ABSTRACT
Nonalcoholic fatty liver disease (NAFLD) is a risk factor for liver cancer and prevalence varies by ethnicity. Along with genetic and lifestyle factors, the gut microbiome (GM) may contribute to NAFLD and its progression to advanced liver disease. Our cross-sectional analysis assessed the association of the GM with hepatic adiposity among African American, Japanese American, White, Latino, and Native Hawaiian participants in the Multiethnic Cohort. We used MRI to measure liver fat and determine nonalcoholic fatty liver disease (NAFLD) status (n = 511 cases) in 1,544 participants, aged 60–77 years, with 12–53% overall adiposity (BMI of 17.8–46.2 kg/m2). The GM was measured by 16S rRNA gene sequencing and, on a subset, by metagenomic sequencing. Alpha diversity was lower overall with NAFLD and in certain ethnicities (African Americans, Whites, and Latinos). In models regressing genus on NAFLD status, 62 of 149 genera (40%) exhibited a significant interaction between NAFLD and ethnicity stratified analysis found 69 genera significantly associated with NAFLD in at least one ethnic group. No single genus was significantly associated with NAFLD across all ethnicities. In contrast, the same bacterial metabolic pathways were over-represented in participants with NAFLD regardless of ethnicity. Imputed secondary bile acid and carbohydrate pathways were associated with NAFLD, the latter of which was corroborated by metagenomics, although different genera in different ethnicities were associated with these pathways. Overall, we found that NAFLD was associated with altered bacterial composition and metabolism, and that bacterial endotoxin, assessed by plasma lipopolysaccharide binding protein (LBP), may mediate liver fat-associated systemic inflammation in a manner that seems to vary by ethnicity.
Introduction
Nonalcoholic fatty liver disease (NAFLD) is estimated to affect >30% of the US population and is closely associated with elevated risk for cardiovascular disease, metabolic syndrome, type 2 diabetes (T2D), and overall malignancy. Metabolic disorders, including NAFLD – hepatic manifestations of obesity – now contribute more to the risk of hepatocellular carcinoma (HCC) than any other etiologies for this cancer in the US.Citation1 Multiple risk factors for NAFLD include ethnicity, genetics, diet, and the gut microbiome (GM) and its metabolites.Citation2 Setiawan et al.Citation3 found that NAFLD was the most common etiology for chronic liver disease (CLD) in all five ethnic groups in the Multiethnic Cohort Study (MEC); however, the proportion of NAFLD-associated CLD varied across ethnic groups. Obesity, especially visceral adiposity which varies across ethnicity, was associated with NAFLD.Citation4 Diet and diet quality also varying across ethnic groups, was associated with NAFLDCitation5,Citation6 and may play a role in ethnic differences in risk of CLD, which have also been associated with different patterns of dietary intake.Citation7,Citation8
The gut-liver axis, whereby the liver is exposed to gut microbial metabolites and endotoxins (i.e., gram-negative bacterial cell wall material), may promote inflammation in the development of NAFLD. GM composition and microbial metabolic pathways,Citation9–11 may influence host metabolism, oxidative stress, and systemic and liver inflammation. Previous studies have identified reduced bacterial diversity with NAFLD but have not identified consistently specific bacterial genera associated with NAFLD, although this discrepancy may be due to small sample sizes and heterogeneous study populations.Citation9,Citation10,Citation12–15 This has limited the potential to develop biomarkers of ectopic fat related to disease risk stratified along ethnicity.Citation4 Despite evidence that NAFLD varies across ethnicities,Citation4,Citation16 the variation in how the GM is associated with NAFLD has not been investigated across ethnic groups.
Our aim was to examine, in a cross-sectional subset of the Multiethnic Cohort, the associations between NAFLD and GM composition and bacterial metabolic pathways. This analysis took advantage of a relatively large sample of the cohort’s five ethnic groups and the state-of-the-art measurements of liver at and NAFLD using MRI It affords the opportunity to measure the association between the microbiome structure and function that may link pathophysiologic pathways to potential clinical relevanceCitation17in treating the racial/ethnic disparity in the severity of NAFLD.
Materials/subjects and methods
Study population
The MEC study has followed 215,000 Hawaii and Los Angeles residents of African American, Japanese American, white, Latino, and Native Hawaiian origin since 1993–1996.Citation18 The Multiethnic Cohort – Adiposity Phenotype Study (MEC-APS) examined 1,861 healthy MEC participants, including men and postmenopausal women aged 60–77 years, of the five main MEC ethnic groups.Citation4 The recruitment was stratified by sex, ethnicity and six body mass index (BMI) categories to balance distribution across a wide range of BMI in each sex-ethnic group. Between 2013 and 2016, participants visited the two affiliated clinical centers in Honolulu, Hawaii, or Los Angeles, California, to undergo a whole-body dual-energy X-ray absorptiometry (DXA) and abdominal magnetic resonance imaging (MRI) scan, anthropometric and resting metabolism measurements, fasting blood and stool sample collections, and repeat administration of the MEC food frequency questionnaire (FFQ) covering over 180 food items including ethnic-specific foods.Citation5,Citation19 Usual dietary intake and the Healthy Eating Index-2010Citation20(HEI-2010; as a measure of overall diet quality) over the past year were estimated from the FFQ.
As detailed in a previous report,Citation4 individuals were excluded for current or recent (<2 years) smoking, amputation or implants, claustrophobia, insulin or thyroid medication, dialysis, and serious health conditions such as chronic viral hepatitis and dialysis. Also, participation was deferred for those with recent history within 6 months of chemotherapy or radiation therapy of the abdomen, antibiotic used, substantial weight change (>20 pounds) or colonoscopy as well as vaccination within 1 month. We also limited the current analysis to nill to moderate alcohol drinkers (men <30 g/day, women <20 g.day based on FFQ). Our study was based on 1,544 of the 1,861 MEC-APS participants after removing 74 that were missing microbiome data, 62 missing liver fat data mostly due to motion artifacts, and 181 who reported high alcohol use. For adjustment of total adiposity, we also removed 15 participants without valid DXA measure which resulted in 1,529 participants in the adjusted analysis including Latinos (n = 325) and African Americans (n = 256) recruited in Los Angeles and Native Hawaiian (n = 246), all whites (n = 316), and Japanese Americans (n = 400), as well as one African American recruited in Hawaii.
The study protocol conformed to the ethical guidelines of the 1975 Declaration of Helsinki and Institutional Review Board reviews were conducted by participating institutions. Signed informed consent was obtained from all study participants.
Assessment of liver fat and NAFLD
We measured percent liver fat using abdominal MRI and total body fatness using DXA as previously describedCitation4 with a method that has shown high accuracy compared to liver biopsy.Citation21 NAFLD was defined as percent liver fat of >5.5%.Citation22
Blood biomarkers
Venous blood (40 mL) was collected after an overnight fast (>8 hours), processed in the MEC laboratories in Hawaii and Los Angeles to components (plasma, buffy coat, serum), and stored at −80°C until shipment for biomarker assays at the UH Cancer Center Analytical Biochemistry Shared Resource laboratory (directed by Dr. Franke). Samples were arranged into batches so that each batch included approximately equal numbers of men and women of each ethnic group and ~10% blind QC duplicates. Plasma lipopolysaccharide binding protein (LBP) was analyzed using a commercial ELISA kit (Cell Sciences, CKH113; coefficient of variation (CV): 0.7%; intraclass correlation coefficient (ICC) 80%). Serum high-sensitivity C-reactive protein (CRP), a measure of systemic inflammation and alanine amino transferase (ALT), a plasma marker of liver dysfunction, were measured as previously reported.Citation23 Our assays for CRP and ALT had a CV of 13.8% and 4.4% and ICCs of 88% and 82%, respectively.
Microbiome Analysis
Sample collection
Stool samples were collected at home using a collection tube containing 5 mL RNAlater (Fisher Scientific) and sterile 5 mm glass beads (Ambion) to facilitate sample dispersion in RNAlater.Citation24 Participants kept their samples in their freezers and brought them to the study clinic. At sample collection, participants filled out a questionnaire to provide collection time, special diets, consumption of probiotic foods in the past year, and whether participants were treated with an oral, injection, or IV form of antibiotics in the past year, and if so, how recently.
