage 3: The stress in Body II decreases as it gradually loses its bearing capacity after the peak strength. The two-body interaction system reaches a critical state of instability when the tangent of load-displacement curve of Body II is parallel to the tangent of load-displacement curve of Body I (), as marked by C1 and C2 in . Stage III corresponds to rockburst occurrence.
Stage 4: The two-body interaction system tends to reach a new stable state with the continuous energy release in Body I and the failure of Body II, marked by D1 and D2 in . Stage IV corresponds to the calm period after rockburst.
A number of high-magnitude microseismic events happen in stage II, which can be used as the microseismic precursors to rockburst. The microseismic monitoring system can capture the microseismic events in stage I and stage II, and the stability of rocks can be evaluated by analysis on the concentration characteristics and source parameters of microseismic events. Furthermore, rockburst in the failure stage may be successfully predicted.
4.3. Acoustic emission pattern of rocks
The heterogeneity of rocks is the source of rockburst precursor. It is reasonable for simplifying the rock as a homogeneous material when conducting stress analysis on engineering structure, however, the heterogeneity of rocks cannot be ignored when studying the rock failure process because some particular phenomenon such as acoustic emission or microseismic pattern are closely related to rock heterogeneity. Microcracks happen prior to the failure of any rock structures because of the heterogeneous property of rock material, which is the basic mechanical principle for rockburst monitoring and prediction. Tang and Xu (Citation1990) considered that the acoustic emission rate of rock is consistent with the damage variable, and achieved the theory description for the characteristics of acoustic emission sequences based on the elastic damage model and statistical distribution hypothesis of rock heterogeneity. There are 3 acoustic emission sequences in the cases of different heterogeneous rocks and stress sources, i.e., mainshock sequence, foreshocks-mainshock-aftershocks sequence, and swarm sequence, as shown in , where k1, k2 and k3 are stiffness of the loading system for the 3 different acoustic emission sequences. The influence of rock heterogeneity on the acoustic emission pattern during the failure of rocks is simulated, as illustrated by . It is obvious that the microcrack precursors for the failure of rocks decreases with an increase in the rock heterogeneity.
Figure 6. Numerical tests for the acoustic emission patterns of rocks with different homogeneity by the RFPA (Tang Citation1997).
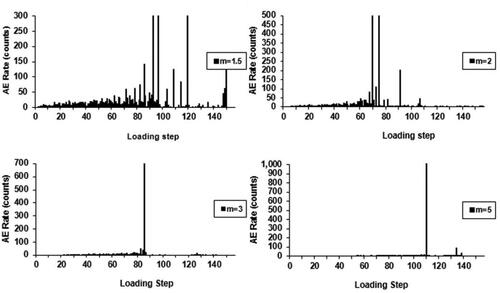
The rock statics and rock dynamics is classified based on the load properties. The load is nearly static and the deformation response is slow for rock static problems, which mainly focus on the stability of rock structure, while the rock dynamics is mainly focus on the rock failure undergoes dynamic loads. The rock failure is a dynamic process developed by the static deformation to rock instability undergoes static loads. The process of rock failure is dynamic in comparison with rock statics, while load of rock failure is static in comparison with rock dynamics. The dynamic failure process is not resulted by dynamic loads but the result of rock instability due to gradual decrease in bearing capacity undergoes static loads. Rockburst causes rock fall and ejection, which is a rock failure phenomenon induced by the violent release of elastic strain energy. The trigger mechanism of rock fall and ejection belongs to the category of rock dynamics, however, the accumulation and release of strain energy prior to the outburst of rocks is a static process. Therefore, a theory incorporates the rock dynamics and statics should be established to research the evolution and mechanism of rockburst.
4.4. Artificial intelligence prediction method of rockburst
Microseismic monitoring is a non-contact geophysical method using high-precision sensors to learn the microcracks within rocks that cannot be heard by human ears. Microseismic monitoring technique is called the eye of stratum fracturing because it can process, analysis and directly display the microcracks within rocks. Additionally, the spatial-temporal distribution and source parameters of the induced microcracks can be calculated by the powerful supporting software. As mentioned in Section 3.1, microseismic monitoring technique is an effective method for prediction and warning of rockburst, because it can capture the microseismic events happened in the stress buildup stage and inelastic deformation stage, and then predict rockburst occurred in the failure stage based on in-depth analysis of the concentration characteristics and source parameters of associated microseismic events. Rockburst occurs in a short time period, and it is difficult for many monitoring technique to predict rockburst successfully because they can only capture the precursors close to rockburst. By contrast, the microseismic monitoring technique can capture the microcracks occurred during the whole evolution process of rockburst, therefore, it may provide enough time for successful prediction and early warning of rockburst by microseismic monitoring.
