Abstract
Gut microbiota play a key role in the host's health system. Broad antibiotic therapy is known to disrupt the microbial balance affecting pathogenic as well as host-associated microbes. The aim of the present study was to investigate the influence of antibiotic paromomycin on the luminal and mucosa-associated microbiota at the DNA (abundance) and RNA (potential activity) level as well as to identify possible differences. The influence of antibiotic treatment on intestinal microbiota was investigated in 5 healthy individuals (age range: 20–22 years). All participants received the antibiotic paromomycin for 3 d. Fecal samples as well as sigmoidal biopsies were collected before and immediately after cessation of antibiotic treatment as well as after a recovery phase of 42 d. Compartment- and treatment status-specific indicator operational taxonomic units (OTUs) as well as abundance- and activity-specific patterns were identified by 16S rRNA and 16S rRNA gene amplicon libraries and high-throughput pyrosequencing. Microbial composition of lumen and mucosa were significantly different at the DNA compared to the RNA level. Antibiotic treatment resulted in changes of the microbiota, affecting the luminal and mucosal bacteria in a similar way. Several OTUs were identified as compartment- and/or treatment status-specific. Abundance and activity patterns of some indicator OTUs differed considerably. The study shows fundamental changes in composition of gut microbiota under antibiotic therapy at both the potential activity and the abundance level at different treatment status. It may help to understand the complex processes of gut microbiota changes involved in resilience mechanisms and on development of antibiotic-associated clinical diseases.
Introduction
The human gut microbiota outnumber human cells by a factor of 10 and are essential for maintaining homeostasis of the gastrointestinal tract and the host's health.Citation1-5 Gut microbiota show considerable compositional variation between individuals, but remain relatively stable over time.Citation6-9 This specific microbial composition can be disrupted by external factors, such as antibiotic (AB) treatment. As most antibiotics eliminate pathogenic and beneficial host-associated microbes alike, their usage can lead to a dramatic compositional imbalance of the gut microbiota. Subsequent intestinal disorders such as antibiotic-associated diarrhea can occur. Pseudomembranous colitis is a highly relevant clinical example where an overgrowth of Clostridium difficile leads to a potentially life-threatening infectious colitis during the course of antibiotic treatment.Citation10 Other side effects of antibiotics are disturbances of host metabolism and absorption of vitamins, as well as increasing numbers of antibiotic-resistant bacterial strains. Even after short-term AB treatment these consequences can persist for years.Citation11,12
Many studies have investigated the impact of AB treatment on the present microbiota in human fecal samples using mostly 16S rRNA gene approaches,Citation12-16 but mucosa-associated microbiota is highly important to be taken in consideration. Mucosal microbiota is in contact with the gut-associated lymphoid tissue and is thus likely to be more important to the host.Citation4,9 This is supported by the finding of Ott et al.Citation17 showing by Single Strand Conformational Polymorphism analysis that mucosa-associated microbiota differ from fecal communities in healthy individuals. Moreover, it is of importance to take the active microbiota into account, as only they contribute to metabolic turnover and were shown to differ from present microbiota in healthy and diseased people.Citation18,19 To our knowledge, there is currently no study exploring both luminal and mucosal microbiota simultaneously at the abundance and activity level.
The antibiotic paromomycin is active against most Gram-negative and many Gram-positive bacteria due to inhibition of protein biosynthesis. Paromomycin is used for preoperative reduction of intestinal microbiota, treatment of hepatic encephalopathy and treatment of tapeworms and amebiasis. One more reason for the use of paromomycin as antibiotic in this study is that this antibiotic substance is not absorbed in significant amounts to human circulation, but is selectively working in the human gut. The use of this locally acting antibiotic substance helped avoiding any general effects in our population of healthy volunteers.
We hypothesize (i) that composition of luminal and mucosal microbiota is differentially affected by AB treatment and (ii) that there are differences between microbial abundance and activity levels in association with the above-mentioned factors. Differences and changes in the microbial community of lumen and mucosa were assessed by pyrosequencing at the 16S rDNA and rRNA level. With reference to Blazewicz et al. we treat bacterial rRNA level amplicon counts as an indicator for “potential” metabolic activity.Citation20
Results
Sequence and OTU statistics
Preprocessing and quality check removed 19% of the sequences resulting in a final dataset of 404988 sequences (combined DNA and RNA level data). Thereof 59931 sequences were left after random subsampling. Sequences were assigned to a total of 4293 OTUs, with 2217 and 2823 OTUs at the DNA and RNA level, respectively. Relative abundances of bacterial phyla and families are depicted in , respectively.
Large effects due to antibiotic treatment on α diversity at the DNA level
illustrates the distribution of Shannon numbers equivalent 1D. The numbers equivalent of a diversity index (here: Shannon entropy) is the number of equally likely elements (here: OTUs) needed to produce the given value of the diversity index.Citation21 So this measure of α diversity incorporates both OTU richness and evenness. gives the evaluation results of the generalized least squares mixed models fitted to 1D. At the DNA level, factors “Compartment” and “Treatment status” had a fairly large combined effect on 1D, as shown by the marginal adjusted explained variance (R2adj.) of ∼25% of the model. About half of the explained variance in the model could be attributed to the random factor “Subject” (, compare conditional R2adj. of ∼50% to marginal R2adj.). While the “Compartment” effect was only weakly significant with slightly larger values of 1D in mucosa compared to lumen (, PCompartment DNA = 0.040; ), the “Treatment status” effect was substantial (, PTreatment status DNA <0 .001). The latter is due to the conspicuous “drop” in 1D immediately after AB treatment (), while after 46 d α diversity nearly reached the pre-AB treatment level (). In contrast, effects of the factors under study on 1D were not observable at the RNA level (). Notably, an interaction effect “Compartment:Treatmen statust” on 1D was not apparent at either level, thus the effect of AB treatment must be considered the same for lumen and mucosa.
