ABSTRACT
Alterations of gut microbiota have been implicated in multiple diseases including cancer. However, the gut microbiota spectrum in lung cancer remains largely unknown. Here we profiled the gut microbiota composition in a discovery cohort containing 42 early-stage lung cancer patients and 65 healthy individuals through the 16S ribosomal RNA (rRNA) gene sequencing analysis. We found that lung cancer patients displayed a significant shift of microbiota composition in contrast to the healthy populations. To identify the optimal microbiota signature for noninvasive diagnosis purpose, we took advantage of Support-Vector Machine (SVM) and found that the predictive model with 13 operational taxonomic unit (OTU)-based biomarkers achieved a high accuracy in lung cancer prediction (area under curve, AUC = 97.6%). This signature performed reasonably well in the validation cohort (AUC = 76.4%), which contained 34 lung cancer patients and 40 healthy individuals. To facilitate potential clinical practice, we further constructed a ‘patient discrimination index’ (PDI), which largely retained the prediction efficiency in both the discovery cohort (AUC = 92.4%) and the validation cohort (AUC = 67.7%). Together, our study uncovered the microbiota spectrum of lung cancer patients and established the specific gut microbial signature for the potential prediction of the early-stage lung cancer.
Introduction
Lung cancer is one of the most devastating diseases worldwideCitation1, which is composed of small-cell lung cancer (SCLC) and non-small cell lung cancer (NSCLC). NSCLC accounts for over 80% of lung cancer and is further divided into lung adenocarcinoma (ADC), squamous cell carcinoma (SCC) and large cell carcinoma (LCC).Citation2,Citation3 Most of the lung cancer patients are initially diagnosed at advanced disease stages, often accompanied by poor prognosis. Thus, early diagnosis of lung cancer would tremendously improve disease management and patient survival.Citation4 It is therefore clinically important to develop noninvasive biomarkers with high sensitivity and specificity.
The gut microbiota strongly influences metabolic, endocrine and immune systems, not only locally at the mucosal level but also systemically at the host level. Recent studies have demonstrated that the gut microbiota is in connection with multiple diseases, including autism spectrum disorder, stroke, nonalcoholic fatty liver disease, atopic dermatitis and type 2 diabetes.Citation5–Citation11 These studies suggest the valuable diagnostic potential of microbiota-derived signatures. Interestingly, recent studies uncover the potential association between gut microbiota and lung diseases such as asthma, chronic obstructive pulmonary disease (COPD) and respiratory infection.Citation12–Citation16 Microbiota-accessible carbohydrates can change the gut microbiota and increase short-chain fatty acid (SCFA) to shape the lung immunity.Citation17 SCFAs may stimulate regulatory T cell, Th2 cells and IL-22+ type 3 innate lymphoid cells in the lung to protect against airway inflammation via T cell receptor signaling.Citation18,Citation19 Moreover, the mouse model study also establishes the link between the microbiota-immune crosstalk and lung cancer development.Citation20 Population study shows that the increase of antibiotic usage correlates with the increase of lung cancer incidence.Citation21 Despite the extensive progression in linking gut microbiota with lung disease (‘gut-lung axis’),Citation22–Citation25 the lung cancer-related microbiota spectrum remains largely unknown.
Here we recruited a discovery cohort containing 42 early-stage lung cancer patients and 65 healthy individuals for 16S rRNA gene sequencing analysis of gut microbiota. We uncovered the microbial spectrum of lung cancer patients and identified the specific microbiome signature potentially useful for early-stage lung cancer prognosis.
Results
Gut microbial profile of early-stage lung cancer patients
To determine whether gut microbial changes were associated with early-stage lung cancer, we performed the 16S rRNA gene sequencing using fecal microbiome samples from a Chinese cohort containing 42 lung cancer patients and 65 healthy individuals. This cohort served as the ‘discovery cohort.’ To validate the findings from this cohort, we recruited another independent cohort of 34 patients and 40 controls (referred to as the ‘validation cohort’). None of these patients had gastrointestinal tract disorders or took antibiotics/probiotics within last 8 weeks. The age and body mass index (BMI) were comparable between the lung cancer group and healthy control group (). The male/female ratio was slightly low in healthy group in contrast to lung cancer group in the discovery cohort (10/55 versus 18/24), whereas this ratio was comparable in the validation cohort (22/18 versus 16/18) (Table S1). All the patients are NSCLC (37 ADC, 3 SCC and 2 LCC in the discovery cohort, 29 ADC, 4 SCC and 1 LCC in the validation cohort), consistent with the epidemiological characteristic of lung cancer in recent decades.Citation26
Table 1. Baseline characteristics of the discovery cohort.
Patients in the discovery cohort were at early stages, including stage 0 (adenocarcinoma in situ, AIS) (14.29%), stage I (76.19%) and stage II (9.52%). Most of these patients (92.86%) had non-metastatic lung cancer (). Through sequencing data analyses, approximately 6,000,000 sequences were annotated against the rRNA library database (Greengenes) and analyzed at the operational taxonomic unit (OTU) level (Table S2). The 97% identity of rRNA sequence was used as the cutoff to define the OTUs. We identified 1175 OTUs from the discovery cohort. Both lung cancer group and healthy control group showed comparable numbers of average OTUs (). Moreover, the richness and diversity of microbiota assessed by Shannon and Chao indices were also comparable (). These data indicated similar global community α-diversity between the lung cancer and healthy groups.
Figure 1. Gut microbiota dysbiosis in lung cancer patients. (a) Numbers of OTUs observed in patients and healthy controls. (b) Microbial richness based on the Chao index and diversity based on the Shannon index. The box represented the interquartile range (IQR) between the first and the third quartiles, and the midline represented the median. Whiskers extended to values within 1.5 times IQR. Circles indicated outliers beyond the whiskers. (c) Principal coordinates analysis (PCoA) for lung cancer (orange) and healthy control samples (green). Left, Unweighted UniFrac; right, Bray–Curtis. Significant differences were observed between patients and healthy controls with ANOSIM test (UniFrac, R = 0.0912, P = .003; Bray–Curtis, R = 0.0739, P = .007). The first two principal coordinates (PCs) were each labeled with the percentage of variance explained.