Sample processing
Stool samples were stored in RNAlater at −80°C at study centers and shipped in bulk on dry ice to Fred Hutchinson Cancer Research Center (Fred Hutch). Stool samples were thawed and homogenized, and genomic DNA was extracted.Citation24 Briefly, to optimize bacterial genomic DNA extraction, we did bead beating at 45s (2x) each with samples placed on ice in between. Quality control samples, duplicate participant samples, and processing blanks were used to assess variation in library preparation and sequencing batches.Citation25
For paired-end sequencing of the V1–V3 region of the 16S rRNA gene, the 27 F mod forward PCR primer sequence was 5°-AGRGTTNGATCMTGGCTYAG-3°. The 519 R reverse PCR primer sequence was 5°-GTNTTACNGCGGCKGCTG-3°. Three PCR (20 µl; 20 ng genomic DNA) reactions were performed using the HotStarTaq Plus Master Mix Kit (QIAGEN) under the following conditions: 94°C for 3 minutes, followed by 28 cycles of 94°C for 30 seconds, 53°C for 40 seconds, and 72°C for 1 minute, after which a final elongation step at 72°C for 5 minutes was performed. After amplification, quality of the PCR products were checked in 2% agarose gel. The three PCR products were pooled together in equal proportions based on their molecular weight and DNA concentrations. Paired-end sequencing performed at Molecular Diagnostics, LLP (Shallowater, TX) on the MiSeq using MiSeq Reagent Kit v3 following the manufacturer’s guidelines to obtain 2 × 300 bp paired-end reads (Illumina, San Diego, CA). On a subset of samples chosen from the NAFLD vs non-NAFLD groups from each ethnicity (n = 30; six from each ethnicity), we performed whole-genome shotgun sequencing on an Illumina HiSeq generating 2×150bp paired-end reads. FastQ files were exported and securely transferred (BaseSpace, Illumina) to Fred Hutch for bioinformatic analysis.
Microbiome bioinformatic data processing
To classify bacterial taxonomy, sequences were processed using QIIME v.1.8Citation26 as previously described.Citation25 The filtering strategy for operational taxonomic units (OTUs) included parameters in QIIME to exclude low abundant sequences, singletons, and chimeras. Briefly, the OTU table was filtered using the QIIME script filter_OTUs_from_OTU_table.py with – min_count_fraction set to 0.00005. Additional OTU entries were filtered out if they were detected as chimeras using QIIME’s identify_chimeric_seqs.py script with method blast_fragments. Final filtering excluded genera which appeared in <10% of the subjects.Citation27 Bacterial functional genes were imputed from 16S rRNA genes counts that were adjusted for batch (see below).Citation27
Sequence reads were processed for bioinformatic analysis of the metagenomes (n = 30) with the KneadData v 0.5.1 quality control pipeline, which uses Trimmomatic (version 0.36), BMTagger filtering, and decontamination algorithms to remove low-quality read bases and host (human) reads, respectively.Citation28 Trimmomatic was run with parameters MAXINFO:80:0.5 and MINLEN:50. Functional profiling was performed using HUMAnN2 version 0.11.2Citation29 with reads de-paired and implementing DiamondCitation30 to map reads against UniRef90.Citation31 Sequences per gene family were counted, normalized for length and alignment quality, and linked to pathways using MetaCyc.Citation32 Data matrices of the abundance of genes, gene families, and genes in metabolic pathways were generated. We quantified the abundance of the UniRef90 gene families and gene names for secondary bile acids (Supplemental Table 2) and carbohydrate metabolism (Table 3)Citation33 by summing the normalized counts of all genes that mapped to these pathways.
Enzymes in carbohydrate metabolism were identified using the CAZy database (31 July 2019 ver., URL http://bcb.unl.edu/dbCAN2/download/Databases/CAZyDB.07312019.fa). A DIAMOND (version 0.9.22) database was generated from the FASTA file and used to align metagenomics reads, where hits were retained if the e-value <10−20. Data for statistical analysis were filtered for abundance (cells with less than 46 counts which represents the 25th percentile of non-zero counts were placed to zero) and prevalence (variables that had greater than or equal to 75% zero counts were removed). All sequences follow MIMARKS standardCitation34 and are publicly available in the Sequence Read Archive (http://www.ncbi.nlm.nih.gov/sra/.)
On unrarefied count data, we applied ComBat-seqCitation35 designed to remove unwanted (non-biological) variation, while preserving potentially relevant variation in sequence count data. This was applied by including, for each sample, information on sequencing batch along with each participant’s sex and ethnicity. An additional set of quality-control samples run in each batch were also used in this process. The resulting adjusted counts were used in all subsequent statistical analyses.
Statistical Analysis
We examined the association between the GM and NAFLD among the 1,529 MEC-APS participants.Citation35,Citation36 Results from each significance test are reported as a “q” value and statistical significance was defined by an FDR cutoff less than 0.05.Citation37 Analyses were performed using R Version 4.0.2.
Participant characteristics
Demographic, anthropometric, health, lifestyle, and dietary measures were examined overall and by NAFLD status (, , and Supplemental Figures 5E–9E). These were assessed using t-tests for continuous variables and chi-squared tests for categorical variables as were the subset of participants for which we did metagenomic sequencing (Supplemental Table 4).
Table 1. MEC-APS participant characteristics by NAFLD status (liver fat>5.5%)
Figure 1. Overview of the microbiome in NAFLD in the MEC-APS study. 1A.) Distribution of individuals with and without NAFLD. 1B.) Variation in the microbiome between individuals with and without NAFLD illustrated by a PCOA of weighted Unifrac metric. 1 C.) Vector overlay of the demographic, anthropometric, health, diet, and lifestyle variables. 1D.) Alpha diversity in subjects with and without NAFLD by ethnicity and 1E.) Variation in the microbiome explained by the demographic anthropometric, health, diet, and lifestyle variables using perMANOVA analysis
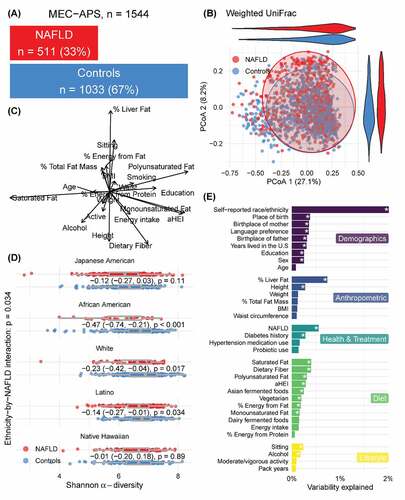
Alpha and beta diversity
Linear regression was used to model Shannon alpha diversity by NAFLD status and its interaction with ethnicity, adjusted for sex and total adiposity (from DXA) and in similar models stratified by ethnic groups. To quantify the extent to which various demographics, anthropometric, health, diet, and lifestyle measures were associated with the overall gut microbiota composition, we implemented perMANOVACitation38 using weighted UniFrac distances between pairs of taxon abundance vectors (Supplemental Table 5).Citation39
Phyla and Genera
Beta-binomial regression models were fit, marginally, for each genus and phylum using the R package ‘corncob.’Citation40 The observed count of each taxon and the sample’s sequencing depth were used to model the population proportion of each taxon relative to covariates. The beta component models the probability parameter of the binomial distribution to better account for the overdispersion and contains mean and overdispersion components for each taxon. For genera present in at least 5% of the entire participants, we first modeled each taxon by including a NAFLD-ethnicity interaction term, adjusted by sex and percent total fat for both mean and overdispersion components. Since this interaction was significant in 62 out of 149 genera (Supplementary Table 1B), we concluded there is strong evidence for ethnic-specific associations between NAFLD status and taxon abundance and refitted these models stratified by each of the five ethnic groups. In analyses stratified by ethnicity, we only modeled those genera present in at least 25% of each group. We used a likelihood ratio test to assess differences in mean proportions by NAFLD status.
The association of microbial metabolic pathways with liver fat
Linear regression was used to model percent liver fat, as the dependent variable, by piCRUST-imputed metabolic pathways.Citation27 Pathway counts were adjusted for batch using ComBat-seq and each sample was normalized using the centered-log ratio (CLR).Citation41 CLR values were standardized (mean 0 and standard deviation 1) for comparison of pathway coefficients across models. Models were adjusted for total adiposity, sex, and self-reported ethnicity. We fit additional models stratified by sex and ethnicity adjusted only for total adiposity.
In a subset of 27 samples that were assessed using metagenomic sequencing, we tested whether the relative abundance of sequenced genes in six metagenomic pathways differed by NAFLD status using a Wilcoxon rank-sum test. We similarly tested 146 glucosyl hydrolase enzymes.
Mediation analysis
Mediation analysis was used to assess whether the association between percent liver fat and a marker of systemic inflammation (CRP) was mediated by LBP. For this analysis, we used 1,326 participants having non-missing, non-zero measures of CRP. In models stratified by ethnicity, we estimated direct and indirect effects by regressing log(CRP) on percent liver fat, with and without LBP, and regressing of LBP onto percent liver fat. Bootstrapped confidence intervals were used to evaluate the direct, indirect, and mediating effects.Citation42,Citation43 All models were adjusted for sex and percent total fat mass.
