Abstract
The increasing occurrence of disasters worldwide has motivated researchers to continuously evaluate potential technological advances to support disaster management (DM) as well as emergency response. The advent of Volunteered Geographic Information (VGI) offers the possibility of near real-time data collection or the possibility of massive disaster and post-disaster data collection. VGI is a type of geographic information provided by volunteers who have no formal training in geoinformatics and geographic information systems (GIS). The objective of this review aimed to examine research publications that address VGI in the context of DM, focusing on VGI data quality. From the collected metadata of publications published in the Web of Science (WoS) on crowdsourcing and VGI in the context of DM, we extracted and processed those articles related to data quality using the text mining method and a bibliometric approach. The research addresses the quality of VGI data and its fit for purpose for DM studies that rely on accurate and reliable geographic information for successful management through identified topics. The article concludes by highlighting the potential of VGI to provide valuable information for DM, while also pointing to the need for further research to identify and improve the quality of VGI data.
1. Introduction
A decade has already passed since Goodchild (Citation2007) announced a revolution in collecting data where citizens would become sensors. Technology related to the internet and low-cost devices for collecting data has evolved significantly. He emphasized user-generated content as a revolutionary innovation in geospatial data science. Beyond the scope of traditional geospatial data collection based on surveying and remote sensing methodologies, individuals can now collect data using various low-cost sensors in terms of volunteered geographic information (VGI). This can be attributed to the emergence of new technologies, such as digital platforms, Citizen Science problem-solving platforms, apps and the continuous rise of widespread internet engagement (Neto and Santos Citation2018). VGI is rapidly evolving in different countries and for various purposes. VGI-based models can be considered as independent or complementary mechanisms, especially when official datasets do not offer open, accessible and timely geoinformation (Moghadas et al. Citation2022). Citizen Science is the process of involving the broader public in scientific research efforts, where they contribute actively without necessarily having a formal background in the subject. An example of this is when communities, during a flood event, use mobile apps to report water levels, damages and even rescue needs. Such real-time data provided by the public can be invaluable for disaster response teams and flood mapping specialists, offering a ground-level perspective (Chow et al. Citation2023).
VGI is often associated with ‘Citizen Science’ and ‘crowdsourcing.’ When we talk about VGI, we think of geospatial data or information created by volunteers, who are usually not experts in geoinformatics (Camponovo and Freundschuh Citation2014; Čekada and Lisec Citation2019). In this regard, VGI usually involves user training or guidance (Goodchild Citation2007). Technologies are being designed and implemented today to allow everyone to easily collect geospatial data, even without being a qualified geographer or land surveyor (Cetl et al. Citation2019). VGI is a form of crowdsourcing or Citizen Science in which contributors act as non-experts and volunteers to achieve a specific goal. Crowdsourcing is, therefore, a broader term encompassing all ventures involving collective participation. VGI is conceptually suitable for crowdsourcing, especially when a large amount of data needs to be collected within a certain time frame. However, not all VGI projects use crowdsourcing. Some aim to collect information from a small group of people or a targeted group of experts in the field (Goodchild Citation2007; Capineri Citation2016; Albuquerque et al. Citation2016; Li et al. Citation2018). Crowdsourcing with scientific goals we can call Citizen Science, hile VGI can be considered a form of Citizen Science, as the public contributes to data collection, not all Citizen Science projects fall under VGI. The crucial difference is the geographical or spatial character of the data in VGI.
It is also worth noting that VGI emphasizes the geographical component of the data, while Citizen Science emphasizes the participatory nature of the scientific research process. These two areas often overlap, especially in projects where the collection of geographical data is essential. However, the terminologies emphasize different aspects of the participatory process: the nature of the data in VGI and the act of scientific participation in Citizen Science. VGI organizations have recognized the power of social media and the possibilities of integrating Citizen Science concepts to gather information needed for decision-making (Heinzelman and Waters Citation2010; Mooney et al. Citation2011; Becker and Bendett Citation2015).
Conversely, VGI may be limited by geographic scope, duration of the initiative, and usually by prescribed instructions. With the development of new trends and the availability of new technologies, it is becoming a new impetus for different contexts of disaster management (DM), which has already been observed by Haworth and Bruce (Citation2015) . In this scenario, DM refers to the coordination and management of resources using tools to improve overall disaster response (Coppola Citation2006; Carter Citation2008). Considering VGI as an element of citizen science, it plays a central role in different phases of a disaster: before, during and after the event (Kocaman et al. Citation2018). The most important problem at present is the coordination between information systems and technologies needed to improve the quality and usefulness of disaster risk information for decision makers (Ostermann and Spinsanti Citation2011). Following is the VGI quality assessment, which is still out of practical efficiency despite the academic effort to create the quality evaluation method (Antoniou and Skopeliti Citation2015). Notably, the current literature touches upon the quality assessment of VGI but often lacks a practical approach, especially in systems like Spatial Data Infrastructures (SDI) (Demetriou et al. Citation2017), which tend to be a basis for effective DM among all other applications.
Government institutions opting to be more influential in addressing all phases of DM are often challenged by insufficiently developed SDIs for natural and other elementary disasters (Manfré et al. Citation2012) and the lack of organizational participation in crowdsourcing knowledge (Lin et al. Citation2018). SDI for DM require real-time data collection, interoperability, accuracy, scalability, security and ease of use. The development of SDI for DM has been hampered by a lack of standardization, limited real-time capabilities, insufficient funding and poor system integration. In Gregorio Rosario et al. Citation2023, it is emphasized that people and policy factors are the most important critical factors for enhancing SDI performance that needs to be resolved to facilitate DM. The limited participation of organizations in crowdsourcing further exacerbates the problems, leading to data gaps, quality issues, reduced data diversity and missed opportunities for collaboration. An inclusive and collaborative approach to SDI development is essential for effective DM.
For instance, when evaluating the use of VGI in DM, Haworth and Bruce (Citation2015) identified barriers in several areas, such as collecting and displaying data, ensuring data quality and security, managing data and empowerment through VGI. Their categorization acknowledges and supports existing theories, including the four phases of the DM approach: prevention, preparedness, response and recovery (PPRR) (Abrahams Citation2001; Cronstedt Citation2002; Bajracharya et al. Citation2011; Rogers Citation2011; Xiao et al. Citation2015; Ehnis Citation2018; Moghadas et al. Citation2023). Categorizing VGI in DM faces challenges, such as diverse and complex data sources, concerns about data quality since it’s often contributed by non-experts, and scalability issues during large disaster events. Other hurdles include the interoperability of different data formats, spatial biases which might overlook certain areas and privacy concerns regarding personal information in VGI. Furthermore, integrating VGI into established DM systems requires operational changes, and there’s often skepticism about its reliability among DM professionals.
Crowdsourcing through VGI has enabled citizens to participate in all stages of the DM cycle (Haworth and Bruce Citation2015), so we focused on identifying data quality challenges in individual phases of DM. Moreover, such an approach has been used in other crises, such as business (Rogers Citation2011) and other risk management (Hodges Citation2000). In some versions, stages occur as prevention, mitigation, preparedness, response and recovery (PMPRR), or five steps in DM (Manfré et al. Citation2012).
This research addresses the challenges of VGI implementation in DM and focuses on data quality. In general, the integrated activities of DM refer to the four phases of DM, i.e. PPRR. The prevention and preparedness phases are critical prior to the event leading to the disaster, while the recovery phase refers to the long-term actions to restore the damaged ecosystem. However, the response phase requires short-term actions based on near real-time data to support decisions (Galasso et al. Citation2011). In addition, the spatial information background for DM continues to benefit from recent scientific developments in satellite and aerial imagery data collected by various sensors. Therefore, more and more spatial datasets for disaster monitoring and management can integrate other data sources and ensure effective use of the collected data.
In addition, with the increasing popularity of VGI platforms and social media, information provided by volunteer users is becoming a valuable source for DM, including in the emergency response phase (Feng and Sester Citation2018; Li et al. Citation2018). Although VGI is becoming more widespread, their use in decision-making compared to authoritative data still needs to be questioned due to the lack of trust and reliability. However, a potential shift to consider local ‘knowledge’ instead of ‘science’ could solve the trust problem and strengthen the resilience of vulnerable communities in disaster risk management (Hicks et al. Citation2019).
Meanwhile, the importance of VGI as a promising data source for DM has already been recognized (Poser and Dransch Citation2010; Albuquerque et al. Citation2016; Arapostathis Citation2020). Citizen sensing has gained prominence through many disasters, providing additional awareness of the post-disaster response and recovery phase. Moreover, because providing VGI is an easy and inexpensive way to help (Pepe et al. Citation2018), individuals are tempted to develop altruistic traits as digital humanitarianism increases (Meier Citation2015). Despite the best intentions, crowdsourcing participants may provide incomplete data. On platforms, such as social media, misinformation and rumours can spread quickly and overshadow useful, spatially relevant information (Mulder et al. Citation2016; Mejri et al. Citation2017). Against this backdrop, our study seeks to bridge the gaps. We delve deep into the challenges of implementing VGI in DM, with a keen focus on data quality, an area often underexplored in the literature. Quality assurance is critical when using crowdsourced data, as its reliability and accuracy must be ensured for effective decision-making in DM. Therefore, there is a need to review the existing quality assurance methods and identify their limitations, as well as propose new and practical approaches specifically tailored for VGI and crowdsourcing used in DM (Riccardi Citation2016). This article aims to provide a systematic review of research publications that address the quality of VGI and crowdsourcing in the context of DM. We implemented the following three-step strategy: identifying and analysing relevant publications from the Web of Science (WoS) (i), detecting research topics using an unsupervised machine learning algorithm (ii) and finally identifying data quality challenges in individual phases of DM (iii).