The contour of microseismic event density, magnitude-frequency relation, energy concentration and variation of apparent stress accompanying with apparent volume is often used as microseismic criteria to evaluate and predict rockburst based on the SSS theory (stress buildup, stress shadow and stress transfer) in seismology (Tang et al. Citation2009). The microseismic criteria may be able evaluate the rockburst risk and intensity quantitatively, note that there may exist contradiction between the multi-parameters in some cases of rockburst prediction. It is difficult and inefficient to manually process a huge number of seismic data recorded by microseismic monitoring system, additionally, the precision of effective microseismic data is heavily dependent on the picking of the P-wave and S-wave, which influences the success rate of rockburst prediction. The method using multi-parameters of microseismic events is only available for predicting rockbursts at a limited tunnel section in a short period. Many influence factors, e.g., construction progress, new geological conditions revealed by tunnel face, and support scheme, should be taken into consideration to improve the accuracy of rockburst prediction.
The microseismic criteria should be artificially modified based on the rockburst characteristics in a short term, and this is a process of continuous learning and judgment. Artificial intelligence may finally replace humans to monitor and warn of rockburst through learning the ways of processing and thinking by human. With the development of high-performance parallel computing, artificial intelligence can support more and more complex models, and the accuracy of rockburst prediction by artificial intelligence will be higher than that by humans. Early warning of rockburst by artificial intelligence can be divided into three steps. First, carrying out the correlation and principal component analysis of source parameters, and selecting the parameters of low correlation and clear mechanical meaning for artificial intelligence learning. Second, construction of a probabilistic neural network model for rockburst prediction and early warning, which takes the cumulative microseismic event counts, cumulative energy, b value and cumulative apparent volume as eigenvectors, and takes the possibility and intensity of rockburst as prediction results. Third, making the finally prediction for the possibility and intensity of rockburst. illustrates the general mode and probabilistic neural network model for rockburst prediction. Picking of the P-wave is of great importance for microseismic event positioning, and the accuracy of microseismic event positioning can be effectively improved by reducing the picking error of P-wave arrival time. The ratio of short time average to long time average (STA/LTA) is most frequently used to pick up P-wave arrival time. In this study, an effective method for recognition and positioning microseismic events is proposed integrating threshold of STA/LTA, waveform type, waveform amplitude, frequency, energy, magnitude and analog sound.
5. Early warning of rockburst in engineering
5.1. Limits for early warning of rockburst
There are some problems for early warning of rockburst based on a large number of microseismic monitoring data in mining engineering and tunneling engineering, which mainly lays in difficulties in interpretation of rockburst precursors, complex rockburst mechanism, recognition of critical microseismic event parameters for rockburst warning, inadequate rockburst criteria, and low success rate of rockburst warning. The listed problems may limit the rapid development of monitoring and early warning of rockburst in deep underground engineering.
Rockburst mechanism is not clear. Rockburst is a catastrophe hazard induced by progressive failure of rocks, and both of the tension and shear cracks are caused by the localized deformation of rocks developed from uniform deformation. The excavation unloading deformation of highly stressed rocks in a given period of time is the key factor induced rockburst. However, many scholars try to find the quantitative indicators for rockburst prediction in the perspective of energy and stress wave, and ignore the time effect of rock deformation, which over emphasis the external factors such as excavation disturbance and blast. The deformation of highly stressed surrounding rocks induced by excavation unloading increases in a given period of time, therefore, the surrounding rocks cannot adjust to a new equilibrium instantaneously.
Rockburst precursors is difficult to interpret. The rockburst prediction is difficult with many problems, which is mainly caused by the difficulties in detection and recognition of the complex precursor information. The low-magnitude earthquakes are often used to predict high-magnitude earthquakes in seismology, however this method using the single microseismic precursor is not significant for rockburst prediction in general. There is an energy accumulation period prior to rockburst, and few low-energy microseismic events happens during the energy accumulation period. Therefore, the microseismic activities is inactive prior to the violent rockburst, and this phenomenon is called lack of shock prior to rockburst (Dou et al. Citation2018). The quiet period of microseismic activities prior to rockburst is difficult to capture and discriminate by in-situ monitoring.