Results
Participant characteristics by NAFLD status
As reported previously,Citation4 the prevalence of NAFLD varied widely by ethnicity, especially higher in Japanese Americans and lower in African Americans than in other groups (). As expected, NAFLD cases, compared to non-cases, had higher mean levels of percent liver fat, total fat mass, BMI, waist circumference, higher prevalence of diabetes history and hypertension medication use. NAFLD cases and non-cases had similar intake of probiotics and total energy, but cases had higher percent energy from fat, protein, and saturated fats and lower levels of energy-adjusted dietary fiber and overall diet quality, determined with a HEI-2015 score. A sedentary lifestyle and smoking were significantly higher in participants with NAFLD whereas alcohol intake and activity were higher in participants without NAFLD. Mean plasma LBP, CRP, and ALT concentrations were greater in the NAFLD group overall and within each ethnic group, although significance varied by ethnicity ().
Table 2. Plasma Concentrations of LBP, CRP and ALT in MEC-APS participants by NAFLD status, overall and stratified by ethnicity
Gut microbiome
Microbiome sequencing averaged 33,422 16S rRNA gene sequences per sample with an average length of 499 bp. We identified 10 phyla, 152 genera, and 1,311 OTUs. We sampled metagenomes in a subset of participants (n = 27) which averaged 20.3 M reads ± 2.8 M reads per sample before quality filtering and 20.2 M reads ± 2.9 M reads afterward, with a mean of 0.5% of the reads removed including human and poor quality reads. Sequence length was 148 bp ± 14 bp before QC, and 143 bp ± 19 bp after QC. Three of the original 30 samples were removed from the metagenomic analysis due to participant’s high alcohol consumption leaving a total of 27 samples of which 13 were in the non-NAFLD category and 14 were in the NAFLD category and mapping rate of high-quality reads was similar across ethnic groups (Supplemental Table 6).
We modeled the association of alpha diversity (Shannon Index) with NAFLD in the entire sample with adjustment for sex, total fat mass, ethnicity, and the interaction of NAFLD with ethnicity. Alpha diversity was inversely associated with NAFLD status overall (p = 0.034). There was a significant interaction of ethnicity and NAFLD and in stratified analysis among African Americans (p < 0.001), whites (p = 0.017) and Latinos (p = 0.034) (; Supplemental Figures 5D–9D).
We tested the association of overall gut microbial composition (beta diversity) with NAFLD status using perMANOVACitation38 with respect to weighted UniFrac,Citation39 including terms for sex, total fat mass, ethnicity, and the interaction of NAFLD with ethnicity. We observed a significant ethnicity−by−NAFLD interaction (p = 0.014) which explained 0.44% of the variability. Microbial beta diversity was associated with NAFLD among all participants (q < 0.05) (). Self-reported ethnicity (2%), percent liver fat (0.74%), NAFLD (0.55%), and dietary fiber (0.4%), and saturated fat (0.04%) were among the variables that explained the most the overall variation in the microbiome (). In ethnicity stratified results (Supplemental Figures 5–9), NAFLD and % liverfat explained a significant but small amount of variation in the overall microbiome composition (Supplemental Figures 5–9 A-F).
Over all participants, at the phylum-level, we observed associations with seven out of 10 phyla and 62 out of 149 genera (Supplemental Table 1A and 1B). Fusobacteria (p < 0.0001), Bacteroidetes (p < 0.0001), and Proteobacteria (p < 0.0001) were positively associated with NAFLD. At the genus level, 14 genera were positively associated with NAFLD (all q < 0.01) and 48 genera (all q < 0.01) were inversely associated with NAFLD (Supplemental Table 1B).
We observed a significant interaction between NAFLD status and ethnicity in phyla (Supplemental Figure 1). Proteobacteria were positively associated with African American subjects with NALFD and Actinobacteria were positively associated with white subjects with NAFLD. Fusobacteria was inversely associated with NAFLD in Blacks and positively associated in whites. Tenericutes were inversely associated with NAFLD in African Americans, whites, and Latinos. Firmicutes and Synergistetes were inversely associated with NAFLD in African Americans and whites. The prevalence and abundance of genera (Supplemental Table 1B; Supplemental Figures 5–9). Genera were significantly positively associated with liver fat in at least one ethnic group (as summarized in ; Supplemental Table 1B), although only R. gnavus group (Japanese Americans, whites, and Latinos) and Enterobacter (Japanese Americans, African Americans, and whites)Citation44 were found in common across three ethnicities. Megamonas was enriched in Hawaiians and depleted in African Americans with NAFLD. Alloprevotella was increased in whites but decreased in Latinos, and Klebsiella was enriched in whites and decreased in Native Hawaiians. Christensenellaceae was negatively associated with African American subjects with NAFLD. Among those genera that differed significantly in abundance by NAFLD status within a single ethnic group, AggregatibacterCitation45–47 and Lachnoclostridium were enriched in Latinos with NAFLD, Blautia was enriched in Japanese Americans, and Parasutterella was enriched in Native Hawaiians. The prevalence and abundance of Fusobacterium varied across ethnic groups (Supplemental Table 1B and Supplemental Figure 4).
Figure 2. Heatmap of regression coefficients for association with NAFLD resulting from beta-binomial regression models of genera counts stratified by self-report ethnicity. Genera significantly enriched or depleted in individuals with NAFLD are shown. Coefficients are adjusted for sex, and total fat mass. Red = positive, blue = negative
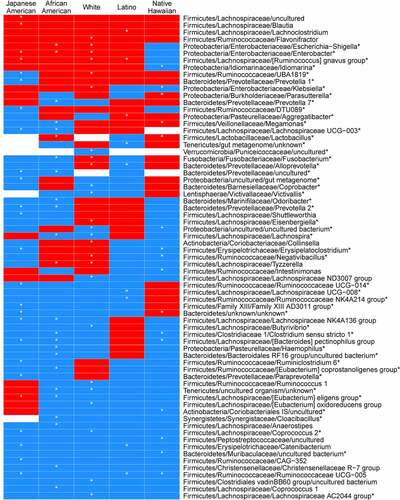
Several genera were inversely associated with NAFLD although there were some divergent patterns for genera within families across ethnicities (; Supplemental Table 1B). We found that among the genera in the Lachnospiraceae family, Coprococcus-2 was decreased in Japanese Americans and whites whereas Lachnospira was decreased African American and whites, and Lachnospiraceae UCG-003 was decreased in Japanese Americans and African Americans with NAFLD. In the Ruminococcaceae, some genera were unique to an ethnic group: five unique genera only in Japanese Americans, two genera unique to Native Hawaiians and one genera each in Latinos, African Americans, and whites. In the Bacteroidetes, Prevotella-1 and Prevotella-7 were decreased in Japanese American and African American participants, respectively, and an uncultured Prevotellaceae was decreased in both. Coprobacter was decreased in whites, an uncultured genera in the Muribaculaceae in whites, and Odoribacter in African Americans. The most common genera that were significantly inversely associated with NAFLD were the Bacteroides pectinophilus group in Japanese Americans, African Americans, and Native Hawaiians and Ruminococcaceae UCG-005 in Japanese Americans, Latinos, and Native Hawaiians. In the Family Erysipelotrichaceae, Catenibacterium was decreased in Japanese American and Latino participants with NAFLD whereas Erysipelatoclostridium was decreased in Native Hawaiians and Japanese Americans.
ALT, a measure of liver damage, was associated with genera in a beta binomial regression stratified by ethnicity and adjusted for sex and total adiposity (Supplemental Table 7). R. gnavus was significantly positively associated with ALT in three ethnicities: Japanese Americans, whites, and Latinos. Klebsiella was significantly positively associated with ALT in whites and Native Hawaiians. Megamonas was inversely associated with ALT in African Americans and positively associated in Native Hawaiians. Several single genera in the Lachnospriaceae, Ruminococcaeae, and Christensenellaceae were negatively associated with ALT in a specfic ethnicity.
Microbial metabolic pathways were associated with liver fat (; Supplemental Table 5, Supplemental Figure 2). Among all participants, we found a positive association of liver fat with imputed bacterial functional genes involved in secondary bile acid metabolism and carbohydrate metabolism. Japanese American males showed a positive association with carbohydrate metabolism and Native Hawaiian females showed a positive association with secondary bile acids metabolism (conjugate metabolism of taurine and C1 amino acids) and other pathways associated with branched chain amino acids and fatty acid synthesis.Citation48,Citation49
Figure 3. Heatmap of the association between imputed microbial functional pathways with percent liver fat by sex and ethnicity in the MEC-APS (n = 1529). The colors represent the magnitude of the pathway standardized coefficient in the linear model of the natural log of percent liver fat on each pathway, adjusted for sex, ethnicity, total fat mass, and sequencing batch. The gene counts were inferred from piCRUST and pathways summarized by summing all counts in the pathway and then normalized for the total number of sequences per sample, which were then standardized for the model. Asterisks indicate a BH corrected p value less than 0.1. (Also see Supplemental Table 5.)