2. Materials and methods
Our main objective for this study was to provide a comprehensive overview and understanding of the academic literature on the quality of VGI and crowdsourcing in DM. The research methodology used is based on the methods of Aria and Cuccurullo (Citation2017), Kankanamge et al. (Citation2019) and Yan et al. (Citation2020).
The articles for this report were found in the WoS database accessed on 13/3/2023. The search strategy was determined by the keywords ‘VGI’, ‘crowdsourcing’, ‘quality’ and ‘disaster’. We conducted our search on article titles, keywords and abstracts in WoS using the specified query.
The first step was to export data from WoS using the query:
((VGI AND disaster AND quality) OR (crowdsourcing AND disaster AND quality))
The Bag-of-Words (BoW) model is used to describe text features and for the information retrieval process (IR), along with further analysis in Section 4. Such a model produces a single corpus, which represents the collection of words for each data instance (Demšar et al. Citation2013). displays a word cloud derived from the word frequencies found in the titles, abstracts and keywords of the records retrieved using the specified search terms.
Figure 1. Word cloud based on the number of occurrences of the searched terms. The figure was created using Orange data mining (Demšar et al. Citation2013).
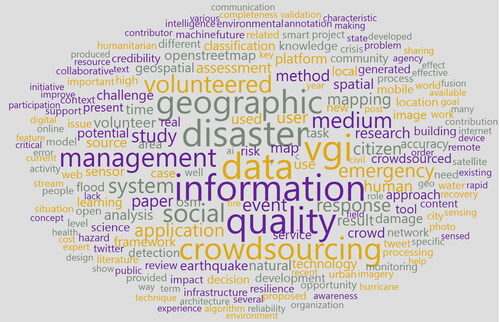
In , the size of each word corresponds to its frequency within the dataset; larger words indicate higher frequency. For instance, terms like ‘quality’, ‘disaster’, ‘crowdsourcing’ and ‘volunteered’ prominently feature due to their centrality to our research focus. The colors in the word cloud are utilized for visual differentiation and do not signify any specific thematic categorization. Hierarchical clustering, a method chosen for its ability to group similar topics, was later used to categorize articles and uncover new perspectives and patterns in the literature.
We used data mining to narrow down the research area and uncover characteristics related to the quality of VGI and crowdsourcing in DM. Data mining provides opportunities for semantic analysis and context checking, therefore the summaries of the extracted articles were grouped using unsupervised machine learning, e.g. hierarchical clustering (Vijaya and Bateja Citation2017). The hierarchical clustering method grouped similar articles and thus introduced new aspects to the literature review. shows the research workflow, which consists of three groups of activities: data pre-processing, analysis and results.
The first step was to prepare the data for data mining using algorithms for data cleaning, transformation and tokenization. Word tokenization and normalization were used to pre-process the text by excluding unnecessary words for analysis (stop words, articles, etc.). In the next step, the workflow was extended to include the BoW model, where the text is converted into a fixed-length vector by counting the number of words occurring in the text (weight) as an additional feature with which we compare the occurrence of words in the text. The third step was the presentation and visualization of the results, where the findings from the hierarchical clustering of the previous step were presented. Accordingly, similar works were mechanically identified and grouped under thematic clusters to reveal similarities from the literature review and highlight areas related to the quality of user-generated content ().
Part of the research used bibliometrix (Aria and Cuccurullo Citation2017) to automatically identify and analyse trends and patterns in the data. Bibliometrix is a free and open-source software package for the R statistical language for quantitative analysis in bibliometrics and scientometrics.
It is important to recognize the limitations of our research methodology. We focused heavily on the WoS database, and this approach may have resulted in overlooking valuable articles available in other databases. While our search terms were carefully chosen to be comprehensive, they may not capture all the nuances of the vast VGI and DM field. In addition, by focusing on DM we may not have given due consideration to the broader challenges associated with VGI. Our research provides a snapshot of the current VGI landscape, but given the rapid evolution of the field, new challenges may emerge beyond the timeframe of our study. Furthermore, the motivations and behaviours of volunteers contributing to VGI are multifaceted.
3. Results
Following the three-step strategy, the results are presented in three sections: identifying and analysing relevant publications from the WoS (i), detecting research topics using an unsupervised machine learning algorithm (ii), and finally identifying data quality challenges in individual phases of DM (iii).
3.1. Trends and themes of publications in VGI, crowdsourcing and data quality
An examination of the most popular topics in the dataset from 2010 to 2023 was conducted based on the keywords provided by the author. The parameters were set from 2010 to 2023, with a minimum frequency of 5, and 3 words per year. The word cloud in demonstrates the most used keywords. Still, a hierarchical arrangement of topics is provided in below to further understand what topics researchers discuss annually.
Figure 3. Trending topics of publications in VGI, crowdsourcing and data quality. The figure was created using the bibliometrix R-package web interface biblioshiny (Aria and Cuccurullo Citation2017).
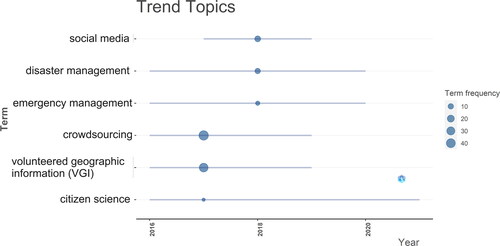
Analysis of the trending topics by keywords shows that ‘crowdsourcing’ is the highest at 46, ‘volunteered geographic information’ at 38, social media at 12, and DM at 10. In addition, the authors of the articles indicated keywords that are closely related to the topic of their publications. This analysis showed that crowdsourcing is the cumulative trending topic in the VGI DM literature by the frequency of these keywords over the years. Therefore, VGI should be addressed, likely due to the increasing need for data collection, which can be done quickly and accurately using crowdsourcing techniques. In addition, the use of social media has been increasingly discussed in DM in recent years due to its potential to quickly reach large audiences and promptly disseminate information as one of the sources. serves as a visual representation of the evolving discourse in VGI, crowdsourcing and data quality from 2010 to 2023. By highlighting trends in the use of keywords, the figure highlights the importance of crowdsourcing and its impact on data collection and DM. In addition, the inclusion of a hierarchical arrangement provides a more detailed breakdown of annual topic priorities, to track the growth and shift in academic focus over the indicated period. References to the frequency of certain keywords and discussion of the role of social media highlight the current importance of these technologies in the field of DM and data accuracy.
Looking at the timeline, it is evident that in the last 5 years, crowdsourcing and VGI have stopped being used, while social media, disaster and emergency management are still present in the author’s keywords.
Following the thematic analysis () of the author’s keywords and their interrelationships to round up the topics, it is evident that some terms have evolved. The subjects in this figure are shown in properties (density on the vertical and centrality on the horizontal axis) coordinate system. Centrality is the degree of correlation between different topics, density measures cohesiveness among nodes. The more relationships a node has with others in the thematic network, the greater its centrality and importance.
Figure 4. Map of thematic evolution. The figure was created using the bibliometrix R-package (Aria and Cuccurullo Citation2017).
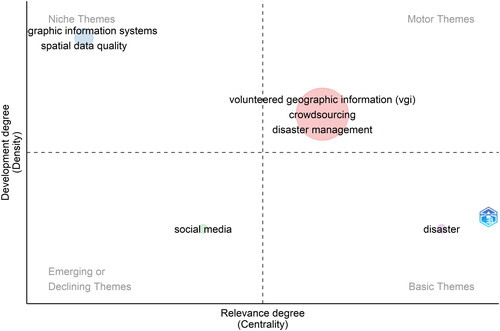
Overall, the analysis of the VGI DM quality literature suggests that the field is evolving and that different topics are gaining importance over the years. Although VGI, disaster and management remain popular topics discussed by researchers, they are being complemented by other topics related to the field. This suggests that researchers are increasingly focusing on different aspects of VGI DM, such as crowdsourcing, multiple sensors fusion neogeography and data quality, to gain further insight into the field.
3.2. Detected topics on data quality in crowdsourcing
As mentioned earlier, data mining was used to narrow down the thematic analysis and discover features related to the quality of VGI and crowdsourcing for DM. Since data mining enabled semantic analysis and context checking, we grouped the extracted articles into five clusters using hierarchical clustering ().
Figure 5. Dendrogram of five clusters defined by the method of hierarchical clustering. The figure was created using the Orange data mining (Demšar et al. Citation2013).