Figure 3. Distribution of Shannon numbers equivalent values at DNA and RNA level depending on factors “Compartment” and “Treatment status.” Colored guiding lines mark the mean values for factor levels of “Compartment” at the respective treatment status: (A) before antibiotic treatment (day 0); (B) immediately after cessation of antibiotic treatment (day 4); (C) 6 weeks after cessation of antibiotic treatment (day 46).
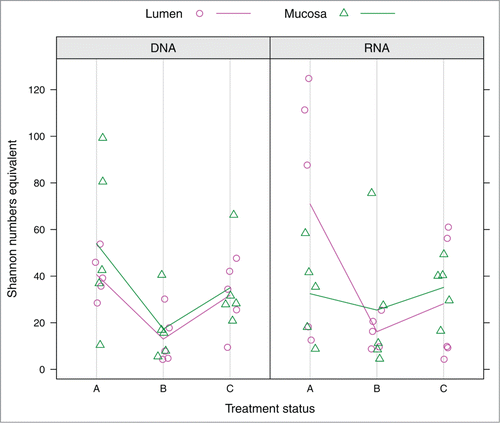
Table 1. Evaluation results of modeling Shannon numbers equivalent 1D by factors “Compartment” and “Treatment status” at the DNA and RNA level. 1D was modeled by generalized least squares mixed models with a random intercept for factor “Patient.” In both cases, a “Treatment status”-dependent variance structure was introduced to compensate for heteroscedasticity. P-values are given for individual factors and for their interaction term. Values below the significance level of 0.05 are marked in bold-face. Marginal and conditional adjusted explained variance (R2adj.) are stated for the final models (i.e., after removal of non-significant effects).
Significant differences due to antibiotic treatment and compartments in β diversity
OTU distribution based on ribosomal gene (DNA) and RNA abundance levels was investigated in separate redundancy analysis (RDA) models. Proportion of variance explained by the overall model (R2adj.) for DNA was about twice as much as for RNA (R2adj. DNA= 12.5%; R2adj. RNA = 6.4%).
Differences between treatment status (A, before antibiotic treatment (day 0); B, immediately after cessation of antibiotic treatment (day 4); C, 6 weeks after cessation of antibiotic treatment (day 46)) and compartments (lumen vs. mucosa) were statistically significant at both the DNA and RNA level (PTreatment status DNA = 10−4; PTreatment status RNA = 2·10−3; PCompartment DNA = 0.02; PCompartment RNA = 0.05). Pairwise comparisons revealed significant differences between treatment status A and B as well as between A and C at both the DNA and RNA level (PAB DNA = 2·10−5; PAC DNA = 6·10−5; PAB RNA = 10−3; PAC RNA = 0.04). Graphical representations of the RDA models are shown in .
Figure 4. Graphical representations (distance plots) of the redundancy analysis (RDA) models of Hellinger-transformed OTU abundances. Models illustrate the relationships between luminal (magenta) and mucosal (green) samples at different treatment status A (dark) through C (light) at the DNA (A–C) and RNA (D–F) level, controlling for variance between subjects. The three-dimensional models are depicted in 2-dimensional space showing the planes spread out by RDA axes 1 and 2 (A, D), 1 and 3 (B, E), and 2 and 3 (C, F), respectively.
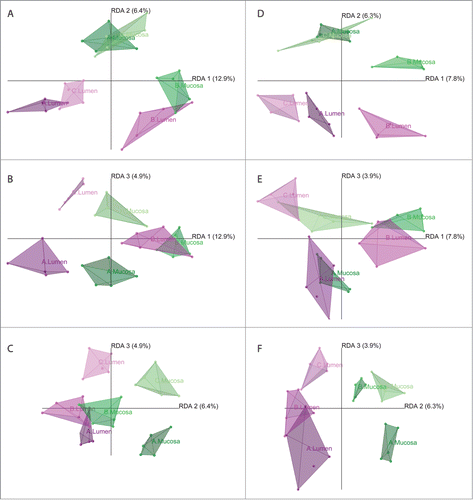
Both the treatment status and the compartment exert a significant effect on the gut microbiota. However, these 2 factors do not interact with each other (P-values for interaction terms: PTreatment status:Compartment DNA = 0.74, PTreatment status:Compartment RNA = 0.45). This indicates that, although the microbiota of lumen and mucosa are inherently different, AB treatment and resilience affected mucosal and luminal microbiota in a similar way. Nevertheless, the gut microbial alterations at DNA and RNA level were different (see below).
Compartment and treatment status-specific indicator OTUs
OTUs listed in are significantly correlated with the axes of the RDA model and thus responsible for differentiation between compartments and treatment status, respectively.
Table 2. Correlated OTUs responsible for differentiation between compartments and/or treatment status with ✓ = OTU was significant at the respective level. rho: Spearman's rank correlation coefficient. Marked in gray: OTUs responsible for differences at the DNA and/or RNA level, but NOT correlated at both levels.
Indicator OTUs at the DNA level for lumen () were affiliated with the genera Clostridium and Coprococcus, whereas indicator OTUs for mucosa were affiliated with Clostridium as well and additionally with Bacteroides, Streptococcus and Ralstonia. Mucosal indicator OTUs at the RNA level were affiliated with Faecalibacterium, Clostridium, Finegoldia, Lachnospiraceae, and Bacteroides, in contrast luminal indicator OTUs have not been detected.