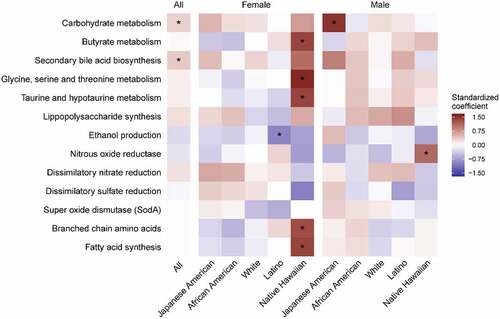
Metagenomic sequencing of functional genes on a subset of participants (n = 27; Supplemental Table 4) showed that microbial carbohydrate enzymes involved in the degradation of hemicellulose was significantly higher in participants with NAFLD (Supplemental Figure 2) and GH43_34, (β-xylosidase, α-L-arabinofuranosidase, Supplemental Figure 2; q < 0.1). This enzyme is found in the genera Blautia (GenBank: QBE95338.1, GenBank: ANU78801.1) and Lachnoclostridium (GenBank: ANU49904.1), that were significantly enriched in the individuals with NAFLD cases (Supplemental Figure 2).
In the mediation models, plasma LBP concentrations mediated 22% (95% CI: 10–34%; p < 0.001) of liver fat’s effect on CRP. When we stratified the mediation models by ethnicity, plasma LBP concentrations in Latinos mediated 44% (95% CI: p < 0.01) of liver fat’s association with CRP. We initially considered whether LBP modified the association of liver fat with CRP within ethnicities by fitting a linear model containing the interaction of liver fat and LBP. We found no evidence for this and concluded that LBP mediates, rather than modifies, the effect of liver fat on CRP.Citation50
Discussion
In the MEC-APS, where we previously reported substantial ethnic differences in the propensity for NAFLD,Citation4 our findings reported here suggest that the GM may contribute in part to the differences. We found that the microbial measures of alpha diversity, microbiome composition, and novel pathophysiologic links through gut bacterial metabolic pathways and endotoxin, were associated with NAFLD, the degree to which often differed by ethnicity. Participants with NAFLD had significantly lower alpha diversity overall and in 3 out of 5 ethnic groups, individually. No single genus was associated with NAFLD across all ethnicities, although different genera associated with production of secondary bile acids were found in common in at least three ethnicities. Carbohydrate metabolism and endotoxin-mediated inflammation were significantly higher in individuals with NAFLD. Ethnic-specific genera that ferment carbohydrates to acetate, a precursor for lipogenesis, were enriched in NAFLD subjects, suggesting common pathophysiologic links in the microbiome contribute to NAFLD.
Dysbiosis associated with decreased diversity of the microbiome starts early in simple liver steatosis and may lay the foundation for progression to inflammation and fibrosis.Citation51,Citation52 Here, we observed that not only did individuals with NAFLD, independently of their total fat mass, have a lower diversity of the gut microbiome (independent of host total fat mass) (), but this association was especially strong among African Americans, Whites, and Latinos (Supplemental Figures 6D and 9D, respectively). Others have shown an inverse association between alpha diversity and NAFLD.Citation14,Citation53,Citation54
Bacterial carbohydrate metabolism that may impact lipogenesis is altered in NAFLD.Citation10 Our findings suggest a pathophysiologic shift in the microbiome that supported lipogenesis in NAFLD. Increased carbohydrate metabolism was accompanied by the ethnic-specific enrichment of genera that ferment carbohydrates to acetateCitation55,Citation56 (Blautia in Japanese Americans, Escherichia-Shigella in African Americas and whites, and Klebsiella in whites) and an enrichment of enzymes carbohydrate metabolism by acetate producing Blautia, enriched with NAFLD in Japanese Americans with NAFLD (q < 0.01) (, Supplemental Figure 2). In contrast, there was a reduction in butyrate pathways and genera (Ruminococcus and Lachnospiraceae;Coprococcus) in whites with NAFLD as others have foundCitation9,Citation13,Citation57 (; Supplemental Table 5). Bacterially produced acetate impacts hepatic lipogenesis not only directly, as a fatty acid precursor, but indirectly as well.Citation58,Citation59 Lipoprotein lipase (LPL) is inhibited by fasting induced adipocyte factor (FIAF) that is produced in hepatocytes and when there is a shift in the gut bacteria that ferment carbohydrates to acetate, it increases lipid storage in the liver.Citation58–61
13Adults with NAFLD have altered bile acid metabolism as shown in small case control studies and in larger, although ethnically homogenous, cohort studies.Citation54,Citation62,Citation63 Imputed secondary bile acid synthesis pathways were significantly increased in all participants with NAFLD (; Supplemental Table 5). In an ethnic-specific manner, ALT, a clinical measure of liver dysfunction, was positively associated with secondary bile acid producing bacteria and support this microbial-driven pathophysiologic link to NAFLD (). Positive associations between ALT and certain genera have been noted in other studies.Citation10, 14 Secondary bile acids have been identified as antagonists of the farnesoid X receptor (FXR), involved in lipid metabolism and glucose homeostasis (reviewed inCitation64) and may lead to predisposition to liver injury and form the pathophysiological basis for clinical therapies.Citation65 Therefore, a GM that produces secondary bile acids could enhance lipid accumulation in the liver through reduced liver FXR activation. The R. gnavus group and Escherichia-Shigella have members that convert primary to secondary bile acids.Citation66 In our study, R. gnavus was positively associated with NAFLD in Latinos, Japanese Americans, and whites whereas Escherichia-Shigella, was significantly enriched in African Americans with NAFLD. Lachnoclostridium and Blautia, involved in the 7α-dehydroxylation of primary bile acids to secondary bile acids,Citation67–70 were positively associated with NAFLD overall (Supplemental Tables 1B), and in Latinos or Japanese Americans, respectively (). Enrichment of carbohydrate metabolizing enzymes in Lachnoclostridium was shown in Latinos (q = 0.03) (, Supplemental Figure 2). Microbial metabolism of taurine and glycine involved in the deconjugation of primary bile acids with NAFLD was enriched in Native Hawaiian womenCitation71–76 as was Parasutterella. This genus has been associated with secondary bile acid metabolism and reduction in tauro-conjugated bile acids in pre-clinical models.Citation77 Multiple unique genera impact bile acid metabolism and suggest that novel treatments such as FXR agonists may be altered in advanced disease across multiple ethnicities although currently this has only been tested in preclinical models.Citation78–80
Endotoxin (or lipopolysaccharide, LPS) from gram negative bacteria binds to LBP and activates toll-like receptor 4 (TLR4) in the liver, releasing pro-inflammatory cytokines and chemokines through the NFk-B inflammation cascade and may be an important contributor to the progression from NAFLD to inflammation-based liver disease.Citation52,Citation81–83 In individuals with NAFLD, we observed enrichment of endotoxin-producing bacteria in Enterobacteriaceae, Pasteurellaceae, and Veillonellaceae (Supplemental Table 1B), although different genera were positively associated with NAFLD in different ethnic groups (, Supplemental Table 1B). The abundance of Pasteurellaceae was a predictor of morality linked to acute-on-chronic liver failure in a longitudinal study of 42 Chinese subjects.Citation78 Additionally, we showed that overall systemic inflammation (as measured by CRP) was associated with NAFLD and, among Latinos, this was potentially mediated through the LBP pathway. Other investigators could differentiate mild/moderate NAFLD from advanced fibrosis with inflammation by an increase in endotoxin-producing β-Proteobacteria, especially Escherichia coli, Enterobacter, Aggregatibacter, and Klebsiella, as we found in Japanese Americans, African Americans, and whites.Citation11,Citation44 Additionally, Sookoian et al. showed that LPS was enriched in individuals with liver steatosis in LatinosCitation70 and we found Aggregatibacter, an endotoxin producing bacteria, was significantly more prevalent in Latinos with NAFLD (Supplemental Table 1B). Megamonas, a member of the Veilonellaceae that also produces endotoxin, was also significantly prevalent and positively associated with NAFLD in Native Hawaiians (Supplemental Table 1B) suggesting a novel pathway linked to systemic inflammation in NAFLD unique to this ethnic group.