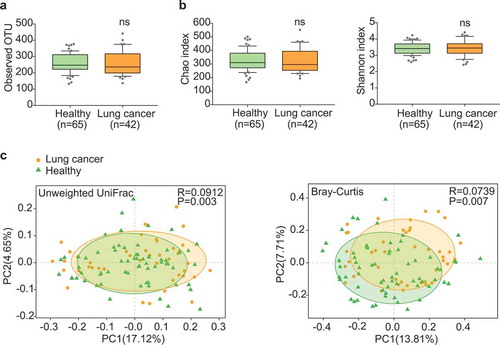
Detailed analyses uncovered certain unique microbial taxa in either lung cancer group or healthy group (Figure S1 and Table S3). A total of 97 OTUs, 18 species, 12 genera and 1 phylum were detectable only in the cancer group, whereas another 163 OTUs, 33 species and 22 genera were only in the healthy group. This indicated that the composition of gut microbiota may have changed during the development of lung cancer. Principle coordinates analysis (PCoA) and ANOSIM test revealed a significant global difference of microbiome composition and abundance (Unweighted UniFrac p = .003 and Bray–Curtis p = .007) ().
Specific gut microbial signature in lung cancer patients
We next examined the abundance of each phylum, genus and species detected in the discovery cohort. In total, 3 phyla, 13 genera, and 20 species showed significantly lower abundance among lung cancer patients (, File S1), whereas 4 phyla, 11 genera, and 15 species were conversely enriched (, File S1). Despite some low-abundant phyla characteristically present in the cancer group, several abundant phyla, such as Firmicutes, Bacteroidetes, Proteobacteria and Actinobacteria, also differed significantly between these two groups. Proteobacteria, which included many known pathogenic microorganisms,Citation27,Citation28 was found highly abundant in the cancer group. At the genus level, Ruminococcus, an uncharacterized genus of family Enterobacteriaceae, and an uncharacterized genus of family Lachnospiraceae were highly enriched in the cancer group (), whereas Faecalibacterium, Streptococcus, Bifidobacterium and Veillonella were significantly enriched in the healthy group (). More abundant species in the cancer group were primarily from the Bacteroides and Proteobacteria phyla. In contrast, species decreased significantly in the cancer group were mainly from the Firmicutes and Actinobacteria. Firmicutes and Actinobacteria are known to promote colonic luminal SCFA production and modulate inflammation and tumor formation in mice and humans.Citation29–Citation31 Moreover, reduced ratio of Firmicutes/Bacteroidetes in the lung cancer group may result in decreased circulating SCFA and thus influence host systemic immunity and inflammation.Citation32 These data indicate that lung cancer might be associated with the increase of pathogens and the decrease of certain probiotic microbes.
Figure 2. Differential abundance of gut microbiota in lung cancer and healthy controls. The taxa decreased (a) and increased (b) in patients with lung cancer at the phylum, genus, and species levels, p < .05. Green and orange represented the healthy controls (n = 65) and lung cancer patients (n = 42), respectively. The distributions of taxa were based on the number of reads post-filtering and rarefying. The abundance in each group was plotted as log10 scale on the y axis. P values were calculated using the two-tailed Wilcoxon rank-sum test. Description of boxplots was the same as in . Taxa were named as their lowest possible level of classification, with unclassified (unc) indicating no further classification available.
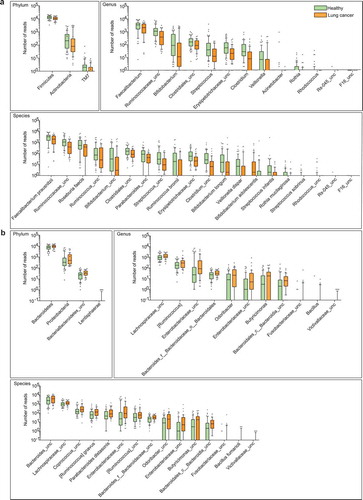
Gut microbial compositions correlate with tumor stages and subtypes
We next compared the microbial compositions according to tumor subtypes and metastatic status in the discovery cohort (). Several lung cancer-related microbes such as Blautia obeum, Akkermansia muciniphila, Lactobacillus salivarius and an uncharacterized genus of family Coriobacteriaceae increased only in the three metastatic patients (). No microbe was found to be specifically increased only in the non-metastatic patients when compared with the healthy controls and the metastatic patients. The three main subtypes of NSCLC (ADC, SCC, and LCC) seemed to have differential microbiota profiles (). It is worth noting that the numbers of SCC and LCC (three cases and two cases, respectively) were too small to draw a conclusive point. Future efforts are certainly necessary to recruit more patients for subtype-specific microbial studies.
Figure 3. Association between clinical variables and gut microbiota abundance. Heat map showing the relative abundances of the 50 most abundant species in samples grouped by metastatic status (a) and lung cancer subtypes (c), respectively. Species were in rows, and the relative abundance was indicated by color gradient. The most significantly changed species were further analyzed in each group (b, d). Description of boxplots was the same as in . Two-tailed Wilcoxon rank-sum test, *p < .05, **p < .01, ***p < .001.