Early warning system for rockburst is not adequate. The prediction, early warning and control system for rockburst is not adequate in China, and the critical warning point and warning criteria are not clear, which may be weak in the warning of high-frequency rockburst with an increase in excavation depth. The sudden changes in microseismic event counts and energy are often used to warning of rockburst at present, however, both of the initiation and propagation of microcracks are possible to cause the rapid increases in microseismic event counts and energy, which may not induce rockburst hazard. Therefore, warning criteria for rockburst using the sudden changes in microseismic event counts and energy are not adequate, and it results in a low success rate of rockburst warning. Therefore, a novel and effective warning system for rockburst hazard is in dire need of construction.
5.2. Extremely intense rockburst in the Jinping II Hydropower Station
The Jinping II Hydropower Station locates on the Jinping Bend of Yalong River at the border of Liangshan Yi Autonomous Region, Sichuan, Southwest China. The Jinping II Hydropower Station, with the total installed capacity of 480 MW, generates power using the natural water head drop of about 310 m within 150 km on the lower reach of Yalong River. The water diversion system consists of 4 headrace tunnel, 2 auxiliary tunnel and a drainage tunnel (highlighted by red line), as shown in . The drainage tunnel is excavated by the TBM with a circular cross-section, and it is 7 m in diameter and 16.73 km in length. The auxiliary tunnels have an average length of 17.5 km with a cross-sectional dimension of 5.5 m × 5.7 m (width × height) and 6 m × 6.25 m, respectively. The headrace tunnels 2# and 4# were excavated by the drill and blast method, with the diameter of 13 m and 11.8 m after lining. While, the east sections of headrace tunnels 1# and 3# were excavated by TBM with the diameter of 11.2 m after lining. The headrace tunnels pass through the main peak of Jinping mountains with the burial depth of 1500-2525 m, and surrounding rocks of the headrace tunnels are mainly marble, limestone, crystalline, limestone and sandstone. Rock masses are hard, intact and compacted, with the saturated uniaxial compressive strength and elastic modulus of 30-114 MPa and 25-40 GPa, respectively. The measured maximum principal stress of the Jinping II Hydropower Station is 46 MPa, and the maximum principal stress by regression analysis is 70 MPa (Li et al. Citation2012).
An extremely intense rockburst occurred in the drainage tunnel on November 28, 2009 with the maximum failure depth of 9 m, and more than 400 m3 rock volume was ejected to the free surface. The support system of 30 m along tunnel axis was completely destroyed, additionally, 7 workers died and 1 worker injured during the extremely rockburst. Microseismic activities during the evolution of the extremely intense rockburst were well recorded by the microseismic monitoring system, and the extremely intense rockburst was successfully predicted by analyzing the evolution of spatial-temporal distribution of microseismic activities. Tang et al. (Citation2009) studied variation of the stress regime (SSS theory) during the evolution of earthquakes, and the stress regime around the source area can be changed by the earthquakes. A new earthquake may be triggered due to high stress buildup and may not be triggered because of stress shadow at the source area. illustrates contours of microseismic event density during the evolution of the extremely intense rockburst, and the contours are able to reflect variation of stress regime of surrounding rocks. The blue, cyan and green areas in are relative safe areas with low event densities, while the yellow and red areas are of high rockburst risk with high event densities. A large number of microseismic events concentrated in #1 zone 22 days prior to the extremely intense rockburst, as marked by the white ellipse in , which results in a gradual increase in the microseismic event density. The contour of microseismic event density is cyan and blue in #1 zone in , therefore, the surrounding rock is stable with a low rockburst risk. The microseismic event density of #1 zone tends to be stable with the advancement of tunnel face, while it rapidly increased in #2 zone 14 days prior to the extremely intense rockburst, see . Few microseismic events happened in #1 zone, and the rockburst risk is relieved because stress release or stress transfer to #2 zone. A lot of microcracks were induced in the highly stressed surrounding rock in #2 zone due to stress buildup and stress transfer from #1 zone, and rockburst risk in #2 zone is high, see the yellow and red colors in . The microcracks induced by excavation unloading kept clustering in the rockburst core in #2 zone resulting a rapid increase in microseismic density, see the red area in , and it is inferred that the stress regime in the rockburst core increased to a very high level because of successive stress buildup. It is obvious that the microcracks is propagated and nucleated to large-scale cracks 2 days prior to the extremely rockburst, and surrounding rock is unstable with an extremely high rockburst risk. An extremely intense rockburst occurred in the rockburst core in the drainage tunnel of the Jinping II Hydropower Station on November 28, 2009, which verifies effectiveness of prediction and early warning of rockburst by microseismic monitoring.