Oral bacteria have been implicated in inflammation-based diseases (e.g., cardiovascular disease, T2D, colorectal cancer (CRC), and NASH).Citation84,Citation85 We observed a higher abundance Fusobacterium, Aggregatibacter, and Alloprevotella in participants presenting with NAFLD, which varied by ethnicity (). In our study, Alloprevotella was significantly increased in whites with NAFLD and others have Alloprevotella enriched in liver biopsies in subjects with NAFLD.Citation70 Aggregatibacter has been associated with NAFLD and altered glucose metabolism.Citation46,Citation47 Fusobacterium was significantly decreased in African Americans with NAFLD (, Supplemental Figure 4). In contrast, whites with NAFLD showed a significant enrichment in Fusobacterium and a higher percentage of whites had Fusobacterium in their stool (, Supplemental Figure 4). Fecal enrichment in stool of the oral pathogen Fusobacterium, has been associated with NAFLD and with inflammation and fibrosis in NASH.Citation12,Citation61,Citation86 The clinical relevance of Fusobacterium may be as an alterable prognostic marker linked to prevention through changes in periodontal and oral hygiene.Citation87
The GM from healthy individuals have high diversity bolstered by butyrate producing bacteria, a key energy source of gut epithelium.Citation88 In our study, the butyrate-producing genera of the Lachnospiraceae and Ruminococcaceae were diverse but these patterns were unique to different ethnic groups. The Bacteroides pectinophilus group, which degrade complex carbohydrates found in fiber, was significantly increased in Native Hawaiians, Japanese Americans, and African Americans without NAFLD. The Christensenellaceae R-7 group was depleted in NAFLD in African Americans but enriched in whites.Citation89 Several large human population studies have found Christensenella were enriched in healthy weight individuals and inversely associated with NAFLD.Citation90,Citation91 Christensenellaceae and members of this consortia has also been associated with a lean phenotype in humans and mouse models of obesity, a shift in SCFA metabolism,Citation92 and members of the Christensenellaceae are one of the most strongly heritable bacterial groups.Citation89,Citation93
A major strength of this study is the broad representation of ethnicity, sex, and BMI and corresponding well-curated metadata, including the state-of-the-art MRI measurement of liver fat used both to determine NAFLD status and to analyze as a continuous variable, comprehensive bacterial profiling, and metagenomic analysis. While our diverse study population supports the generalizability of the findings, given the older age of the individuals, the results may not be generalizable to younger individuals. We did not include medication use in our models which may independently alter NAFLD. Although some of our subjects with NAFLD may have been using metformin to control T2D,Citation94 a recent meta-analysis suggests that while weight and glucose control were improved with metformin, it did not substantially impact liver disease, studies suggest that metformin does not alter NAFLD status.Citation95 Additionally, subjects were excluded from the MEC APS if they had cancer and other bowel diseases. Therefore, subjects using chemotherapy drugs shown to impact NAFLD would have been excluded and reduce the probability of this bias.Citation95 In this study, we did not assess liver fibrosis that may have different associations with GM than steatosis. Although our results are suggestive of several candidate bacteria and pathways for NAFLD etiology, our single timepoint analysis does not provide strong causal inferences that the associated GM traits affect temporal changes in liver fat. Additionally, we had smaller sample sizes when stratified by ethnicity. Longitudinal studies are needed, especially in different ethnic groups, to establish the role of the microbiome in the development of fatty liver and the transition to more inflammatory and fibrotic liver diseases. Further, extending beyond our GM analysis, the application of metagenomic sequencing approaches that allow genomic reconstruction will identify different species and strains that may impact NAFLD.
We have observed that aspects of the GM composition and metabolism are associated with NAFLD, overall and in ethnic-specific manners among generally healthy older adults. Additionally, systemic inflammation may be mediated in part by the microbiome and varies by ethnicity. Microbial-associated mechanisms may provide insight into the development of NAFLD. Once replicated in other studies, ethnic-specific microbial composition and pathophysiologic pathways can provide the basis for targeted therapies, such as narrow spectrum antibiotics,Citation96 diet,Citation6,Citation97 fecal transplantsCitation98,Citation99 or phage therapies,Citation100 for future clinical treatment specific to the microbiome. Microbiome-mediated pathways may provide an actionable ethnic-specific target to reduce inflammation and reduce the transition from simple steatosis to advanced disease.
Ethics approval and consent to participate
The study protocol conformed to the ethical guidelines of the 1975 Declaration of Helsinki and Institutional Review Board approval was obtained from all participating institutions and signed informed consent was obtained from the study
Availability of data and materials
Sequencing files have been submitted to the SRA for public access (PRJNA629344).
Supplemental Material
Download Zip (4 MB)Disclosure statement
None of the authors reported any competing interests.
Supplemental data
Supplemental data for this paper can be accessed on the publisher’s website.
Additional information
Funding
References
- Kochanek KD, Murphy SL, Xu J, Deaths: T-VB. Final Data for 2014. Nat Vital Stat Rep. 2016;65:1–19.
- White DL, Thrift AP, Kanwal F, Davila J, El-Serag HB. Incidence of hepatocellular carcinoma in all 50 United States, from 2000 through 2012. Gastroenterology. 2017;152:812–20 e5. doi:10.1053/j.gastro.2016.11.020.
- Setiawan VW, Stram DO, Porcel J, Lu SC, Le Marchand L, Noureddin M. Prevalence of chronic liver disease and cirrhosis by underlying cause in understudied ethnic groups: the multiethnic cohort. Hepatology. 2016;64(1969–77):PMC5115980. doi:10.1002/hep.28677.
- Lim U, Monroe KR, Buchthal S, Fan B, Cheng I, Kristal BS, Lampe JW, Hullar MA, Franke AA, Stram DO, et al. Propensity for intra-abdominal and hepatic adiposity varies among ethnic groups. Gastroenterology. PMC6409195 2019;156:966–75e10. doi:10.1053/j.gastro.2018.11.021.
- Maskarinec G, Lim U, Jacobs S, Monroe KR, Ernst T, Buchthal SD, Shepherd JA, Wilkens LR, Le Marchand L, Boushey CJ. Diet quality in midadulthood predicts visceral adiposity and liver fatness in older ages: the Multiethnic Cohort Study. Obesity (Silver Spring). 2017;25(1442–50):PMC5604249. doi:10.1002/oby.21868.
- Bajaj JS, Idilman R, Mabudian L, Hood M, Fagan A, Turan D, White MB, Karakaya F, Wang J, Atalay R, et al. Diet affects gut microbiota and modulates hospitalization risk differentially in an international cirrhosis cohort. Hepatology. 2018;68:234–247. doi:10.1002/hep.29791.
- Wang X, Liu Z, Wang K, Wang Z, Sun X, Zhong L, Deng G, Song G, Sun B, Peng Z, et al. Additive effects of the risk alleles of PNPLA3 and TM6SF2 on non-alcoholic fatty liver disease (NAFLD) in a Chinese population. Front Genet. 2016;7:140. doi:10.3389/fgene.2016.00140.
- Park SL, Li Y, Sheng X, Hom V, Xia L, Zhao K, Pooler L, Setiawan VW, Lim U, Monroe KR, et al. Genome-wide association study of liver fat: the multiethnic cohort adiposity phenotype study. Hepatol Comm. 2020;4(1112–23):PMC7395069. doi:10.1002/hep4.1533.
- Raman M, Ahmed I, Gillevet PM, Probert CS, Ratcliffe NM, Smith S, Greenwood R, Sikaroodi M, Lam V, Crotty P, et al. Fecal microbiome and volatile organic compound metabolome in obese humans with nonalcoholic fatty liver disease. Clin Gastroenterol Hepatol. 2013;11(868–75):e1–3. doi:10.1016/j.cgh.2013.02.015.
- Boursier J, Mueller O, Barret M, Machado M, Fizanne L, Araujo-Perez F, Guy CD, Seed PC, Rawls JF, David LA, et al. The severity of nonalcoholic fatty liver disease is associated with gut dysbiosis and shift in the metabolic function of the gut microbiota. Hepatology. 2016;63:764–775. doi:10.1002/hep.28356.
- Loomba R, Seguritan V, Li W, Long T, Klitgord N, Bhatt A, Dulai PS, Caussy C, Bettencourt R, Highlander SK, et al. Gut microbiome-based metagenomic signature for non-invasive detection of advanced fibrosis in human nonalcoholic fatty liver disease. Cell Metab. 2017;25:1054–62 e5. doi:10.1016/j.cmet.2017.04.001.
- Shen F, Zheng R-D, Sun X-Q, Ding W-J, Wang X-Y, Fan J-G, Shen F, Zheng RD, Sun XQ, Ding WJ, Wang XY, Fan JG. Gut microbiota dysbiosis in patients with non-alcoholic fatty liver disease. Hepatob Pancreat Dis Int. 2017;16:375–381. 10.1016/S1499-3872(17)60019-5.
- Zhu LX, Baker SS, Gill C, Liu WS, Alkhouri R, Baker RD, Gill SR. Characterization of gut microbiomes in Nonalcoholic Steatohepatitis (NASH) Patients: a connection between endogenous alcohol and NASH. Hepatology. 2013;57:601–609. doi:10.1002/hep.26093.