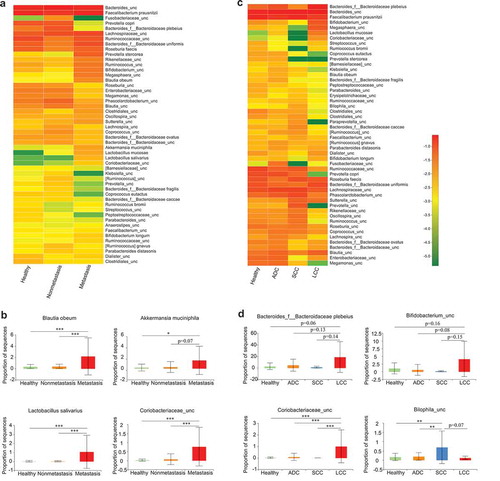
Altered pathways in gut microbial communities of lung cancer patients
We further performed the PICRUSt analysis to predict and test the difference of KEGG pathways between the cancer and healthy groups. Among the 328 metabolic pathways tested, we found 19 pathways increased and 12 decreased in the cancer group (Figure S2). The cancer group was enriched with pathways associated with cellular antigens, steroid biosynthesis, ubiquitin system, transcription-related proteins, bile secretion, and fatty acid elongation in mitochondria. In contrast, the pathways related to bacterial motility proteins, bacterial chemotaxis, flavone, and flavonol biosynthesis apoptosis and G protein-coupled receptors decreased in the cancer group. These data indicated the potentially interesting metabolic reprogramming in the gut microbiota during lung cancer development.
Identification of gut microbial signature for lung cancer prediction
It remains clinically important to develop noninvasive diagnosis biomarkers for early-stage lung cancer.Citation33 With the motivation of constructing a clinical index for early diagnosis using either sequencing or real-time quantitative PCR, we developed a reference-based strategy for biomarker development. For this, we first identified the top reference OTUs that were relatively abundant in both healthy (n = 65) and cancer (n = 42) groups (OTU1063 of Blautia_unc, OTU14 of Faecalibacterium prausnitzii, OTU163 of Bacteroides_unc, OTU312 of Blautia_unc). All other OTUs were then compared against these reference OTUs to determine their relative logarithmic changes (RLCs). Those OTUs with significantly different RLCs between cancer patients and healthy controls were further selected for the identification of candidate markers with the minimum-redundancy maximum-relevancy (mRMR) method. We used the top 30 OTU candidates to train an initial support-vector machine (SVM) model to predict disease status. We then employed a backward selection strategy to gradually narrow down the OTU list using 10-fold cross-validation for performance assessment. The prediction accuracy and true negative rate (TNR) were both considered during the optimization process. To control the error rate of predicting healthy individuals (false positive rate), we emphasized TNR minimization. Thirteen OTU-based markers were finally chosen (, File S2), which highly accurately predicted the disease status in the discovery cohort (AUC = 97.6%) (). To further evaluate whether these markers could be generalized to other samples, we used the validation cohort as an independent test and found a reasonably high predictive power (AUC = 76.4%), albeit lower than that in the original cohort.
Table 2. Thirteen OTU-based markers.
Figure 4. OTU-based diagnostic biomarkers for lung cancer. (a, b) Receiver operating characteristic (ROC) curves of prediction efficacy for the OTU-based predictors using SVM (a) and PDI (b), respectively. Solid and dashed lines indicated the discovery (patient = 42, healthy = 65) and validation (patient = 34, healthy = 40) cohorts, respectively. (c) PDI distributions in early-stage patients and healthy controls. Two-tailed Wilcoxon rank-sum test, **p < .01.
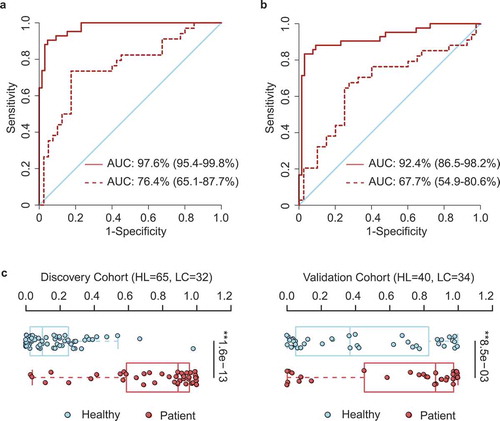
In order to facilitate more convenient diagnosis application, we further constructed a clinical index, the ‘patient discrimination index’ (PDI), which was a weighted score based on the logistic regression coefficients of those OTU markers. Notably, the PDI had a predictive power almost as high as the more complex SVM model, with AUC = 92.4% in the discovery cohort and AUC = 67.7% in the validation cohort (). Distribution of PDIs in the healthy individuals and patients also showed a clear diversification pattern (), indicating its effectiveness in distinguishing lung cancer from healthy individuals.
Discussion
We have demonstrated the characteristic changes of the gut microbiota in early-stage lung cancer patients and identified those microbial candidates that might contribute to lung cancer development. The genus Bifidobacterium and Faecalibacterium are more abundant in controls, whereas the lung cancer patients show the elevated Bacillus (). Members of Bifidobacterium have a variety of probiotic functions, and achieve anti-cancer effects and reduce the inflammation caused by TNF-⍺ and lipopolysaccharides (LPS).Citation34 TNF-⍺ can promote lung cancer metastasis by inducing epithelial–mesenchymal transition.Citation35 A major member of Faecalibacterium, Faecalibacterium prausnitzii, is associated with anti–inflammatory functions via inhibiting NF-κB activation and IL-8 secretion.Citation36 Bacillus spp. in lung sputum may be correlated with an increased lung cancer risk.Citation23
Beyond the simple description of gut microbiota changes in lung cancer patients,Citation37 our study proposes the idea of species-based and OTU-based predictors for early-stage lung cancer. We find that the OTU-based predictor is more accurate and reproducible, possibly due to the usage of detailed 16 S rRNA gene sequences. In the optimal 13 OTU-based microbial markers, several species have been reported to functionally impact upon the host. Roseburia spp. can affect immune maintenance and anti–inflammatory effects through a variety of metabolic pathways.Citation38,Citation39 Similarly, Ruminococcus bromii-related microbes are abundant in human and promote intestinal health by degrading starch and producing SCFAs.Citation40 Streptococcus infantis, significantly reduced in lung cancer patients (), is a microbe colonized in the respiratory tract and causally linked to the chronic lung disease via modulating pulmonary innate immunity with detoxifying polycyclic aromatic hydrocarbons (PAHs).Citation23 The genus Veillonella is associated with Th17-mediated immunity in the lungs.Citation41 Veillonella has been linked to COPD,Citation41 SCC and ADC.Citation42 It may be involved in tumor metastasis through VEGF-b-mediated processesCitation43 or the PI3K signaling pathway.Citation44 In addition to the above metabolic pathways, bile acids (BAs) can activate various nuclear receptors including TGR5, which is recently shown to associate with advanced clinical stages in NSCLC.Citation45 Moreover, we have found increased steroid biosynthesis, bile secretion, and carbohydrate metabolic pathways in gut microbiota of lung cancer patients (Figure S2). It seems possible that the lipid metabolites produced by gut microbiota interact with host lipid homeostasis and even get involved in the pathophysiological processes. However, the detailed link between the gut-lung axis and lung immunity or cancer development remains to be further clarified.