Table 3. Indicator OTUs for the effect of “Compartment” (mucosa vs. lumen) at the DNA and RNA level
At the DNA level indicator OTUs for treatment status A (before antibiotic treatment) compared to treatment status B (immediately after cessation of antibiotic treatment) () were mainly represented by Coprococcus, Eubacterium, Clostridium, Turicibacteriaceae, Blautia, Faecalibacterium, Ruminococcaceae, and Lachnospiraceae. Conversely, indicator species for treatment status B compared to A represented Escherichia and Holdemania. Only a few indicator OTUs affiliated to genera Blautia, Faecalibacterium, and Coprococcus were found for treatment status A at the RNA level. No indicator OTUs were detected for treatment status B. Concerning the comparison of treatment status A and C, indicator OTUs Faecalibacterium and Coprococcus were identified for treatments status A at the DNA level, whereas Blautia and Coprococcus depict indicator OTUs for treatment status A as well but at the RNA level ().
Table 4. Indicator OTUs for the effect “Treatment status” (treatment status A (= before antibiotic treatment) vs. B (=immediately after antibiotic treatment)) at the DNA and RNA level
Table 5. Indicator OTUs for the effect “Treatment status” (treatment status A (= before antibiotic treatment) vs. C (= 6 weeks after cessation of antibiotic treatment)) at the DNA and RNA level
Abundance does not necessarily correspond to potential activity
The majority of OTUs significantly correlating with RDA axes showed matching (i.e., correlated) abundance and potential activity patterns (). The relative proportions of these correlated OTUs are depicted in Figures S1 and S2 at DNA and RNA level, respectively. However, 6 OTUs did not exhibit such a correlation between both levels (; highlighted in gray in ; ) This contrasting pattern was shown for OTUs 3137, 1660, 1708, 2646, 3142, 3744 (C. hiranonis, Eubacterium, Ruminococcus torques, Blautia, Clostridium and Holdemania).
Figure 5. Relative abundances in sample groups of significant OTUs lacking correlation between DNA and RNA levels. Colored guiding lines mark the mean values for factor levels of “Compartment” at the respective treatment status: (A) before antibiotic treatment (day 0); (B) immediately after cessation of antibiotic treatment (day 4); (C) 6 weeks after cessation of antibiotic treatment (day 46).
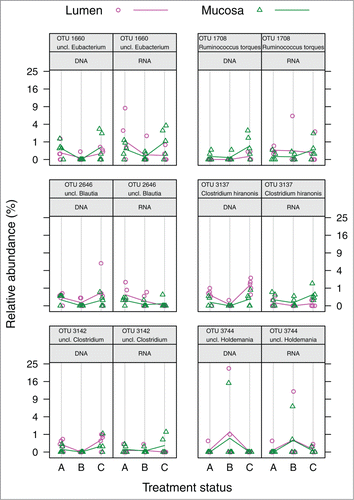
Table 6. OTUs responsible for differences at the DNA and/or RNA level, but NOT correlated at both levels. ✓ indicates significant OTU in the RDA model of the respective level. rho: Spearman's rank correlation coefficient.
Discussion
Antibiotic treatment may cause severe intestinal adverse events, such as pseudomembranous colitis, that are hypothesized to be provoked by the antibiotic-induced dysbalance in the gut microbiota.Citation11-15 Yet these side effects only affect a minority of patients, whereas in most treated individuals the intestinal microbiota appears to recover to a stable balance. While few studies have investigated this phenomenon at the level of fecal microbial communities, we are presenting the first systemic study that simultaneously elucidates the impact of AB treatment and subsequent recovery on both mucosa-associated and luminal microbiota of the human large intestine. Bacteria with high transcriptional activity might have a more important pathophysiological role than inactive bacteria.Citation22-24 We thus studied effects on the bacterial community at both the gene level (with relative 16S rRNA gene amplicon counts indicating abundance) and the transcript level (with relative 16S cDNA amplicon counts indicating general potential metabolic activity sensu Blazewicz et al.Citation20
AB treatment similarly affects luminal and mucosal microbiota
Both α diversity () and β diversity RDA plots () indicate statistically significant differences between mucosal and luminal microbiota as well as a significant influence of AB treatment on gut microbiota at the DNA and (in β diversity) at the RNA level. AB treatment affected gut microbiota of lumen and mucosa in a similar way, although paromomycin is known to be poorly absorbable after oral administration, with almost 100% of the drug recoverable in the stool.
Biopsies provide important additional information alongside fecal samples
The present study revealed important differences between the luminal and mucosa-associated microbiota. OTU 2526 (Bacteroides dorei) is an indicator OTU for mucosa at both the DNA and RNA level. Active mucosa-associated B. dorei prevailed in patients with ulcerative colitis (UC) compared to healthy individuals and has been associated with active celiac disease as well as collagenous colitis.Citation18,25,26 OTU 3137 (Clostridium hiranonis) is an indicator for luminal microbiota at the DNA level and for mucosa at the RNA level (further discussed in the last section). C. hiranonis strains dehydroxylate primary bile acids to form secondary bile acids, which are important for an adequate lipid metabolism.Citation27-30 Increased levels of secondary bile acids have been associated with an increased risk of colon cancer.Citation31,32 OTU 3026, another indicator for mucosa at the RNA level, is affiliated to Faecalibacterium prausnitzii, a species with reported anti-inflammatory capacity.Citation33 Interestingly, indicator OTUs at RNA level were always associated with the mucosal microbiota, whereas the DNA level exhibited indicator OTUs for both, mucosa and lumen. Therefore, a study considering only luminal samples is likely to overlook possible detrimental as well as anti-inflammatory effects on the mucosa exerted by these OTUs. Future studies on gastrointestinal infectious diseases should thus include biopsies to ensure detection of mucosa-associated indicator OTUs, namely at the RNA level.