- Wang B, Jiang X, Cao M, Ge J, Bao Q, Tang L, Chen Y, Li L. Altered fecal microbiota correlates with liver biochemistry in nonobese patients with non-alcoholic fatty liver disease. Sci Rep. 2016;6:32002. doi:10.1038/srep32002.
- Hoyles L, Fernandez-Real JM, Federici M, Serino M, Abbott J, Charpentier J, Heymes C, Luque JL, Anthony E, Barton RH, et al. Molecular phenomics and metagenomics of hepatic steatosis in non-diabetic obese women. Nat Med. 2018;24:1070–1080. doi:10.1038/s41591-018-0061-3.
- Pan JJ, Fallon MB. Gender and racial differences in nonalcoholic fatty liver disease. World J Hepatol. 2014;6:274–283. doi:10.4254/wjh.v6.i5.274.
- Trebicka J, Bork P, Krag A, Arumugam M. Utilizing the gut microbiome in decompensated cirrhosis and acute-on-chronic liver failure. Nat Rev Gastroenterol Hepatol. 2021;18:167–180. doi:10.1038/s41575-020-00376-3.
- Kolonel LN, Henderson BE, Hankin JH, Nomura AMY, Wilkens LR, Pike MC, Stram DO, Monroe KR, Earle ME, Nagamine FS. A multiethnic cohort in Hawaii and Los Angeles: baseline characteristics. Am J Epidemiol. 2000;151:346–357. doi:10.1093/oxfordjournals.aje.a010213.
- Harmon BE, Boushey CJ, Shvetsov YB, Ettienne R, Reedy J, Wilkens LR, Le Marchand L, Henderson BE, Kolonel LN. Associations of key diet-quality indexes with mortality in the multiethnic cohort: the dietary patterns methods project. Am J Clin Nutr. 2015;101:587–597. doi:10.3945/ajcn.114.090688.
- Guenther PM, Kirkpatrick SI, Reedy J, Krebs-Smith SM, Buckman DW, Dodd KW, Casavale KO, Carroll RJ. The Healthy Eating Index-2010 is a valid and reliable measure of diet quality according to the 2010 Dietary Guidelines for Americans. J Nutr. 2014;144:399–407. doi:10.3945/jn.113.183079.
- Guiu B, Loffroy R, Petit JM, Aho S, Ben Salem D, Masson D, Hillon P, Cercueil JP, Krause D. Mapping of liver fat with triple-echo gradient echo imaging: validation against 3.0-T proton MR spectroscopy. Eur Radiol. 2009;19:1786–1793. doi:10.1007/s00330-009-1330-9.
- Rinella ME. Nonalcoholic fatty liver disease: a systematic review. JAMA. 2015;313:2263–2273. doi:10.1001/jama.2015.5370.
- Le Marchand L, Wilkens LR, Castelfranco AM, Monroe KR, Kristal BS, Cheng I, Maskarinec G, Hullar MA, Lampe JW, Shepherd JA, et al. Circulating biomarker score for visceral fat and risks of incident colorectal and postmenopausal breast cancer: the multiethnic cohort adiposity phenotype study. Cancer Epidemiol Biomarkers Prev. 2020;29(966–73):PMC7196505. doi:10.1158/1055-9965.EPI-19-1469.
- Fu BC, Randolph TW, Lim U, Monroe KR, Cheng I, Wilkens LR, Le Marchand L, Hullar MA, Lampe JW. Characterization of the gut microbiome in epidemiologic studies: the multiethnic cohort experience. Ann Epidemiol. 2016;26(373–9):PMC4892953. doi:10.1016/j.annepidem.2016.02.009.
- Fu BC, Randolph TW, Lim U, Monroe KR, Cheng I, Wilkens LR, Le Marchand L, Lampe JW, Hullar MAJ. Temporal variability and stability of the fecal microbiome: the Multiethnic Cohort Study. Cancer Epidemiol Biomarkers Prev. 2019;28(154–62):PMC6625800. doi:10.1158/1055-9965.EPI-18-0348.
- Caporaso JG, Kuczynski J, Stombaugh J, Bittinger K, Bushman FD, Costello EK, Fierer N, Pena AG, Goodrich JK, Gordon JI, et al. QIIME allows analysis of high-throughput community sequencing data. Nat Methods. 2010;7:335–336. doi:10.1038/nmeth.f.303.
- Langille MGI, Zaneveld J, Caporaso JG, McDonald D, Knights D, Reyes JA, Clemente JC, Burkepile DE, Thurber RLV, Knight R, et al. Predictive functional profiling of microbial communities using 16S rRNA marker gene sequences. Nat Biotechnol. 2013;31:814–821. doi:10.1038/nbt.2676.
- Bolger AM, Lohse M, Usadel B. Trimmomatic: a flexible trimmer for Illumina sequence data. Bioinformatics. 2014;30:2114–2120. doi:10.1093/bioinformatics/btu170.
- Abubucker S, Segata N, Goll J, Schubert AM, Izard J, Cantarel BL, Rodriguez-Mueller B, Zucker J, Thiagarajan M, Henrissat B, et al. Metabolic reconstruction for metagenomic data and its application to the human microbiome. PLoS Comput Biol. 2012;8:e1002358. doi:10.1371/journal.pcbi.1002358.
- Buchfink B, Xie C, Huson DH. Fast and sensitive protein alignment using DIAMOND. Nat Methods. 2015;12:59–60. doi:10.1038/nmeth.3176.
- Suzek BE, Wang YQ, Huang HZ, McGarvey PB, Wu CH, Consortium U. UniRef clusters: a comprehensive and scalable alternative for improving sequence similarity searches. Bioinformatics. 2015;31:926–932. doi:10.1093/bioinformatics/btu739.
- Caspi R, Billington R, Ferrer L, Foerster H, Fulcher CA, Keseler IM, Kothari A, Krummenacker M, Latendresse M, Mueller LA, et al. The MetaCyc database of metabolic pathways and enzymes and the BioCyc collection of pathway/genome databases. Nucleic Acids Res. 2016;44:D471–80. doi:10.1093/nar/gkv1164.
- Vital M, Howe AC, Tiedje JM. Revealing the bacterial butyrate synthesis pathways by analyzing (meta)genomic data. MBio. 2014;5:e00889. doi:10.1128/mBio.00889-14.
- Yilmaz P, Kottmann R, Field D, Knight R, Cole JR, Amaral-Zettler L, Gilbert JA, Karsch-Mizrachi I, Johnston A, Cochrane G, et al. Minimum information about a marker gene sequence (MIMARKS) and minimum information about any (x) sequence (MIxS) specifications. Nat Biotechnol. 2011;29:415–420. doi:10.1038/nbt.1823.
- Zhang Y, Parmigiani G, Johnson WE.;ComBat-seq: batch effect adjustment for RNA-seq count data. NAR Genom Bioinf. 2020;2. doi:10.1093/nargab/lqaa078
- Johnson WE, Li C, Rabinovic A. Adjusting batch effects in microarray expression data using empirical Bayes methods. Biostatistics. 2007;8:118–127. doi:10.1093/biostatistics/kxj037.
- Benjamini Y, Hochberg Y. Controlling the false discovery rate - a practical and powerful approach to multiple testing. J Roy Stat Soc B Met. 1995;57:289–300.
- Anderson MJ. A new method for non-parametric multivariate analysis of variance. Austral Ecol. 2001;26:32–46.
- Lozupone C, Hamady M, Knight R. UniFrac–an online tool for comparing microbial community diversity in a phylogenetic context. BMC Bioinform. 2006;7:371. doi:10.1186/1471-2105-7-371.
- Martin BD, Witten D, Willis AD. Modeling microbial abundances and dysbiosis with beta-binomial regression. Ann Appl Stat. 2020;14:94–115. doi:10.1214/19-AOAS1283.
- Quinn TP, Erb I, Gloor G, Notredame C, Richardson MF, Crowley TM. A field guide for the compositional analysis of any-omics data. Gigascience. 2019:8. doi:10.1093/gigascience/giz107.
- Tingley D, Yamamoto T, Hirose K, Keele L, Imai K. Mediation: r package for causal mediation analysis. J Stat Softw. 2014; 59. 10.18637/jss.v059.i05.
- Preacher KJ, Hayes AF. Asymptotic and resampling strategies for assessing and comparing indirect effects in multiple mediator models. Behav Res Methods. 2008;40:879–891. doi:10.3758/BRM.40.3.879.
- Fei N, Bruneau A, Zhang X, Wang R, Wang J, Rabot S, Gerard P, Zhao L. Endotoxin producers overgrowing in human gut microbiota as the causative agents for nonalcoholic fatty liver disease. mBio. 2020;11(1):e03263-19. doi: 10.1128/mBio.03263-19.
