ABSTRACT
The gut microbiota contributes to the regulation of glucose metabolism in pregnancy. Abundance of the genus Collinsella is positively correlated with circulating insulin; however, it is unclear what determines Collinsella abundance. This study aims to validate the correlation between Collinsella and insulin and to elucidate if macronutrient intake alters Collinsella abundance and gut microbiota composition.
Gut microbiota profiles were assessed by 16S rRNA sequencing in 57 overweight and 73 obese pregnant women from the SPRING (Study of PRobiotics IN Gestational diabetes) trial at 16 weeks gestation and correlated with metabolic hormone levels and macronutrient intake. Gut microbiota composition in the top and bottom 10% of dietary fiber intake was evaluated through network analysis.
Collinsella abundance correlated positively with circulating insulin (rho = 0.30, p = 0.0006), independent of maternal BMI, but negatively with dietary fiber intake (rho = −0.20, p = 0.025) in this cohort. Low dietary fiber intake was associated with a gut microbiota favoring lactate fermentation while high fiber intake promotes short-chain fatty acid-producing bacteria.
Low dietary fiber may enable overgrowth of Collinsella and alter the overall fermentation pattern in gut microbiota. This suggests that dietary choices during pregnancy can modify the nutritional ecology of the gut microbiota, with potential deleterious effects on the metabolic and inflammatory health of the host.
Trial registration: ANZCTR 12611001208998, registered 23/11/2011
Background
Alterations in the inflammatory and metabolic responses of the host are a hallmark of many digestive and metabolic diseases and are often accompanied by remarkable changes in the composition of the gut microbiota, referred to as dysbiosis.Citation1 Gut microbiota dysbiosis is a robust feature in type 2 diabetes (T2D), even though the roles of specific microorganisms in inducing or preventing insulin resistance are still unclear.Citation2-Citation4 In normal pregnancy, the natural homeostasis of the gut microbiota changes toward a more inflammatory state,Citation5 which may contribute to the increase in insulin resistance. The microbial communities present in pregnancy are affected by pre-pregnancy body mass index (BMI) and could contribute to the increased risk of impaired glucose and insulin tolerance. In this context, we have recently reported a strong association between the pregnant gut microbiota and the maternal metabolic milieu in overweight and obese women,Citation6 emphasizing a connection between the genus Collinsella and circulating insulin.
The genus Collinsella belongs to the family Coriobacteriaceae and the phylum Actinobacteria. Members of the family Coriobacteriaceae are considered as pathobionts and Collinsella is its dominant taxon.Citation7 They can affect metabolism through altering intestinal cholesterol absorption, decreasing glycogenesis in the liver and increasing triglyceride synthesis. Currently the molecular mechanisms by which Collinsella affects host metabolism are unknown; however, outside pregnancy its abundance has been associated with T2D,Citation9 rheumatoid arthritis,Citation10 and cholesterol metabolism.Citation11 In addition, gnotobiotic approaches have shown that administration of Collinsella reduces the expression of tight junction proteins in enterocytes and stimulates gut leakage,Citation10 which are features associated with metabolic endotoxemia.Citation12
Even when taking into account the large inter-individual variation in the gut microbiota in response to diet, there is evidence that Collinsella abundance depends on the dietary intake of the host. For instance, a high-protein weight loss diet significantly reduces Collinsella abundance.Citation13 Moreover, a fiber-rich macrobiotic diet reduces Collinsella abundance and promotes the growth of fermentative bacteria notable for their production of short-chain fatty acids (SCFA) such as acetate and butyrate, which is associated with an improved metabolic response in T2D patients.Citation14 Thus, by manipulating dietary composition, the composition of the gut microbiota may be altered and improve metabolic outcomes. Whether Collinsella abundance in pregnancy is associated with dietary intake is unclear.
The aim of this study was to validate the observed relationship between Collinsella abundance and insulin levels and to elucidate if Collinsella abundance is correlated with macronutrient intake in early pregnancy in overweight and obese pregnant women. Understanding how dietary choices may alter insulin resistance through changes in the gut microbiome could provide new targets for improving metabolism in pregnancy.
Material and methods
Patients and clinical samples
This validation study includes a subset of 53 overweight and 73 obese pregnant women of the SPRING cohort (Study of Probiotics in the Prevention of Gestational Diabetes Mellitus, ANZCTR 12611001208998).Citation15 Selection of the participants in this sub-study was based on the availability of stool sample at baseline (<16 weeks gestation) and normoglycemia in early pregnancy. Participants were classified according to BMI cut-off values of 25.0–30 kg/m2 (overweight) and > 30.0 kg/m2 (obese). Stool samples were self-collected and stored at −80°C within 24 hours of collection until bacterial DNA extraction. At baseline, fasting maternal blood was collected and analyzed for HbA1c, insulin, c-peptide, blood glucose, triglycerides, total cholesterol, LDL, VLDL and HDL. Dietary intake was assessed at baseline using the Cancer Council Victoria's Dietary Questionnaire for Epidemiological Studies (DQESv2) evaluating ‘usual’ dietary intake from the start of pregnancy.Citation16 Detailed macronutrient profiles were calculated and reported as grams per day.
DNA extraction and 16srRNA gene sequencing
Bacterial DNA was extracted from 0.25 grams of thawed maternal feces using the RBB+C method followed by the Qiagen AllPrep DNA extraction kit, as previously described.Citation17 Mechanical lysis was achieved by homogenization (3 min × 30 Hz) using 0.4 grams of sterile zirconia beads (0.1and 0.5 mm diameter). Extracted purified DNA was quantified by the Nanodrop ND 1000 spectrophotometer.
Faecal DNA was evaluated by high-throughput 16S rRNA gene amplicon sequencing using Illumina MiSeq platform at the University of Queensland's Australian Centre for Ecogenomics (ACE). Barcoded primer set 926F (5′- TCG TCG GCA GCG TCA GAT GTG TAT AAG AGA CAG AAA CTY AAA KGA ATT GRC GG–3′) and 1392R (5′–GTC TCG TGG GCT CGG AGA TGT GTA TAA GAG ACA GAC GGG CGG TGW GTR C–3′) was used to target variable regions: V6-V8 of the 16S rRNA gene. Positive (Escherichia. coli JM109) and negative (deionized sterile water) controls were added to each PCR run. PCR products were barcoded using the Nextera XT V2 Index kit Set A and Set B. Amplicons were purified using AMPure XP beads per manufacturer's instructions. Barcoded-DNA was quantified, normalized and pooled prior to sequencing. Forward and reverse sequences were joined, demultiplexed and quality filtered using the QIIME (Quantitative Insights Into Microbial Ecology, v 1.9.1) pipeline.Citation18 The open reference OTU picking method was selected in the Greengenes reference database with a pairwise identity threshold of 97%. OTUs with an overall abundance <0.0001 were removed. OTUs detected in the reagent negative controls were deleted from the resultant OTU table. The OTU table was rarefied to 3000 sequences per sample. Downstream analyses were performed using the rarefied OTU table across different taxonomic levels. The sequences were deposited in the NCBI database (Accession number: Bioproject PRJNA385231; SRA: SRP106249).