5.3. Intense rockburst in the Hanjiang-to-Weihe River diversion project
The Hanjiang-to-Weihe River Diversion Project is located in the Shaanxi Province, which transfers water from the Hanjiang River of the Yangtze River basin to the Weihe River of the Yellow River basin. The water diversion projects is mainly made up of the Huangjinxia Water Control Project, Sanhekou Water Control Project and Qinling water conveyance tunnel, as shown in . The water diversion project begins at the Huangjinxia reservoir in Yang County, and the outlet of Qinling water conveyance tunnel is the Huangchi River in Zhouzhi County. The water diversion project is planned to transfer 1.5 billon m3 to the Central Shaanxi area in 2030. The Qinling water conveyance tunnel is the first one to penetrate the Qinling Mountains with the length and maximum burial depth of 81.77 km and 2012 m, respectively. The Qinling water conveyance tunnel at the southern Qining tender and northern Qinling tender is excavated by the TBM, and the other sections are excavated by the drilling and blasting method. The tunnel is planned to be completed in 2021 with a general construction time of 6.5 years. The Qinling water conveyance tunnel at the southern Qinling tender locates in the Simudi Town of the Foping County with the length of 18.27 km. An inclined shaft, namely #4 sub-tunnel in , is constructed to solve the problems of long-distance ventilation and gangue transportation. The #4 sub-tunnel is the longest inclined shaft in Asia with an inclined length of the 5820.21 m. The maximum and average slope of the #4 sub-tunnel is −11.96% and −10.79%, respectively. The #4 sub-tunnel is 6.5 m in height and 6.7 m in width with a semicircular arch section, which is excavated by the drilling and blasting method. The surrounding rocks of the #4 sub-tunnel are mainly Indosinian granite with the burial depth of the is 250 m to 1430 m, and the measured maximum horizontal principle stress is 10 MPa to 45 MPa with the dominant direction of N38°W.
An intense rockburst occurred on March 4, 2017 in the #4 sub-tunnel at Chainage 5029 to 5032, and the failure depth of the surrounding rock is about 2 m. shows the spatial-temporal distribution of microseismic events during the evolution of the intense rockburst. The tunnel face was excavated to Chainage 5017 from 0:00 on February 27, 2017 to 0:37 on March 2, 2017. In total of 57 microseismic events were recorded by the microseismic monitoring system, see , and the scatter events are of low energy and magnitude, therefore, the rockburst risk is low according to the inactive microseismic activities. The tunnel face was excavated to Chainage 5023 at 21:28 on March 2, 2017, and 94 microseismic events were captured, some high energy and magnitude events happened close to and in front of the working face, see . The rockburst risk raises with an increase in the number of high energy events. The tunnel face was excavated to Chainage 5026 from 21:28 on March 2, 2017 to 7:00 on March 3, 2017, and 19 microseismic events happened during this period. The microseismic events were mainly concentrated around the working face, and a high event density zone was formed at Chainage 5026 to 5034, see . The tunnel face was excavated to Chainage 5029 from 7:00 on March 3, 2017 to 19:29 on March 3, 2017, and 34 microseismic events were recorded and mainly clustered in the high event density zone, which results in a rapid increase in the event density close to the working face. The surrounding rock is heavily damaged because microcracks may be propagated and nucleated to large-scale cracks, and rockburst risk is high. The tunnel face advanced to Chainage 5032 after the excavation blast at 19:29 on March 3, 2017, and an intense rockburst occurred Chainage 5029 to 5032 at 1:50 on March 4, 2017. The successful cases of rockburst prediction in the Jinping II Hydropower station and the Hanjiang-to-Weihe River Diversion Project indicates that it is effective to use microseismic monitoring technique for prediction and early warning of rockburst.
5.4. Application of artificial intelligence in the Hanjiang-to-Weihe River diversion project
There remains 5000 m at present for the Qinling water conveyance tunnel to be completed, and the tunnel is now constructed at sections of the largest burial depth. High-intensity rockbursts are frequently induced because of the failure of highly stressed hard rocks, which not only poses serious threat to the safety of workers and equipment but also restricts construction progress. The TBM can only advance about 100 m a month influenced by the high-intensity rockbursts. The artificial intelligence is employed to learn the microseismic event information, e.g., source parameters, waveforms, location, and frequency, during the evolution of rockbursts. Then, the artificial intelligence will recognize and analysis valid microseismic events among a large number of seismic data efficiently. Finally, the artificial intelligence will make prediction and warning of rockburst. The artificial intelligence is more efficient in data processing and analysis than human, and accurate and automatic rockburst prediction may be realized based on continuous learning of the big microseismic data by artificial intelligence. Application of artificial intelligence to predict and warn of rockburst in the Hanjiang-to-Weihe River Diversion Project is listed as follows:
Picking the P-wave of seismic waves by recurrent neural network (RNN). 5000 seismic waveforms in the Qinling water conveyance tunnel were selected as original data, and they were resampled with a sampling rate of 5000 Hz. 2000 samples were finally constructed after translation and scaling transform of the original data, and the samples were used for training the RNN. Some cases of P-wave picking by manual and RNN is shown in .