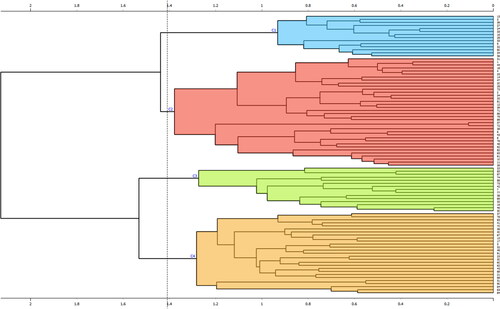
The hierarchical clustering algorithm starts with each article in its group from the formed matrix of distances of text similarity. Then clusters the two closest groups in each step until it reaches a certain number of groups using Ward’s method. In this case, we used manual iteration stop for five clusters of identified topics:
Data quality analysis and evaluation using machine learning (Cluster 1 – C1);
Quality of remote sensing data and hybrid data (Cluster 2 – C2);
Spatio-temporal context of linked data (Cluster 3 – C3);
Social media as a source and tool of voluntary information (Cluster 4 – C4);
Data quality in response and recovery applications regarding various disasters (Cluster 5 – C5).
Articles were grouped into five clusters based on their abstracts’ text-mining method, and the articles’ topics were identified. Although some topics overlapped or did not fit well into the identified clusters due to the small text mining pattern (abstracts of the selected articles), some articles were manually singled out to verify the proposed cluster topics. Below we analyse and discuss all the identified topics in the context of VGI quality in DM.
3.2.1. Data quality analysis and evaluation using machine learning (C1)
Technological development, popularization and availability of machine learning methods have also influenced data collection and processing in crowdsourcing. Such methods have mostly been implemented in selected studies to assess spatial data quality in Big Data datasets (Koswatte et al. Citation2018).
In general, the use of machine learning to assess the quality of VGI can be divided into two groups: integrated data quality assessment (Imran et al. Citation2014; Poorazizi et al. Citation2015; Aissi and Sboui Citation2017; Senaratne et al. Citation2017) and independent data quality assessment (Yuan et al. Citation2018; Koswatte et al. Citation2018; Lin et al. Citation2018; Kashian et al. Citation2019). The integrated quality assessment process incorporates theories or frameworks to automate the discovery of relevant VGIs. On the other hand, the independent data quality assessment cluster contains studies focusing on quality and usability. The existing VGIs can automatically create new datasets with an accompanying assessment of their quality. Several studies have explored the integration of Deep Learning and VGI for improved mapping and disaster assessment. Yuan et al. (Citation2018) successfully combined VGI and Deep Convolutional Neural Networks (CNNs) to generate automated building maps from satellite imagery, for example, in Kano State, Nigeria. Similarly, Zhang, Zhang et al. (Citation2019) used AI for post-disaster damage assessment and introduced CrowdLearn, which combines crowdsourcing with AI to improve the accuracy and timeliness of assessments. Feng and Sester (Citation2018) used Deep Learning to analyse social media content to identify reliable eyewitness accounts of flooding and presented their findings using a web map application that tracks flooding in major European cities.
Machine learning assessment of VGI quality can be divided into integrated data quality assessment, which automates VGI discovery and independent data quality assessment, which focuses on usability and quality. Recent research highlights the effectiveness of combining Deep Learning and VGI for improved mapping, disaster assessment and real-time event tracking using sources, such as satellite imagery and social media content.
To conclude the overview of all VGI quality assessment methods, Senaratne et al. (Citation2017) proposed data mining as an additional tool that can be used regardless of prior geographical knowledge, mainly supervised machine learning methods. Implementing machine learning methods is useful in quality assessment, where the results depend on the quality indicators and the purpose of the data. The Transfer Learning method can also be implemented, which can be helpful when ground truth data is not available. For most machine learning methods presented in selected publications, the larger the available data, the better and more visible results we get.
3.2.2. Quality of remote sensing data and hybrid data (C2)
In this cluster of research articles, a focus is placed on sensor applications, encompassing both on-site and remote methodologies. The included articles delve into various aspects of sensor utilization, exploring their applications in diverse domains and contexts. Tsai et al. (Citation2014) show an interesting way to improve the quality of crowdsourced data in disaster warning and response. Based on statistics, they integrate human and physical sensors and call them symbiotic sensors.
Foody et al. (Citation2015) conducted a VGI experiment to characterize the quality of volunteers based on tagging of captured images. The focus was on data in the form of notes or class tags provided by volunteers who visually interpreted attributes and land cover from satellite sensor images.
Danylo et al. (Citation2018) used VGI in the recovery phase and showed that VGI could be applied in the recovery phase for fast and straightforward image processing in damage assessment. In this case, comparing crowd classifications with experts showed high quality in identifying damaged buildings. Albuquerque et al. (Citation2016) are on a similar path with a study to measure the quality of volunteer ratings for validating geographic information from remote sensing imagery. Li et al. (Citation2021) introduces an automatic surface water mapping method using a deep residual neural network, combining OpenStreetMap (OSM) and Sentinel-2 data, with the SNIC superpixel algorithm for training sample generation. Applied to Germany, the model achieved an 86.32% accuracy in mapping open water surfaces, demonstrating the potential of merging volunteered geographical information and machine learning in large-scale mapping endeavors.
Fitriany et al. (Citation2021) demonstrated the utility of hybrid data usage by correlating meteorological data, Twitter activity and satellite data, the research evaluated Indonesia’s current fire detection methods and proposed a new approach using crowdsourced tweets. The findings suggest that such data could enhance Indonesia’s fire detection and mitigation strategies.
Several studies have highlighted the effectiveness and quality of VGI in various applications, from image tagging to damage assessment after disasters. The integration of VGI with remote sensing data and other datasets, as shown in studies focused on surface water mapping and fire detection, further underscores its potential for improving large-scale mapping and disaster response efforts (Moghadas et al. Citation2022).
Cluster C2 shows how VGI can be useful in quality assessment and data generation. In this cluster, post-disaster data processing has slight advantages due to the ability of remote VGI when participants engage in campaigns over the internet, such as remote sensing imagery classification or vectorization. Overall, this cluster provides insight into how VGI can be used to improve the quality of data collected from remote and on-site sensors. It also shows how VGI can be integrated with expert opinion to improve the accuracy and reliability of data.
3.2.3. Spatio-temporal context of linked data (C3)
In this cluster, the proposed data mining method categorized articles that deal with quality assessment methods in information retrieval and linking data within DM systems.
Linked data combines different sources to create a richer dataset by identifying and structuring data from corresponding datasets through semantic queries. This challenge presents an opportunity to use VGI effectively (Ahmad et al. Citation2017). Most research focuses on the semantic and temporal aspects (Sachdeva et al. Citation2017; Feng and Sester Citation2018) of crowdsourced information, including insights gleaned from social media platforms (Castillo Citation2016). Such nuances greatly affect the quality of geospatial data and lead to a more rigorous quality assessment. Using such models to assess data quality is crucial for early detection of events and integration of data into other systems (Ahmad et al. Citation2019). Using VGI, we can identify data patterns and trends, which strengthen the precision and trustworthiness of the data. Once refined, this data can be synchronized with other systems, paving the way for deep analysis and informed decision-making.
Taken together, these studies illuminate how data mining and analytical techniques can assess the quality of VGI data. They also show how they can be integrated into DM systems. The discoveries highlight the enormous potential of data mining and analytical methods to improve the soundness and reliability of VGI data and link them to other platforms.
3.2.4. Social media as a source and tool of voluntary information (C4)
Social media can be a valuable source of voluntary spatial information (Zhang et al. Citation2016; Dittus et al. Citation2017). Especially in disaster situations, we assume that participants are highly motivated and willing to participate, as they tend to share and search for information on already available platforms (Yao et al. Citation2020; Zahra et al. Citation2017). However, using this data type can be challenging due to privacy settings, user permissions and the inability to georeferenced data more accurately. Several articles have shown how social media can be useful in data validation (Yang et al. Citation2019) and even data quality assessment (Castillo Citation2016). One of the most important functions of social media is event detection. Certain events can be detected faster in social media than in traditional media (Toujani and Akaichi Citation2019). As technology evolves, attempts are being made to obtain more accurate location data by using integrative approaches such as multimodal embedding (Zhang et al. Citation2017), multi-element approach (Laylavi et al. Citation2016) and pseudo-relevance feedback approach (Priya et al. Citation2018). Utilizing social media and VGI offers a promising approach for enhancing disaster risk management. Addressing the challenge of incomplete historical records for events like flash-floods, Lowrie et al. (Citation2022) introduce a method to correlate VGI-based flood reports with official data. Using a case study of Hurricane Harvey, the flood reports from the community-operated platform Waze are placed in a spatial framework and compared with authoritative sources. This identifies potential unreported flash flood events, improving understanding of flash flooding during the hurricane and demonstrating the potential to apply this method to other events with available Waze data and official reports.
Volunteer motivation within DM is a specificity driven by the need for information social media is an increasingly important source of voluntary spatial information, particularly during disasters where individuals actively share and seek information. Despite challenges like privacy concerns and data georeferencing issues, research has highlighted its value in data validation, quality assessment and swift event detection. Advanced techniques are now being employed to refine location data from social media. Specifically, Lowrie et al. (Citation2022) showcased how VGI, combined with social media data, can improve disaster risk management. Their work with Hurricane Harvey effectively aligned community-driven flood reports from Waze with official data, uncovering unreported flash floods. Thus, advancements in technology and methodology underscore the growing significance of social media in DM, enabling more accurate, timely and strategic responses.
