ABSTRACT
Chronic disruption of the intestinal microbiota in adult cystic fibrosis (CF) patients is associated with local and systemic inflammation, and has been linked to the risk of serious comorbidities. Supplementation with high amylose maize starch (HAMS) might provide clinical benefit by promoting commensal bacteria and the biosynthesis of immunomodulatory metabolites. However, whether the disrupted CF gut microbiota has the capacity to utilise these substrates is not known. We combined metagenomic sequencing, in vitro fermentation, amplicon sequencing, and metabolomics to define the characteristics of the faecal microbiota in adult CF patients and assess HAMS fermentation capacity. Compared to healthy controls, the faecal metagenome of adult CF patients had reduced bacterial diversity and prevalence of commensal fermentative clades. In vitro fermentation models seeded with CF faecal slurries exhibited reduced acetate levels compared to healthy control reactions, but comparable levels of butyrate and propionate. While the commensal genus Faecalibacterium was strongly associated with short chain fatty acid (SCFA) production by healthy microbiota, it was displaced in this role by Clostridium sensu stricto 1 in the microbiota of CF patients. A subset of CF reactions exhibited enterococcal overgrowth, resulting in lactate accumulation and reduced SCFA biosynthesis. The addition of healthy microbiota to CF faecal slurries failed to displace predominant CF taxa, or substantially influence metabolite biosynthesis. Despite significant microbiota disruption, the adult CF gut microbiota retains the capacity to exploit HAMS. Our findings highlight the potential for taxa associated with the altered CF gut microbiotato mediate prebiotic effects in microbial systems subject to ongoing perturbation, irrespective of the depletion of common commensal clades.
Introduction
Cystic fibrosis (CF) is an autosomal recessive disorder that results in altered transport of electrolytes and fluid across epithelial surfaces. Chronic suppurative respiratory disease, arising due to impaired clearance of dehydrated airway secretions, is typically the principal cause of morbidity and mortality.Citation1 However, CF is also associated with chronic gastrointestinal (GI) disease.Citation2–Citation4 Impaired mucin hydration, reduced bicarbonate secretion, abnormal bile salt physiology, and impaired fat absorption, all contribute to GI dysfunction and chronic inflammation.Citation2,Citation5 In turn, GI inflammation is implicated in mucosal abnormalities and increased intestinal permeabilityCitation2, and contributes to an increased risk of a range of intestinal morbidities, including cancer.Citation6 Indeed, rates of GI malignancy are significantly higher in patients with CF compared with the general population.Citation7–Citation9 Chronic local inflammation also contributes to a systemic pro-inflammatory state, with GI inflammation linked to elevated circulating cytokines and chemokines, soluble adhesion molecules, and acute phase reactants.Citation10
The abnormal CF gut environment caused by intestinal exocrine malfunction, combined with the effects of antibiotic therapies for chronic lung infections, results in significant alteration of the gut microbiota.Citation11-Citation18 As a consequence, CF patients exhibit a reduced relative abundance of commensal fermentative bacteria, including members of the Bifidobacterium genusCitation11,Citation12,Citation15,Citation16 and Clostridium clusters XIVa and IV (which include Faecalibacterium prausnitzii and Ruminococcus bromii).Citation11,Citation15-Citation17 At the same time, the relative abundance of pro-inflammatory or potentially pathogenic taxa, particularly Proteobacteria (including Escherichia coli) and enterococci (including Enterococcus faecalis and Enterococcus faecium) is increased.Citation13,Citation14,Citation16,Citation17
Altered CF gut microbiology is likely to compound the pro-inflammatory effects of the underlying disease. In non-CF contexts, alterations to the intestinal microbiota, sometimes referred to as “dysbiosis”, has been shown to contribute to the dysregulation of mucosal immunityCitation19, and an increase in levels of genotoxic, pro-inflammatory, and hepato-modulatory metabolites.Citation20 By preventing carbohydrate fermentation by members of the colonic microbiota, changes in microbiota composition can also result in reduced production of protective metabolites, including short chain fatty acids (SCFAs).Citation21,Citation22 SCFAs have a wide range of anti-inflammatory properties, including the ability to increase colonic regulatory T cellsCitation23, alter dendritic cell and macrophage functionCitation24, and reduce production of pro-inflammatory cytokines.Citation25,Citation26 SCFAs are also important in the regulation of glycaemic responses and triglyceride metabolismCitation27, are essential for colonic epithelial homeostasisCitation28, and barrier functionCitation29, and contribute substantially to energy reclamation from the gut.Citation30 Reduced SCFA production might therefore be critical in CF, where patients experience chronic inflammation and nutritional malabsorption.
Prebiotics are non-digestible compounds that confer health benefits by promoting the proliferation of commensal gut species or the production of beneficial metabolites. These compounds include resistant starches (RS); complex carbohydrates that are not digested and absorbed in the small intestine, but pass into the large bowel and act as substrates for bacterial fermentation.Citation31 By increasing the levels of fermentation substrate in the colon, supplementation with compounds such as high amylose maize starch (HAMS) can increase both the abundance of potentially beneficial microbesCitation32, and the production of SCFAs.Citation33 RS supplementation has proven to be effective in reducing the risk of both cancer and diabetes by suppressing the development of epithelial adducts Citation34, and reducing insulin resistance and markers of systemic inflammation.Citation35
However, despite a growing interest in the potential clinical benefits of dietary prebiotics in CF, it is not known whether the CF intestinal microbiota retains the capacity to exploit these substrates. Clinical prebiotic use typically assumes the competence of the recipient’s microbiome in utilising the supplemented substrates. A failure to assess microbial capacity prior to supplementation potentially contributes to inter-subject differences in prebiotic response.Citation36 Prebiotic approaches also typically fail to consider the potential to cause detrimental changes in individuals in whom the gut microbiota is already substantially disrupted, such as the selective promotion of potentially pathogenic taxa.
Our aim was to investigate the potential for HAMS supplementation to provide microbiome-mediated benefit in patients with CF. We undertook a detailed metagenomic analysis of the faecal microbiome composition and functional capacity in adult CF patients. Utilising an in vitro fermentation model, we then assessed the response to HAMS of faecal microbiota from CF patients and healthy non-CF controls, including the resulting changes in microbiota structure, global metabolomic output, and SCFA production.
Results
Faecal microbiota characteristics in adults with CF
Faecal microbiota composition differed significantly between CF patients and healthy non-CF controls (-). Taxa richness was reduced in CF samples (median observed OTUs, CF: 272 [IQR: 107], control: 496 [IQR: 102], p < 0.0001), as was Faith’s phylogenetic diversity (median diversity, CF: 10.1 [IQR: 3.3]; control: 16.4 [IQR: 3.7], p < 0.0001). Simpson’s evenness did not differ significantly between groups (p = 0.27). Beta diversity, assessed based on weighted UniFrac distances, also differed significantly between CF samples and controls (P(perm) = 0.0001, square root estimated components of variation (ECV) = 0.19, 9,919 permutations; ). Differences in microbiota composition were characterised by a significantly greater relative abundance of important commensal taxa, such as Faecalibacterium prausnitzii, Eubacterium rectale, Prevotella copri, and Ruminococcus bromii in non-CF subjects, and a greater relative abundance of Clostridium symbiosum, Veillonella parvula, Enterococcus faecalis, and Ruminococcus gnavus in patients with CF (alpha < 0.05 for Kruskal-Wallis test and LDA> 4, data not shown).