Recognition of valid microseismic events. A sample set consists of 100 seismic waveform was used to construct the fuzzy neural network structure based on fish swarm optimization, which takes the P-wave arrival time, maximum amplitude, duration time, dominant frequency, frequency band width and corner frequency were as input variables, and the seismic wave types as output variable. The fuzzy neural network model was trained to learn and analyze the samples, and used to distinguish different kinds of seismic waves. The seismic waves can be successfully recognized with a high accuracy of 97%, which can be used for recognize valid microcrack signals in the Qinling water conveyance tunnel efficiently among a large number of seismic waves. The result of recognition of valid microseismic events is listed in .
Construction and validation of rockburst risk samples
Table 2. Recognition of valid microseismic events.
In total of 193 rockburst risk samples were constructed based on the rockburst prediction by engineer, and 175 of them were selected as training set, the others as testing set. The probabilistic neural networks including four-layer network structure, i.e., input layer, mode layer, sum layer and output layer, was used to learn the rockburst risk samples. Additionally, cross-validation was used to better utilize the samples and reduce the risk of over fitting. The testing results for rockburst prediction by probabilistic neural networks is listed , note that the test is based on limited rockburst and microseismic data in the Hanjiang-to-Weihe River Diversion Project takeing the rockbrust risk by predicted human as the real data. The probabilistic neural networks model is effective and efficient for rockburst prediction because the rockbursts of training set and testing set were predicted with high accuracies of 84.6% and 86.7%, respectively.
Table 3. Testing results based on practical data for the Hanjiang-to-Weihe River Diversion Project.
Based on the microseismic events distribution figure at the downstream of No. 4 tunnel from June 20 to 29, 2018, shown in , the Artificial Intelligence Processing System predicted the possibility of rockburst and issued a strong rockburst notification on June 29, with a risk range of K39 + 390 ∼ 415m and a risk probability of 90%.
From 3:46 to 4:12 in the morning of June 30, 2018, the working face downstream of No. 4 tunnel by drilling and blasting method was constructed to the position of No. K39 + 401m, and a strong rockburst occurred (). The vertical buried depth of the tunnel at this position is 1216 m. Under the influence of the long cross joints, a deep caved cavity is formed. The longitudinal length of the caved cavity is about 4 m, the circumferential length is about 10 m, and the deepest caved cavity is about 5.8 m. The maximum single rockburst energy detected by the microseismic monitoring system was 4.36 million Joules, equivalent to about 218 kg of No. 2 rock explosive. From 5:30 to 6:15 on July 1, another strong rockburst occurred in this section, accompanied by structural collapse, and the maximum depth of the cavity was more than 10 meters.
6. Conclusions
Rockburst mechanism is complex and not clear at present, however, the rock microcracking precursors to rockburst is obvious. It is feasible to evaluate the rockburst risk and predict rockburst in underground engineering based on the evolution of microcracks. The microseismic monitoring technique, which can capture and analyze the microcracks in a limited rock volume, is widely used in underground engineering with high rockburst risk. The microseismic monitoring technique will realize high-accuracy early warning of the occurrence time, location and intensity of rockburst with the rapid development of big data and artificial intelligence. Some conclusions are drawn in this study, as follows:
(1) Rockburst mechanism is extremely complex, and it is difficult to predict the occurrence and intensity of rockburst by a general theory or monitoring technique. A method for monitoring and warning of rockburst is proposed to prevent rockburst or reduce the rockburst risk. Main points of the proposed method are numerical simulation based on the monitoring, warning of rockburst based on the numerical simulation, and control of rockburst based on the warning.
(2) Rockburst monitoring and prediction is more likely to achieve than earthquakes, and feasibility of rockburst monitoring and prediction is described in terms of the source area, evolution period, and research scale.