3.2.5. Data quality in response and recovery applications regarding various disasters (C5)
The increasing availability of the internet and portable devices has contributed to developing applications supporting DM. However, important issues for these applications are data quality elements such as completeness, thematic accuracy, logical consistency, temporal quality, positional accuracy and usability (ISO Citation2013). Therefore, in this cluster, we assess the quality of data by using two approaches, namely quality control frameworks during a disaster (Goodchild and Glennon Citation2010; Mirbabaie et al. Citation2016; Chen et al. Citation2017; Raja and Thomas Citation2019) and after a disaster (Koswatte et al. Citation2015; Fritz et al. Citation2017; Solís et al. Citation2018; Yan et al. Citation2017; Yuan and Liu Citation2018; Pezzica et al. Citation2019). Quality control during a disaster is vital for saving more lives or reducing the damage caused by a disaster. The analyses performed on data after a disaster can provide important insights; however, they are primarily implemented in various applications to improve data quality during a disaster.
Currently, the biggest challenges for crowdsourcing are data quality and a way to engage and sustain citizen participation over the longer term (Fritz et al. Citation2017). Great potential is recognized in exploring the motivations of contributors, whose contributions are later used in applications to correct the quality of streaming data. In this way, data gaps in applications (Yuan and Liu Citation2018) and even systems, such as Critical Infrastructure (CI) (Fekete et al. Citation2015) or SDI are addressed (Koswatte et al. Citation2015).
The increasing prevalence of internet access and mobile devices has boosted the applications of DM, but ensuring data quality, including aspects, such as completeness and accuracy, remains key. To address these issues, there are two primary approaches: Quality control during and after a disaster, the latter improving data quality in real-time scenarios. While crowdsourcing shows promise, challenges, such as long-term citizen participation and ensuring data quality remain, highlighting the need to understand participants’ motivations and address data gaps in various applications and systems. Moreover, further research is needed to explore how to engage and sustain citizen participation to ensure the quality of data collected through crowdsourcing.
3.3. VGI quality challenges according to the PPRR theory
From the collected articles, we extract articles divided by the dominant PPRR phase as test training sets of the DM cycle to identify the corresponding data quality challenges in VGI. Of the 92 articles related to quality, 18 were selected that contained clear labels and references to individual phases of the DM cycle. Therefore, we categorized the articles based on the mentions of the identified linking words in the abstract from the previous steps combined with the mentions of the keywords. shows the identified words associated with each phase and the number of articles. Since we wanted to identify the primary phase for each article, we filtered the data using link words and synonyms.
Table 1. A training data set for observed articles in quality dataset related to the DM cycle.
We used a data training test for a predictive model to classify other articles in a quality set by phase of PPRR cycle. We classify articles by using a Logistic Regression learner. The predictive model achieved a classification accuracy score of 0.83, indicating that it was able to correctly classify 83% of the articles that it predicted as being in the PPRR cycle. This indicates that the model was able to successfully identify the phase of the PPRR cycle that each article fell in. In the figure below, we see the distribution of classified articles using logistic regression.
According to the proposed classification, as can be seen in , more than half articles in the quality collection are related to disaster response (55.43%), followed by the prevention category (28.26%). This indicates that the researchers are mostly focused on the response to disasters. This is likely since these topics are a relatively new area of research, and there is much to be explored and studied. Additionally, the disaster response category is likely more immediately relevant to practitioners and policy makers, as it is concerned with providing rapid and effective interventions in times of crisis. The other two categories, recovery (13.04%) and preparedness (3.26%) tend to focus on longer-term issues and strategies, which may be less appealing to the research community.
Figure 6. Frequency of articles according to PPRR cycle. The figure was created using Orange data mining (Demšar et al. Citation2013).
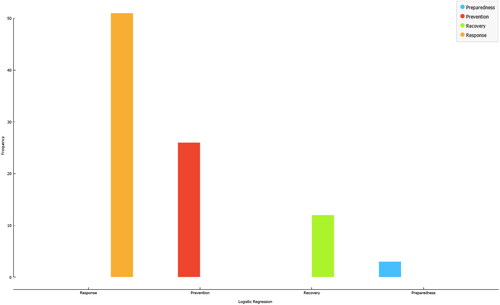
Reviewing the categorized articles, we conclude that VGI is present in all phases of the PPRR (), in the first two mainly through quality assurance activities. In contrast, VGI manifests itself in the other two phases through quality assessments. One of the biggest challenges with VGI is motivating participants. Lack of motivation leads to fewer volunteers, which directly affects data quality and willingness to evaluate the data. The challenge of motivation is more pronounced in the first two phases of the PPRR cycle, as people become more engaged and active in VGI campaigns after a disaster. Citizen Sensing continues to be triggered by the increasing number of disasters, creating awareness of the post-disaster response and recovery phase, which is even more intensified by the severity of a disaster. This phenomenon entices individuals to develop altruistic traits by providing VGI, offering an easy and inexpensive way for the public to help (Pepe et al. Citation2018) within the framework of digital humanitarianism (Burns Citation2015; Meier Citation2015).
Hedberg et al. (Citation2019) studied contributor behaviour and interactions to address trust, transparency, and reliability. They have qualified the behaviour of OSM contributors through a multigraph approach to obtain a more comprehensive map of contributor interactions. However, the data collected is constantly compromised by the limitations of crowdsourced projects, such as the need for demographic and location information of contributors, which is often not collected or incomplete due to commercial interests (See et al. Citation2016). Geospatial data quality is continuously improving, which has been encouraged by the rapid emergence of information technology in DM (Bekker and Felus Citation2019).
Quality criteria of voluntary data were identified in a comprehensive review by Mirbabaie et al. (Citation2016): accuracy, completeness, consistency, trustworthiness, timeliness and validity. In contrast, Aissi and Sboui (Citation2017) took a narrower approach and used only completeness, freshness and trust. Foody (Citation2014) focused on the impact of missing data as a critical challenge for voluntary data, while Koswatte et al. (Citation2015) proposed a semantic approach for unstructured data to address this issue. In addition, some authors advocate a holistic approach to data quality in DM, encompassing spatial, temporal and causal context and the characteristics of data collection tools (Fekete et al. Citation2015).
4. Discussion
Our main goal was to examine the state of the literature in the scientific databases considering the VGI quality with a particular application within the DM cycle. The three-step strategy, presented in Section 3, has identified some of the main highlights in this context (Section 4), starting from thematic evolution (), building on the five established research topics () up to the critical challenges of VGI quality in PPRR theory (). Unique to our approach, we found it imperative to differentiate between general VGI application features and those specific to DM.
Figure 7. PPRR approach to VGI quality challenges (adopted from Ehnis (Citation2018)).
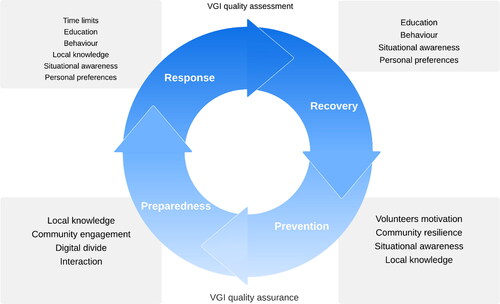
4.1. VGI quality challenges in general
Considering previous research, it is clear that the field of VGI has attracted a lot of attention, especially in terms of its potential. However, our research pivots this discussion around by highlighting the specific applicability of VGI within the DM cycle. This shift in focus finds its basis in evolving perspectives on spatial data quality. Although Siebritz et al. (Citation2012) and Verplanke et al. (Citation2016) have highlighted this issue, our study goes deeper and explores the intricacies of VGI constraints in real DM scenarios. This in-depth investigation sets our research apart from others, especially when compared to studies, such as the one on OpenStreetMap roads in developing countries by Čekada Triglav and Lisec (Citation2019). While they have underlined the potential of VGI in geographic settings, we have tried to contextualize this within the DM paradigm.
In parallel, the discourse around VGI data quality is vibrant. For example, a well-organized VGI campaign combined with efficient platforms and guidance from volunteers can enhance data quality, as shown by Albuquerque et al. (Citation2016), who report remarkable scores for accuracy, sensitivity and precision. The wisdom-of-the-crowd approach, as advocated by Foody et al. (Citation2018), further contributes to quality improvement, especially when using weighted voting procedures based on the nuances of the contributed data.
As VGI initiatives gain traction, the call for rigorous quality control mechanisms grows louder. Gómez-Barron et al. (Citation2019) highlight this, advocating for processes, such as peer reviews, feedback loops and expert-driven validation to improve the accuracy and reliability of data. While the VGI landscape is promising, it is not without challenges. Many authors, despite their sincere intentions, may lack technical expertise, inadvertently introducing inaccuracies. This highlights the importance of frameworks to assess the credibility and accuracy of VGI data, a point echoed by Fritz et al. (Citation2017). Quality checks such as those used by Mejri et al. (Citation2017), which match crowdsourced data with official datasets, are an example of this approach. At the same time, new protocols are being developed to improve the quality of VGI data, as proposed by Mooney et al. (Citation2016).