Figure 1. Microbiota characteristics of CF (red) and non-CF (black) faecal samples at baseline (pre-fermentation). A) Observed OTUs between microbiota community of CF and non-CF samples. B) Principal coordinate analysis (PCoA) derived from weighted UniFrac distance of CF and non-CF samples, PERMANOVA, P(perm) = 0.0001, Pseudo-F = 7.27.
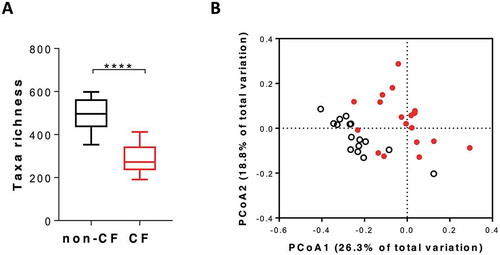
The representation of functional genes within both CF and healthy faecal metagenomes also differed significantly (P(perm) = 0.0019, ECV = 6.15, 9,939 permutations). This divergence included significant differences in the proportion of normalised reads that mapped to individual KEGG pathways, including important genes involved in carbohydrate metabolism (Supplementary Figure1). The gene encoding phosphotransacetylase (pta), an enzyme that catalyses the conversion of acetyl-CoA to acetyl-phosphate in the acetate biosynthesis pathway, was significantly lower in CF metagenomes compared to healthy controls (Supplementary Figure 2A). In contrast, galA, the gene that encodes alpha-galactosidase in the galactose metabolic pathway, was significantly more highly represented in CF metagenomes (Supplementary Figure 1). No significant differences were observed in the representation of normalised levels of butyrate or propionate biosynthesis pathway genes in CF and healthy controls (Supplementary Figure 2B-C), suggesting that the fermentation pathways resulting in the production of these SCFAs is conserved in the CF metagenome.
SCFA production during in vitro fermentation of HAMS
Levels of acetate, butyrate, propionate, and total SCFA were determined before and after in vitro HAMS fermentation by CF and control faecal slurries (-). Acetate levels were significantly lower in CF reactions compared to controls (median concentration, CF: 9.36 mmol/L [IQR: 6.63], control: 21.48 mmol/L [IQR: 11.40], p = 0.0007, ). While total SCFA levels were also significantly reduced (median concentration, CF: 15.03 mmol/L [IQR: 12.77], control: 28.74 mmol/L [IQR: 5.89], p = 0.0001, ), levels of butyrate and propionate did not differ significantly between groups.
Figure 2. SCFA production of non-CF control and CF samples during the in vitro fermentation. Net production (post-fermentation subtracted from pre-fermentation) of A) acetate; B) butyrate; C) propionate; and D) total SCFA. *** p < 0.001, **** p < 0.0001.
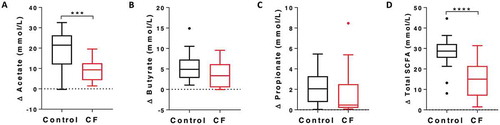
SCFA production was associated with changes in the relative abundance of different bacterial taxa in CF and control reactions following HAMS fermentation (-; Supplementary Figure 3). In healthy controls, acetate concentration showed a strong positive correlation with the relative abundance of Dorea (r = 0.78, p = 0.021, ) and Anaerostipes (r = 0.72, p = 0.039, Supplementary Figure 3); butyrate concentrations were positively correlated with relative abundance of the Faecalibacterium (r = 0.84, p = 0.007, ); and propionate concentration was significantly correlated with the relative abundance of the Bacteroides (r = 0.77, p = 0.049, ). In reactions using CF faecal inocula, butyrate levels were strongly correlated with the relative abundance of Clostridium sensu stricto cluster 1 (Clostridium ss1, r = 0.83, p = 0.0036, ) and an unclassified member of the Clostridiales order (r = 0.67, p = 0.0036, Supplementary Figure 3). Propionate concentrations in CF reactions were significantly correlated with the relative abundance of the Veillonella genus (r = 0.87, p = 0.0071 ). Acetate concentrations did not correlate significantly with any bacterial taxa in CF reactions, although a positive trend with the unclassified member of the Clostridiales order was observed (r = 0.67, p = 0.12, ). Taxa that showed positive correlations with SCFA production in healthy controls, including Dorea, Anaerostipes, Faecalibacterium, Bacteroides, were all significantly lower in post-fermentation CF samples (Supplementary Figure 4).
Figure 3. Representative taxa that were most stongly correlated with SCFA concentration post fermentation for non-CF control (black) and CF (red) samples (Full data of correlation between taxa and SCFA is included in Supplementary Figure 3). P values were from spearman correltaion adjusted by Benjamini-Hochberg FDR procedure.
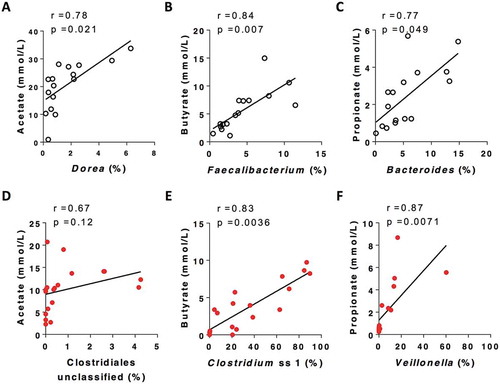
Inter-subject variation in SCFA production
HAMS fermentation reactions were highly reproducible, both in terms of SCFA production (R = −0.0006, p = 0.42, ANOSIM for experiment run 1 and run 2) and shifts in microbiota composition (R = −0.037, p = 0.69, ANOSIM for experiment run 1 and run 2). However, substantial inter-subject differences in SCFA biosynthesis were observed within both CF and control groups (Supplementary Figure 5A-H). CF and healthy control subjects were each divided into “high responder” (high-R) and “low responder” (low-R) groups according to changes in median acetate, butyrate, propionate and total SCFA concentrations during fermentation (Supplementary Figure 5A-H). In reactions involving faecal slurries from healthy individuals, high butyrate production was associated with a high relative abundance of Faecalibacterium (). In contrast, low butyrate production was associated with a high relative abundance of Clostridium ss1 (), with a significant inverse correlation between these two taxa (). While a similar dichotomy was seen for CF samples, the bacterial taxa associated with high and low responder status were different. CF high-R samples (for both acetate and butyrate) were associated with a high relative abundance of Clostridium ss1, while CF low-R samples showed a high relative abundance of Enterococcus (-). Again, a significant negative correlation was observed between these taxa (r = −0.62, p = 0.005, .