- Gholizadeh P, Pormohammad A, Eslami H, Shokouhi B, Fakhrzadeh V, Kafil HS. Oral pathogenesis of Aggregatibacter actinomycetemcomitans.Microb Pathog. 2017;113:303–311. doi:10.1016/j.micpath.2017.11.001.
- Iwasaki T, Hirose A, Azuma T, Ohashi T, Watanabe K, Obora A, Deguchi F, Kojima T, Isozaki A, Tomofuji T. Correlation between ultrasound-diagnosed non-alcoholic fatty liver and periodontal condition in a cross-sectional study in Japan. Sci Rep. 2018;8:7496. doi:10.1038/s41598-018-25857-z.
- Komazaki R, Katagiri S, Takahashi H, Maekawa S, Shiba T, Takeuchi Y, Kitajima Y, Ohtsu A, Udagawa S, Sasaki N, et al. Periodontal pathogenic bacteria, Aggregatibacter actinomycetemcomitans affect non-alcoholic fatty liver disease by altering gut microbiota and glucose metabolism. Sci Rep. 2017;7:13950. doi:10.1038/s41598-017-14260-9.
- Gaggini M, Carli F, Rosso C, Buzzigoli E, Marietti M, Della Latta V, Ciociaro D, Abate ML, Gambino R, Cassader M, et al. Altered amino acid concentrations in NAFLD: impact of obesity and insulin resistance. Hepatology. 2018;67:145–158. doi:10.1002/hep.29465.
- Schwiertz A, Taras D, Schafer K, Beijer S, Bos NA, Donus C, Hardt PD. Microbiota and SCFA in lean and overweight healthy subjects. Obesity. 2010;18:190–195. doi:10.1038/oby.2009.167.
- VanderWeele TJ. Mediation analysis: a practitioner’s guide. Annu Rev Public Health. 2016;37:17–32. doi:10.1146/annurev-publhealth-032315-021402.
- Tsuchida T, Friedman SL. Mechanisms of hepatic stellate cell activation. Nat. Rev. Gastroenterol. Hepatol. 2017;14:397–411. doi:10.1038/nrgastro.2017.38.
- Lee G, You HJ, Bajaj JS, Joo SK, Yu J, Park S, Kang H, Park JH, Kim JH, Lee DH, et al. Distinct signatures of gut microbiome and metabolites associated with significant fibrosis in non-obese NAFLD. Nat Commun. 2020;11:4982. doi:10.1038/s41467-020-18754-5.
- Yun Y, Kim HN, Lee EJ, Ryu S, Chang Y, Shin H, Kim HL, Kim TH, Yoo K, Kim HY. Fecal and blood microbiota profiles and presence of nonalcoholic fatty liver disease in obese versus lean subjects. PLoS One. 2019;14:e0213692. doi:10.1371/journal.pone.0213692.
- Ruuskanen MO, Aberg F, Mannisto V, Havulinna AS, Meric G, Liu Y, Loomba R, Vazquez-Baeza Y, Tripathi A, Valsta LM, et al. Links between gut microbiome composition and fatty liver disease in a large population sample. Gut Microbes. 2021;13:1–22. doi:10.1080/19490976.2021.1888673.
- Rios-Covian D, Ruas-Madiedo P, Margolles A, Gueimonde M, De Los Reyes-gavilan CG, Salazar N. Intestinal short chain fatty acids and their link with diet and human health. Front Microbiol. 2016;7:185. doi:10.3389/fmicb.2016.00185.
- Neijssel OM, Tempest DW. The regulation of carbohydrate metabolism in Klebsiella aerogenes NCTC 418 organisms, growing in chemostat culture. Arch Microbiol. 1975;106:251–258. doi:10.1007/BF00446531.
- Wong VW, Tse CH, Lam TT, Wong GL, Chim AM, Chu WC, Yeung DK, Law PT, Kwan HS, Yu J, et al. Molecular characterization of the fecal microbiota in patients with nonalcoholic steatohepatitis–a longitudinal study. PLoS One. 2013;8:e62885. doi:10.1371/journal.pone.0062885.
- Bäckhed F, Ding H, Wang T, Hooper LV, Koh GY, Nagy A, Semenkovich CF, Gordon JI. The gut microbiota as an environmental factor that regulates fat storage. Proc Natl Acad Sci. 2004;101(44):15718–15723. doi:10.1073/pnas.0407076101.
- Alex S, Lange K, Amolo T, Grinstead JS, Haakonsson AK, Szalowska E, Koppen A, Mudde K, Haenen D, Al-Lahham S, et al. Short-chain fatty acids stimulate angiopoietin-like 4 synthesis in human colon adenocarcinoma cells by activating peroxisome proliferator-activated receptor gamma. Mol Cell Biol. 2013;33:1303–1316. doi:10.1128/MCB.00858-12.
- Kohjima M, Higuchi N, Kato M, Kotoh K, Yoshimoto T, Fujino T, Yada M, Yada R, Harada N, Enjoji M, et al. SREBP-1c, regulated by the insulin and AMPK signaling pathways, plays a role in nonalcoholic fatty liver disease. Int J Mol Med. 2008;21:507–511.
- Rau M, Rehman A, Dittrich M, Groen AK, Hermanns HM, Seyfried F, Beyersdorf N, Dandekar T, Rosenstiel P, Geier A. Fecal SCFAs and SCFA-producing bacteria in gut microbiome of human NAFLD as a putative link to systemic T-cell activation and advanced disease. United Eur Gastroent. 2018;6:1496–1507. doi:10.1177/2050640618804444.
- Janssen AWF, Houben T, Katiraei S, Dijk W, Boutens L, Van Der Bolt N, Wang ZN, Brown JM, Hazen SL, Mandard S, et al. Modulation of the gut microbiota impacts nonalcoholic fatty liver disease: a potential role for bile acids. J Lipid Res. 2017;58:1399–1416. doi:10.1194/jlr.M075713.
- Alferink LJ, Radjabzadeh D, Erler NS, Vojinovic D, Medina-Gomez C, Uitterlinden AG, Knegt RJ, Amin N, Ikram MA, Janssen H, et al.Microbiomics, metabolomics, predicted metagenomics and hepatic steatosis in a population-based study of 1355 adults. Hepatology. 2020;73(3)968-982. doi.10.1002/hep.31417.
- Utzschneider KM, Kratz M, Damman CJ, Hullar M. Mechanisms linking the gut microbiome and glucose metabolism. J Clin Endocrinol Metab. 2016;101:1445–1454. doi:10.1210/jc.2015-4251.
- Albillos A, de Gottardi A, Rescigno M. The gut-liver axis in liver disease: pathophysiological basis for therapy. J Hepatol. 2020;72:558–577.
- Lee JY, Arai H, Nakamura Y, Fukiya S, Wada M, Yokota A. Contribution of the 7beta-hydroxysteroid dehydrogenase from Ruminococcus gnavus N53 to ursodeoxycholic acid formation in the human colon. J Lipid Res. 2013;54:3062–3069.
- Hirano S, Nakama R, Tamaki M, Masuda N, Oda H. Isolation and characterization of thirteen intestinal microorganisms capable of 7 alpha-dehydroxylating bile acids. Appl Environ Microbiol. 1981;41:737–745.
- Ridlon JM, Harris SC, Bhowmik S, Kang DJ, Hylemon PB. Consequences of bile salt biotransformations by intestinal bacteria. Gut Microbes. 2016;7:22–39. doi:10.1080/19490976.2015.1127483.
- Ridlon JM, Kang DJ, Hylemon PB. Isolation and characterization of a bile acid inducible 7alpha-dehydroxylating operon in Clostridium hylemonae TN271.Anaerobe. 2010;16:137–146. doi:10.1016/j.anaerobe.2009.05.004.
- Sookoian S, Salatino A, Castano GO, Landa MS, Fijalkowky C, Garaycoechea M, Pirola CJ. Intrahepatic bacterial metataxonomic signature in non-alcoholic fatty liver disease. Gut. 2020;69(8):1483–1491. doi:10.1136/gutjnl-2019-318811.
- Guo C, Xie C, Ding P, Qin G, Mo W, Cao X, Zheng S. Quantification of glycocholic acid in human serum by stable isotope dilution ultra performance liquid chromatography electrospray ionization tandem mass spectrometry. J Chromatogr B Analyt Technol. Biomed Life Sci. 2018;1072:315–319. doi:10.1016/j.jchromb.2017.11.037.
- Pedersen HK, Gudmundsdottir V, Nielsen HB, Hyotylainen T, Nielsen T, Jensen BA, Forslund K, Hildebrand F, Prifti E, Falony G, et al. Human gut microbes impact host serum metabolome and insulin sensitivity. Nature. 2016;535:376–381. doi:10.1038/nature18646.