Validation of 16S genus Collinsella and family Coriobacteriaceae by qPCR
The 16S rRNA results were technically validated by performing semi-quantitative PCR of gene densities for Collinsella and Coriobacteriaceae with primers based on.Citation19 Targeted Collinsella and Coriobacteriaceae qPCR reactions contained 12.5 μL of SYBR Green polymerase chain reaction 2X master mix (Bio-Rad Laboratories), 0.25 μM of each primer, 1.5 mM MgCl2 and 15 ng of template to a final volume of 25 μL. All assays were performed in duplicate on the iQ5 real-time PCR machine (Bio-Rad Laboratories, Hercules, CA) with the following program: 1 cycle of 94°C for 5 minutes and 35 cycles of 94°C for 45 seconds, 60°C for 30 seconds and 72°C for 50 seconds. Levels of Collinsella and Coriobacteriaceae gene density in all samples were normalized with total bacteria content as an endogenous control evaluated using forward: 5′ GCA GGC CTA ACA CAT GCA AGT C 3′ and reverse: 5′ CTG CTG CCT CCC GTA GGA GT 3′.
Statistical analyses
Data are presented as median with interquartile range (Q1–Q3) since the 16S rRNA sequence data does not conform to the normal distribution. Spearman rank correlation coefficient testing was used to find associations between maternal clinical characteristics, dietary intake and Collinsella abundance. Two-sample comparisons were made using Mann-Whitney U tests. Backward multiple linear regression analysis of factors influencing circulating insulin levels was performed including maternal BMI, Collinsella abundance and dietary fiber intake in the model. A p value < 0.05 was considered statistically significant. Microbiota alpha diversity analysis was determined by the Chao-1 and Shannon indices. Beta-diversity was further analyzed by principal component analysis (PCA) and its significance was tested using the Anosim statistic test. Women were grouped ranked on fiber consumption and only the top and bottom 10% were included in the network analysis. The cut-off of 10% was based on the number of women that consumed the recommended dietary fiber intake for pregnancy of >28 grams/day. Network analysis enables the identification of co-occurring bacteria and mutual exclusive bacteria based on high and low dietary fiber intake. PICRUSt was used to identify the predictive metagenome function for all 130 samples. The Kyoto Encyclopaedia of Genes and Genomes (KEGG) Orthologs classification was used and applied to the 16S rRNA gene data. To identify the predictive biological pathways that were significantly different between the corrected high and low fiber groups, the LEfSe (Linear Discriminant Analysis of Effect Size) algorithm was applied. Taxa abundances are associated with fiber intake using Spearman's rho and Bray-Curtis dissimilarity distance method computed by 1000-fold permutation. Significant associations are reported as FDR < 0.05 and nodes (taxa) are colored based on their association with high or low fiber intake. Microbiome analyses were performed within QIIME and Calypso software tool (http://bioinfo.qimr.edu.au/calypso/) v 7.12.Citation20
Results
Study population
The relationship between genus Collinsella and maternal glycemic status was evaluated in a sub-group of 57 overweight and 73 obese pregnant women at <16 weeks gestation from the SPRING cohort. These women remained normoglycemic throughout pregnancy. Clinical and dietary characteristics are presented in . Obese women were recruited slightly earlier into the study than their overweight counterparts. Obese women had significantly elevated insulin, C-peptide, HbA1C, HDL, VLDL and maternal triglyceride levels but no differences in total cholesterol, LDL cholesterol or fasting glucose levels compared with overweight women (). However, all clinical measurements were within the normal pregnancy range, indicating that this population is healthy despite their increased BMI. In this cohort, maternal BMI was positively correlated with circulating fasting insulin (rho = 0.56, p<0.0001). Macronutrient intake in pregnancy was assessed including recalled dietary intake between start of pregnancy up to 16 weeks gestation and was similar in overweight and obese pregnant women ().
Table 1. Fasting biochemical and dietary characteristics of pregnant women at 16 weeks gestation according to maternal BMI.
Collinsella and maternal glycemic status
The profile of the gut microbiota was assessed by 16S rRNA gene sequencing of fecal samples obtained at 16 weeks gestation. A total of 7,589,739 sequences (median: 59787 (17338–96408)) after quality filtering and assembling paired end reads were retained. The genus Collinsella had a median abundance of 0.87 (0.08–2.45)% of the total bacterial sequences in each woman. Eight different OTUs belonging to genus Collinsella were identified, four matched as Collinsella aerofaciens and one corresponding to Collinsella stercoris with the remaining three OTUs only providing genus but not species information.
In the current data set, metabolic parameters were correlated with Collinsella abundance (). Insulin levels were positively associated with Collinsella in the group overall (rho = 0.36, p < 0.0001) as well as in the overweight and obese pregnant women separately (overweight: rho = 0.33, p = 0.013; obese: rho = 0.42, p = 0.0002, ()), indicating that the relationship was independent of maternal BMI in this cohort. This is supported by the finding that Collinsella abundance is not different between overweight and obese pregnant women (overweight: 0.73 (0.02–2.5)%; obese 1.02 (0.07–2.5)%, p = 0.739) () and by the observation that overweight women with high Collinsella abundance have high insulin levels and obese women with low Collinsella abundance have low insulin levels. C-peptide levels and HOMA-IR were also positively associated with Collinsella; however fasting glucose and Hb1Ac were not associated with Collinsella abundance (). In contrast, there were no significant associations between the maternal total cholesterol, LDL cholesterol or triglycerides and Collinsella abundance. Moreover, 16S Collinsella and Coriobacteriaceae abundances were validated by qPCR, resulting in a significant positive association between 16S sequences and qPCR targeted sequences (Collinsella: rho = 0.63, p < 0.0001; Coriobacteriaceae: rho = 0.50, p < 0.0001). Furthermore, there was a positive correlation between insulin and Collinsella abundance as assessed by qPCR (Supplementary Fig. 1).
Table 2. Metabolic parameters and their correlation with genus Collinsella abundance at 16 weeks gestation.