Figure 4. Butyrate production associated bacteria in low- and high- responder (low-R and high-R) control and CF reactions A) Relative abundance of Faecalibacterium in control low-R and high-R. B) Relative abundance of Clostridium ss1 in non-CF low-R and high-R. C) Correlation between relative abundance of Clostridium ss1 and Faecalibacterium (spearman) in non-CF sample (black). D) Relative abundance of Clostridium ss1 in CF low-R and high-R. E) Relative abundance of Enterococcus in CF low-R and high-R. F) Correlation between relative abundance of Clostridium ss1 and Enterococcus (spearman) in CF sample (red).
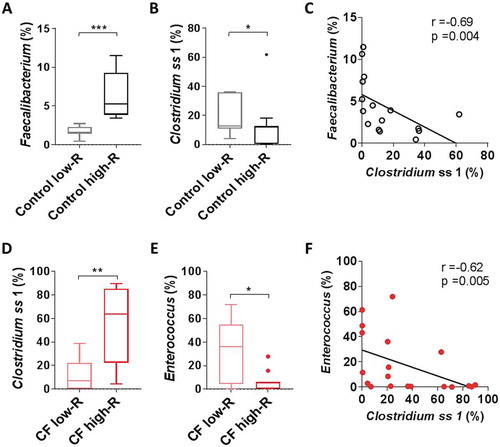
1H-NMR metabolomic analysis
Significant changes in metabolome composition occurred during fermentation in both CF slurry and healthy control slurry reactions (Supplementary Figure 6A-B). While metabolic pathways associated with HAMS fermentation were broadly comparable between CF and control groups (Supplementary Figure 6C-D), significant differences in relative abundance of specific metabolites were observed. Levels of acetate, formate, trimethylamine, and tyramine were all significantly higher in control post-fermentation metabolomes compared to CF reactions (-). In contrast, the relative contributions of cystine, glycine, methionine, and proline to the metabolome were all significantly higher in post-fermentation CF reactions compared to control reactions (-). Metabolites that were significantly elevated in CF reactions were all of relatively low abundance, with their elevation potentially reflecting lower levels of the high abundance metabolites, such as acetate and formate. Post-fermentation levels of lactate, a precursor of acetate, butyrate and propionate, trended higher for CF low-R compared to CF high-R, but did not achieve significance (median peak intensity, CF low-R: 260.9 [IQR: 110.2]; CF high-R: 26.5 [IQR:35.9], p = 0.058, Supplementary Fig. 7D).
Impact of healthy microbiota on SCFA biosynthesis in CF samples
The extent to which the addition of microbiota from healthy control samples could influence taxon predominance and SCFA production by CF faecal slurries was then investigated. The addition of microbiota from high-R control samples to high-R CF samples prior to fermentation did not, however, lead to the displacement of Clostridium ss1 by Faecalibacterium as the taxon most closely correlated with post-fermentation butyrate levels (Supplementary Fig. 8). SCFA levels were also not changed significantly. The impact of introducing microbiota from high-R healthy control samples to low-R CF samples was then assessed. Again, despite the addition of material from samples that contained abundant fermentative commensal taxa, no significant increase in SCFA biosynthesis, or decrease in the post-fermentation relative abundance of Enterococcus, was observed (-).
Figure 6. Total SCFA production (A) and relative abundance of Enterococcus (B) for CF low-Responder (low-R) faecal sample supplemented with non-CF control high-Responder (high-R) sample in a ratio of 1:0.1 of bacterial cells in the in vitro fermentation with HAMS. *Control is the combination of the top 3 non-CF control high-R.
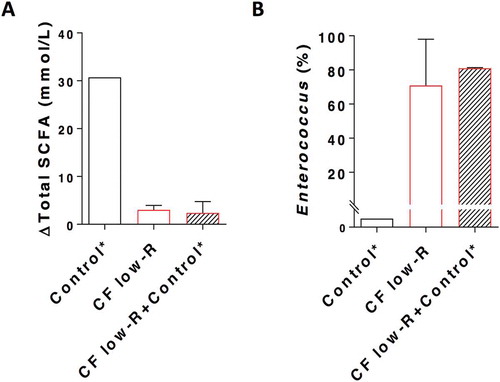
Discussion
We investigated the relative ability of faecal microbiota from adult CF patients and healthy non-CF controls to produce SCFAs through HAMS fermentation, and the bacterial taxa responsible. We report that the CF gut microbiota retains the ability to ferment HAMS in many cases, but that taxa that are characteristic of the altered CF faecal microbiota replace common commensal taxa in undertaking this role. In a subset of CF patients, the presence of HAMS leads to enterococcal overgrowth and the accumulation of lactate, suggesting that a prebiotic strategy would not be appropriate in all instances.
In keeping with previous analyses of the faecal microbiome in paediatric and adult CF patientsCitation11,Citation14–Citation17, we found the relative abundance of common fermentative commensal taxa to be substantially reduced in CF subjects, compared to healthy adults. Particularly notable was the absence of important commensal taxa, such as Faecalibacterium prausnitzii, Eubacterium rectale, and Ruminococcus bromii. These are considered to be keystone species in the degradation of complex carbohydrates and the biosynthesis of SCFA in healthy individuals.Citation37,Citation38 Although diet is an import factor for shaping the gut microbiota, other factors, such as antibiotic exposure and disease related gastrointestinal dysfunction, contribute to an altered gut microbiota in patients with CF. To reflect the requirement for any potential clinical prebiotic approach to provide benefit despite variations in disease, diet, and antibiotic exposure, we conducted our analysis using samples collected within the context of routine care. HAMS was selected as a fermentation substrate based on its potential as a candidate for clinical translation (in addition to its low cost, HAMS has Food and Drug Administration “generally regarded as safe” status).
Despite the low relative abundance of common fermentative taxa, SCFA biosynthesis was still evident in reactions using CF faecal slurries. Perhaps surprisingly, levels of butyrate and propionate production were not significantly lower than those obtained using healthy control slurries. This production of butyrate by the CF faecal microbiota was strongly associated with increases in the relative abundance of Clostridium ss1, a large cluster of Clostridium species that includes both commensal and pathogenic species.Citation39 Members of Clostridium ss1 vary in their response to fermentation substrates, but exhibit a consistent capacity to synthesise butyrate.Citation40,Citation41 Clostridial abundance in CF faeces has been the focus of previous investigations, due to high rates of asymptomatic carriage of hypervirulent toxigenic strains of Clostridium difficile (Clostridium cluster XIa).Citation42 The increased abundance of members of the Clostridium genus observed here was not unexpected and might reflect their ability to form spores that are highly resilient to antibiotics. Indeed, reports of increasing C. difficile carriage ratesCitation43–Citation47 possibly reflect increases in cumulative antibiotic exposure that accompany improved CF patient longevity.Citation48 In contrast, commensal members of the Clostridiales order that are not capable of sporulation, such as the Faecalibacterium and Ruminococcus genera, showed reduced relative abundance compared to healthy controls. Clostridium ss1 exhibited a trend towards negative correlation with butyrate production in healthy controls, in whom butyrogenesis was primarily associated with Faecalibacterium. These findings suggest a competitive relationship between Clostridium ss1 and Faecalibacterium in the fermentation of HAMS. In the absence of Faecalibacterium in CF patients, Clostridium ss1, where present, is able to exploit HAMS as a fermentation source, resulting in high levels of butyrate production.