Several other species enriched in lung cancer patients are also reported in other diseases or infections, such as Bacteroides thetaiotaomicron. BLAST analysis of the sequence of Bacteroides_unc gave the maximum of identity (99.55%) with the 16S rRNA gene sequence of Bacteroides thetaiotaomicron. This microbe can infect tissues exposed to gut microbiota and increase the free sialic acid in the gut, which can contribute to the growth of pathogenic microbes such as Clostridum difficile and Escherichia coli.Citation46–Citation48
Different cancer types appear to have their own unique gut microbial characteristics.Citation49–Citation53 In our study, lung cancer patients also show a distinct microbial signature (), which is partially shared by other cancer types. For example, phyla Bacteroidetes also increases in colorectal cancer and breast cancer,Citation50,Citation53 whereas genera Bifidobacterium and Faecalibacterium prausnitzii similarly decrease in pancreatic cancer and colorectal cancer, respectively.Citation51,Citation53 Interestingly, Akkermansia muciniphila, which increases in lung cancer, is found to be altered in all the other four cancer types discussed here.Citation49–Citation53 Moreover, this microbe is further enriched in the patients with advanced-stage lung cancer (), indicating that it potentially contributes to lung cancer malignant progression.
We also recognize several limitations of our study. Although the 16S rRNA gene sequencing is widely applied for microbiota characterization, it has a limitation in uncovering the full genetic contents when compared to metagenomic sequencing. This method is also not very powerful in characterizing microbiota at the species level. Nonetheless, it is technically mature and affordable for large-scale study. Smoking is known to increase the lung cancer risk by altering the pulmonary microbial composition.Citation54 We find that smoking status has a significant impact upon certain microbes (Table S4), but three smokers could not be distinguished from the nonsmokers by the OTU markers (Figure S3). However, the fact of only three patients in our study cohort requires future recruitment of more smokers. Moreover, we observe the decrease of the predictive accuracy in the validation cohort. We reason this could be due to the high inter-sample and inter-cohort variability. Future enlargement of study cohort is expected to further improve the prediction power.
In conclusion, we characterize the systemic alterations of gut microbiota in lung cancer patients. We uncover the microbial signature associated with early-stage lung cancer and develop a highly accurate OTU-based predictor for early diagnosis of lung cancer.
Patients and Methods
Patient recruitment
All 76 fecal samples from lung cancer patients were collected in Shanghai Pulmonary Hospital, Tongji University School of Medicine. The 105 fecal samples from healthy volunteers were collected in The Tenth People’s Hospital Affiliated to Tongji University upon routine physical examination. Fecal samples were collected according to the approved protocol by the local ethics committees ahead of procedure of enrollment, and written informed consent was obtained from all patients and volunteers. Lung cancer was diagnosed according to the international guidelines by comprehensive consideration of lung biopsy, imaging examination, clinical symptoms, physical signs, laboratory tests, medical history, progress notes and cancer-associated comorbidities. All clinical information was collected according to standard procedures. Patients suffering from gastrointestinal tract disorders were excluded. Individuals who received antibiotics or probiotics within latest 8 weeks were excluded. As for healthy control samples, physical examination including routine examination of blood, urine, and feces, liver function, renal function, electrocardiogram, and chest X-ray results were used to exclude any unhealthy sample. Comprehensive clinical information for the enrolled participants, including age, gender, body mass index (BMI), tumor stage, tumor type, tumor metastasis, and smoking status are summarized in and Table S1. The study was conducted in accordance with the Declaration of Helsinki and Rules of Good Clinical Practice.
Sample collection, DNA extraction, and sequencing
Fecal samples freshly collected from each participant were immediately frozen and stored in −20°C freezer as described.Citation55 Total DNA was extracted using the QIAamp DNA Stool Mini Kit (Qiagen, Hilden, Germany) according to the manufacturer’s protocols. The extracted products were determined by agarose gel electrophoresis (1% w/v agarose). DNA quantification was measured using NanoDrop 2000 (Thermo Scientific, USA). The V3-V4 region of 16S rRNA gene was amplified with barcode-indexed primers 338F (5’-ACTCCTACGGGAGGCAGCA-3’) and 806R (5’-GGACTACHVGGGTWTCTAAT-3’), using TransStart FastPfu Polymerase. Amplicons were then purified by gel extraction (AxyPrep DNA GelExtraction Kit, Axygen Biosciences, Union City, CA, USA) and were quantified using QuantiFluor-ST (Promega, USA). The sequencing reaction was conducted using Illumina MiSeq instrument (Illumina, San Diego, USA).