Impact of major macronutrients on Collinsella abundance and insulin homeostasis
To determine whether intake of specific macronutrients are related to Collinsella abundance, correlation analyses were performed between Collinsella relative abundance and carbohydrate, protein, fiber, total fat, saturated fat, polyunsaturated fat, monounsaturated fat and starch intake. Detailed spearman coefficients and p values are reported in Supplementary Table 1. Of all macronutrient components, only dietary fiber was negatively correlated with Collinsella abundance (rho = −0.20, p = 0.025) (). Grouping women according to fiber consumption below or above the median of 17.4 g fiber/day (IQR 13.7–21.5), women with lower fiber intake (n = 66) had a higher Collinsella abundance than women with higher daily fiber intake (n = 64) (p = 0.026) (). When grouping women according to the mean Australian fiber intake (20 g/day; 2011–2012 Australian Health Survey: Usual Nutrient Intakes, Australian Bureau of Statistics publication 4364.0.55.008) for women between 18 and 50 years of age, women with lower fiber intake (n = 84) still had higher Collinsella abundance (0.011 (0.024–0.026)) than those with higher daily fiber intake (n = 43; 0.004 (0-0.022); p = 0.028). Furthermore, circulating fasting insulin was negatively correlated with dietary fiber intake (rho = −0.20, p = 0.023) (). No significant associations between maternal BMI and macronutrient intake other than dietary fiber were found. To assess whether circulating insulin can be predicted from maternal BMI, Collinsella abundance and dietary fiber intake, multiple linear regression analysis was performed. Of all predictor variables, maternal BMI (standardized coefficient beta: 0.520) and Collinsella abundance (standardized coefficient beta: 0.363) within the gut, together predict more than 80% of the variation in circulating insulin in overweight and obese pregnant women (p<0.0001) (Supplementary Table 2).
Figure 2. Connections between Collinsella relative abundance, circulating insulin and dietary fiber intake. (A) Negative correlation between dietary fiber intake and Collinsella relative abundance. (B) Comparison of genus Collinsella between women with low and high dietary fiber intake. (C) Negative correlation between dietary fiber intake and insulin levels in overweight and obese pregnant women.
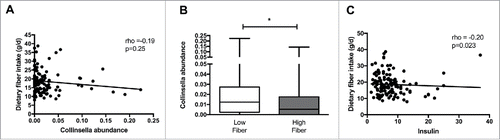
Microbial community shift based on maternal low fiber intake
The inter-individual differences in daily fiber intake were large among the pregnant women in this cohort. To determine whether fiber intake in pregnancy was associated with the presence or absence of groups of bacteria, network analysis was performed. Network and diversity analyses were performed at genus level, including all genera. Network nodes were colored based on high (red) or low (green) fiber consumption with the intensity of the color indicating the strength of the association between the genus and dietary fiber. The participants were ranked on fiber intake and only the top and bottom 10% of dietary fiber intake (30.8 (29.4–35.9) g/day) (n = 13) and (10.3 (9.3–10.5) g/day) (n = 13) respectively) were included in the analysis. Low fiber intake was strongly associated with the genus Collinsella but also with the genera Prevotella, Methanobrevibacter, Dialister and Biophila. In contrast, high fiber intake was associated with higher abundance of the genus Akkermansia followed by the genera Holdemania, Phascolarctobacterium, Odoribacter, Megasphaera, Coprococcus and Butyricimonas (). While fiber ingestion regulates the abundance of certain taxa, both overall beta-diversity (p = 0.651) () and alpha diversity (Shannon index p = 0.705, Chao 1 index p = 0.667) were not significantly different between women with low or high dietary fiber intake indicating that there are no overall differences in the number of bacterial species present. However, macronutrient and energy intake were significantly different between the high and low fiber groups (Supplementary Table 3). To ensure that the differences in bacterial networks were not due to the differences in overall energy intake, fiber consumption was corrected for total energy intake in all women. The women were ranked again and only the top (0.23 (0.21–0.25) g/kJ) and bottom (0.32 (0.29–0.43) g/kJ) 10% were included in the network analysis (Supplementary Table 4). The energy-adjusted network analysis showed additional taxonomic differences (). The gut microbiota composition between high and low fiber consumption was now significantly different (R = 0.117, p = 0.029, ). While low dietary fiber was still associated with Collinsella and Bilophila, the genera: Bifidobacterium, Streptococcus, Lactococcus, Blautia, Turicibacter, Adlercreutzia, Eubacterium and Sutterella also were more abundant in the low fiber group. In contrast, women with higher fiber consumption were still associated with genera Holdemania, Phascolarctobacterium and Coprococcus as well as the genera Bacteroides, Dorea, Faecalibacterium, Roseburia, Acidaminococcus, Lachnospira, Ruminococcus and Desulfovibrio. No differences in alpha diversity were detected in the energy-adjusted fiber analyses of the microbiota (Shannon index p = 0.140, Chao 1 index p = 0.934). The predictive metagenome software PICRUSt was used to examine if a high fiber diet after correction for energy intake was associated with a different functioning of the microbial groups. The KEGG Orthologues revealed that pathways encoding for glutamine, glutamate and pyrimidine metabolism were related to the corrected high fiber group. In contrast, the low fiber group was more associated with the biosynthesis of unsaturated fatty acids, lysine degradation, aminobenzoate degradation and cell motility and secretion ().
Figure 3. Differences in microbiota composition according to high and low fiber intake. (A) Network analysis based on high (n = 13) and low (n = 13) dietary fiber intake. Genera are represented as nodes. Green nodes represent genera overrepresented in the low-fiber group. Red nodes represent genera overrepresented in the high-fiber group. Color intensity explains the strength of the associations with fiber intake. (B) Principal component analysis showing the clustering of selected samples based on high (red) and low (green) fiber intake. (C) Identification of significant associations between low and high dietary fiber groups by Anosim test.
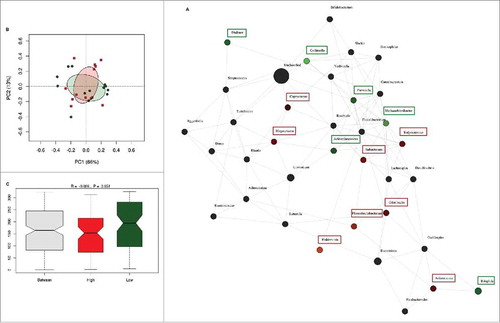
Figure 4. Network analysis after correction of dietary fiber by total energy intake. (A) Network analysis based on high (n = 13) and low (n = 13) dietary fiber intake. Genera are represented as nodes. Green nodes represent genera overrepresented in the low-fiber group. Red nodes represent genera overrepresented in the high-fiber group. Color intensity explains the strength of the associations with fiber intake. (B) Principal component analysis showing the clustering of selected samples based on high (red) and low (green) fiber intake. (C) Identification of significant associations between low and high dietary fiber groups by Anosim test.