Propionate levels following in vitro fermentation were not significantly lower in CF patients compared to healthy controls. However, the bacterial taxa that were associated with this process were again different. Rather than members of the genus Bacteroides, which were strongly associated with propionate levels in control reactions, propionate biosynthesis in CF reactions were associated with the relative abundance of members of the genus Veillonella. While most Veillonella species are unable to ferment carbohydrates, they can produce propionate by fermenting succinate produced by other taxa.Citation49 The replacement of Bacteroides by Veillonella in the CF microbiota might be due, in part, to the acquisition of antibiotic resistance by members of the Veillonella genus.Citation49–Citation51
In contrast to butyrate and propionate, post-fermentation levels of acetate were significantly reduced in CF reactions compared with healthy controls. Acetate can be synthesised by a number of enteric bacteriaCitation52 mainly via the CoA-transferase pathway, involving the combined activities of phosphotransacetylase (PTA) and acetate kinase (AckA).Citation53 Acetate production in healthy controls was positively correlated with the relative abundance of Anaerostipes and Dorea genera; taxa that were substantially less prevalent in post-fermentation CF microbiota. The combination of reduced levels of genes encoding acetate biosynthesis, and a low prevalence of taxa that are able to metabolise acetate (e.g. Faecalibacterium, Roseburia and Coprococcus), suggests that acetate levels in CF reactions reflected reduced production, rather than increased bacterial utilisation.
To further understand the fermentation capacity of CF faecal microbiota, we compared the post-fermentation metabolome CF and healthy control reactions. The lower levels of acetate, and its intermediate formate, in CF reactions support our findings based on GC-FID results that CF has reduced capacity to produce acetate. Other metabolomic differences relating to non-starch components of the growth medium differed between CF and control reactions, including a reduced synthesis of trimethylamine and tyramine, and reduced utilisation of cystine and glycine, although these metabolites were observed in low abundance.
The failure of a subset of CF samples (CF low-R) to produce appreciable levels of SCFA was strongly associated with high Enterococcus relative abundance in post-fermentation microbiota. Enterococcal overgrowth is a common consequence of antibiotic exposure, in both animal modelsCitation54,Citation55 and humans.Citation56 Enterococci are capable of lactate biosynthesis and their high relative abundance was strongly associated with high lactate concentrations. Lactate can be further converted to acetate, butyrate and propionate by lactate-utilising bacteria.Citation57,Citation58 While this process does not typically occur at low pH (< 5.2)Citation57, post-fermentation pH did not differ significantly between CF low-R and high-R samples (data not shown). These findings suggest that lactate accumulation, observed in CF low-R reactions, where Enterococcus was predominant, resulted from reduced abundance of lactate-utilising taxa.
The implications of potential lactate accumulation on host physiology remain unclear. Luminal lactate has been shown to influence dendritic differentiation,Citation59 stimulate enterocyte hyper-proliferation in starvation-refed mice,Citation60 and to modulate LPS-dependent monocyte activation in vitro.Citation61,Citation62 Clinically, high faecal lactate concentrations have been reported in ulcerative colitisCitation63 and in chronic fatigue syndrome, along with an increased abundance of Enterococcus faecalis.Citation64 Our metagenomic analysis indicated that high enterococcal abundance was also associated with the presence of genes capable of raffinose metabolism. Raffinose is a trisaccharide composed of galactose, glucose, and fructose, which is part of the FODMAP group of oligosaccharides.Citation65 Raffinose, is particularly abundant in leguminous seeds,Citation66 and the ability to degrade raffinose as a source of glucose might therefore provide an additional benefit in Enterococcus-dominated dysbiotic CF microbiota.
While our analyses suggest that HAMS supplementation in patients with CF would result in increased microbial production of SCFAs or lactate, it is important to also consider potential deleterious changes in intestinal microbiology. Increases in the relative abundance of Clostridium ss1 might include potential pathogens, such as C. perfingens,, while the Enterococcus genus contains species that are capable of causing serious infections, such as E. faecium. Asymptomatic carriage of toxigenic C. difficile is common in patients with CF and was detected in two of the CF samples analysed in this study, although the relative abundance of this pathogen did not increase in during HAMS fermentation (assessed by qPCR, data not shown). However, the potential for HAMS to confer a selective advantage on potential pathogens suggests dietary supplementation in CF patients would require careful microbiological monitoring.
Where prebiotic strategies fall short of their desired clinical impact, the low abundance of taxa that are able to exploit them might be a contributory factor. Interest in synbiotic approaches (preparations that combine prebiotics with viable commensal bacteria) therefore continues to grow. However, we found that the introduction of microbiota from healthy control samples had little impact on the microbiota composition or fermentation productivity of CF slurries. How Clostridium ss1 or Enterococcus are able to remain numerically predominant during starch fermentation, even in the presence of genera such as Faecalibacterium and Roseburia, remains unclear. However, the out-competition of other bacteria during a rapid growth phase, combined with an ability to produce inhibitory compounds, such as bacteriocins,Citation67 might be contributory factors.
Our study had a number of limitations. We assessed stool, a sample that, while convenient, only approximates the intestinal microbiota. Furthermore, in vitro fermentation represents only a broad approximation of conditions within the colon.Citation68 Despite these limitations, we demonstrate that the highly dysbiotic CF gut microbiota retains the ability to exploit HAMS as a growth substrate, and a basis for SCFA production, in many cases. This ability is conferred by bacteria whose prevalence results from CF-associated changes in in the faecal microbiota . Manipulation of intestinal microbiota composition and function using dietary prebiotic supplementation offers exciting opportunities to achieve clinical benefit in CF. However, enterococcal overgrowth and lactate accumulation in microbiota from a subset of CF patients in response to HAMS suggests that a precision approach to clinical deployment would be most appropriate.
Conclusions
Despite significant alterations in composition, the adult CF gut microbiota retains the capacity to generate health-associated metabolites through HAMS fermentation. These findings suggest that the clinical potential of HAMS supplementation in adult CF patients warrants investigation. Our study also highlight that prebiotic effects can be mediated by taxa that are characteristic of disrupted microbial systems, despite ongoing perturbation, a finding that is contrary to the common assumptions that the persistence of commensal fermentative clades is necessary for such an effect. Finally, this study shows the potential for prebiotic effects to differ significantly depending on an individual’s starting gut microbiota and underlines importance of personalised approaches to their deployment.
Materials and methods
Study population
This study was approved by Mater Hospital Ethics Board (HREC/15/MHS/131). Adult CF patients were recruited from the Mater Adult Cystic Fibrosis Clinic, Brisbane. Healthy non-CF adult controls were recruited from Mater Hospital staff and associates. Subject characteristics are presented in . Stool samples were frozen and transported to the South Australian Health and Medical Research Institute, Adelaide, for analysis.
Table 1. Study population characteristics.