- Shoaie S, Ghaffari P, Kovatcheva-Datchary P, Mardinoglu A, Sen P, Pujos-Guillot E, de Wouters T, Juste C, Rizkalla S, Chilloux J, et al. Quantifying diet-induced metabolic changes of the human gut microbiome. Cell Metab. 2015;22:320–331. doi:10.1016/j.cmet.2015.07.001.
- Dey N, Wagner VE, Blanton LV, Cheng JY, Fontana L, Haque R, Ahmed T, Gordon JI. Regulators of gut motility revealed by a gnotobiotic model of diet-microbiome interactions related to travel. Cell. 2015;163:95–107. doi:10.1016/j.cell.2015.08.059.
- Staley C, Kelly CR, Brandt LJ, Khoruts A, Sadowsky MJ. Complete microbiota engraftment is not essential for recovery from recurrent Clostridium difficile infection following fecal microbiota transplantation. mBio. 2016:7. doi:10.1128/mBio.01965-16.
- Llopis M, Cassard AM, Wrzosek L, Boschat L, Bruneau A, Ferrere G, Puchois V, Martin JC, Lepage P, Le Roy T, et al. Intestinal microbiota contributes to individual susceptibility to alcoholic liver disease. Gut. 2016;65:830–839. doi:10.1136/gutjnl-2015-310585.
- Ju T, Kong JY, Stothard P, Willing BP. Defining the role of Parasutterella, a previously uncharacterized member of the core gut microbiota. ISME J. 2019;13(6):1520–1534. doi:10.1038/s41396-019-0364-5.
- Verbeke L, Farre R, Verbinnen B, Covens K, Vanuytsel T, Verhaegen J, Komuta M, Roskams T, Chatterjee S, Annaert P, et al. The FXR agonist obeticholic acid prevents gut barrier dysfunction and bacterial translocation in cholestatic rats. Am J Pathol. 2015;185:409–419. doi:10.1016/j.ajpath.2014.10.009.
- Ubeda M, Lario M, Munoz L, Borrero MJ, Rodriguez-Serrano M, Sanchez-Diaz AM, Del Campo R, Lledo L, Pastor O, Garcia-Bermejo L, et al. Obeticholic acid reduces bacterial translocation and inhibits intestinal inflammation in cirrhotic rats. J Hepatol. 2016;64:1049–1057. doi:10.1016/j.jhep.2015.12.010.
- Sorribas M, Jakob MO, Yilmaz B, Li H, Stutz D, Noser Y, de Gottardi A, Moghadamrad S, Hassan M, Albillos A, et al. FXR modulates the gut-vascular barrier by regulating the entry sites for bacterial translocation in experimental cirrhosis. J Hepatol. 2019;71:1126–1140. doi:10.1016/j.jhep.2019.06.017.
- Pradere JP, Troeger JS, Dapito DH, Mencin AA, Schwabe RF. Toll-like receptor 4 and hepatic fibrogenesis. Semin Liver Dis. 2010;30:232–244. doi:10.1055/s-0030-1255353.
- Citronberg JS, Curtis KR, White E, Newcomb PA, Newton K, Atkinson C, Song X, Lampe JW, Hullar MA. Association of gut microbial communities with plasma lipopolysaccharide-binding protein (LBP) in premenopausal women. ISME J. 2018;12(7):1631–1641. PMC6018759. doi:10.1038/s41396-018-0064-6.
- Dapito DH, Mencin A, Gwak GY, Pradere JP, Jang MK, Mederacke I, Caviglia JM, Khiabanian H, Adeyemi A, Bataller R, et al. Promotion of hepatocellular carcinoma by the intestinal microbiota and TLR4. Cancer Cell. 2012;21:504–516. doi:10.1016/j.ccr.2012.02.007.
- Bui FQ, CLC A-D-S, Huynh B, Trinh A, Liu J, Woodward J, Asadi H, Ojcius DM. Association between periodontal pathogens and systemic disease. Biomed J. 2019;42:27–35. doi:10.1016/j.bj.2018.12.001.
- Mancabelli L, Milani C, Lugli GA, Turroni F, Cocconi D, van Sinderen D, Ventura M. Identification of universal gut microbial biomarkers of common human intestinal diseases by meta-analysis. FEMS Microbiol Ecol. 2017;93(12). doi.10.1093/femsec/fix153.
- Bullman S, Pedamallu CS, Sicinska E, Clancy TE, Zhang X, Cai D, Neuberg D, Huang K, Guevara F, Nelson T, et al. Analysis of Fusobacterium persistence and antibiotic response in colorectal cancer. Science. 2017;358:1443–1448. doi:10.1126/science.aal5240.
- Bajaj JS, Matin P, White MB, Fagan A, Deeb JG, Acharya C, Dalmet SS, Sikaroodi M, Gillevet PM, Sahingur SE. Periodontal therapy favorably modulates the oral-gut-hepatic axis in cirrhosis. Am J Physiol Gastrointest Liver Physiol. 2018;315:G824–G37. doi:10.1152/ajpgi.00230.2018.
- McBurney MI, Davis C, Fraser CM, Schneeman BO, Huttenhower C, Verbeke K, Walter J, Latulippe ME. Establishing what constitutes a healthy human gut microbiome: state of the science, regulatory considerations, and future directions. J Nutr. 2019;149:1882–1895. doi:10.1093/jn/nxz154.
- Goodrich JK, Waters JL, Poole AC, Sutter JL, Koren O, Blekhman R, Beaumont M, Van Treuren W, Knight R, Bell JT, et al. Human genetics shape the gut microbiome. Cell. 2014;159:789–799. doi:10.1016/j.cell.2014.09.053.
- Konikoff T, Gophna U. Oscillospira: a central, enigmatic component of the human gut microbiota. Trends Microbiol. 2016;24:523–524. doi:10.1016/j.tim.2016.02.015.
- Peters BA, Shapiro JA, Church TR, Miller G, Trinh-Shevrin C, Yuen E, Friedlander C, Hayes RB, Ahn J. A taxonomic signature of obesity in a large study of American adults. Sci Rep-Uk. 2018;8: DOI ARTN 9749. doi:10.1038/s41598-018-28126-1.
- Ruaud A, Esquivel-Elizondo S, de la Cuesta-Zuluaga J, Waters JL, Angenent LT, Youngblut ND, Ley RE. Syntrophy via interspecies H2 transfer between Christensenella and Methanobrevibacter underlies their global cooccurrence in the human gut. mBio. 2020;11(1):03235019. doi.10.1128/mBio.03235-19
- Beaumont M, Goodrich J, Jackson M, Yet I, Davenport E, Vieira-Silva S, Debelius J, Pallister T, Mangino M, Raes J, et al. Heritable components of the human fecal microbiome are associated with visceral fat. Genome Biol. 2016;17:189. doi:10.1186/s13059-016-1052-7.
- Maskarinec G, Raquinio P, Kristal B, Wilkens LR, Franke A, Lim U, Le Marchand L, Lampe JW, Hullar MAJ. The gut microbiome and diabetes status in the Multiethnic Cohort. Curr Dev Nutr. 2020;4:1450. doi:10.1093/cdn/nzaa061_078.
- Blazina I, Selph S. Diabetes drugs for nonalcoholic fatty liver disease: a systematic review. Syst Rev. 2019;8:295. doi:10.1186/s13643-019-1200-8.
- Melander RJ, Zurawski DV, Melander C. Narrow-spectrum antibacterial agents. Medchemcomm. 2018;9:12–21. doi:10.1039/C7MD00528H.
- Dhiman RK, Rana B, Agrawal S, Garg A, Chopra M, Thumburu KK, Khattri A, Malhotra S, Duseja A, Chawla YK. Probiotic VSL#3 reduces liver disease severity and hospitalization in patients with cirrhosis: a randomized, controlled trial. Gastroenterology. 2014;147:1327–37 e3. doi:10.1053/j.gastro.2014.08.031.
- Wortelboer K, Nieuwdorp M, Herrema H. Fecal microbiota transplantation beyond Clostridioides difficile infections. EBioMedicine. 2019;44:716–729. doi:10.1016/j.ebiom.2019.05.066.
- Witjes JJ, Smits LP, Pekmez CT, Prodan A, Meijnikman AS, Troelstra MA, Bouter KEC, Herrema H, Levin E, Holleboom AG, et al. Donor fecal microbiota transplantation alters gut microbiota and metabolites in obese individuals with steatohepatitis. Hepatol Commun. 2020;4:1578–1590. doi:10.1002/hep4.1601.
- Duan Y, Llorente C, Lang S, Brandl K, Chu H, Jiang L, White RC, Clarke TH, Nguyen K, Torralba M, et al. Bacteriophage targeting of gut bacterium attenuates alcoholic liver disease. Nature. 2019;575:505–511. doi:10.1038/s41586-019-1742-x.