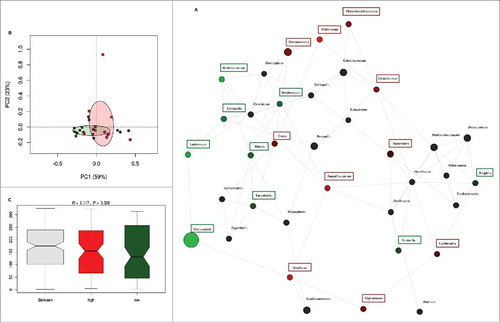
Discussion
Maternal insulin resistance results in a greater risk of developing gestational diabetes and future T2D later in life. The current validation cohort confirms the positive association between genus Collinsella and circulating insulin at early gestation.Citation6 This correlation is independent of maternal BMI in this cohort of overweight and obese pregnant women even though BMI itself is a major determinant of circulating fasting insulin levels. This indicates that high Collinsella abundance may contribute to the progression of insulin resistance during pregnancy. In addition, the results of the present study indicate that gestational fiber intake is associated to Collinsella abundance. This could have implications for dietary advice specific to increasing fiber intake, which may increase the abundance of advantageous commensals such as SCFA producers.
Dietary fiber is an essential substrate for commensal bacteria for the production of SCFAs and can directly affect the gut microbiota.Citation21 It increases fecal bulking and binds to bile acids in the gut, contributing to decreased cholesterol absorption.Citation22,Citation23 In addition, dietary fiber fermentation has the ability to decrease postprandial blood glucose and insulin responses.Citation22 Low fiber ingestion enables growth of microbes capable of using host-secreted mucus glycoproteins and other “non-fiber” energy sources for growth with potential effects on the colonic mucus barrier as well as pathogen overgrowth and proliferation.Citation24 Alterations in taxa may also change the entire gut microbiota.Citation25 The genus Collinsella is often described as a strictly anaerobic pathobiont producing lactate (instead of butyrate or other SCFA). Its abundance is increased in T2D patients when compared to healthy control subjects,Citation9,Citation26 although a recent study in young, lean South-American individuals indicated that it may be ubiquitously present even in people with normal BMI.Citation27 Non-butyrate-producing lactate-utilizing bacteria inhibit intestinal mucin synthesis, which has been associated with pancreatic β cell autoimmunity and type 1 diabetes.Citation28 Moreover, Collinsella contributes to the pathogenesis in rheumatoid arthritis by increasing gut permeability, reducing the expression of tight junction proteins in epithelial cells and inducing the expression of IL-17 network cytokines,Citation10 which are frequently involved in inflammatory diseases.Citation29 Augmented gut permeability is believed to result in leakage of bacteria and endotoxins from the gut lumen to the mesenteric lymph nodes and portal circulation, stimulating peritoneal, intestinal and hepatic macrophagesCitation30 to release numerous cytokines that are key inducers of insulin resistance.Citation31 Therefore, we hypothesize that low dietary fiber consumption in early pregnancy results in the overgrowth of Collinsella spp. disturbing gut integrity, which in turn contributes to increased inflammation and onset of maternal hyperglycemia. Outside pregnancy, there is a clear link between obesity and low-level inflammation,Citation32 which could further interact with Collinsella-induced inflammation. However, in pregnancy, this link is not as clearCitation33 and it would therefore be of interest to investigate if our findings can be extended to lean pregnant women.
Women with the highest and lowest dietary fiber intake displayed different in silico fecal microbial community dynamics. The recommended daily intake of dietary fiber for pregnant women in Australia and New Zealand is 28 g/day.Citation34 In our cohort only 10% reached or exceeded the recommended dietary fiber intake. This may reflect our specific study population of overweight and obese women but is in line with other reported cohorts.Citation35,Citation36 Correction of dietary fiber by total energy intake was performed to obtain a measure of fiber intake that was independent of overall energy intake. Greater Collinsella abundance was associated with low fiber intake in both the corrected and uncorrected network analyses, suggesting a strong connection with fiber intake independent of overall energy intake. Outside pregnancy, a fiber-deprived western diet, a fiber-deprived red meat diet and a diet high in refined wheat bread both increase the relative abundance of Collinsella,Citation37-Citation39 whereas a fiber-rich diet reduces Collinsella abundance and improves glucose metabolism in T2D patients.Citation14 Since Collinsella spp. do not degrade dietary fiber but can ferment a wide range of different carbohydrates including complex sugars such as starch,Citation40 the growth of Collinsella spp is favored by a diet with reduced fiber content. In our corrected network analysis, the interactions between community members and fiber intake became clearer, enabling the identification of two compositionally distinct clusters. Besides Collinsella, the low-fiber diet was characterized by a higher abundance of some major lactate-producing bacteria including Bifidobacterium, Streptococcus, Lactococcus, Blautia and Turicibacter in addition to genera Eubacterium, Bilophila, Adlercreutzia and Sutterella. It is unclear whether consumption of a low fiber diet implied a diet that promotes lactate formation (e.g. dairy products). However, increases in intestinal lactate concentrations have been linked to insulin resistanceCitation41 and greater intestinal permeability.Citation42 Lactate concentrations in the gut lumen depend on the balance between its production, (host) absorption and (microbe) utilization, and under normal physiological conditions lactate does not accumulate in the human colon.Citation43 Some bacteria use lactate as an alternative energy source and synthesize butyrate and propionate from lactate, which helps avoid metabolic acidosis.Citation44 In vitro studies have shown that lactate produced by lactic acid bacteria, e.g. Bifidobacterium, can be further metabolized by members of the Eubacterium genus.Citation45 These in vitro studies are consistent with the observed changes here in women with low fiber intake, and suggest that lactate-metabolizing bacteria like Eubacterium may attenuate the detrimental effects from lactate production in the gastrointestinal tract.Citation46
In contrast, in both network analyses high dietary fiber intake was associated with greater levels of bacteria widely recognized to be “beneficial”, and similar in composition to non-pregnant healthy subjects.Citation47,Citation48 Absolute and relative amounts of SCFA have been considered as biomarkers of health status outside pregnancy. Keystone species within the gut microbiome associated to Clostridium clusters XIVa, IV and IX (Roseburia, Coprococcus, Ruminococcus, Dorea, Lachnospira, Faecalibacterium, Acidaminococcus) were more abundant in women with high fiber intakes, in addition to the genera Bacteroides, Holdemania and Phascolarctobacterium. Butyrate producers can play an important role in gut homeostasis by increasing mucin production which results in enhanced tight junction integrity.Citation49 Contrarily, the gut microbiota of subjects with chronic inflammation exhibit decreased abundance of members of Clostridium cluster IV, notably Faecalibacterium prausnitzii and XIVa compared to healthy individuals.Citation50 Furthermore, murine models have shown that acetate produced by members of genus Bacteroides is used by F. prausnitzii for butyrate formation and this has a positive impact on the integrity of the gut mucosal barrier.Citation51 The growth of Holdemania and Phascolarctobacterium are also enhanced by dietary fiber,Citation52 and reduced abundances of Holdemania, and reduced “butyrate-producing capacity” are associated with elevated blood pressure in non-pregnant subjects,Citation53 and elevated blood pressure in pregnancy,Citation54 respectively. Akkermansia spp. are also widely recognized to attenuate inflammation and directly modulate metabolic health of the host.Citation55 However Akkermansia spp. abundance was no longer significantly greater in women with high fiber intake after correction for energy intake. This may be due to a preference of Akkermansia muciniphila for prebiotic compounds such as inulin and/or fructo-oligosaccharides,Citation56 which could explain its relationship with overall caloric intake rather than dietary fiber consumed in our study. Taken together, differences in dietary fiber intake may result in shifts in the pregnant gut microbiota, especially in the balance of fermentation schemes between lactate- and acetate/butyrate producing bacteria. The predicted functional analysis of gut microbiota of women with low and high fiber intake indicated differences in pyrimidine metabolism, aminobenzoate degradation as well as amino acid degradation and metabolism. These pathways have previously been identified as being important determinants for the interaction between gut microbiota composition and human health.Citation57 Further studies are required to unveil the functional roles of these distinct microbiota profiles during pregnancy and if these are similar in lean pregnant women.