In vitro fermentation
To simulate gastric and small intestinal starch digestion, HAMS (Hylon VII, Ingredion ANZ, Pty Ltd) was subjected to in vitro pre-digestion (detailed in Supplementary Methods). Pre-digested HAMS was air-dried and UV-sterilised. The in vitro fermentation procedure was developed based on previously described methodCitation69 with modifications. In brief, a basal medium (pH 6.8) containing tryptone (2.5g/L), yeast extract (0.5g/L), and mineral salts (detailed in Supplementary Methods) adapted from McSweeney et alCitation70 was used as fermentation medium. The medium was boiled and cooled under anaerobic conditions, prior to the addition of cysteine HCl. Fermentation medium (10 mL) was transferred to Hungate tubes (16 x 125 mm) under anaerobic conditions (10% CO2 and 10% H2 in N2), which were stoppered and autoclaved. Faecal sample and pre-digested HAMS were dissolved in anaerobic diluent (pH 6.8)Citation70 to create a slurry. Faecal slurry (10% w/v) containing with 100 mg of faeces and HAMS slurry (20% w/v) containing 100 mg of HAMS were injected to fermentation tubes under anaerobic conditions. Control fermentation tubes contained 500 µl of anaerobic diluent, in place of HAMS slurry. Hungate tubes were incubated at 37°C for 24 h with shaking. Fermentation medium and anaerobic diluent compositions are detailed in Supplementary Methods.
DNA extraction and 16S rRNA gene amplicon sequencing
DNA extractions were performed using a DNeasy PowerSoil HTP 96 DNA Isolation kit (Qiagen), as described previously.Citation71 DNA concentration was quantified fluorometrically with a Quant-iT dsDNA Assay kit (Life Technologies). Amplicon sequencing of the V4 hypervariable region of the bacterial 16S rRNA gene was performed as described previouslyCitation71 using an Illumina MiSeq platform. Paired-end 16S rRNA gene sequence reads were analysed with the Quantitative Insights into Microbial Ecology (QIIME) software (v1.8.0)Citation72 following a previously published study.Citation71 Operational taxonomic units (OTUs) were assigned to the reads using an open reference approach with UCLUST algorithm against the SILVA reference dataset (release 123)Citation73 that clustered at 97% identity. 16S rRNA gene sequence data and Metagenomic sequence data were submitted to the Sequence Read Archive under accession number SRP136140 and SRP136140, respectively. Negative controls were included in all DNA extraction, PCR amplification, and DNA sequencing processes.
Metagenomic sequencing
Metagenomic libraries were fragmented and indexed using Nextera XT DNA Library Prep Kit (Illumina Inc.), and Nextera XT Index kit (Illumina Inc.), respectively as per manufacturer’s instructions. Amplicon libraries were then sequenced on the Illumina HiSeq 2500 platform at the SAHMRI David R Gunn Genomics Suite using Illumina HiSeq SBS 2 x 125bp v4 kit (Illumina Inc.). The sequencing resulted in an average of 18,586,781 ± 4,793,413 reads per sample. Bioinformatic processing of shotgun metagenomic sequence data was performed as described previouslyCitation74, with minor modifications (Supplementary Methods). Relative gene abundances were estimated by dividing the number of the gene-length normalized read counts for each gene by the total of reads from that sample that uniquely mapped to any gene in the catalogue. For analysis of functional capacity, genes annotated against the KEGG database were collapsed row-wise, based on their KEGG orthology identifiers, and summed for each pathway.
SCFA measurement
Pre- and post-fermentation samples were centrifuged at 13,000 g, 4 °C, for 10 min and supernatants collected for SCFA analysis. SCFA concentrations were measured by gas chromatography fitted with a flame ionisation detector (GC-FID, Flinders Analytical, Adelaide) using 4-Methylvaleric acid (Sigma-Aldrich) as internal standards.
Metabolomic analysis
Supernatants obtained from the in vitro fermentation were used for metabolomic analysis. Prior to the analysis, supernatants were frozen at −80°C, lyophilized at −58°C, and resuspended in 600 μl D2O. Subsequently, 1H NMR spectra were acquired under automation at 298 K and 700 MHz on a Bruker Avance II 700 NMR spectrometer (Bruker BioSpin) equipped with a 5-mm helium-cooled quadruple resonance cryoprobe and a cooled SampleJet sample changer.
Statistical analysis
Differences in alpha-diversity, SCFA, specific taxa, genes and metabolites between Control and CF groups were assessed using Mann-Whitney test (GraphPad Prism version 7.00). Group differences in microbiota beta diversity were assessed using the permutational analysis of variance (PERMANOVA) model with 9999 permutations based on the parameters permutation of residuals under a reduced model and a type III sum of squares (Primer-E v.7; Primer-E Ltd.).Citation75 Differences in taxa at the species level, generated from shotgun sequencing between groups at baseline, were analysed by linear discriminant analysis (LDA) effect size (LEfSe) method.Citation76 Correlation between SCFA concentrations and taxa were performed using Spearman non-parametric correlation (GraphPad Prism). Benjamini-Hochberg false discovery rate control (BH-FDR), with a threshold of 0.05, was applied to analysis involving multi-testing. Differences in the metagenomics gene between groups were determined using Limma package in RCitation77, followed by pathway enrichment using MinPath.Citation78 Several criteria were applied; 1) a gene has to be detected in at least 60% of the samples, 2) a gene has to be significantly different between CF and healthy (P ≤ 0.05, with −2 ≤ Log[Fold Change] ≥ 2), and 3) MiniPath-enriched pathways consisted of more than two genes. For metabolomic data, shifts of metabolites during the fermentation were presented in volcano plots with the indication of significantly changed metabolites determined by Mann-Whitney test adjusted with BH-FDR. Impacted pathways were analysed using pathway analysis by MetaboAnalyst 3..0Citation79
Disclosure of Potential Conflicts of Interest
No potential conflicts of interest were disclosed.
Supplemental Material
Download PDF (810.6 KB)Acknowledgments
We thank Dr R.A Atkinson (KCL Centre for Biomolecular Spectroscopy) for his assistance with NMR experiments.
Supplementary material
Supplemental data for this article can be accessed on the publisher’s website.
Additional information
Funding
References
- Chmiel JF, Davis PB. State of the art: why do the lungs of patients with cystic fibrosis become infected and why can’t they clear the infection? Respir Res. 2003;4:8. doi:10.1186/1465-9921-4-8.
- Steel A, Westaby D. Gastrointestinal disease in cystic fibrosis. In: Bush A, Bilton D, Hodson M, editors. Hodson and Geddes’ cystic fibrosis. London: CRC Press; 2016. p. 302–317.
- Ooi CY, Lemberg DA, Day AS. Other causes of gastritis. Pediatric Gastrointestinal Disease: B.C. Decker Inc. 2008:165–174.
- Freeman AJ, Ooi CY. Pancreatitis and pancreatic cystosis in Cystic Fibrosis. J Cyst Fibros. 2017;16(Suppl 2):S79–S86. doi:10.1016/j.jcf.2017.07.004.
- Smyth RL, Croft NM, O’Hea U, Marshall TG, Ferguson A. Intestinal inflammation in cystic fibrosis. Arch Dis Child. 2000;82:394–399.