(3) The interaction system between the surrounding rock and nucleation zone is used to study the evolution of rockburst. The microseismic monitoring technique can capture the microseismic events in the stress buildup stage and inelastic deformation stage, and rockburst in the failure stage may be successfully predicted based on the in-depth analysis on the concentration characteristics and source parameters of a large number of microseismic events.
(4) The semiempirical method of rockburst prediction by manual can be replaced by the automatic method based on artificial intelligence. The artificial intelligence can support more and more complex model with the development of high-performance parallel computing, and the accuracy of rockburst prediction by artificial intelligence will be higher than that by humans, which may realize high-accuracy early warning of the occurrence time, location and intensity of rockburst.
Disclosure statement
The data that support the findings of this study are openly available and no potential conflict of interest was reported by the authors.
Additional information
Funding
References
- Beck DA, Brady BHG. 2002. Evaluation and application of controlling parameters for seismic events in hard-rock mines. Int J Rock Mech Min Sci. 39(5):633–642.
- Blake W. 1967. Rockburst mechanics. Golden, CO: Colorado School of Mines.
- Cai M, Kaiser PK, Martin CD. 2001. Quantification of rock mass damage in underground excavations from microseismic event monitoring. Int J Rock Mech Min Sci. 38(8):1135–1145.
- Cai M, Kaiser PK, Morioka H, et al. 2007. FLAC/P FC coupled numerical simulation of AE in large-scale underground excavations. Inter J of Rock Mech and Min Sci. 44(4):550–564.
- Cao AY, Dou LM, Wang CB, Yao XX, Yu G. 2016. Microseismic precursory characteristics of rock burst hazard in mining areas near a large residual coal pillar: A case study from Xuzhuang Coal Mine, Xuzhou, China. Rock Mech Rock Engin. 49(11):1–16.
- Chen XH, Li WQ, Yan XY. 2012. Analysis on rock burst danger when fully-mechanized caving coal face passed fault with deep mining. Saf Sci. 50(4):645–648.
- Dietz M, Oremek GM, Groneberg DA, Bendels MHK. 2018. What is a rock burst? Zbl Arbeitsmed. 68 (1):45–49.
- Dou LM, Cai W, Cao AY, Guo WH. 2018. Comprehensive early warning of rock burst utilizing microseismic multi-parameter indices. Int J Mining Sci Technol. 28(5):767–774.
- Feng JJ. 2005. Rockburst characteristics and prevention of Erlangshan Tunnel. West-China Explor Engin. 2005(7):110–111. (in Chinese).
- Feng XT, Yu Y, Feng GL, Xiao YX, Chen BR, Jiang Q. 2016. Fractal behaviour of the microseismic energy associated with immediate rockbursts in deep, hard rock tunnels. Tunnelling Underground Space Technol. 51:98–107.
- Gan YX, Wu SC, He YL, Zheng FR, Li BW. 2020. Research on characteristics of AE parameters for rock burst in Wulaofeng tunnel. J Safety Sci Technol. 16(4):76–81. (in Chinese).
- Gibowicz SJ. 1990. Seismicity induced by mining. Adv Geophys. 32:1–74.
- Guo ZQ. 2003. The rock bursts and countermeasures in Zhongnanshan highway tunnel. Modern Tunneling Technology.40(6):58–62. (in Chinese).
- Han K, Yang WB, Chen XF, Wang BY. 2020. Dynamic management control measures of rockburst risk in bayu tunnel construction. Railway Engin. 60(6):65–68. (in Chinese).
- He MC, Miao JL, Feng JL. 2010. Rock burst process of limestone and its acoustic emission characteristics under true-triaxial unloading conditions. Int J Rock Mech Min Sci. 47(2):286–298.
- He MC, Miao JL, Li DJ, Wang CG. 2007. Experimental study of rockburst processes of granite specimen at great depth. Chin J Rock Mech Eng. 26(5):865–876.
- Jenkins FM, Williams TJ, Wideman CJ. 1990. Rock burst mechanism studies at the Lucky Friday Mine. Proceedings of the 31st U.S. Symposium on Rock Mechanics. WV University, Rotterdam: Balkema. p. 955–962.
- Kaiser PK, Tannant DD, McCreath DR. 1996. Canadian rockburst support handbook. Sudbury, ON: Geomechanics Research Centre, Laurentian University; p. 314.
- Kasier PK, Maloney SM. 1997. Ground motion parameters for design of support in burst-prone ground. In: Gibowicz and Lasocki, editors. Rockbursts and seismicity in mines. Rotterdam: Balkema; p. 337–342.
- Li HP. 2017. Rock burst prevention and control technology for diversion tunnel of Jinping two hydropower station. Construct Machinery.2017(4):79–82. (in Chinese).