AB therapy leads to a reduction of beneficial OTUs
The three-day lasting antibiotic treatment resulted in a general decrease of bacterial diversity at the DNA level () and the disappearance of several indicator taxa at the DNA and RNA level. Indicator OTUs at the RNA level were a complete subset of the indicator OTUs at the DNA level (). As the AB treatment affected luminal and mucosal microbiota in a similar way, mucosa and lumen bear the same indicator OTUs at the DNA and RNA level, respectively. Luminal and mucosal indicator OTUs at the DNA and RNA level were affiliated to Blautia, Lachnospiraceae (including Coprococcus), Ruminococcaceae and F. prausnitzii, and decreased due to AB treatment. This was reported before for the present microbiota.Citation14,34 The relative decrease of present and active F. prausnitzii (mean decrease from 2.42% to 0.46% at the DNA level and from 1.00% to 0.10% at the RNA level) as a consequence of AB usage is particularly crucial: apart from the above-mentioned anti-inflammatory capacity,Citation33 this species is known to be less abundant in individuals suffering from inflammatory bowel diseases like Crohn's disease (CD) or UC.Citation35-37 A statistically significant association of prior AB usage and CD was reported as well.Citation38 Taking this into account, we argue that AB-induced reduction of intestinal species such as F. prausnitzii could support the onset of intestinal disorders, possibly fostering CD or UC.
OTU 3137, a minor constituent of both present and potentially active gut microbiota (Figures S1 and S2), is affiliated to Clostridium hiranonis. This species belongs to Clostridium cluster XI which also includes C. difficile.Citation39 The latter is known to increase in relative abundance during broad-spectrum AB therapy, sometimes leading to AB-associated diarrhea and pseudomembranous colitis.Citation10,40,41 In this study, C. hiranonis decreased in relative abundance due to AB treatment (mean decrease from 0.29% to 0.01% at the DNA level). This could lead to a diminished role of C. hiranonis in the bile acid metabolism, possibly resulting in a disturbed lipid metabolism. A recent multi-omic study already showed an attenuated capacity of the host's microbiota to transport and metabolize bile acid and cholesterol due to AB therapy.Citation42
Few indicator OTUs fail to recover within 42 d after AB cessation
Luminal and mucosal indicator OTUs affiliated to F. prausnitzii and Coprococcus at the DNA level and to Blautia and Coprococcus at the RNA level failed to recover sufficiently, as each of them was designated an indicator OTU for treatment status A (pre-AB treatment). At the DNA level, OTUs 3053 (F. prausnitzii) and 2616 (Blautia) were indicators for pre-AB treatment when compared to treatment status B (immediately after cessation of AB treatment), but only OTU 3053 was an indicator for treatment status A when compared to treatment status C (6 weeks after cessation of AB treatment). This points to an accelerated recovery of Blautia compared to F. prausnitzii at the DNA level as shown by Young and Schmidt.Citation34 A different response in recovery of several other intestinal bacterial species after AB treatment was also observed in a long-term study by Jernberg et al.Citation15 Similarly to F. prausnitzii (see above), Coprococcus and Blautia seem to be gut-protective as reduced abundances of Coprococcus were described to be associated with colorectal adenomas and irritable bowel syndrome, respectively. Moreover, Blautia showed a reduction in mucosa-adherent samples of patients suffering from colorectal cancer and hepatic encephalopathy.Citation43-46
OTU abundance and potential activity do not necessarily conform to each other
Abundance and potential activity are well correlated in most significant OTUs (). However, this was not the case for some OTUs representing Eubacterium, Ruminococcus torques, Blautia, C. hiranonis, Clostridium spp. and Holdemania (). Interestingly, C. hiranonis showed significantly higher potential activity in mucosa-associated compared to luminal samples, while just the opposite was the case for its abundance. C. hiranonis constitutes an important factor in the host's metabolic status (see above). Whether a discrepancy between abundance and activity of an OTU contributes to metabolic patterns or represents clinical disease markers is still unknown and needs to be investigated in further studies. As the present study was conducted on healthy individuals, it would be interesting to investigate patients to unravel disease-specific patterns possibly underlying OTU abundance-activity discrepancies.
Conclusion and Outlook
This study revealed that AB treatment can lead to significant alterations of present and potentially active microbiota. Luminal and mucosa-associated microbiota are affected in a similar way. We identified compartment-specific as well as AB-influenced taxa, such as Blautia, B. dorei, F. prausnitzii, and C. hiranonis. At the RNA level only mucosal indicator OTUs were identified. During and after cessation of AB treatment some OTUs behaved inconsistently with respect to their abundance and potential activity patterns. Therefore, future gut microbial studies should consider present and active members of both the luminal and mucosal microbiota.
Patients and Methods
Study participants and study design
Fecal samples and biopsies were collected from 5 healthy volunteers (all women, aged 20–22 years). Criteria for participation in the study were: no antibiotic, antimycotic or probiotic therapy and no hospitalization or diarrhea in the previous 6 months; no current infection; no relative or absolute contraindications against paromomycin. All individuals gave their written consent as approved by the local ethical committee of the Medical Faculty of the Christian-Albrechts-University (CAU) of Kiel, Germany. The antibiotic paromomycin (Pfizer, Berlin, Germany) was administered at a dose of 4 g·d−1 over a period of 3 d. A series of fecal samples and sigmoid biopsies were obtained before (A = day 0) and immediately after cessation of AB treatment (B = day 4) as well as 6 weeks after cessation (C = day 46). Prior to sigmoidoscopy a rectal lavage was administered to increase the safety of examination (Yal™ rectal infusion, containing 13.4 g sorbitol in a total volume of 67.5 ml, Trommsdorf, Alsdorf, Germany). Biopsies and fecal samples were stored at −80°C until further use.