However, we need to be aware in this area that voluntarily provided geographic data is, at its core, derived from voluntary response samples. As Fonte et al. (Citation2017) have pointed out, the biases associated with such data can vary greatly depending on the task and expertise of the individual participant. Volunteers have different assessment skills and decision-making criteria, which can lead to different interpretations of the data. Haklay (Citation2013) therefore suggests a stratified classification of contributors, ranging from simple sensors to participants in complicated tasks, a framework that may mitigate some of the inherent biases of VGI.
4.2. VGI quality challenges in DM
By diving deep into the DM-specific challenges, our research nuances the complications facing VGI. Chen et al.’s (Citation2017) study highlighted these challenges, and our work uniquely differentiates them between phases of DM PPRR. While issues related to the credibility of data from VGI sources on social media are consistent with previous findings, our study provides, among other things, a deeper exploration in the context of early disaster detection and highlights the reliability of social media platforms as important data sources in densely populated areas and volunteer motivation.
Moreover, the multifaceted dimensions of VGI quality, in the context of DM, introduce distinct challenges and factors to consider. Communication is critical in disasters as it provides relevant information that helps with evacuation plans and increases support for damage assessment. Here, the participatory character of VGI, as demonstrated by Feng et al. (Citation2022), becomes particularly clear here. They point to the rapid mobilization of platforms, such as the Humanitarian OpenStreetMap Team in response to disasters and highlight the potential of collaborative mapping to improve the quality and quantity of reports on natural disasters. However, they also point out that while such data directly informs disaster response, it is rarely integrated into social media VGI, highlighting a gap in the utilization of these data sources.
Feng et al. (Citation2022) also show that crowdsourced information, such as that collected via the U-Flood project or the Ushahidi platform, often has higher location accuracy than typical VGI from social media. This suggests a valuable intersection where participatory VGI can complement social media data and contribute to a more robust and accurate disaster response. The VGI could be an important source of this information as much of the data is available online to the public (Haworth et al. Citation2018). According to Chen et al. (Citation2017) and Li et al. (Citation2021), challenges in using VGI for DM include data availability, data quality, data management and legal issues.
As we delve into the VGI quality challenges in DM, it is important to recognize the geographical differences in the application of VGI. The underrepresentation of high-risk areas in VGI research and application is a pressing issue, particularly in regions with vulnerable populations and infrastructure susceptible to climate-related hazards. This aligns with the findings of Tzavella et al. (Citation2022), which point to a significant need for targeted funding to improve disaster preparedness and response capacity in such high-risk countries, especially those with limited economic capacity. The integration of VGI into DM processes provides a proactive approach to the timely dissemination of critical information that aids in mitigation, preparedness, response and recovery. This proactive use of VGI is critical not only for immediate response, but also for the long-term recovery and resilience of affected communities.
While VGIs are an important source of information during disasters, their effectiveness can be hampered by the same geographic inequalities that affect the regions they are intended for. It is therefore imperative that future research and funding strategies prioritise the inclusion and active participation of these underrepresented regions in the production and application of VGIs. In this way, we can work towards closing the gap in DM and ensure that VGIs fully realize their potential as a tool for global resilience.
Social media as a VGI reservoir for DM shows remarkable effectiveness, especially in early disaster detection. Apart from privacy issues, trust issues with such data can potentially be mitigated by scale and diversity. The challenges we have identified span the entire framework of DM PPRR (as shown in ). The literature on VGI and DM distinguishes between a quality assurance perspective and a quality assessment perspective. In the recovery phase, for example, voluntary participation often uses VGI sources, such as aerial photography and social media to assess damage, as demonstrated after Hurricane Sandy (Munro et al. Citation2013) and Zhang et al. (Citation2016). However, this approach is not immune to quality issues, with several studies pointing to inconsistencies between volunteer and expert assessments (Tavra et al. Citation2021).
Volunteer motivation within DM is a specificity driven by the need for information (Zook et al. Citation2010; Hjorth and Kim Citation2011) and a sense of control over the situation (Riccardi Citation2016), and the volunteer engagement curve can be strengthened through targeted training (Rogers Citation2011; Riccardi Citation2016; Haworth Citation2016; Zhang, Fan et al. Citation2019).
Gómez-Barron et al. (2019) presented a framework that lists motivational factors from recognition to community engagement and also suggests different engagement strategies. According to the study, the framework identifies four main needs that drive volunteers’ motivation to contribute to volunteer geographic information systems (VGIS): the need for information, the need for recognition and reputation, the need for learning and personal growth and the need for social interaction and community engagement. In addition, the framework highlights several activities that can be used to encourage contributions, including providing clear goals and feedback, creating supportive and inclusive communities, offering training and capacity building and recognizing and rewarding volunteers for their contributions. Citizens can and should be integrated into the disaster monitoring system as one of its key elements (Wahlström Citation2015). However, it is crucial to determine what is behind the process and how to provide reliable data that can be used for decision-making (Tavra et al. Citation2021). When it comes to data quality in DM, a key focus is on the behaviour and interactions of contributors to investigate their transparency, trust and reliability (Buffi Citation2018). For example, Haworth et al. (Citation2018) cite trust issues as one of the biggest challenges in VGI.
Due to the need to address and anticipate disasters, humanitarian operations are evolving significantly (Jeansoulin Citation2016). Unfortunately, the increasing number of disasters and the influx of different data and analyses continue to create new opportunities for DM, which is an important contribution to the use of VGI platforms (Zahra et al. Citation2017). However, this process requires continuous improvement in the reliability and accuracy of VGI. In contrast to the broader VGI discourse, the field of DM requires a particular focus on volunteer motivation due to its emerging and high-profile nature, as we have drawn parallels with several studies highlighting the various motivational factors that drive VGI contributions within the field DM. As the humanitarian sector evolves with the increasing frequency of disasters and the flood of data, VGI platforms in DM have proven critical, as noted by Zahra et al. (Citation2017). Given the urgency and high stakes of DM, it is crucial to continuously improve the accuracy and credibility of VGI while increasing volunteer motivation.
5. Conclusions and future directions
The use of crowdsourcing and VGI in DM introduces a variety of challenges, particularly in relation to data quality, bias and trust. Despite these concerns, the motivation of volunteers in disasters highlights the potential for further development of VGI tools, particularly for processing vast amounts of data in crises to improve DM systems across stages. Our review offers a new perspective to the VGI literature by highlighting the role of VGI within the DM cycle and contributing to the ongoing discourse of many geospatial experts working on Big Data in DM.
Our findings point to several avenues for future research. There is an urgent need to further investigate the demographics, social influences, economic biases, transparency and trustworthiness while maintaining volunteer privacy. The ubiquitous role of social media, which presents both a challenge and an opportunity, underscores the need for DM to skilfully harness information from the contributors. Moreover, as indicated in , the motivation and preferences of contributors play a crucial role, which calls for further empirical studies. The horizon seems promising, with perspectives, such as integrating machine learning, refining VGI platforms and emphasizing active training of volunteers to ensure the quality of VGI.
In our research methodology, we based our preliminary assessments on the abstracts of the shortlisted entries and considered them as key indicators for our in-depth review. This hierarchical clustering of abstracts proved efficient for the thematic categorization of articles. However, on closer examination, we note certain limitations. Because we relied solely on the abstracts, we may have missed subtle details contained in the full texts. This methodological decision was driven by the need to navigate a vast landscape of literature while maintaining structure. Nonetheless, future efforts may include a more thorough review of full texts to further strengthen the robustness of our findings.
In summary, this article has shed light on the challenges and opportunities of integrating VGI into DM, and we emphasize the importance of DM organizations equipping themselves to maximize the benefits of crowdsourcing data. With concerted efforts, VGI experts can work together to develop replicable, sophisticated systems to promote awareness and engagement among public and private stakeholders.
Abbreviations | ||
VGI | = | Volunteered Geographic Information |
DM | = | Disaster Management |
WoS | = | Web of Science |
BoW | = | Bag-of-Words |
IR | = | Information Retrieval |
CNNs | = | Convolutional Neural Networks |
OSM | = | Open Street Map |
SNIC | = | Simple Non-Iterative Clustering |
CI | = | Critical Infrastructure |
SDI | = | Spatial Data Infrastructure |
PPRR | = | Prevention, Preparedness, Response, Recovery |
ISO | = | International Organization for Standardization |
VGIS | = | Volunteered Geographic Information Systems |
Disclosure statement
No potential conflict of interest was reported by the authors.
Data and codes availability statement
The data and codes that support the findings of this study are available at the private link: https://figshare.com/s/48fbada166ead6c6e6e8.
Additional information
Funding
References
- Abrahams J. 2001. Disaster management in Australia: the national emergency management system. Emerg Med (Fremantle). 13(2):165–173. doi: 10.1046/j.1442-2026.2001.00205.x.
- Ahmad K, Pogorelov K, Riegler M, Conci N, Halvorsen P. 2019. Social media and satellites: disaster event detection, linking and summarisation. Multimed Tools Appl. 78(3):2837–2875. doi: 10.1007/s11042-018-5982-9.
- Ahmad K, Riegler M, Pogorelov K, Conci N, Halvorsen P, De Natale F. 2017. Jord: a system for collecting information and monitoring natural disasters by linking social media with satellite imagery. Proceedings of the. 15th International Workshop on Content-Based Multimedia Indexing; Jun 19–21; Florence, Italy. New York: ACM. p. 1–6. doi: 10.1145/3078971.3079013.