- Li Y. 2019. Tunnel microseismic monitoring system and engineering application of TBM construction section in the north of Hanjiweiling. Highway Traffic Technol (Application Technology Edition). 15 (7):290–292. (in Chinese).
- Li SJ, Feng XT, Li ZH, Chen BR, Jiang Q, Wu SY, Xu HJ. 2012. In situ monitoring of rockburst nucleation and evolution in the deeply buried tunnels of Jinping II hydropower station. Eng Geol. 13–138:85–96.
- Li SL, Feng XT, Wang YJ, Yang NG. 2001. Evaluation of rockburst proneness in a deep hard rock mine. J Northeastern Univ (Nat Sci). 22(1):60–63. (in Chinese).
- Li SL, Yin XG, Zheng WD, Trifu C. 2005. Research of multi-channel microseismic monitoring system and its application to fankou lead-zinc mine. Chinese J Rock Mech Engin. 24(12):20482–22053. (in Chinese).
- Li XB, Zhou J, Huang LQ, Liu ZX. 2020. Review and prospect of gold mining technology in China. Gold. 41(9):41–50.
- Linkov AV. 1992. Dynamic phenomena in mines and the problem of stability. Minnesota: University of Minnesota.
- Liu F, Tang CA, Ma TH, Tang LX. 2019. Characterizing rockbursts along a structural plane in a tunnel of the Hanjiang-to-Weihe river diversion project by microseismic monitoring. Rock Mech Rock Eng. 52(6):1835–1856.
- Liu WD, Yu QJ, Lu JE, Zhang SJ. 2009. An analysis on mechanism of rockburst during deep mining excavation in Linglong gold mine. China Mining Magazine. 18 (05):112–115. (in Chinese).
- Lu CP, Dou LM, Zhang N, Xue JH, Wang XN, Liu H, Zhang JW. 2013. Microseismic frequency-spectrum evolutionary rule of rockburst triggered by roof fall. Int J Rock Mecha Mining Ences. 64:6–16.
- Luo X, King A, Ross J. 2000. Microseismic monitoring at Southern Colliery for understanding roof fracturing mechanisms. Brisbane.
- Ma TH, Tang CA, Cai M. 2014. Analysis, monitoring and control of rockburst. Dalian: Dalian University of Technology Press. (in Chinese).
- Ma TH, Tang CA, Tang SB, Liang K, Yu Q, Kong DQ, Xu Z. 2018. Rockburst mechanism and prediction based on microseismic monitoring. Int J Rock Mech Min Sci. 110:177–188.
- Ma TH, Tang CA, Tang LX, Zhang WD, Wang L. 2015. Rockburst characteristics and microseismic monitoring of deep-buried tunnels for Jinping II Hydropower Station. Tunnelling Underground Space Technol. 49:345–368.
- Milev AM, Spottisswoode SM. 2002. Effect of the rock properties on mining-induced seismicity around the venterdrop contact roof, Witwatersrand Basin, South Africa. Pure Appl Geophys. 2002(159):165–177.
- Morriosn RGK. 1942. Report on the Rockburst Situation in Ontario Mines. Transactions, Can Institut Min Metallurg. 45:225–272.
- Ortlepp WD. 2005. RaSiM Comes of age-A review of the contribution to the understanding and control of mine rockbursts. In: Potvin Y, Hudyma M, editors. Controlling seismic risk: Proceedings of Sixth International Symposium on Rockburst and Seismicity in Mines. Australian Centre for Geomechanics, Nedlands. p. 3–20.
- Qian QH. 2012. Challenges faced by underground projects construction safety and countermeasures. Chinese J Rock Mech Engin. 31(10):1945–1956. (in Chinese).
- Rehbock-Sander M, Jesel T. 2018. Fault induced rock bursts and micro-tremors – Experiences from the Gotthard Base Tunnel. Tunnel Underground Space Technol Incorp Trenchless Technol Res. 81:358–366.
- Ren FY, Ding S, Ren GY, Liu ZY. 2012. Experimental study on stope rockburst control in Erdaogou gold mine. J Northeastern Univ (Nat Sci). 33 (06):891–894.
- Russenes BF. 1974. Analysis of rock spalling for tunnels in steep valley sides. Norwegian: Norwegian Institute of Technology.
- Sang YF, Yu KS, Mao JH, Yu XT. 1992. Monitoring of stope surrounding rock stability and acoustic emission location system. Quarterly of CIMR. 12(3):34–37. (in Chinese).