- Choi Y, Kim JW, Nam KH, Han SH, Kim JW, Ahn SH, Park DJ, Lee K-W, Lee HS, Kim -H-H. Systemic inflammation is associated with the density of immune cells in the tumor microenvironment of gastric cancer. Gastric Cancer. 2017;20:602–611. doi:10.1007/s10120-016-0642-0.
- Neglia JP, FitzSimmons SC, Maisonneuve P, Schoni MH, Schoni-Affolter F, Corey M, Lowenfels AB. The risk of cancer among patients with cystic fibrosis. Cystic Fibrosis and Cancer Study Group. N Engl J Med. 1995;332:494–499.
- Maisonneuve P, Marshall BC, Lowenfels AB. Risk of pancreatic cancer in patients with cystic fibrosis. Gut. 2007;56:1327–1328. doi:10.1136/gut.2007.125278.
- Schoni MH, Maisonneuve P, Schoni-Affolter F, Lowenfels AB. Cancer risk in patients with cystic fibrosis: the European data. CF/CSG Group. J R Soc Med. 1996;89(Suppl 27):38–43.
- De Rose V, Oliva A, Messore B, Grosso B, Mollar C, Pozzi E. Circulating adhesion molecules in cystic fibrosis. Am J Respir Crit Care Med. 1998;157:1234–1239. doi:10.1164/ajrccm.157.4.9704134.
- Duytschaever G, Huys G, Bekaert M, Boulanger L, De Boeck K, Vandamme P. Dysbiosis of bifidobacteria and Clostridium cluster XIVa in the cystic fibrosis fecal microbiota. J Cyst Fibros. 2013;12:206–215. doi:10.1016/j.jcf.2012.10.003.
- Scanlan PD, Buckling A, Kong W, Wild Y, Lynch SV, Harrison F. Gut dysbiosis in cystic fibrosis. J Cyst Fibros. 2012;11:454–455. doi:10.1016/j.jcf.2012.03.007.
- Hoffman LR, Pope CE, Hayden HS, Heltshe S, Levy R, McNamara S, Jacobs MA, Rohmer L, Radey M, Ramsey BW, et al. Escherichia coli dysbiosis correlates with gastrointestinal dysfunction in children with cystic fibrosis. Clin Infect Dis. 2014;58:396–399. doi:10.1093/cid/cit715.
- Manor O, Levy R, Pope CE, Hayden HS, Brittnacher MJ, Carr R, Radey MC, Hager KR, Heltshe SL, Ramsey BW, et al. Metagenomic evidence for taxonomic dysbiosis and functional imbalance in the gastrointestinal tracts of children with cystic fibrosis. Sci Rep. 2016;6:22493. doi:10.1038/srep22493.
- Duytschaever G, Huys G, Bekaert M, Boulanger L, De Boeck K, Vandamme P. Cross-sectional and longitudinal comparisons of the predominant fecal microbiota compositions of a group of pediatric patients with cystic fibrosis and their healthy siblings. Appl Environ Microbiol. 2011;77:8015–8024. doi:10.1128/AEM.05933-11.
- Schippa S, Iebba V, Santangelo F, Gagliardi A, De Biase RV, Stamato A, Bertasi S, Lucarelli M, Conte MP, Quattrucci S, et al. Cystic fibrosis transmembrane conductance regulator (CFTR) allelic variants relate to shifts in faecal microbiota of cystic fibrosis patients. PLoS One. 2013;8:e61176. doi:10.1371/journal.pone.0061176.
- Burke DG, Fouhy F, Harrison MJ, Rea MC, Cotter PD, O’Sullivan O, Stanton C, Hill C, Shanahan F, Plant BJ, et al. The altered gut microbiota in adults with cystic fibrosis. BMC Microbiol. 2017;17:58. doi:10.1186/s12866-017-0968-8.
- Nielsen S, Needham B, Leach ST, Day AS, Jaffe A, Thomas T, Ooi CY. Disrupted progression of the intestinal microbiota with age in children with cystic fibrosis. Sci Rep. 2016;6:24857. doi:10.1038/srep24857.
- Rakoff-Nahoum S, Paglino J, Eslami-Varzaneh F, Edberg S, Medzhitov R. Recognition of commensal microflora by toll-like receptors is required for intestinal homeostasis. Cell. 2004;118:229–241. doi:10.1016/j.cell.2004.07.002.
- Murphy EA, Velazquez KT, Herbert KM. Influence of high-fat diet on gut microbiota: a driving force for chronic disease risk. Curr Opin Clin Nutr Metab Care. 2015;18:515–520. doi:10.1097/MCO.0000000000000209.
- Machiels K, Joossens M, Sabino J, De Preter V, Arijs I, Eeckhaut V, Ballet V, Claes K, Van Immerseel F, Verbeke K, et al. A decrease of the butyrate-producing species Roseburia hominis and Faecalibacterium prausnitzii defines dysbiosis in patients with ulcerative colitis. Gut. 2014;63:1275–1283. doi:10.1136/gutjnl-2013-304833.
- Carding S, Verbeke K, Vipond DT, Corfe BM, Owen LJ. Dysbiosis of the gut microbiota in disease. Microb Ecol Health Dis. 2015;26:26191.
- Arpaia N, Campbell C, Fan X, Dikiy S, van der Veeken J, deRoos P, Liu H, Cross JR, Pfeffer K, Coffer PJ, et al. Metabolites produced by commensal bacteria promote peripheral regulatory T-cell generation. Nature. 2013;504:451–455. doi:10.1038/nature12726.
- Chang PV, Hao L, Offermanns S, Medzhitov R. The microbial metabolite butyrate regulates intestinal macrophage function via histone deacetylase inhibition. Proc Natl Acad Sci U S A. 2014;111:2247–2252. doi:10.1073/pnas.1322269111.
- Saemann MD, Bohmig GA, Osterreicher CH, Burtscher H, Parolini O, Diakos C, Stöckl J, Hörl WH, Zlabinger GJ. Anti-inflammatory effects of sodium butyrate on human monocytes: potent inhibition of IL-12 and up-regulation of IL-10 production. FASEB J. 2000;14:2380–2382. doi:10.1096/fj.00-0359fje.
- Freeland KR, Wolever TMS. Acute effects of intravenous and rectal acetate on glucagon-like peptide-1, peptide YY, ghrelin, adiponectin and tumour necrosis factor-alpha. Br J Nutr. 2010;103:460–466. doi:10.1017/S0007114509991863.
- Higgins JA. Resistant starch: metabolic effects and potential health benefits. J AOAC Int. 2004;87:761–768.
- Alvaro A, Sola R, Rosales R, Ribalta J, Anguera A, Masana L, Vallvé JC. Gene expression analysis of a human enterocyte cell line reveals downregulation of cholesterol biosynthesis in response to short-chain fatty acids. IUBMB Life. 2008;60:757–764. doi:10.1002/iub.110.
- Wang HB, Wang PY, Wang X, Wan YL, Liu YC. Butyrate enhances intestinal epithelial barrier function via up-regulation of tight junction protein Claudin-1 transcription. Dig Dis Sci. 2012;57:3126–3135. doi:10.1007/s10620-012-2259-4.