- Aissi S, Sboui T. 2017. Towards evaluating geospatial metadata quality in the context of VGI. Proc Comput Sci. 109:686–691. doi: 10.1016/j.procs.2017.05.377.
- Albuquerque J, Herfort B, Eckle M. 2016. The tasks of the crowd: a typology of tasks in geographic information crowdsourcing and a case study in humanitarian mapping. Remote Sens. 8(10):859. doi: 10.3390/rs8100859.
- Antoniou V, Skopeliti A. 2015. Measures and indicators of VGI quality: an overview. ISPRS Ann Photogramm Remote Sens Spatial Inf Sci. II-3/W5:345–351. doi: 10.5194/isprsannals-II-3-W5-345-2015.
- Arapostathis SG. 2020. Fundamentals of volunteered geographic information in disaster management related to floods. Flood impact mitigation and resilience enhancement. London: IntechOpen. doi: 10.5772/intechopen.92225.
- Aria M, Cuccurullo C. 2017. Bibliometrix: an R-tool for comprehensive science mapping analysis. J Informetr. 11(4):959–975. doi: 10.1016/j.joi.2017.08.007.
- Bajracharya B, Childs I, Hastings P. 2011. Climate change adaptation through land use planning and disaster management: local government perspectives from Queensland. Proceedings of 17th Pacific Rim Real Estate Society Conference; Jan 16–19; Gold Coast, Australia. Sydney, Australia: PRRES Inc. p. 16–19.
- Becker D, Bendett S. 2015. Crowdsourcing solutions for disaster response: examples and lessons for the US government. Proc Eng. 107:27–33. doi: 10.1016/j.proeng.2015.06.055.
- Bekker I, Felus Y. 2019. Quality control for crowdsourcing large-scale topographic maps. Int Arch Photogramm Remote Sens Spatial Inf Sci. XLII-2/W13(2/W13):1201–1205. doi: 10.5194/isprs-archives-XLII-2-W13-1201-2019.
- Buffi G. 2018. [Assessment of seismic behaviour of large concrete dams by means of geomatic techniques and finite element modelling] [doctoral dissertation]. Florence, Italy: University of Florence.
- Burns R. 2015. Rethinking big data in digital humanitarianism: practices, epistemologies, and social relations. GeoJournal. 80(4):477–490. doi: 10.1007/s10708-014-9599-x.
- Capineri C. 2016. The nature of volunteered geographic information. European handbook of crowdsourced geographic information. London: Ubiquity Press. p. 15–33. doi: 10.5334/bax.b.
- Camponovo ME, Freundschuh SM. 2014. Assessing uncertainty in VGI for emergency response. Cartograph Geographic Inform Sci. 41(5):440–455. doi: 10.1080/15230406.2014.950332.
- Carter WN. 2008. Disaster management: a disaster manager’s handbook. Manila, Philippines: Asian Development Bank.
- Castillo C. 2016. Big crisis data: social media in disasters and time-critical situations. Cambridge: Cambridge University Press.
- Cetl V, Ioannidis C, Dalyot S, Doytsher Y, Felus Y, Haklay M, Mueller H, Potsiou C, Rispoli E, Siriba D. 2019. New trends in geospatial information: the land surveyors role in the era of crowdsourcing and VGI. FIG Publication No 73. ISBN 978-87-92853-86-8. https://www.fig.net/resources/publications/figpub/pub73/figpub73.asp.
- Čekada MT, Lisec A. 2019. Opportunities for using the volunteered geographic information within the national spatial infrastructure. Geod Vestn. 63(02):199–212. doi: 10.15292/geodetski-vestnik.2019.02.199-212.
- Chen H, Zhang WC, Deng C, Nie N, Yi L. 2017. Volunteered geographic information for disaster management with application to earthquake disaster databank and sharing platform. IOP Conf Ser Earth Environ Sci. 57(1):012015. doi: 10.1088/1755-1315/57/1/012015.
- Chow TE, Chien J, Meitzen K. 2023. Validating the quality of volunteered geographic information (VGI) for flood modeling of Hurricane Harvey in Houston, Texas. Hydrology. 10(5):113. doi: 10.3390/hydrology10050113.
- Coppola DP. 2006. Introduction to international disaster management. Amsterdam, Netherlands: Elsevier. doi: 10.1016/b978-0-12-801477-6.09002-x.
- Cronstedt M. 2002. Prevention, preparedness, response, recovery-an outdated concept? Austral J Emergency Manag. 17:10–13.
- Danylo O, Moorthy I, Sturn T, See L, Laso Bayas J-C, Domian D, Fraisl D, Giovando C, Girardot B, Kapur R, et al. 2018. The Picture Pile tool for rapid image assessment: a demonstration using Hurricane Matthew. ISPRS Ann Photogramm Remote Sens Spatial Inf Sci. IV-4:27–32. doi: 10.5194/isprs-annals-IV-4-27-2018.
- Demetriou D, Campagna M, Racetin I, Konecny M. 2017. Integrating spatial data infrastructures (SDIs) with volunteered geographic information (VGI) for creating a global GIS platform. In: Foody G, See L, Fritz, Mooney P, Olteanu-Raimond AM, Fonte CC, Antoniou V, editors. Mapping and the citizen sensor. London: Ubiquity Press. p. 273–297. doi: 10.5334/bbf.l.
- Demšar J, Curk T, Erjavec A, Gorup Č, Hočevar T, Milutinovič M, Možina M, Polajnar M, Toplak M, Starič A, Štajdohar M, Umek L, Žagar L, Žbontar J, Žitnik M, Zupan B. 2013. Orange: data mining toolbox in Python. J Mach Learn Res. 14(1):2349–2353.
- Dittus M, Quattrone G, Capra L. 2017. Mass participation during emergency response: event-centric crowdsourcing in humanitarian mapping. Proceedings of the 2017 ACM Conference on Computer Supported Cooperative Work and Social Computing, Feb 25–Mar 1; Portland, Oregon. New York: ACM. p. 1290–1303. doi: 10.1145/2998181.2998216.
- Ehnis CF. 2018. [Social media within emergency management organisations-A case study exploring social media utilisation for emergency and disaster management] [doctoral dissertation]. New South Wales, Australia: The University of Sydney Business School.
- Fekete A, Tzavella K, Armas I, Binner J, Garschagen M, Giupponi C, Mojtahed V, Pettita M, Schneiderbauer S, Serre D. 2015. Critical data source; tool or even infrastructure? Challenges of geographic information systems and remote sensing for disaster risk governance. ISPRS Int J Geo-Inf. 4(4):1848–1869. doi: 10.3390/ijgi4041848.
- Feng Y, Huang X, Sester M. 2022. Extraction and analysis of natural disaster-related VGI from social media: review, opportunities and challenges. Int J Geograph Inform Sci. 36(7):1275–1316. doi: 10.1080/13658816.2022.2048835.
- Feng Y, Sester M. 2018. Extraction of pluvial flood relevant volunteered geographic information (VGI) by deep learning from user generated texts and photos. ISPRS Int J Geo-Inf. 7(2):39. doi: 10.3390/ijgi7020039.
- Fonte CC, Antoniou V, Bastin L, Estima J, Arsanjani JJ, Bayas JCL, See L, Vatseva R. 2017. Assessing VGI data quality. In: Foody G, See L, Fritz S, Mooney P, Olteanu-Raimond AM Fonte CC, Antoniou V, editors. Mapping and the citizen sensor. London: Ubiquity Press. p. 137–163. doi: 10.5334/bbf.g.
- Foody GM. 2014. Rating crowdsourced annotations: evaluating contributions of variable quality and completeness. Int J Digital Earth. 7(8):650–670. doi: 10.1080/17538947.2013.839008.
- Foody GM, See L, Fritz S, van der Velde M, Perger C, Schill C, Boyd DS, Comber A. 2015. Accurate attribute mapping from volunteered geographic information: issues of volunteer quantity and quality. Cartograph J. 52(4):336–344. doi: 10.1179/1743277413y.0000000070.
- Foody G, See L, Fritz S, Moorthy I, Perger C, Schill C, Boyd D. 2018. Increasing the accuracy of crowdsourced information on land cover via a voting procedure weighted by information inferred from the contributed data. ISPRS Int J Geo-Inf. 7(3):80. doi: 10.3390/ijgi7030080.
- Fritz S, See L, Brovelli M, See L, Fritz S. 2017. Motivating and sustaining participation in VGI. In: Foody G, Mooney P, Olteanu-Raimond AM, Fonte CC, Antoniou V, editors. Mapping and the citizen sensor. London: Ubiquity Press. p. 93–117. doi: 10.5334/bbf.e.
- Fitriany AA, Flatau PJ, Khoirunurrofik K, Riama NF. 2021. Assessment on the use of meteorological and social media information for forest fire detection and prediction in Riau, Indonesia. Sustainability. 13(20):11188. doi: 10.3390/su132011188.
- Galasso F, Rongier C, Lauras M, Gourc D. 2011. Disaster management control: decision support through performance assessment. IFAC Proc Vol. 44(1):10758–10763. doi: 10.3182/20110828-6-IT-1002.01621.