16S rRNA gene sequencing data analysis
Raw reads were processed using the QIIME software package.Citation56 In the discovery cohort, the average reads and sequences were 44628.05 (min = 37036; max = 44550), 437.19 (min = 430.42; max = 442.93) in lung cancer group and 54293.15 (min = 48360; max = 72175), 435.58 (min = 427.89; max = 445.46) in healthy control group. In the validation cohort, the average reads and sequences were 36754.50 (min = 30093; max = 44732), 436.42 (min = 430.94; max = 441.39) in lung cancer group and 37909.93 (min = 30166; max = 44984), 433.69 (min = 427.13; max = 444.96) in healthy control group. Each sample has the reads number 22804 post-filtering and rarefying. Operational taxonomic units (OTUs) were picked at 97% similarity cutoff, and the taxonomy was analyzed by the RDP Classifier algorithm against the Greengenes database. α-diversity was measured from the OTU table post-filtering by the Chao and Shannon indexes. β-diversity was estimated by Unweighted UniFrac and Bray–Curtis measures, which were applied to build phylogenetic distance matrices and perform principal coordinates analysis (PCoA). ANOSIM analysis, namely the similarity analysis, was a nonparametric test to compare groups (two or more). These analyses were performed with the free online platform of Majorbio I-Sanger Cloud Platform (www.i-sanger.com).
Statistical analyses
Statistical analyses were carried out in GraphPad Prism Software (San Diego, CA), QIIME and in R packages (V.2.15.3). To work with normalized data, we analyzed an equal number of sequences from all samples in the discovery cohort. Wilcoxon rank-sum test was used to identify microbiota differences. We performed Fisher’s exact test to analyze contingency tables. P < .05 was considered significant. Receiver operating characteristic (ROC) was used to evaluate the performance of potential biomarkers.
Marker selection by mRMR
All markers retained were first filtered by the minimal-Redundancy-Maximal-Relevance (mRMR) algorithm,Citation57 and the top 30 best ones were screened from the discovery cohort and selected for further analysis. Then, we performed a backward selection strategy and a 10-fold cross-validation search to select the optimal subset of microbes, named as markers. We finally selected a set of 13 gut microbial markers as the optimal combination for patient discrimination.
Definition of PDI
To facilitate the clinical application of the selected microbial markers, we further constructed a clinical index, patient discrimination index (PDI), which is partly related to a previously proposed index,Citation22 for discrimination of patients. However, one important difference from its original version is that each marker has been assigned with a weight, which could be estimated through logistic regression. The PDIs based on OTUs and species were calculated, respectively:
PDIOTU=1-1/(1+exp(0.00142+
OTU818:s__Bacteroides_unc.VS.OTU939:s__Bacteroidaceae ovatus×0.509+
OTU163:s__Bacteroides_unc.VS.OTU939:s__Bacteroidaceae ovatus×-1.06+
OTU786:s__Streptococcus infantis.VS.OTU312:s__Blautia_unc×-0.837+
OTU358:s__Erysipelotrichaceae_unc.VS.OTU1063:s__Blautia_unc×-0.23+
OTU236:s__Clostridiales_unc.VS.OTU1100:s__Lachnospiraceae_unc×-0.0581 +
OTU1283:s__Roseburiafaecis.VS.OTU1063:s__Blautia_unc×0.113+
OTU888:s__Bacteria_unc.VS.OTU939:s__Bacteroidaceae ovatus×0.979+
OTU163:s__Bacteroides_unc.VS.OTU1002:s__Bacteroides_unc×1.38+
OTU1222:s__Clostridium_unc.VS.OTU1002:s__Bacteroides_unc×-0.668+
OTU1285:s__Ruminococcus bromii.VS.OTU1100:s__Lachnospiraceae_unc×-0.185+OTU890:s__Enterobacteriaceae_unc.VS.OTU312:s__Blautia_unc×-0.0239+
OTU1353:s__Veillonelladispar.VS.OTU1100:s__Lachnospiraceae_unc×0.0151+
OTU1222:s__Clostridium_unc.VS.OTU312:s_Blautia_unc×0.392))
PDISpecies=1-1/(1+exp(-8.07+
s__Odoribacte_unc.VS.s__Oscillospira_unc×0.538+
s__Clostridium_unc.VS.s__Blautia_unc×-0.222+
s__Ruminococcusbromii.VS.s__Lachnospiraceae_unc×-0.165+
s__Streptococcus_unc.VS.s__Coprococcus_unc×-0.408+
s__Bifidobacteriumlongum.VS.s__Lachnospiraceae_unc×-0.0764+
s__Ruminococcus_unc.VS.s__Blautia_unc×-0.248+
s__TM7-3_unc.VS.s__Coprococcus_unc×-0.0978+
s__Erysipelotrichaceae_unc.VS.s__Lachnospiraceae_unc×-0.833+
s__Roseburia faecis.VS.s__Coprococcus_unc×-0.267))
Author Contributions
HBJ and JFC designed experiments and supervised the study. YJZ, ZYF, YX and JZ performed experiments and analyzed data. PZ, JJZ and GNJ collected patient fecal and provided the clinical information. RYG and HLQ provided the fecal from healthy control. ZYF preformed the bioinformatics analyses. SY, YY, SHW, CDL, SYC, HYH, and LH provided helpful comments. HBJ and JFC interpreted the results. HBJ, JFC, ZYF, and YJZ wrote the manuscript. All authors read and approved the final manuscript.
Disclosure of Potential Conflicts of Interest
No potential conflicts of interest were disclosed.
Supplemental Material
Download PDF (1.1 MB)Acknowledgement
The authors gratefully acknowledge the support of SA-SIBS scholarship program.
Supplementary material
Supplemental data for this article can be accessed on the publisher’s website.