DNA and RNA extraction, PCR amplification of V1 to V2
Simultaneous extraction of DNA and RNA from biopsies was performed with the AllPrep DNA/RNA Mini Kit (Qiagen, Hilden, Germany) with an additional bead-beating step after addition of RLT buffer to enhance cell lysis. RNA extraction included an additional on-column DNase digestion using a DNase solution/RDD buffer mix. Extracted RNA was reverse-transcribed into cDNA with the High Capacity cDNA Reverse Transcription Kit (Applied Biosystems, Foster City, USA) according to the manufacturer's instructions. Total DNA from fecal samples was extracted using FastDNA™SPIN KIT FOR SOIL (Qiagen, Hilden, Germany), with a preceding incubation in 200 µl TL-Buffer and 25 µl proteinase K for 2 hours at 56°C. Extraction of RNA from stool was performed using PowerMicrobiome™ RNA Isolation Kit (MO BIO Laboratories Inc., Carlsbad, CA) according to the manufacturer's protocol. Variable regions V1 and V2 of the 16S rRNA gene and 16S cDNA were amplified and sequenced with 454 pyrosequencing as previously described.Citation47
Data processing
Data were preprocessed using MOTHUR version 1.26.0 as previously reported.Citation48,49
General information on statistical analysis
All downstream computations were performed in R v2.15.2.Citation50 Unless stated otherwise, analyses were separately performed with DNA and RNA level data. P-values in multiple testing scenarios were subject to Benjamini-Hochberg correction (“Q-values”).Citation51 For convenience, treatment status levels are coded A (before AB treatment), B (immediately after cessation of AB treatment), and C (after 42-day recovery phase).
Alpha diversity and taxonomic plots
Alpha diversity was described by effective OTU richness 1D (aka. Shannon numbers equivalent), which takes both the number and relative abundance of OTUs into account.Citation21 1D was fitted to the experimental factors “Treatment status” (with treatment status A, B and C as levels) and “Compartment” (with lumen and mucosa as levels). As framework for statistical fitting we used generalized least squares mixed models with a random intercept for factor “Subject” implemented in function lme of the R package nlme v3.1–117.Citation52 Models were compared by means of the Akaike Information Criterion (AIC) and validated following the recommendations of Zuur et al.Citation53 Introduction of a “Treatment status”-dependent variance structure compensated for heteroscedasticity. Significance of individual factors and their interaction term was determined by likelihood-ratio tests. Marginal and conditional adjusted explained variance (R2adj.) were calculated for the final models with the R package lmmfit v1.0.Citation54 Marginal R2adj. describes the proportion of variance explained by the fixed factors alone, while conditional R2adj. describes the proportion of variance explained by both fixed and random factors. R2 values were adjusted for the number of fixed effects and the number of correlation structure parameters.
For an initial overview of the distribution of bacterial taxa across samples, OTU counts were added up at the phylum and family level, respectively. The family Erysipelotrichaceae was moved from the phylum Tenericutes into the phylum Firmicutes to reflect recent taxonomic changes.Citation55
Data transformation and statistical model evaluation in β diversity
Multivariate statistics was conducted via redundancy analysis (RDA).Citation56 as implemented in the R package vegan v2.1–20.Citation57 OTU count data were Hellinger-transformed in order to produce valid results in RDA.Citation58 The design of the present study corresponds to a multivariate mixed-effects model in which the OTU count data are explained by 2 fixed effects (denoted as “Treatment status” and “Compartment”) nested within the random subject effect. Homogeneity of variances for the 2 fixed effects was assessed using function betadisper. The appropriate statistical model was determined via stepwise selection by function ordistep. Significance of individual effects within the selected model was determined by 104 restricted permutations. Variance partitioning was done with varpart. 3D visualizations of the RDA models were produced in kinemage format.Citation59 using the R package R2Kinemage developed by SCN and displayed in KiNG v2.21.Citation60
Pairwise post-hoc tests in β diversity
Individual post-hoc tests were performed on the treatment status combinations A-B and A-C with appropriate subsets of the OTU count data. To control for the random effect between study participants, we first conducted a regression on factor “Subject.” Residuals of this regression were then subject to a permutational ANOVA (function adonis) with 105 permutations, using the levels of factor “Compartment” as strata.
Determination of significant OTUs
OTUs significantly correlated with an axis in the RDA model were determined using function envfit with 105 permutations. In order to reduce the number of tests in this procedure, OTUs were pre-filtered according to their vector lengths calculated from corresponding RDA scores (scaling 1) by profile likelihood selection.Citation61
Indicator analysis
Significant OTUs were further subject to indicator analysis with the R package indicspecies v1.6.5.Citation62 Indicator OTUs (in analogy to indicator species).Citation62 are OTUs that prevail in a certain sample type while found only infrequently and irregularly in a comparison sample type. Each analysis was preceded by a regression on factor “Subject” to avoid confounding inter-individual effects. Residuals were subject to a second regression on the respective other fixed effect. Residuals of this second regression were transformed to positive values by subtraction of their minimum and used as input for indicator analysis (function multipatt) with 105 permutations.
Between-level OTU correlations
Relationships between DNA and RNA level counts of OTUs were investigated by Spearman rank correlation. Any OTU determined “significant” as outlined above at either the DNA or RNA level was included.
Disclosure of Potential Conflicts of Interest
No potential conflicts of interest were disclosed
Author Contribution
The authors made the following contributions to the study. Conceived and designed experiments: F-AH, HK, PCR, StS, AKF, and SJO. Performed experiments: F-AH, HK, TK, AKF, and SJO. Analyzed data: HK, F-AH, and SCN. Contributed analysis tools: SCN, CK, PCR, and StS. Wrote paper: F-AH, HK, SCN, RAS, and SJO. F-AH HK, and SCN contributed equally. AKF, SJO shared senior authorship.