- Goodchild MF, Glennon JA. 2010. Crowdsourcing geographic information for disaster response: a research frontier. Int J Digital Earth. 3(3):231–241. doi: 10.1080/17538941003759255.
- Goodchild MF. 2007. Citizens as sensors: the world of volunteered geography. GeoJournal. 69(4):211–221. doi: 10.1007/s10708-007-9111-y.
- Gómez-BarrÓn JP, Manso-Callejo MÁ, Alcarria R. 2019. Needs, drivers, participants and engagement actions: a framework for motivating contributions to volunteered geographic information systems. J Geogr Syst. 21(1):5–41. doi: 10.1007/s10109-018-00289-5.
- Rosario Michel G, Gonzalez-Campos ME, Manzano Aybar F, Jiménez Durán T, Crompvoets J. 2023. Identifying critical factors to enhance SDI performance for facilitating disaster risk management in small island developing states. Surv Rev. 55(389):114–126. doi: 10.1080/00396265.2021.2024969.
- Haklay M. 2013. CitSciand volunteered geographic information: overview and typology of participation. Crowdsourcing geographic knowledge. Dordrecht, the Netherlands: Springer Netherlands; p. 105–122. doi: 10.1007/978-94-007-4587-2_7.
- Haworth B. 2016. Emergency management perspectives on volunteered geographic information: opportunities, challenges, and change. Comput Environ Urban Syst. 57:189–198. doi: 10.1016/j.compenvurbsys.2016.02.009.
- Haworth B, Bruce E. 2015. A review of volunteered geographic information for disaster management. Geograph Compass. 9(5):237–250. doi: 10.1111/gec3.12213.
- Haworth B, Bruce E, Whittaker J, Read R. 2018. The good, the bad, and the uncertain: contributions of volunteered geographic information to community disaster resilience. Front Earth Sci. 6:183. doi: 10.3389/feart.2018.00183.
- Hedberg TD, Krima S, Camelio JA. 2019. Method for enabling a root of trust in support of product data certification and traceability. J Comput Inf Sci Eng. 19(4):1. doi: 10.1115/1.4042839.
- Heinzelman J, Waters C. 2010. Crowdsourcing crisis information in disaster-affected Haiti. Washington (DC): US Institute of Peace.
- Hicks A, Barclay J, Chilvers J, Armijos MT, Oven K, Simmons P, Haklay M. 2019. Global mapping of citizen science projects for disaster risk reduction. Front Earth Sci. 7. doi: 10.3389/feart.2019.00226.
- Hjorth L, Kim KH. 2011. The mourning after: a case study of social media in the 3.11 earthquake disaster in Japan. Telev New Media. 12(6):552–559. doi: 10.1177/1527476411418351.
- Hodges A. 2000. Emergency risk management. Risk Manag. 2(4):7–18. doi: 10.1057/palgrave.rm.8240064.
- Imran M, Castillo C, Lucas J, Meier P, Vieweg S. 2014. AIDR: artificial intelligence for disaster response. Proceedings of the 23rd International Conference on World Wide Web; Apl 7–11; Seoul, Korea. New York: ACM. p. 159–162. doi: 10.1145/2567948.2577034.
- ISO. 2013. ISO 19157:2013–Geographic information–data quality. Geneva, Switzerland: International Organization for Standardization.
- Jeansoulin R. 2016. Review of forty years of technological changes in geomatics toward the big data paradigm. ISPRS Int J Geo-Inf. 5(9):155. doi: 10.3390/ijgi5090155.
- Kankanamge N, Yigitcanlar T, Goonetilleke A, Kamruzzaman M. 2019. Can volunteer crowdsourcing reduce disaster risk? A systematic review of the literature. Int J Disaster Risk Reduct. 35:101097. doi: 10.1016/j.ijdrr.2019.101097.
- Kashian A, Rajabifard A, Richter KF, Chen Y. 2019. Automatic analysis of positional plausibility for points of interest in OpenStreetMap using coexistence patterns. Int J Geograph Inform Sci. 33(7):1420–1443. doi: 10.1080/13658816.2019.1584803.
- Kocaman S, Anbaroglu B, Gokceoglu C, Altan O. 2018. A review on citizen science (CITSCI) applications for disaster management. Int Arch Photogramm Remote Sens Spatial Inf Sci. XLII-3/W4:301–306. doi: 10.5194/isprs-archives-XLII-3-W4-301-2018.
- Koswatte S, McDougall K, Liu X. 2015. SDI and crowdsourced spatial information management automation for disaster management. Survey Rev. 47(344):307–315. doi: 10.1179/1752270615y.0000000008.
- Koswatte S, McDougall K, Liu X. 2018. VGI and crowdsourced data credibility analysis using spam email detection techniques. Int J Digital Earth. 11(5):520–532. doi: 10.1080/17538947.2017.1341558.
- Laylavi F, Rajabifard A, Kalantari M. 2016. A multi-element approach to location inference of twitter: a case for emergency response. ISPRS Int J Geo-Inf. 5(5):56. doi: 10.3390/ijgi5050056.
- Li H, Zech J, Ludwig C, Fendrich S, Shapiro A, Schultz M, Zipf A. 2021. Automatic mapping of national surface water with OpenStreetMap and Sentinel-2 MSI data using deep learning. Int J Appl Earth Obs Geoinf. 104:102571. doi: 10.1016/j.jag.2021.102571.
- Li Z, Wang C, Emrich CT, Guo D. 2018. A novel approach to leveraging social media for rapid flood mapping: a case study of the 2015 South Carolina floods. Cartograph Geograph Inform Sci. 45(2):97–110. doi: 10.1080/15230406.2016.1271356.
- Lin WY, Wu TH, Tsai MH, Hsu WC, Chou YT, Kang SC. 2018. Filtering disaster responses using crowdsourcing. Autom Constr. 91:182–192. doi: 10.1016/j.autcon.2018.03.016.
- Lowrie C, Kruczkiewicz A, McClain SN, Nielsen M, Mason SJ. 2022. Evaluating the usefulness of VGI from Waze for the reporting of flash floods. Sci Rep. 12(1):5268. doi: 10.1038/s41598-022-08751-7.
- Manfré LA, Hirata E, Silva JB, Shinohara EJ, Giannotti MA, Larocca APC, Quintanilha JA. 2012. An analysis of geospatial technologies for risk and natural disaster management. ISPRS Int J Geo-Inf. 1(2):166–185. doi: 10.3390/ijgi1020166.
- Meier P. 2015. Digital humanitarians. How big data is changing the face of humanitarian. Boca Raton (FL): CRC Press.
- Mejri O, Menoni S, Matias K, Aminoltaheri N. 2017. Crisis information to support spatial planning in post disaster recovery. Int J Disaster Risk Reduct. 22:46–61. doi: 10.1016/j.ijdrr.2017.02.007.
- Mirbabaie M, Stieglitz S, Volkeri S. 2016. Volunteered geographic information and its implications for disaster management. 49th Hawaii International Conference on System Sciences (HICSS); Jan 5–8; Koloa, US. New Jersey: IEEE. p. 207–216. doi: 10.1109/hicss.2016.33.
- Moghadas M, Rajabifard A, Fekete A, Kötter T. 2022. A framework for scaling urban transformative resilience through utilizing volunteered geographic information. ISPRS Int J Geo-Inf. 11(2):114. doi: 10.3390/ijgi11020114.
- Moghadas M, Fekete A, Rajabifard A, Kötter T. 2023. The wisdom of crowds for improved disaster resilience: a near-real-time analysis of crowdsourced social media data on the 2021 flood in Germany. GeoJournal. 88(4):4215–4241. doi: 10.1007/s10708-023-10858-x.
- Mooney P, Minghini M, Laakso M, Antoniou V, Olteanu-Raimond AM, Skopeliti A. 2016. Towards a protocol for the collection of VGI vector data. ISPRS Int J Geo-Inf. 5(11):217. doi: 10.3390/ijgi5110217.
- Mooney P, Sun H, Yan L. 2011. VGI as a dynamically updating data source in location-based services in urban environments. Proceedings of the 2nd International Workshop on Ubiquitous Crowdsourcing; Sep 17–21; Beijing, China. New York: ACM. p. 13–16. doi: 10.1145/2030100.2030105.
- Mulder F, Ferguson J, Groenewegen P, Boersma K, Wolbers J. 2016. Questioning big data: crowdsourcing crisis data towards an inclusive humanitarian response. Big Data Soc. 3(2):205395171666205. doi: 10.1177/2053951716662054.
- Munro R, Schnoebelen T, Erle S. 2013. Quality analysis after action report for the crowdsourced aerial imagery assessment following hurricane sandy. Proceedings of the 10th International ISCRAM Conference; May 12–15; Baden-Baden, Germany. Baden-Baden, Germany: Karlsruher Institut fur Technologie.
- Neto FRA, Santos CA. 2018. Understanding crowdsourcing projects: a systematic review of tendencies, workflow, and quality management. Inf Process Manage. 54(4):490–506. doi: 10.1016/j.ipm.2018.03.006.