Additional information
Funding
References
- Siegel RL, Miller KD, Jemal A. Cancer Statistics, 2017. CA Cancer J Clin. 2017;67:7–30. doi:10.3322/caac.21387.
- Tuveson DA, Jacks T. Modeling human lung cancer in mice: similarities and shortcomings. Oncogene. 1999;18:5318–5324. doi:10.1038/sj.onc.1203107.
- Jackson EL, Willis N, Mercer K, Bronson RT, Crowley D, Montoya R, Jacks T, Tuveson DA. Analysis of lung tumor initiation and progression using conditional expression of oncogenic K-ras. Genes Dev. 2001;15:3243–3248. doi:10.1101/gad.943001.
- Chen LL, Blumm N, Christakis NA, Barabasi AL, Deisboeck TS. Cancer metastasis networks and the prediction of progression patterns. Br J Cancer. 2009;101:749–758. doi:10.1038/sj.bjc.6605214.
- Amar J, Chabo C, Waget A, Klopp P, Vachoux C, Bermúdez‐Humarán LG, Smirnova N, Bergé M, Sulpice T, Lahtinen S, et al. Intestinal mucosal adherence and translocation of commensal bacteria at the early onset of type 2 diabetes: molecular mechanisms and probiotic treatment. EMBO Mol Med. 2011;3:559–572. doi:10.1002/emmm.201100159.
- Castellarin M, Warren RL, Freeman JD, Dreolini L, Krzywinski M, Strauss J, Barnes R, Watson P, Allen-Vercoe E, Moore RA, et al. Fusobacterium nucleatum infection is prevalent in human colorectal carcinoma. Genome Res. 2012;22:299–306. doi:10.1101/gr.126516.111.
- De Vadder F, Kovatcheva-Datchary P, Goncalves D, Vinera J, Zitoun C, Duchampt A, Bäckhed F, Mithieux G. Microbiota-generated metabolites promote metabolic benefits via gut-brain neural circuits. Cell. 2014;156:84–96. doi:10.1016/j.cell.2013.12.016.
- Gaboriau-Routhiau V, Rakotobe S, Lecuyer E, Mulder I, Lan A, Bridonneau C, Rochet V, Pisi A, De Paepe M, Brandi G, et al. The key role of segmented filamentous bacteria in the coordinated maturation of gut helper T cell responses. Immunity. 2009;31:677–689. doi:10.1016/j.immuni.2009.08.020.
- Henao-Mejia J, Elinav E, Jin C, Hao L, Mehal WZ, Strowig T, Thaiss CA, Kau AL, Eisenbarth SC, Jurczak MJ, et al. Inflammasome-mediated dysbiosis regulates progression of NAFLD and obesity. Nature. 2012;482:179–185. doi:10.1038/nature10809.
- MacFabe DF, Cain DP, Rodriguez-Capote K, Franklin AE, Hoffman JE, Boon F, Taylor A, Kavaliers M, Ossenkopp K. Neurobiological effects of intraventricular propionic acid in rats: possible role of short chain fatty acids on the pathogenesis and characteristics of autism spectrum disorders. Behav Brain Res. 2007;176:149–169. doi:10.1016/j.bbr.2006.07.025.
- Song H, Yoo Y, Hwang J, Na YC, Kim HS. Faecalibacterium prausnitzii subspecies-level dysbiosis in the human gut microbiome underlying atopic dermatitis. J Allergy Clin Immunol. 2016;137:852–860. doi:10.1016/j.jaci.2015.08.021.
- Arrieta MC, Stiemsma LT, Dimitriu PA, Thorson L, Russell S, Yurist-Doutsch S, Kuzeljevic B, Gold MJ, Britton HM, Lefebvre DL, et al. Early infancy microbial and metabolic alterations affect risk of childhood asthma. Sci Transl Med. 2015;7:307ra152. doi:10.1126/scitranslmed.aab2271.
- Thorburn AN, McKenzie CI, Shen S, Stanley D, Macia L, Mason LJ, Roberts LK, Wong CHY, Shim R, Robert R, et al. Evidence that asthma is a developmental origin disease influenced by maternal diet and bacterial metabolites. Nat Commun. 2015;6:7320. doi:10.1038/ncomms8320.
- Trompette A, Gollwitzer ES, Yadava K, Sichelstiel AK, Sprenger N, Ngom-Bru C, Blanchard C, Junt T, Nicod LP, Harris NL, et al. Gut microbiota metabolism of dietary fiber influences allergic airway disease and hematopoiesis. Nat Med. 2014;20:159–166. doi:10.1038/nm.3444.
- He Y, Wen Q, Yao F, Xu D, Huang Y, Wang J. Gut-lung axis: the microbial contributions and clinical implications. Crit Rev Microbiol. 2017;43:81–95. doi:10.1080/1040841X.2016.1176988.
- Budden KF, Gellatly SL, Wood DL, Cooper MA, Morrison M, Hugenholtz P, Hansbro PM. Emerging pathogenic links between microbiota and the gut-lung axis. Nat Rev Microbiol. 2017;15:55–63. doi:10.1038/nrmicro.2016.142.
- Gray LEK, O’Hely M, Ranganathan S, Sly PD, Vuillermin P. The maternal diet, gut bacteria, and bacterial metabolites during pregnancy influence offspring asthma. Front Immunol. 2017;8:365. ARTN. doi:10.3389/fimmu.2017.00365.
- Gray J, Oehrle K, Worthen G, Alenghat T, Whitsett J, Deshmukh H. Intestinal commensal bacteria mediate lung mucosal immunity and promote resistance of newborn mice to infection. Sci Transl Med. 2017;9 doi: ARTN eaaf9412. doi:10.1126/scitranslmed.aaf9412.
- Tamburini S, Clemente JC. Gut microbiota: neonatal gut microbiota induces lung immunity against pneumonia. Nat Rev Gastroenterol Hepatol. 2017;14:263–264. doi:10.1038/nrgastro.2017.34.