Ethics Approval
Ethical Board of the Medical Faculty of the Christian-Albrechts-University, Kiel, Germany. Patient consent obtained.
Supplemental_Material.zip
Download Zip (64.3 KB)Acknowledgments
We thank Anita Hellmann, Manuela Kramp, Sanaz Sedghpour Sabet, and Katja Cloppenborg-Schmidt for technical assistance and Markus B Schilhabel as well as Sven Künzel for their expertise in 454 sequencing.
Supplemental Material
Supplemental data for this article can be accessed on the publisher's website
References
- Hooper LV, Gordon JI. Commensal host-bacterial relationships in the gut. Science 2001; 292:1115-8; PMID:11352068
- Whitman WB, Coleman DC, Wiebe WJ. Prokaryotes: the unseen majority. Proc Natl Acad Sci U S A 1998; 95:6578-83; PMID:9618454
- Bäckhed F, Ley RE, Sonnenburg JL, Peterson DA, Gordon JI. Host-bacterial mutualism in the human intestine Science 2005; 307:1915-20; PMID:15790844
- Eckburg PB, Bik EM, Bernstein CN, Purdom E, Dethlefsen L, Sargent M, Gill SR, Nelson KE, Relman DA. Diversity of the human intestinal microbial flora Science 2005; 308:1635-8; PMID:15831718
- Neish AS. Microbes in gastrointestinal health and disease. Gastroenterology 2009; 136:65-80; PMID:19026645
- Costello EK, Lauber CL, Hamady M, Fierer N, Gordon JI, Knight R. Bacterial community variation in human body habitats across space and time. Science 2009; 326:1694-7; PMID:19892944
- Franks AH, Harmsen HJ, Raangs GC, Jansen GJ, Schut F, Welling GW. Variations of bacterial populations in human feces measured by fluorescent in situ hybridization with group-specific 16S rRNA-targeted oligonucleotide probes. Appl Environ Microbiol. 1998; 64:3336-45; PMID:9726880
- Turnbaugh PJ, Hamady M, Yatsunenko T, Cantarel BL, Duncan A, Ley RE, Sogin ML, Jones WJ, Roe BA, Affourtit JP, et al. A core gut microbiome in obese and lean twins. Nature 2009; 457:480-4; PMID:19043404
- Zoetendal EG, von Wright A, Vilpponen-Salmela T, Ben-Amor K, Akkermans AD, de Vos WM. Mucosa-associated bacteria in the human gastrointestinal tract are uniformly distributed along the colon and differ from the community recovered from feces. Appl Environ Microbiol 2002; 68:3401-7; PMID:12089021
- Sullivan A, Endlund C, Nord CE. Effect of antimicrobial agents on the ecological balance of human microflora. Lancet Infect Dis 2001; 1:101-14; PMID:11871461
- Jakobsson HE, Jernberg C, Andersson AF, Sjölund-Karlsson M, Jansson JK, Engstrand L. Short-term antibiotic treatment has differing long-term impacts on the human throat and gut microbiome. PLoS One 2010; 5:e9836; PMID:20352091
- Löfmark S, Jernberg C, Jansson JK, Edlund C. Clindamycin-induced enrichment and long-term persistence of resistant Bacteroides spp. and resistance genes. J Antimicrob Chemother 2006; 58:1160-7; PMID:17046967
- Dethlefsen L, Relman DA. Incomplete recovery and individualized responses of the human distal gut microbiota to repeated antibiotic perturbation. Proc Natl Sci U S A 2011; 15:4554-61; PMID:20847294
- Dethlefsen L, Huse S, Sogin ML, Relman DA. The pervasive effects of an antibiotic on the human gut microbiota, as revealed by deep 16S rRNA sequencing. PLoS Biol. 2008; 6:e280; PMID:19018661
- Jernberg C, Löfmark S, Edlund C, Jansson JK. Long-term ecological impacts of antibiotic administration on the human intestinal microbiota. ISME J. 2007; 1:56-66; PMID:18043614
- Robinson CJ, Young VB. Antibiotic administration alters the community structure of the gastrointestinal micobiota. Gut Microbes 2010; 1:279-84; PMID:20953272; http://dx.doi.org/10.4161/gmic.1.4.12614
- Ott SJ, Musfeldt M, Timmis KN, Hampe J, Wenderoth DF, Schreiber S. In vitro alterations of intestinal bacterial microbiota in fecal samples during storage. Diagn Microbiol Infect Dis 2004; 50:237-45; PMID:15582296; http://dx.doi.org/10.1016/j.diagmicrobio.2004.08.012
- Rehman A, Lepage P, Nolte A, Hellmig S, Schreiber S, Ott SJ, Transcriptional activity of the dominant gut mucosal microbiota in chronic inflammatory bowel disease patients. J Med Microbiol 2010; 59:1114-22; PMID:20522625; http://dx.doi.org/10.1099/jmm.0.021170-0