- Senfaute G, Chambon C, Bigarre P, Guise Y, Josien JP. 1997. Spatial distribution of mining tremors and the relationship to rockburst hazard. Pure Appl Geophys. 150(3-4):451–459.
- Shen B, King A, Guo H. 2008. Displacement, stress and seismicity in roadway roofs during mining -induced failure. Int J Rock Mech Min Sci. 45(5):672–688.
- Solak T. 2009. Ground behavior evaluation for tunnels in blocky rock masses. unnelling Underground Space Technol. 24 (3):323–330.
- Tang CA. 1997. Numerical simulation of progressive rock failure and associated seismicity. Int J Rock Mech Min Sci. 34(2):249–261.
- Tang CA, Ma TH, Ding XL. 2009. On stress-forecasting strategy of earthquakes from stress buildup, stress shadow and stress transference (SSS) based on numerical approach. Earthq Sci. 22(1):53–62.
- Tang LZ, Pan CL, Yang CX, Guo R. 2006. Establishment and application of microseismicity monitoring system in Dongguashan Copper Mine. Metal Mine. (10):41–44. (in Chinese).
- Tang CA, Wang JM, Zhang JJ. 2010. Preliminary engineering application of microseismic monitoring technique to rockburst prediction in tunneling of Jinping II project. J Rock Mech Geotech Eng. 2(3):193–208.
- Tang CA, Xu XH. 1990. A cusp catastrophic model of rock unstable failure. Chinese J Rock Mech Engin. 9(2):100–107. (in Chinese).
- Teuscher P. 2009. Loetschberg base tunnel challenges in interior construction. BETON- Stahlbetonbau. 104(12):8520859.
- Urbancic TI, Trifu CI. 2000. Recent advances in seismic monitoring technology at Canadian mines. J Appl Geophys. 45(4):225–237.
- Wang JQ. 2007. Rockburst criticality evaluation and prevention counter measures in gold deposits, Hongxin gold mine as an example. Gold (6). 24–28. (in Chinese).
- Wang CH, Gao GY, Yang SX, Yao R, Huang LY. 2019. Analysis and prediction of stress fields of Sichuan-Tibet railway area based on contemporary tectonic stress field zoning in Western China. Chinese J Rock Mech Engin. 38(11):2242–2253. (in Chinese).
- Wang WQ, Hu QG, Ning GZ. 2018. Estimation of In-situ stress in rock mass in the tunnel of N-J hydropower station excavated with TBM. Soil Engin Foundation. 32(4):428–432. (in Chinese).
- Wang QH, Li XH, Ai JR, Tang BM, Yang J, Hu SB. 2003. Numerical simulation of rock deformation and rock-burst of Tongyu tunnel. Underground Space. (3):291–295. (in Chinese).
- Wang TS, Liu CY, Li HJ. 2019. Characteristics of Surrounding rock on the rock burst area of the Uzbekistan Kamchik tunnel. J Water Resour Architect Engin. 17(2):206–210. (in Chinese).
- Xiao YX, Feng XT, Li SJ, Feng GL, Yu Y. 2016. Rock mass failure mechanisms during the evolution of rockbursts in tunnels. Int J Rock Mech Min Sci. 83:174–181.
- Xie HP, Ju Y, Li LY. 2005. Criteria for strength and structural failure of rocks based on energy dissipation and energy release principles. Chinese J Rock Mech Engin. 24(17):3003–3010. (in Chinese).
- Xu SX. 1959. Design, construction and testing results of the type-581 seismograph. Acta Geophys Sin. 8(2):109–122.
- Yan ST. 2019. Study on correlation of influencing factors of rock burst in Gangmulashan Tunnel. Railway Construct Technol. 2019(11):10–13.. (in Chinese).
- Yan WB, Wang TT, Liu T. 2018. Rockburst mechanism and danger zone division of deep mining area of Changba Lead-Zinc Mine. Mining Res Devel. 38(5):50–55. (in Chinese).
- Zhang JJ, Fu BJ. 2008. Rockburst and its criteria and control. Chinese J Rock Mech Engin. 2008(10):2034–2042. (in Chinese).
- Zhang CQ, Lu JJ, Chen J, Zhou H, Yang FJ. 2017. Discussion on rock burst proneness indexes and their relation. Rock Soil Mech. 38(5):1397–1404. (in Chinese).
- Zou CJ. 1996. Study on rockburst classifications of power tunnels of tianshengqiao (II) hydropower project. Hongshui River. 1996(3):48–50.