- Jin C, Lagoudas GK, Zhao C, Bullman S, Bhutkar A, Hu B, Ameh S, Sandel D, Liang XS, Mazzilli S, et al. Commensal microbiota promote lung cancer development via gammadelta T cells. Cell. 2019;176:998–1013 e16. doi:10.1016/j.cell.2018.12.040.
- Boursi B, Mamtani R, Haynes K, Yang YX. Recurrent antibiotic exposure may promote cancer formation–Another step in understanding the role of the human microbiota? Eur J Cancer. 2015;51:2655–2664. doi:10.1016/j.ejca.2015.08.015.
- Qin N, Yang F, Li A, Prifti E, Chen Y, Shao L, Guo J, Le Chatelier E, Yao J, Wu L, et al. Alterations of the human gut microbiome in liver cirrhosis. Nature. 2014;513:59–64. doi:10.1038/nature13568.
- Hosgood HD, Sapkota AR, Rothman N, Rohan T, Hu W, Xu J, Vermeulen R, He X, White JR, Wu G, et al. The potential role of lung microbiota in lung cancer attributed to household coal burning exposures. Environ Mol Mutagen. 2014;55:643–651. doi:10.1002/em.21878.
- Rutten EPA, Lenaerts K, Buurman WA, Wouters EFM. Disturbed intestinal integrity in patients with COPD: effects of activities of daily living. Chest. 2014;145:245–252. doi:10.1378/chest.13-0584.
- Wang J, Li F, Wei H, Lian ZX, Sun R, Tian Z. Respiratory influenza virus infection induces intestinal immune injury via microbiota-mediated Th17 cell-dependent inflammation. J Exp Med. 2014;211:2397–2410. doi:10.1084/jem.20140625.
- Ettinger DS, Wood DE, Akerley W, Bazhenova LA, Borghaei H, Camidge DR, Cheney RT, Chirieac LR, D’Amico TA, Demmy TL, et al. Non-small cell lung cancer, version 6.2015. J Natl Compr Canc Netw. 2015;13:515–524. doi:10.6004/jnccn.2015.0071.
- Mukhopadhya I, Hansen R, El-Omar EM, Hold GL. IBD-what role do Proteobacteria play? Nat Rev Gastroenterol Hepatol. 2012;9:219–230. doi:10.1038/nrgastro.2012.14.
- Shin NR, Whon TW, Bae JW. Proteobacteria: microbial signature of dysbiosis in gut microbiota. Trends Biotechnol. 2015;33:496–503. doi:10.1016/j.tibtech.2015.06.011.
- Jenq RR, Taur Y, Devlin SM, Ponce DM, Goldberg JD, Ahr KF, Littmann ER, Ling L, Gobourne AC, Miller LC, et al. Intestinal blautia is associated with reduced death from graft-versus-host disease. Biol Blood Marrow Transplant. 2015;21:1373–1383. doi:10.1016/j.bbmt.2015.04.016.
- Sivan A, Corrales L, Hubert N, Williams JB, Aquino-Michaels K, Earley ZM, Benyamin FW, Man Lei Y, Jabri B, Alegre M-L, et al. Commensal Bifidobacterium promotes antitumor immunity and facilitates anti-PD-L1 efficacy. Science. 2015;350:1084–1089. doi:10.1126/science.aac4255.
- Zhang LL, Chen X, Zheng PY, Luo Y, Lu GF, Liu ZQ, Huang H, Yang P-C. Oral Bifidobacterium modulates intestinal immune inflammation in mice with food allergy. J Gastroenterol Hepatol. 2010;25:928–934. doi:10.1111/j.1440-1746.2009.06193.x.
- Liu S, Li E, Sun Z, Fu D, Duan G, Jiang M, Yu Y, Mei L, Yang P, Tang Y, et al. Altered gut microbiota and short chain fatty acids in Chinese children with autism spectrum disorder. Sci Rep. 2019;9:287. doi:10.1038/s41598-018-36430-z.
- Riihimaki M, Hemminki A, Fallah M, Thomsen H, Sundquist K, Sundquist J, Hemminki K. Metastatic sites and survival in lung cancer. Lung Cancer. 2014;86:78–84. doi:10.1016/j.lungcan.2014.07.020.
- Klemenak M, Dolinsek J, Langerholc T, Di Gioia D, Micetic-Turk D. Administration of bifidobacterium breve decreases the production of TNF-alpha in children with celiac disease. Dig Dis Sci. 2015;60:3386–3392. doi:10.1007/s10620-015-3769-7.
- Shang GS, Liu L, Qin YW. IL-6 and TNF-alpha promote metastasis of lung cancer by inducing epithelial-mesenchymal transition. Oncol Lett. 2017;13:4657–4660. doi:10.3892/ol.2017.6048.
- Sokol H, Pigneur B, Watterlot L, Lakhdari O, Bermudez-Humaran LG, Gratadoux JJ, Blugeon S, Bridonneau C, Furet J-P, Corthier G, et al. Faecalibacterium prausnitzii is an anti-inflammatory commensal bacterium identified by gut microbiota analysis of Crohn disease patients. P Natl Acad Sci USA. 2008;105:16731–16736. doi:10.1073/pnas.0804812105.
- Zhuang H, Cheng L, Wang Y, Zhang YK, Zhao MF, Liang GD, Zhang M-C, Li Y-G, Zhao J-B, Gao Y-N, et al. Dysbiosis of the gut microbiome in lung cancer. Front Cell Infect Microbiol. 2019;9:112. doi:10.3389/fcimb.2019.00112.
- Louis P, Flint HJ. Diversity, metabolism and microbial ecology of butyrate-producing bacteria from the human large intestine. FEMS Microbiol Lett. 2009;294:1–8. doi:10.1111/j.1574-6968.2009.01514.x.