- Peris-Bondia F, Latorre A, Artacho A, Moya A, D'Auria G. The active human gut microbiota differs from the total microbiota. PLoS One 2011; 6:e22448; PMID:21829462; http://dx.doi.org/10.1371/journal.pone.0022448
- Blazewicz SJ, Barnard RL, Daly RA, Firestone MK. Evaluating rRNA as an indicator of microbial activity in environmental communities: limitations and uses. ISME J. 2013; 7:2061-8; PMID:23823491; http://dx.doi.org/10.1038/ismej.2013.102
- Jost L. Partitioning diversity into independent alpha and beta components. Ecology 2007; 88:2427-39; PMID:18027744; http://dx.doi.org/10.1890/06-1736.1
- Ott SJ, Plamondon S, Hart A, Begun A, Rehman A, Kamm MA, Schreiber S. Dynamics of the mucosa-associated flora in ulcerative colitis patients during remission and clinical relapse. J Clin Microbiol 2008; 46:3510-3; PMID:18701655; http://dx.doi.org/10.1128/JCM.01512-08
- Ott SJ, Musfeldt M, Wenderoth DF, Hampe J, Brant O, Fölsch UR, Timmis KN, Schreiber S. Reduction in diversity of the colonic mucosa associated bacterial microflora in patients with active inflammatory bowel disease. Gut 2004; 53:685-93; PMID:15082587; http://dx.doi.org/10.1136/gut.2003.025403
- Sokol H, Lepage P, Seksik P, Doré J, Marteau P. Temperature gradient gel electrophoresis of fecal 16S rRNA reveals active Escherichia coli in the microbiota of patients with ulcerative colitis. J Clin Microbiol 2006; 44:3172-7; PMID:16954244; http://dx.doi.org/10.1128/JCM.02600-05
- Sánchez E, Donat E, Ribes-Koninckx, C, Calabuig M, Sanz Y. Intestinal Bacteroides species associated with coeliac disease. J Clin Pathol 2010; 63:1105-11; PMID:20972239; http://dx.doi.org/10.1136/jcp.2010.076950
- Gustafsson RJ, Ohlsson B, Benoni C, Jeppsson B, Olsson C. Mucosa-associated bacteria in two middle-aged women diagnosed with collagenous colitis. World J Gastroenterol 2012; 18:1628-34; PMID:22529692; http://dx.doi.org/10.3748/wjg.v18.i14.1628
- Ridlon JM, Kang DJ, Hylemon PB. Bile salt biotransformations by human intestinal bacteria. J Lipid Res 2006; 47:241-59; PMID:16299351; http://dx.doi.org/10.1194/jlr.R500013-JLR200
- Wells JE, Hylemon PB. Identification and characterization of a bile acid 7 α-dehydroxylation operon in Clostridium sp. strain TO-931, a highly active 7 α-dehydroxylating strain isolated from human feces. Appl Environ Microbiol. 2000; 66:1107-13; PMID:10698778; http://dx.doi.org/10.1128/AEM.66.3.1107-1113.2000
- Archer IM, Harper PS, Wusteman FS. Multiple forms of iduronate 2-sulphate sulphatase in human tissues and body fluids. Biochim Biophys Acta 1982; 708:134-40; PMID:6816283; http://dx.doi.org/10.1016/0167-4838(82)90213-8
- Hirano S, Masuda N, Oda H, Imamura T. Transformation of bile acids by mixed microbial cultures from human feces and bile acid transforming activities of isolated bacterial strains. Microbiol Immunol 1981; 25:271-82; PMID:7253965; http://dx.doi.org/10.1111/j.1348-0421.1981.tb00029.x
- Baptissart M, Vega A, Maqdasy S, Caira F, Baron S, Lobaccaro JM, Volle DH. Bile acids: From digestion to cancers. Biochimie 2012; 95(3):504-17; PMID:22766017.; http://dx.doi.org/10.1016/j.biochi.2012.06.022
- Degirolamo C, Modica S, Palasciano G, Moschetta A. Bile acids and colon cancer: Solving the puzzle with nuclear receptors. Trends Mol Med 2011; 17:564-72; PMID:21724466; http://dx.doi.org/10.1016/j.molmed.2011.05.010
- Sokol H, Seksik P, Furet JP, Firmesse O, Nion-Larmurier I, Beaugerie L, Cosnes J, Corthier G, Marteau P, Doré J. Low counts of Faecalibacterium prausnitzii in colitis microbiota. Inflamm Bowel Dis. 2009; 15:1183-9; PMID:19235886; http://dx.doi.org/10.1002/ibd.20903
- Young VB, Schmidt TM. Antibiotic-associated diarrhea accompanied by large-scale alterations in the composition of the fecal microbiota. J Clin Microbiol 2004; 42:1203-6; PMID:15004076; http://dx.doi.org/10.1128/JCM.42.3.1203-1206.2004
- Fujimoto T, Imaeda H, Takahashi K, Kasumi E, Bamba S, Fujiyama Y, Andoh A. Decreased abundance of Faecalibacterium prausnitzii in the gut microbiota of Crohn's disease. J Gastroenterol Hepatol 2012; 28(4):613-9; PMID:23216550.