- Ostermann FO, Spinsanti L. 2011. A conceptual workflow for automatically assessing the quality of volunteered geographic information for crisis management. Proceedings of AGILE 2011; Aug 7–13; Salt Lake City. New Jersey: IEEE. p. 1–6.
- Pepe A, Bonano M, Bordogna G, Brovelli M, Calo F, Carrara P, Congedo L, Frigerio L, Imperatore P, Lanari R, Lanucara S, Manzo M, Munafo M. 2018. The 'urban geomatics for bulk information generation, data assessment and technology awareness’ project: detection, representation and analysis of the urban scenario changes. IGARSS 2018–International Geoscience and Remote Sensing Symposium; Jul 22–27; Valencia, Spain. New Jersey: IEEE. doi:10.1109/igarss.2018.8518843.
- Pezzica C, Piemonte A, Bleil de Souza C, Cutini V. 2019. Photogrammetry as a participatory recovery tool after disasters: a grounded framework for future guidelines. Int Arch Photogramm Remote Sens Spatial Inf Sci. XLII-2/W15:921–928. doi: 10.5194/isprs-archives-XLII-2-W15-921-2019.
- Poorazizi ME, Hunter AJ, Steiniger S. 2015. A volunteered geographic information framework to enable bottom-up disaster management platforms. ISPRS Int J Geo-Inf. 4(3):1389–1422. doi: 10.3390/ijgi4031389.
- Poser K, Dransch D. 2010. Volunteered geographic information for disaster management with application to rapid flood damage estimation. Geomatica. 64(1):89–98.
- Priya S, Bhanu M, Dandapat SK, Ghosh K, Chandra J. 2018. Characterising infrastructure damage after earthquake: a split-query based IR approach. ACM International Conference on Advances in Social Networks Analysis and Mining (ASONAM); Aug 28–31; Barcelona, Spain. New Jersey: IEEE. p. 202–209.
- Raja G, Thomas A. 2019. Safer: crowdsourcing based disaster monitoring system using software defined fog computing. Mobile Netw Appl. 24(5):1414–1424. doi: 10.1007/s11036-019-01267-x.
- Riccardi MT. 2016. The power of crowdsourcing in disaster response operations. Int J Disaster Risk Reduct. 20:123–128. doi: 10.1016/j.ijdrr.2016.11.001.
- Rogers P. 2011. Development of Resilient Australia: enhancing the PPRR approach with anticipation, assessment and registration of risks. Austral J Emergency Manag. 26: 54–58. doi: 10.3316/ielapa.80630554640444
- Sachdeva S, McCaffrey S, Locke D. 2017. Social media approaches to modeling wildfire smoke dispersion: spatiotemporal and social scientific investigations. Inform Commun Soc. 20(8):1146–1161. doi: 10.1080/1369118X.2016.1218528.
- See L, Mooney P, Foody G, Bastin L, Comber A, Estima J, Fritz S, Kerle N, Jiang B, Laakso M, et al. 2016. Crowdsourcing, citizen science or volunteered geographic information? The current state of crowdsourced geographic information. ISPRS Int J Geo-Inf. 5(5):55. doi: 10.3390/ijgi5050055.
- Senaratne H, Mobasheri A, Ali AL, Capineri C, Haklay M. 2017. A review of volunteered geographic information quality assessment methods. Int J Geograph Inform Sci. 31(1):139–167. doi: 10.1080/13658816.2016.1189556.
- Siebritz L, Sithole G, Zlatanova S. 2012. Assessment of the homogeneity of volunteered geographic information in South Africa. XXII ISPRS Congress, Commission IV, Melbourne; Australia 25 August-1 September 2012; IAPRS XXXIX-B4 2012. French, German: International Society for Photogrammetry and Remote Sensing (ISPRS). doi: 10.5194/isprsarchives-XXXIX-B4-553-2012.
- Solís P, McCusker B, Menkiti N, Cowan N, Blevins C. 2018. Engaging global youth in participatory spatial data creation for the UN sustainable development goals: the case of open mapping for malaria prevention. Appl Geogr. 98:143–155. doi: 10.1016/j.apgeog.2018.07.013.
- Tavra M, Racetin I, Peroš J. 2021. The role of crowdsourcing and social media in crisis mapping: a case study of a wildfire reaching Croatian City of Split. Geoenviron Dis. 8(1):1–16. doi: 10.1186/s40677-021-00181-3.
- Toujani R, Akaichi J. 2019. Event news detection and citizens community structure for disaster management in social networks. Online Inform Rev. 43(1):113–132. doi: 10.1108/OIR-03-2018-0091.
- Tsai PH, Lin YJ, Ou YZ, Chu ETH, Liu JW. 2014. A framework for fusion of human sensor and physical sensor data. IEEE Trans Syst Man Cybern Syst. 44(9):1248–1261. doi: 10.1109/TSMC.2014.2309090.
- Tzavella K, Skopeliti A, Fekete A. 2022. Volunteered geographic information use in crisis, emergency and disaster management: a scoping review and a web atlas. Geo-Spatial Inform Sci. 1–32. doi: 10.1080/10095020.2022.2139642.
- Verplanke J, McCall MK, Uberhuaga C, Rambaldi G, Haklay M. 2016. A shared perspective for PGIS and VGI. Cartograph J. 53(4):308–317. doi: 10.1080/00087041.2016.1227552.
- Vijaya AS, Bateja R. 2017. A review on hierarchical clustering algorithms. J Eng Appl Sci. 12(24):7501–7507. doi: 10.36478/jeasci.2017.7501.7507.
- Wahlström M. 2015. New Sendai framework strengthens focus on reducing disaster risk. Int J Disaster Risk Sci. 6(2):200–201. doi: 10.1007/s13753-015-0057-2.
- Xiao Y, Huang Q, Wu K. 2015. Understanding social media data for disaster management. Nat Hazards. 79(3):1663–1679. doi: 10.1016/b978-0-12-819047-0.00015-9.
- Yan Y, Eckle M, Kuo CL, Herfort B, Fan H, Zipf A. 2017. Monitoring and assessing post-disaster tourism recovery using geotagged social media data. ISPRS Int J Geo-Inf. 6(5):144. doi: 10.3390/ijgi6050144.
- Yan Y, Feng CC, Huang W, Fan H, Wang YC, Zipf A. 2020. Volunteered geographic information research in the first decade: a narrative review of selected journal articles in GIScience. Int J Geograph Inform Sci. 34(9):1765–1791. doi: 10.1080/13658816.2020.1730848.
- Yang J, Yu M, Qin H, Lu M, Yang C. 2019. A twitter data credibility framework—Hurricane Harvey as a use case. IJGI. 8(3):111. doi: 10.3390/ijgi8030111.
- Yao W, Zhang C, Saravanan S, Huang R, Mostafavi A. 2020. Weakly-supervised fine-grained event recognition on social media texts for disaster management. AAAI. 34(01):532–539. doi: 10.1609/aaai.v34i01.5391.
- Yuan F, Liu R. 2018. Crowdsourcing for forensic disaster investigations: hurricane Harvey case study. Nat Hazards. 93(3):1529–1546. doi: 10.1007/s11069-018-3366-0.
- Yuan J, Chowdhury PKR, McKee J, Yang HL, Weaver J, Bhaduri B. 2018. Exploiting deep learning and volunteered geographic information for mapping buildings in Kano, Nigeria. Sci Data. 5(1):180217. doi: 10.1038/sdata.2018.217.
- Zahra K, Ostermann FO, Purves RS. 2017. Geographic variability of Twitter usage characteristics during disaster events. Geospatial Inform Sci. 20(3):231–240. doi: 10.1080/10095020.2017.1371903.
- Zhang C, Fan C, Yao W, Hu X, Mostafavi A. 2019. Social media for intelligent public information and warning in disasters: an interdisciplinary review. Int J Inf Manage. 49:190–207. doi: 10.1016/j.ijinfomgt.2019.04.004.
- Zhang C, Liu L, Lei D, Yuan Q, Zhuang H, Hanratty T, Han J. 2017. Triovecevent: embedding-based online local event detection in geo-tagged tweet streams. Proceedings of the 23rd ACM SIGKDD International Conference on Knowledge Discovery and Data Mining; Aug 13–17; Halifax, NS Canada. New York: ACM. p. 595–604.
- Zhang C, Zhou G, Yuan Q, Zhuang H, Zheng Y, Kaplan L, Wang S, Han J. 2016. Geoburst: real-time local event detection in geo-tagged tweet streams. Proceedings of the 39th International ACM SIGIR conference on Research and Development in Information Retrieval; Jul 17–21; Pisa, Italy. New Jersey: IEEE. p. 513–522. doi:10.1145/2911451.2911519.
- Zhang D, Zhang Y, Li Q, Plummer T, Wang D. 2019. Crowdlearn: A crowd-ai hybrid system for deep learning-based damage assessment applications. 39th International Conference on Distributed Computing Systems (ICDCS); July 7–9; Dallas, Texas. New Jersey: IEEE. p. 1221–1232.
- Zook M, Graham M, Shelton T, Gorman S. 2010. Volunteered geographic information and crowdsourcing disaster relief: a case study of the Haitian earthquake. World Med Health Policy. 2(2):7–33. doi: 10.2202/1948-4682.1069.