The interplay between dietary fiber, glucose metabolism and pregnancy has been extensively investigated.Citation58 Prior studies have shown the protective role of dietary fibers and polyunsaturated fats in decreasing the risk of developing GDMCitation59; however, the involvement of the gut microbiota in maternal glucose homeostasis has not been fully explored. In this cohort, only fiber ingestion was negatively associated with maternal insulin levels and with Collinsella abundance. Since Collinsella can dehydroxylate bile acids improving cholesterol reuptake in the enterohepatic pathway,Citation60 it could contribute to increasing circulating cholesterol levels although our results indicate that it is not a major regulator of cholesterol levels in women who remain normoglycemic in pregnancy. We were unable to replicate the associations between Collinsella abundance and lipid metabolism, which we reported in a previous subset of SPRING participants in early pregnancy.Citation6 This difference likely results from the absence of women who developed GDM in the current cohort but which made up 50% of the previous cohort and in whom larger excursions in lipids were already present at baseline.
The present findings indicate that a maternal diet that is low in dietary fiber intake may modify gut microbiota composition, which may contribute to susceptibility to impaired glucose tolerance in overweight and obese pregnant women. Therefore medical nutrition therapy along with gut microbiota modification may provide avenues of non-pharmacologic management for elevated maternal glycemic profiles. It is still unclear what the optimal strategy for the reduction of Collinsella abundance is, and if pregnancy outcomes can be improved by lowering its abundance within the pregnant microbiota. Murine models could potentially directly evaluate the role of Collinsella during gestation. In addition the duration and persistance of low-fiber induced dysbiosis in gestation is undetermined given that we have studied only samples obtained in early gestation. The use of food frequency questionnaires is well accepted but does have inherent biases; however, the dietary intake in this study resembles that of other pregnancy cohorts.Citation61,Citation62 Furthermore, since the women in this cohort did not develop GDM and the interactions between Collinsella abundance and insulin were independent of maternal BMI, the correlation between dietary fiber intake and gut microbiota composition may be generalizable to the obstetric population as a whole although the relationship needs to be replicated in lean women as well as at later stages of pregnancy. As different types of dietary fibers can modulate distinctive taxa, future studies are warranted to confirm if different sources of dietary fibers alter the underlying mechanism of the observed association.
Conclusion
This study highlights the significant association between Collinsella abundance in the maternal gut microbiota and maternal metabolic health in early pregnancy in overweight and obese women, which may be affected by dietary fiber intake. These results offer promising perspectives for additional strategies to improve maternal metabolic and inflammatory health.
Declarations
Ethics approval and consent to participate
This study was approved by the Human Research Ethics committees of the Royal Brisbane and Women's Hospital (HREC/RBWH/11/467) and The University of Queensland (2012000080). Written informed consent was obtained from all participants prior to enrolment in the trial. All experiments were performed in accordance with relevant guidelines and regulations.
Consent for publication
Consent was obtained within the informed consent procedures for the SPRING trial.
Availability of data and material
The sequences are available in the NCBI database (Accession number: Bioproject PRJNA385231; SRA: SRP106249).
Competing interest
The SPRING study has received probiotics and placebo products from Chr. Hansen A/S. The authors have no further conflicts of interest.
Author contributions
L.F.G-A provided intellectual input, researched data, performed analysis and wrote and edited the manuscript. H.L.B, H.D.M and L.K.C provided intellectual input and edited the manuscript. S.A.W provided nutritional advice and edited the manuscript. M.M provided intellectual input and technical expertise and edited the manuscript. M.D.N provided intellectual input, designed the research and wrote and edited the manuscript. Dr Dekker Nitert is the guarantor of this work and, as such, had full access to all the data in the study and takes responsibility of the integrity of the data and the accuracy of the data analysis.
2017GUTMICROBES0084R1-s02.docx
Download MS Word (6 MB)Acknowledgment
The authors would like to sincerely thank the SPRING participants and the SPRING trial group members Anne Tremellen, Katie Foxcroft, Dr Jacinta Tobin, Dr Chris McSweeney, Prof Peter O'Rourke and Dr Barbara Lingwood.
Funding
The SPRING study is supported by the National Health & Medical Research Council (grant no. 1028575) and the Royal Brisbane and Women's Hospital Foundation.
References
- Qin J, Li R, Raes J, Arumugam M, Burgdorf KS, Manichanh C, Nielsen T, Pons N, Levenez F, Yamada T, et al. A human gut microbial gene catalogue established by metagenomic sequencing. Nature. 2010;464:59–65. doi:10.1038/nature08821. PMID:20203603.