- Tamanai-Shacoori Z, Smida I, Bousarghin L, Loreal O, Meuric V, Fong SB, Bonnaure-Mallet M, Jolivet-Gougeon A. Roseburia spp.: a marker of health? Future Microbiol. 2017;12:157–170. doi:10.2217/fmb-2016-0130.
- Abell GC, Cooke CM, Bennett CN, Conlon MA, McOrist AL. Phylotypes related to Ruminococcus bromii are abundant in the large bowel of humans and increase in response to a diet high in resistant starch. FEMS Microbiol Ecol. 2008;66:505–515. doi:10.1111/j.1574-6941.2008.00527.x.
- Mur LA, Huws SA, Cameron SJ, Lewis PD, Lewis KE. Lung cancer: a new frontier for microbiome research and clinical translation. Ecancermedicalscience. 2018;12:866. doi:10.3332/ecancer.2018.866.
- Yan X, Yang M, Liu J, Gao R, Hu J, Li J, Zhang L, Shi Y, Guo H, Cheng J, et al. Discovery and validation of potential bacterial biomarkers for lung cancer. Am J Cancer Res. 2015;5:3111–3122.
- Yang X, Zhang Y, Hosaka K, Andersson P, Wang J, Tholander F, Cao Z, Morikawa H, Tegnér J, Yang Y, et al. VEGF-B promotes cancer metastasis through a VEGF-A-independent mechanism and serves as a marker of poor prognosis for cancer patients. Proc Natl Acad Sci U S A. 2015;112:E2900–9. doi:10.1073/pnas.1503500112.
- Paik PK, Shen RL, Won H, Rekhtman N, Wang L, Sima CS, Arora A, Seshan V, Ladanyi M, Berger MF, et al. Next-generation sequencing of stage IV squamous cell lung cancers reveals an association of pi3k aberrations and evidence of clonal heterogeneity in patients with brain metastases. Cancer Discov. 2015;5:610–621. doi:10.1158/2159-8290.Cd-14-1129.
- Liu XQ, Chen B, You WJ, Xue S, Qin H, Jiang HD. The membrane bile acid receptor TGR5 drives cell growth and migration via activation of the JAK2/STAT3 signaling, pathway. in non-small cell lung cancer. Cancer Lett. 2018;412:194–207. doi:10.1016/j.canlet.2017.10.017.
- Mishra S, Imlay JA. An anaerobic bacterium, Bacteroides thetaiotaomicron, uses a consortium of enzymes to scavenge hydrogen peroxide. Mol Microbiol. 2013;90:1356–1371. doi:10.1111/mmi.12438.
- Baumler AJ, Sperandio V. Interactions between the microbiota and pathogenic bacteria in the gut. Nature. 2016;535:85–93. doi:10.1038/nature18849.
- Curtis MM, Hu Z, Klimko C, Narayanan S, Deberardinis R, Sperandio V. The gut commensal Bacteroides thetaiotaomicron exacerbates enteric infection through modification of the metabolic landscape. Cell Host Microbe. 2014;16:759–769. doi:10.1016/j.chom.2014.11.005.
- Ren ZG, Li A, Jiang JW, Zhou L, Yu ZJ, Lu HF, Xie H, Chen X, Shao L, Zhang R, et al. Gut microbiome analysis as a tool towards targeted non-invasive biomarkers for early hepatocellular carcinoma. Gut. 2019;68:1014–1023. doi:10.1136/gutjnl-2017-315084.
- Yang JQ, Tan QW, Fu QY, Zhou YJ, Hu YY, Tang SL, Zhou Y, Zhang J, Qiu J, Lv Q, et al. Gastrointestinal microbiome and breast cancer: correlations, mechanisms and potential clinical implications. Breast Cancer-Tokyo. 2017;24:220–228. doi:10.1007/s12282-016-0734-z.
- Ren ZG, Jiang JW, Xie HY, Li A, Lu HF, Xu SY, Zhou L, Zhang H, Cui G, Chen X, et al. Gut microbial profile analysis by MiSeq sequencing of pancreatic carcinoma patients in China. Oncotarget. 2017;8:95176–95191. doi:10.18632/oncotarget.18820.
- Meng CT, Bai CM, Brown TD, Hood LE, Tian Q. Human gut microbiota and gastrointestinal cancer. Genom Proteom Bioinf. 2018;16:33–49. doi:10.1016/j.gpb.2017.06.002.
- Shah MS, DeSantis TZ, Weinmaier T, McMurdie PJ, Cope JL, Altrichter A, Yamal J-M, Hollister EB. Leveraging sequence-based faecal microbial community survey data to identify a composite biomarker for colorectal cancer. Gut. 2018;67:882–891. doi:10.1136/gutjnl-2016-313189.
- Lee SH, Sung JY, Yong D, Chun J, Kim SY, Song JH, Chung KS, Kim EY, Jung JY, Kang YA, et al. Characterization of microbiome in bronchoalveolar lavage fluid of patients with lung cancer comparing with benign mass like lesions. Lung Cancer. 2016;102:89–95. doi:10.1016/j.lungcan.2016.10.016.
- Maurice CF, Haiser HJ, Turnbaugh PJ. Xenobiotics shape the physiology and gene expression of the active human gut microbiome. Cell. 2013;152:39–50. doi:10.1016/j.cell.2012.10.052.
- Caporaso JG, Kuczynski J, Stombaugh J, Bittinger K, Bushman FD, Costello EK, Fierer N, Peña AG, Goodrich JK, Gordon JI, et al. QIIME allows analysis of high-throughput community sequencing data. Nat Methods. 2010;7:335–336. doi:10.1038/nmeth.f.303.
- Ding C, Peng H. Minimum redundancy feature selection from microarray gene expression data. J Bioinform Comput Biol. 2005;3:185–205. doi:10.1142/s0219720005001004.