- Ruppin H, Bar-Meir S, Soergel KH, Wood CM, Schmitt MG Jr. Absorption of short-chain fatty acids by the colon. Gastroenterology. 1980;78:1500–1507.
- Birt DF, Boylston T, Hendrich S, Jane JL, Hollis J, Li L, McClelland J, Moore S, Phillips GJ, Rowling M, et al. Resistant starch: promise for improving human health. Adv Nutr. 2013;4:587–601. doi:10.3945/an.113.004325.
- Walker AW, Ince J, Duncan SH, Webster LM, Holtrop G, Ze X, Brown D, Stares MD, Scott P, Bergerat A, et al. Dominant and diet-responsive groups of bacteria within the human colonic microbiota. Isme J. 2011;5:220–230. doi:10.1038/ismej.2010.118.
- Phillips J, Muir JG, Birkett A, Lu ZX, Jones GP, O’Dea K, Young GP. Effect of resistant starch on fecal bulk and fermentation-dependent events in humans. Am J Clin Nutr. 1995;62:121–130. doi:10.1093/ajcn/62.1.121.
- Le Leu RK, Winter JM, Christophersen CT, Young GP, Humphreys KJ, Hu Y, Gratz SW, Miller RB, Topping DL, Bird AR, et al. Butyrylated starch intake can prevent red meat-induced O6-methyl-2-deoxyguanosine adducts in human rectal tissue: a randomised clinical trial. Br J Nutr. 2015;114:220–230. doi:10.1017/S0007114515001750.
- Aliasgharzadeh A, Dehghan P, Gargari BP, Asghari-Jafarabadi M. Resistant dextrin, as a prebiotic, improves insulin resistance and inflammation in women with type 2 diabetes: a randomised controlled clinical trial. Br J Nutr. 2015;113:321–330. doi:10.1017/S0007114514003675.
- McLoughlin RF, Berthon BS, Jensen ME, Baines KJ, Wood LG. Short-chain fatty acids, prebiotics, synbiotics, and systemic inflammation: a systematic review and meta-analysis. Am J Clin Nutr. 2017;106:930–945. doi:10.3945/ajcn.117.156265.
- Louis P, Young P, Holtrop G, Flint HJ. Diversity of human colonic butyrate-producing bacteria revealed by analysis of the butyryl-CoA: acetateCoA-transferase gene. Environ Microbiol. 2010;12:304–314. doi:10.1111/j.1462-2920.2009.02066.x.
- Ze X, Duncan SH, Louis P, Flint HJ. Ruminococcus bromii is a keystone species for the degradation of resistant starch in the human colon. Isme J. 2012;6:1535–1543. doi:10.1038/ismej.2012.4.
- Gupta RS, Gao B. Phylogenomic analyses of clostridia and identification of novel protein signatures that are specific to the genus Clostridium sensu stricto (cluster I). Int J Syst Evol Microbiol. 2009;59:285–294. doi:10.1099/ijs.0.001792-0.
- Rainey FA, Hollen BJ, Small A. Genus I. Clostridium Prazmowski 1880, 23AL. In: Vos P, Garrity G, Jones D, editors. Bergey’s manual of systematic bacteriology volume 3: the Firmicutes. New York: Spring; 2009. p. 738–828.
- Chen CK, Blaschek HP. Effect of acetate on molecular and physiological aspects of Clostridium beijerinckii NCIMB 8052 solvent production and strain degeneration. Appl Environ Microbiol. 1999;65:499–505.
- Burke DG, Harrison MJ, Fleming C, McCarthy M, Shortt C, Sulaiman I, Murphy DM, Eustace JA, Shanahan F, Hill C, et al. Clostridium difficile carriage in adult cystic fibrosis (CF); implications for patients with CF and the potential for transmission of nosocomial infection. J Cyst Fibros. 2017;16:291–298. doi:10.1016/j.jcf.2016.09.008.
- Wu TC, Fung JC. Evaluation of the usefulness of counterimmunoelectrophoresis for diagnosis of Clostridium difficile-associated colitis in clinical specimens. J Clin Microbiol. 1983;17:610–613.
- Welkon CJ, Long SS, Thompson CM Jr., Gilligan PH. Clostridium difficile in patients with cystic fibrosis. Am J Dis Child. 1985;139:805–808.
- Peach SL, Borriello SP, Gaya H, Barclay FE, Welch AR. Asymptomatic carriage of Clostridium difficile in patients with cystic fibrosis. J Clin Pathol. 1986;39:1013–1018.
- Yahav J, Samra Z, Blau H, Dinari G, Chodick G, Shmuely H. Helicobacter pylori and Clostridium difficile in cystic fibrosis patients. Dig Dis Sci. 2006;51:2274–2279. doi:10.1007/s10620-006-9271-5.
- Pokorny CS, Bye PT, MacLeod C, Selby WS. Antibiotic-associated colitis and cystic fibrosis. Dig Dis Sci. 1992;37:1464–1468.
- Hurley MN, McKeever TM, Prayle AP, Fogarty AW, Smyth AR. Rate of improvement of CF life expectancy exceeds that of general population–observational death registration study. J Cyst Fibros. 2014;13:410–415. doi:10.1016/j.jcf.2013.12.002.
- Rainey FA. Family X. Veillonellaceae Rogosa 1971b, 232AL. In: Vos P, Garrity G, Jones D, editors. Bergey’s manual of systematic bacteriology volume 3: theFirmicutes. New York: Spring; 2009. p. 1059–1129.
- Ready D, Pratten J, Roberts AP, Bedi R, Mullany P, Wilson M. Potential role of Veillonella spp. as a reservoir of transferable tetracycline resistance in the oral cavity. Antimicrob Agents Chemother. 2006;50:2866–2868. doi:10.1128/AAC.00217-06.
- Worlitzsch D, Rintelen C, Bohm K, Wollschlager B, Merkel N, Borneff-Lipp M, Döring G. Antibiotic-resistant obligate anaerobes during exacerbations of cystic fibrosis patients. Clin Microbiol Infect. 2009;15:454–460. doi:10.1111/j.1469-0691.2008.02659.x.
- Flint HJ, Duncan SH, Scott KP, Louis P. Links between diet, gut microbiota composition and gut metabolism. Proc Nutr Soc. 2015;74:13–22. doi:10.1017/S0029665114001463.
- Ferry JG. Acetate kinase and phosphotransacetylase. Methods Enzymol. 2011;494:219–231. doi:10.1016/B978-0-12-385112-3.00011-1.
- Ubeda C, Taur Y, Jenq RR, Equinda MJ, Son T, Samstein M, Viale A, Socci ND, van Den Brink MRM, Kamboj M, et al. Vancomycin-resistant Enterococcus domination of intestinal microbiota is enabled by antibiotic treatment in mice and precedes bloodstream invasion in humans. J Clin Invest. 2010;120:4332–4341. doi:10.1172/JCI43918.