- Qin J, Li Y, Cai Z, Li S, Zhu J, Zhang F, Liang S, Zhang W, Guan Y, Shen D, et al. A metagenome-wide association study of gut microbiota in type 2 diabetes. Nature. 2012;490:55–60. doi:10.1038/nature11450. PMID:23023125.
- Cani PD, Osto M, Geurts L, Everard A. Involvement of gut microbiota in the development of low-grade inflammation and type 2 diabetes associated with obesity. Gut Microbes. 2012;3:279–88. doi:10.4161/gmic.19625. PMID:22572877.
- Larsen N, Vogensen FK, van den Berg FW, Nielsen DS, Andreasen AS, Pedersen BK, Al-Soud WA, Sørensen SJ, Hansen LH, Jakobsen M. Gut microbiota in human adults with type 2 diabetes differs from non-diabetic adults. PLoS One. 2010;5:e9085. doi:10.1371/journal.pone.0009085. PMID:20140211.
- Koren O, Goodrich JK, Cullender TC, Spor A, Laitinen K, Backhed HK, Gonzalez A, Werner JJ, Angenent LT, Knight R, et al. Host remodeling of the gut microbiome and metabolic changes during pregnancy. Cell. 2012;150:470–80. doi:10.1016/j.cell.2012.07.008. PMID:22863002.
- Gomez-Arango LF, Barrett HL, McIntyre HD, Callaway LK, Morrison M, Dekker Nitert M, et al. Connections Between the Gut Microbiome and Metabolic Hormones in Early Pregnancy in Overweight and Obese Women. Diabetes. 2016;65:2214–23. doi:10.2337/db16-0278. PMID:27217482.
- Chow J, Tang H, Mazmanian SK. Pathobionts of the gastrointestinal microbiota and inflammatory disease. Curr Opin Immunol. 2011;23:473–80. doi:10.1016/j.coi.2011.07.010. PMID:21856139.
- Thomas Clavel PL, Cedric Charrier. The Family Coriobacteriaceae. Book Chapter: The Prokaryotes. 2014:201–38.
- Lambeth SM, Carson T, Lowe J, Ramaraj T, Leff JW, Luo L, Bell CJ, Shah VO. Composition, Diversity and Abundance of Gut Microbiome in Prediabetes and Type 2 Diabetes. J Diabetes Obes. 2015;2:1–7. PMID:26756039.
- Chen J, Wright K, Davis JM, Jeraldo P, Marietta EV, Murray J, Nelson H, Matteson EL, Taneja V. An expansion of rare lineage intestinal microbes characterizes rheumatoid arthritis. Genome Med 2016;8:43. doi:10.1186/s13073-016-0299-7. PMID:27102666.
- Ridlon JM, Kang DJ, Hylemon PB. Bile salt biotransformations by human intestinal bacteria. J Lipid Res. 2006;47:241–59. doi:10.1194/jlr.R500013-JLR200. PMID:16299351.
- Cani PD, Possemiers S, Van de Wiele T, Guiot Y, Everard A, Rottier O, Geurts L, Naslain D, Neyrinck A, Lambert DM, et al. Changes in gut microbiota control inflammation in obese mice through a mechanism involving GLP-2-driven improvement of gut permeability. Gut. 2009;58:1091–103. doi:10.1136/gut.2008.165886. PMID:19240062.
- Walker AW, Ince J, Duncan SH, Webster LM, Holtrop G, Ze X, Brown D, Stares MD, Scott P, Bergerat A, et al. Dominant and diet-responsive groups of bacteria within the human colonic microbiota. ISME J. 2011;5:220–30. doi:10.1038/ismej.2010.118. PMID:20686513.
- Candela M, Biagi E, Soverini M, Consolandi C, Quercia S, Severgnini M, Peano C, Turroni S, Rampelli S, Pozzilli P, et al. Modulation of gut microbiota dysbioses in type 2 diabetic patients by macrobiotic Ma-Pi 2 diet. Br J Nutr. 2016;116:80–93. doi:10.1017/S0007114516001045. PMID:27151248.
- Nitert MD, Barrett HL, Foxcroft K, Tremellen A, Wilkinson S, Lingwood B, Tobin JM, McSweeney C, O'Rourke P, McIntyre HD, et al. SPRING: an RCT study of probiotics in the prevention of gestational diabetes mellitus in overweight and obese women. BMC Pregnancy Childbirth. 2013;13:50. doi:10.1186/1471-2393-13-50. PMID:23442391.
- Giles GG IP. Dietary Questionnaire for Epidemiological Studies (Version 2). Australia: The cancer council Victoria; 1996.
- Yu Z, Morrison M. Improved extraction of PCR-quality community DNA from digesta and fecal samples. BioTechniques. 2004;36:808–12. PMID:15152600.
- Caporaso JG, Kuczynski J, Stombaugh J, Bittinger K, Bushman FD, Costello EK, Fierer N, Peña AG, Goodrich JK, Gordon JI, et al. QIIME allows analysis of high-throughput community sequencing data. Nat Methods. 2010;7:335–6. doi:10.1038/nmeth.f.303. PMID:20383131.
- Kageyama A, Sakamoto M, Benno Y. Rapid identification and quantification of Collinsella aerofaciens using PCR. FEMS Microbiol Lett. 2000;183:43–7. doi:10.1111/j.1574-6968.2000.tb08931.x. PMID:10650200.
- Zakrzewski M, Proietti C, Ellis JJ, Hasan S, Brion MJ, Berger B, Krause L. Calypso: a user-friendly web-server for mining and visualizing microbiome-environment interactions. Bioinformatics (Oxford, England). 2017;33:782–783. doi:10.1093/bioinformatics/btw725.
- Tarini J, Wolever TM. The fermentable fibre inulin increases postprandial serum short-chain fatty acids and reduces free-fatty acids and ghrelin in healthy subjects. Applied Physiology, Nutrition, and Metabolism = Physiologie appliquee, Nutrition et Metabolisme. 2010;35:9–16. doi:10.1139/H09-119. PMID:20130660.
- Alminger M, Eklund-Jonsson C. Whole-grain cereal products based on a high-fibre barley or oat genotype lower post-prandial glucose and insulin responses in healthy humans. Eur J Nutr. 2008;47:294–300. doi:10.1007/s00394-008-0724-9. PMID:18633670.
- Martinez I, Lattimer JM, Hubach KL, Case JA, Yang J, Weber CG, Louk JA, Rose DJ, Kyureghian G, Peterson DA, et al. Gut microbiome composition is linked to whole grain-induced immunological improvements. ISME J. 2013;7:269–80. doi:10.1038/ismej.2012.104. PMID:23038174.