- Duboc H, Rajca S, Humbert L, Farabos D, Maubert M, Grondin V, Jouet P, Bouhassira D, Seksik P, Sokol H, et al. Increase in fecal primary bile acids and dysbiosis in patients with diarrhea-predominant irritable bowel syndrome. Neurogastroenterol Motil. 2012; 24:513-20; PMID:22356587; http://dx.doi.org/10.1111/j.1365-2982.2012.01893.x
- Jia W, Whitehead RN, Griffiths L, Dawson C, Waring RH, Ramsden DB, Hunter JO, Cole JA. Is the abundance of Faecalibacterium prausnitzii relevant to Crohn's disease? FEMS Microbiol Lett 2010; 310:138-44; PMID:20695899; http://dx.doi.org/10.1111/j.1574-6968.2010.02057.x
- Card T. Antibiotic use and the development of Crohn's disease. Gut 2004; 53:246-50; PMID:14724158; http://dx.doi.org/10.1136/gut.2003.025239
- Kitahara M, Takamine F, Imamura T, Benno Y. Clostridium hiranonis sp. nov., a human intestinal bacterium with bile acid 7α-dehydroxylating activity. Int J Syst Evol Microbiol 2001; 51:39-44; PMID:11211270
- Navaneethan U, Venkatesh PG, Shen B. Clostridium difficile infection and inflammatory bowel disease: understanding the evolving relationship. World J Gastroenterol 2010; 16:4892-904; PMID:20954275; http://dx.doi.org/10.3748/wjg.v16.i39.4892
- Dawson LF, Valiente E, Wren BW. Clostridium difficile–a continually evolving and problematic pathogen. Infect Genet Evol 2009; 9:1410-7; PMID:19539054; http://dx.doi.org/10.1016/j.meegid.2009.06.005
- Pérez-Cobas AE, Gosalbes MJ, Friedrich A, Knecht H, Artacho A, Eismann K, Otto W, Rojo D, Bargiela R, von Bergen M, et al. Gut microbiota disturbance during antibiotic therapy: a multi-omic approach. Gut 2013; 62:1591-601; PMID:23236009; http://dx.doi.org/10.1136/gutjnl-2012-303184
- Bajaj JS, Hylemon PB, Ridlon JM, Heuman DM, Daita K, White MB, Monteith P, Noble NA, Sikaroodi M, Gillevet PM. Colonic mucosal microbiome differs from stool microbiome in cirrhosis and hepatic encephalopathy and is linked to cognition and inflammation. Am J Physiol Gastrointest Liver Physiol 2012; 303:G675-85; PMID:22821944; http://dx.doi.org/10.1152/ajpgi.00152.2012
- Chen W, Liu F, Ling Z, Tong X, Xiang C. Human intestinal lumen and mucosa-associated microbiota in patients with colorectal cancer. PLoS One 2012; 7:e39743; PMID:22761885; http://dx.doi.org/10.1371/journal.pone.0039743
- Kassinen A, Krogius-Kurikka L, Mäkivuokko H, Rinttilä T, Paulin L, Corander J, Malinen E, Apajalahti J, Palva A. The fecal microbiota of irritable bowel syndrome patients differs significantly from that of healthy subjects. Gastroenterology 2007; 133:24-33; PMID:17631127; http://dx.doi.org/10.1053/j.gastro.2007.04.005
- Shen XJ, Rawls JF, Randall T, Burcal L, Mpande CN, Jenkins N, Jovov B, Abdo Z, Sandler RS, Keku TO. Molecular characterization of mucosal adherent bacteria and associations with colorectal adenomas. Gut Microbes 2010; 1:138-47; PMID:20740058; http://dx.doi.org/10.4161/gmic.1.3.12360
- Rehman A, Sina C, Gavrilova O, Häsler R, Ott S, Baines JF, Schreiber S, Rosenstiel P. Nod2 is essential for temporal development of intestinal microbial communities. Gut 2011; 60:1354-62; PMID:21421666; http://dx.doi.org/10.1136/gut.2010.216259
- Schloss PD, Westcott SL, Ryabin T, Hall JR, Hartmann M, Hollister EB, Lesniewski RA, Oakley BB, Parks DH, Robinson CJ, et al. Introducing mothur: open-source, platform-independent, community-supported software for describing and comparing microbial communities. Appl Environ Microbiol 2009; 75:7537-41; PMID:19801464; http://dx.doi.org/10.1128/AEM.01541-09
- Stratil SB, Neulinger SC, Knecht H, Friedrichs AK, Wahl M. Temperature-driven shifts in the epibiotic bacterial community composition of the brown macroalga Fucus vesiculosus. Microbiologyopen n/a–n/a 2013; 2(2):338-49; PMID:23568841; http://dx.doi.org/10.1002/mbo3.79
- R core team. R: A language and environment for statistical computing. http://www.r-project.org/ 2012
- Benjamini Y, Hochberg Y. Controlling the false discovery rate: a practical and powerful approach to multiple testing. J R Stat Soc Ser B Stat Methodol 1995; 57:289-300
- Pinheiro JC, Bates DM, DebRoy S, Sarkar D, R Core Team. nlme: Linear and nonlinear mixed effects models 2014
- Zuur AF, Ieno EN, Walker NJ, Saveliev AA, Smith GM. Mixed effects models and extensions in ecology with R. Stat. Biol. Heal. Springer 2009
- Maj A. lmmfit: Goodness-of-fit-measures for linear mixed models with one-level-grouping. http://cran.r-project.org/package=lmmfit 2011
- Ludwig W, Schleifer KH, Whitman WB in Bergey's Man. Syst. Bacteriol 2009; 1-13 Springer
- Borcard D, Legendre P, Gillet F. Numerical ecology with R. 2011
- Oksanen J, Kindt R, Blanchet FG, Legendre P, Minchin PR, O'Hara RB, Simpson GL, Solymos P, Stevens MHH, Wagner H. vegan: Community ecology package. R package version 2.1-20; http://CRAN.R-project.org/package=vegan 2012
- Legendre P, Gallagher E. Ecologically meaningful transformations for ordination of species data. Oecologia 2001; 129:271-80; http://dx.doi.org/10.1007/s004420100716
- Richardson DC, Richardson JS. The kinemage: A tool for scientific communication. Protein Sci 1992; 1:3-9; PMID:1304880; http://dx.doi.org/10.1002/pro.5560010102
- Chen VB, Davis IW, Richardson DC. KING (Kinemage, Next Generation): a versatile interactive molecular and scientific visualization program. Protein Sci 2009; 18:2403-9; PMID:19768809; http://dx.doi.org/10.1002/pro.250
- Zhu M, Ghodsi Ali. Automatic dimensionality selection from the scree plot via the use of profile likelihood. Comput. Stat. Data Anal 2006; 51:918-30; ; http://dx.doi.org/10.1016/j.csda.2005.09.010
- De Cáceres M, Legendre P. Associations between species and groups of sites: indices and statistical inference. Ecology 2009; 90:3566-74; PMID:20120823; http://dx.doi.org/10.1890/08-1823.1