- Hendrickx AP, Top J, Bayjanov JR, Kemperman H, Rogers MR, Paganelli FL, Bonten MJM, Willems RJL. Antibiotic-Driven dysbiosis mediates intraluminal agglutination and alternative segregation of enterococcus faecium from the intestinal epithelium. MBio. 2015;6:e01346–15. doi:10.1128/mBio.01346-15.
- Hufnagel M, Liese C, Loescher C, Kunze M, Proempeler H, Berner R, Krueger M. Enterococcal colonization of infants in a neonatal intensive care unit: associated predictors, risk factors and seasonal patterns. BMC Infect Dis. 2007;7:107. doi:10.1186/1471-2334-7-89.
- Belenguer A, Duncan SH, Holtrop G, Anderson SE, Lobley GE, Flint HJ. Impact of pH on lactate formation and utilization by human fecal microbial communities. Appl Environ Microbiol. 2007;73:6526–6533. doi:10.1128/AEM.00508-07.
- Bourriaud C, Robins RJ, Martin L, Kozlowski F, Tenailleau E, Cherbut C, Michel C. Lactate is mainly fermented to butyrate by human intestinal microfloras but inter-individual variation is evident. J Appl Microbiol. 2005;99:201–212. doi:10.1111/j.1365-2672.2005.02605.x.
- Gottfried E, Kunz-Schughart LA, Ebner S, Mueller-Klieser W, Hoves S, Andreesen R, Mackensen A, Kreutz M. Tumor-derived lactic acid modulates dendritic cell activation and antigen expression. Blood. 2006;107:2013–2021. doi:10.1182/blood-2005-05-1795.
- Okada T, Fukuda S, Hase K, Nishiumi S, Izumi Y, Yoshida M, Hagiwara T, Kawashima R, Yamazaki M, Oshio T, et al. Microbiota-derived lactate accelerates colon epithelial cell turnover in starvation-refed mice. Nat Commun. 2013;4:1654. doi:10.1038/ncomms2668.
- Dietl K, Renner K, Dettmer K, Timischl B, Eberhart K, Dorn C, Hellerbrand C, Kastenberger M, Kunz-Schughart LA, Oefner PJ, et al. Lactic acid and acidification inhibit TNF secretion and glycolysis of human monocytes. J Immunol. 2010;184:1200–1209. doi:10.4049/jimmunol.0902584.
- Peter K, Rehli M, Singer K, Renner-Sattler K, Kreutz M. Lactic acid delays the inflammatory response of human monocytes. Biochem Biophys Res Commun. 2015;457:412–418. doi:10.1016/j.bbrc.2015.01.005.
- Vernia P, Caprilli R, Latella G, Barbetti F, Magliocca FM, Cittadini M. Fecal lactate and ulcerative colitis. Gastroenterology. 1988;95:1564–1568.
- Sheedy JR, Wettenhall RE, Scanlon D, Gooley PR, Lewis DP, McGregor N, Stapleton DI, Butt HL, DE Meirleir KL. Increased d-lactic Acid intestinal bacteria in patients with chronic fatigue syndrome. In Vivo. 2009;23:621–628.
- Gibson PR, Shepherd SJ. Personal view: food for thought–western lifestyle and susceptibility to Crohn’s disease. The FODMAP Hypothesis. Aliment Pharmacol Ther. 2005;21:1399–1409. doi:10.1111/j.1365-2036.2005.02506.x.
- Tester RF, Karkalas J. Carbohydrates: classification and properties. In: Trugo L, Finglas PM, editors. Encyclopedia of food sciences and nutrition. Cambridge (MA): Academic Press; 2003. p. 862–875.
- Ness IF, Diep DB, Ike Y. Enterococcal bacteriocins and antimicrobial proteins that contribute to niche control. In: Gilmore MS, Clewell DB, Ike Y, Shankar N, editors. Enterococci: from commensals to leading causes of drug resistant infection. Boston: Massachusetts Eye and Ear Infirmary; 2014.
- Long W, Xue Z, Zhang Q, Feng Z, Bridgewater L, Wang L, Zhao L, Pang X. Differential responses of gut microbiota to the same prebiotic formula in oligotrophic and eutrophic batch fermentation systems. Sci Rep. 2015;5:13469. doi:10.1038/srep13469.
- Edwards CA, Gibson G, Champ M, Jensen BB, Mathers JC, Nagengast F, Rumney C, Quehl A. In vitro method for quantification of the fermentation of starch by human faecal bacteria. J Sci Food Agr. 1996;71:209–217. doi:10.1002/(ISSN)1097-0010.
- McSweeney CS, Denman SE, Mackie RI. Rumen bacteria. In: Makkar HPS, McSweeney CS, editors. Methods in gut microbial ecology for ruminants. Dordrecht: International Atomic Energy Agency; 2005. p. 23–37.
- Choo JM, Leong LE, Rogers GB. Sample storage conditions significantly influence faecal microbiome profiles. Sci Rep. 2015;5:16350. doi:10.1038/srep16350.
- Caporaso JG, Kuczynski J, Stombaugh J, Bittinger K, Bushman FD, Costello EK, Fierer N, Peña AG, Goodrich JK, Gordon JI, et al. QIIME allows analysis of high-throughput community sequencing data. Nat Methods. 2010;7:335–336. doi:10.1038/nmeth.f.303.
- Quast C, Pruesse E, Yilmaz P, Gerken J, Schweer T, Yarza P, Peplies J, Glöckner FO. The SILVA ribosomal RNA gene database project: improved data processing and web-based tools. Nucleic Acids Res. 2013;41:D590–D6. doi:10.1093/nar/gks1219.
- Nielsen HB, Almeida M, Juncker AS, Rasmussen S, Li J, Sunagawa S, Plichta DR, Gautier L, Pedersen AG, Le Chatelier E, et al. Identification and assembly of genomes and genetic elements in complex metagenomic samples without using reference genomes. Nat Biotechnol. 2014;32:822–828. doi:10.1038/nbt.2939.
- Anderson MJ, Walsh DCI. PERMANOVA, ANOSIM, and the Mantel test in the face of heterogeneous dispersions: what null hypothesis are you testing? Ecol Monogr. 2013;83:557–574. doi:10.1890/12-2010.1.
- Segata N, Izard J, Waldron L, Gevers D, Miropolsky L, Garrett WS, Huttenhower C. Metagenomic biomarker discovery and explanation. Genome Biol. 2011;12:R60. doi:10.1186/gb-2011-12-10-r102.
- Ritchie ME, Phipson B, Wu D, Hu Y, Law CW, Shi W, Smyth GK. limma powers differential expression analyses for RNA-sequencing and microarray studies. Nucleic Acids Res. 2015;43:e47. doi:10.1093/nar/gkv007.
- Ye Y, Doak TG. A parsimony approach to biological pathway reconstruction/inference for genomes and metagenomes. PLoS Comput Biol. 2009;5:e1000465. doi:10.1371/journal.pcbi.1000509.
- Xia J, Wishart DS. Using MetaboAnalyst 3.0 for Comprehensive Metabolomics Data Analysis. Curr Protoc Bioinformatics. 2016;55:14 0 1–0 91. doi:10.1002/cpbi.11.