- Desai MS, Seekatz AM, Koropatkin NM, Kamada N, Hickey CA, Wolter M, Pudlo NA, Kitamoto S, Terrapon N, Muller A, et al. A Dietary Fiber-Deprived Gut Microbiota Degrades the Colonic Mucus Barrier and Enhances Pathogen Susceptibility. Cell. 2016;167:1339–53.e21. doi:10.1016/j.cell.2016.10.043. PMID:27863247.
- Brown K, DeCoffe D, Molcan E, Gibson DL. Diet-induced dysbiosis of the intestinal microbiota and the effects on immunity and disease. Nutrients. 2012;4:1095–119. doi:10.3390/nu4081095. PMID:23016134.
- Rajilic-Stojanovic M, de Vos WM. The first 1000 cultured species of the human gastrointestinal microbiota. FEMS Microbiol Rev. 2014;38:996–1047. doi:10.1111/1574-6976.12075. PMID:24861948.
- Fujio-Vejar S, Vasquez Y, Morales P, Magne F, Vera-Wolf P, Ugalde JA, Navarrete P, Gotteland M. The Gut Microbiota of Healthy Chilean Subjects Reveals a High Abundance of the Phylum Verrucomicrobia. Front Microbiol. 2017;8:1221. doi:10.3389/fmicb.2017.01221. PMID:28713349.
- Brown CT, Davis-Richardson AG, Giongo A, Gano KA, Crabb DB, Mukherjee N, Casella G, Drew JC, Ilonen J, Knip M, et al. Gut microbiome metagenomics analysis suggests a functional model for the development of autoimmunity for type 1 diabetes. PLoS One. 2011;6:e25792. doi:10.1371/journal.pone.0025792. PMID:22043294.
- Ma HY, Xu J, Liu X, Zhu Y, Gao B, Karin M, Tsukamoto H, Jeste DV, Grant I, Roberts AJ, et al. The role of IL-17 signaling in regulation of the liver-brain axis and intestinal permeability in Alcoholic Liver Disease. Curr Pathobiol Rep. 2016;4:27–35. doi:10.1007/s40139-016-0097-3. PMID:27239399.
- Muriel Derrien DA, Siv Ahrne, Bengt Jeppsson, Go ̈ ran Molin, Nadia Osman, Jelena Stsepetova, Elaine E. Vaughan, Willem M. de Vos, Arthur C. Ouwehand. The Intestinal Mucosa as a Habitat of the Gut Microbiota and a Rational Target for Probiotic. Functionality and Safety Microbial Ecology in Health and Disease. 2004;16:137–44. doi:10.1080/08910600410032286.
- Cani PD, Amar J, Iglesias MA, Poggi M, Knauf C, Bastelica D, Neyrinck AM, Fava F, Tuohy KM, Chabo C, et al. Metabolic endotoxemia initiates obesity and insulin resistance. Diabetes. 2007;56:1761–72. doi:10.2337/db06-1491. PMID:17456850.
- Wensveen FM, Valentić S, Šestan M, Turk Wensveen T, Polić B. The “Big Bang” in obese fat: Events initiating obesity-induced adipose tissue inflammation. Eur J Immunol. 2015;45:2446–56. doi:10.1002/eji.201545502. PMID:26220361.
- Pendeloski KPT, Ono E, Torloni MR, Mattar R, Daher S. Maternal obesity and inflammatory mediators: A controversial association. Am J Reprod Immunol. 2017;77:e12674-n/a. doi:10.1111/aji.12674.
- The Nutrient Reference Values N, MoH. Nutrient Reference Values for Australia and New Zealand Including Recommended Dietary Intakes. NHMRC Publications. 2006.
- Koren O, Goodrich JK, Cullender TC, Spor A, Laitinen K, Kling Backhed H, Gonzalez A, Werner JJ, Angenent LT, Knight R, et al. Host Remodeling of the Gut Microbiome and Metabolic Changes during Pregnancy. Cell. 2012;150:470–80. doi:10.1016/j.cell.2012.07.008. PMID:22863002.
- Röytiö H, Mokkala K, Vahlberg T, Laitinen K. Dietary intake of fat and fibre according to reference values relates to higher gut microbiota richness in overweight pregnant women. British Journal of Nutrition. 2017;118:343–52. doi:10.1017/S0007114517002100. PMID:28901891.
- Amato KR, Yeoman CJ, Cerda G, Schmitt CA, Cramer JD, Miller ME, Gomez A, Turner TR, Wilson BA, Stumpf RM, et al. Variable responses of human and non-human primate gut microbiomes to a Western diet. Microbiome. 2015;3:53. doi:10.1186/s40168-015-0120-7. PMID:26568112.
- Lappi J, Salojarvi J, Kolehmainen M, Mykkanen H, Poutanen K, de Vos WM, Salonen A. Intake of whole-grain and fiber-rich rye bread versus refined wheat bread does not differentiate intestinal microbiota composition in Finnish adults with metabolic syndrome. J Nutr. 2013;143:648–55. doi:10.3945/jn.112.172668. PMID:23514765.
- Foerster J, Maskarinec G, Reichardt N, Tett A, Narbad A, Blaut M, Boeing H. The Influence of Whole Grain Products and Red Meat on Intestinal Microbiota Composition in Normal Weight Adults: A Randomized Crossover Intervention Trial. PLoS One. 2014;9:e109606. doi:10.1371/journal.pone.0109606. PMID:25299601.
- Kageyama A, Benno Y, Nakase T. Phylogenetic and phenotypic evidence for the transfer of Eubacterium aerofaciens to the genus Collinsella as Collinsella aerofaciens gen. nov., comb. nov. Int J Syst Bacteriol. 1999;49 Pt 2:557–65. doi:10.1099/00207713-49-2-557.
- Choi CS, Kim YB, Lee FN, Zabolotny JM, Kahn BB, Youn JH. Lactate induces insulin resistance in skeletal muscle by suppressing glycolysis and impairing insulin signaling. Am J Physiol Endocrinol Metab. 2002;283:E233–40. doi:10.1152/ajpendo.00557.2001. PMID:12110527.
- Grootjans J, Thuijls G, Verdam F, Derikx JP, Lenaerts K, Buurman WA. Non-invasive assessment of barrier integrity and function of the human gut. World J Gastrointest Surg. 2010;2:61–9. doi:10.4240/wjgs.v2.i3.61. PMID:21160852.
- El Aidy S, Van den Abbeele P, Van de Wiele T, Louis P, Kleerebezem M. Intestinal colonization: how key microbial players become established in this dynamic process: microbial metabolic activities and the interplay between the host and microbes. BioEssays: news and reviews in molecular, cellular and developmental biology. 2013;35:913–23. PMID:23946088.
- Rios-Covian D, Ruas-Madiedo P, Margolles A, Gueimonde M, de Los Reyes-Gavilan CG, Salazar N. Intestinal Short Chain Fatty Acids and their Link with Diet and Human Health. Front Microbiol. 2016;7:185. doi:10.3389/fmicb.2016.00185. PMID:26925050.
- Belenguer A, Duncan SH, Calder AG, Holtrop G, Louis P, Lobley GE, Flint HJ. Two routes of metabolic cross-feeding between Bifidobacterium adolescentis and butyrate-producing anaerobes from the human gut. Appl Environ Microbiol. 2006;72:3593–9. doi:10.1128/AEM.72.5.3593-3599.2006. PMID:16672507.
- Duncan SH, Louis P, Flint HJ. Lactate-utilizing bacteria, isolated from human feces, that produce butyrate as a major fermentation product. Appl Environ Microbiol. 2004;70:5810–7. doi:10.1128/AEM.70.10.5810-5817.2004. PMID:15466518.
- den Besten G, van Eunen K, Groen AK, Venema K, Reijngoud DJ, Bakker BM. The role of short-chain fatty acids in the interplay between diet, gut microbiota, and host energy metabolism. J Lipid Res. 2013;54:2325–40. doi:10.1194/jlr.R036012. PMID:23821742.
- De Filippo C, Cavalieri D, Di Paola M, Ramazzotti M, Poullet JB, Massart S, Collini S, Pieraccini G, Lionetti P. Impact of diet in shaping gut microbiota revealed by a comparative study in children from Europe and rural Africa. Proc Natl Acad Sci U S A. 2010;107:14691–6. doi:10.1073/pnas.1005963107. PMID:20679230.
- Peng L, Li ZR, Green RS, Holzman IR, Lin J. Butyrate enhances the intestinal barrier by facilitating tight junction assembly via activation of AMP-activated protein kinase in Caco-2 cell monolayers. J Nutr. 2009;139:1619–25. doi:10.3945/jn.109.104638. PMID:19625695.
- Frank DN, St Amand AL, Feldman RA, Boedeker EC, Harpaz N, Pace NR. Molecular-phylogenetic characterization of microbial community imbalances in human inflammatory bowel diseases. Proc Natl Acad Sci U S A. 2007;104:13780–5. doi:10.1073/pnas.0706625104. PMID:17699621.
- Wrzosek L, Miquel S, Noordine ML, Bouet S, Joncquel Chevalier-Curt M, Robert V, Philippe C, Bridonneau C, Cherbuy C, Robbe-Masselot C, et al. Bacteroides thetaiotaomicron and Faecalibacterium prausnitzii influence the production of mucus glycans and the development of goblet cells in the colonic epithelium of a gnotobiotic model rodent. BMC Biol. 2013;11:61. doi:10.1186/1741-7007-11-61. PMID:23692866.
- Lecomte V, Kaakoush NO, Maloney CA, Raipuria M, Huinao KD, Mitchell HM, Morris MJ. Changes in gut microbiota in rats fed a high fat diet correlate with obesity-associated metabolic parameters. PLoS One. 2015;10:e0126931. doi:10.1371/journal.pone.0126931. PMID:25992554.
- Honour J. Gut Dysbiosis and Hypertension–new or resurrected. Endocrine Abstracts. 2016;44:SE1.3. doi:10.1530/endoabs.44.SE1.3.
- Gomez-Arango LF, Barrett HL, McIntyre HD, Callaway LK, Morrison M, Dekker Nitert M. Increased Systolic and Diastolic Blood Pressure Is Associated With Altered Gut Microbiota Composition and Butyrate Production in Early Pregnancy. Hypertension (Dallas, Tex : 1979). 2016;68:974–81. doi:10.1161/HYPERTENSIONAHA.116.07910. PMID:27528065.
- Everard A, Belzer C, Geurts L, Ouwerkerk JP, Druart C, Bindels LB, Guiot Y, Derrien M, Muccioli GG, Delzenne NM, et al. Cross-talk between Akkermansia muciniphila and intestinal epithelium controls diet-induced obesity. Proc Natl Acad Sci U S A. 2013;110:9066–71. doi:10.1073/pnas.1219451110. PMID:23671105.
- Derrien M, Belzer C, de Vos WM. Akkermansia muciniphila and its role in regulating host functions. Microb Pathog. 2016;106:171–181. doi:10.1016/j.micpath.2016.02.005. PMID:26875998.
- Larsen PE, Dai Y. Metabolome of human gut microbiome is predictive of host dysbiosis. GigaScience. 2015;4:42. doi:10.1186/s13742-015-0084-3. PMID:26380076.
- Tieu J, Crowther CA, Middleton P. Dietary advice in pregnancy for preventing gestational diabetes mellitus. Cochrane Database Syst Rev. 2008:Cd006674. PMID:18425961.
- Zhang C, Liu S, Solomon CG, Hu FB. Dietary fiber intake, dietary glycemic load, and the risk for gestational diabetes mellitus. Diabetes Care. 2006;29:2223–30. doi:10.2337/dc06-0266. PMID:17003297.
- Wegner K, Just S, Gau L, Mueller H, Gerard P, Lepage P, Clavel T, Rohn S. Rapid analysis of bile acids in different biological matrices using LC-ESI-MS/MS for the investigation of bile acid transformation by mammalian gut bacteria. Anal Bioanal Chem. 2017;409:1231–45. doi:10.1007/s00216-016-0048-1. PMID:27822648.
- Dodd JM, Turnbull D, McPhee AJ, Deussen AR, Grivell RM, Yelland LN, Louise J, Mcphee AJ, Robinson JS, Owens JA. Antenatal lifestyle advice for women who are overweight or obese: LIMIT randomised trial. BMJ. 2014;348:g1285. doi:10.1136/bmj.g1285. PMID:24513442.
- Grivell RM, Yelland LN, Deussen A, Crowther CA, Dodd JM. Antenatal dietary and lifestyle advice for women who are overweight or obese and the effect on fetal growth and adiposity: the LIMIT randomised trial. BJOG : an international journal of obstetrics and gynaecology. 2016;123:233–43. doi:10.1111/1471-0528.13777. PMID:26841216.
- Abbassi-Ghanavati M, Greer LG, Cunningham FG. Pregnancy and laboratory studies: a reference table for clinicians. Obstet Gynecol. 2009;114:1326–31. doi:10.1097/AOG.0b013e3181c2bde8. PMID:19935037.