ABSTRACT
Long-term sequelae of coronavirus disease (COVID)-19 are frequent and of major concern. Severe acute respiratory syndrome coronavirus 2 (SARS-CoV-2) infection affects the host gut microbiota, which is linked to disease severity in patients with COVID-19. Here, we report that the gut microbiota of post-COVID subjects had a remarkable predominance of Enterobacteriaceae strains with an antibiotic-resistant phenotype compared to healthy controls. Additionally, short-chain fatty acid (SCFA) levels were reduced in feces. Fecal transplantation from post-COVID subjects to germ-free mice led to lung inflammation and worse outcomes during pulmonary infection by multidrug-resistant Klebsiella pneumoniae. transplanted mice also exhibited poor cognitive performance. Overall, we show prolonged impacts of SARS-CoV-2 infection on the gut microbiota that persist after subjects have cleared the virus. Together, these data demonstrate that the gut microbiota can directly contribute to post-COVID sequelae, suggesting that it may be a potential therapeutic target.
Introduction
The newly emerged β-coronavirus, severe acute respiratory syndrome-coronavirus-2 (SARS-CoV-2), has caused more than 618 million confirmed cases and 6,8 million deaths globally as of March 2023.Citation1 After the clearance of SARS-CoV-2, long-term complications of coronavirus disease-2019 (COVID-19) are common even among patients with mild or asymptomatic disease during the acute phase.Citation2–4 SARS-CoV-2 requires the angiotensin-converting enzyme 2 (ACE2) and Transmembrane Serine Protease 2 (TMPRSS2) to infect lung cells.Citation5 The gastrointestinal tract expresses high levels of these receptors and is also vulnerable.Citation6,Citation7 Gut microbiota has been shown to influence COVID-19 severity and long-term post-COVID effects.Citation7,Citation8
The human gut microbiome includes trillions of microorganisms, primarily bacteria, which form a complex and well-recognized ecosystem. An imbalance in the gut microbiota composition, referred to as dysbiosis, is a major factor in disease development and can be caused by viral infections and other respiratory challenges.Citation8–11 Individuals who experience severe COVID-19 have reduced diversity and abundance of the commensal gut microbiota.Citation9,Citation12,Citation13 Dysbiosis of the gut microbiota was observed up to 1 year after the initial infection and virus clearance post-COVID.Citation12 Thus, these long-term changes in the gut microbiota could contribute to the symptoms of long-COVID, but there is currently no direct evidence for this link.Citation14,Citation15
Here, we investigated whether the gut microbiota derived from individuals previously infected with SARS-CoV-2, who had mild or no symptoms could induce post-COVID consequences. To investigate this, we transferred human fecal microbiota to germ-free mice as an experimental approach. Our data provide evidence that gut microbiota from COVID-19 patients can cause sequelae from infection in the absence of SARS-CoV-2, including the induction of lung inflammation and brain dysfunction.
Results
Comparison of clinical, dietary, and microbiological parameters between controls (non-COVID) and post-COVID subjects
We recruited 131 volunteers: seventy-two (55%) subjects had SARS-CoV-2 infection (post-COVID group), and fifty-nine (45%) subjects were COVID-19-naïve healthy control subjects (). The experimental design and clinical characteristics of all the subjects are summarized in Figure S1 and , respectively. Among the post-COVID subjects: sixty-four (89%) had mild/moderate illness during the symptomatic period, and feces were collected between 1 and 4 months after initial symptoms. The exact time for samples collected from infection was summarized in Supplementary Table S1. Additionally, thirty-one (48%) post-COVID subjects reported experiencing gastrointestinal symptoms during SARS-CoV-2 infection (). Eight volunteers (11%) had confirmed exposure to SARS-CoV-2 by serological methods (when the samples were collected when vaccines were unavailable) but were asymptomatic. Factors affecting gut microbiota between the two groups, including preexisting comorbidities such as hypertension, hypothyroidism, irritable bowel disease, and chronic respiratory diseases, were similar between post-COVID individuals and controls (). Dietary habits were also analyzed () and show similarity between the groups (). The use of antibiotics (three months before and during SARS-CoV-2 infection) was reported in 24 (33%) post-COVID subjects and 7 (12%) control subjects, with significant differences between both groups (). Notably, all fecal samples tested negative for SARS-CoV-2 nucleic acid by RT-qPCR at the time of collection ().
Figure 1. Clinical characteristics and food habits were associated with gut microbiota composition and an antimicrobial resistance profile in Enterobacteriaceae species of post-COVID and control human subjects. (a) experimental design: collection of feeding habits, clinic survey, and fecal microbiota composition analysis of 59 control and 72 post-COVID subjects (N = 131). (b) Co-morbidities in control and post-COVID groups. (c) feeding composition (N = 131). (d) antibiotic-treated control and post-COVID subjects (N = 131). (e) SARS-CoV-2 quantification by RT-qPCR in the feces of control and post-COVID subjects, a.U.: arbitrary units (N = 131). (f) 16S rRNA sequencing of gut microbiota from control and post-COVID subjects at the family level (N = 44). Principal Component analysis based on weighted Unifrac distances (p = 0.900), a β-diversity index (N = 44). α-diversity analysis based on Shannon, Simpson, and Chao1 indexes (N = 44). (g) Enterobacteriaceae quantification in fecal samples of the subjects (N = 131). (H) Frequency of Enterobacteriaceae strains present in the fecal samples of human subjects as multidrug-resistant, resistant, or nonresistance (N = 131). Statistical analysis: Fisher’s exact test was used in D, Wilcoxon and PerMANOVA pairwise tests were used in F, unpaired Student’s t-test was used in G, and Chi-square test was used in H. Data are shown as mean and standard deviation (SD). See also Figure S1.
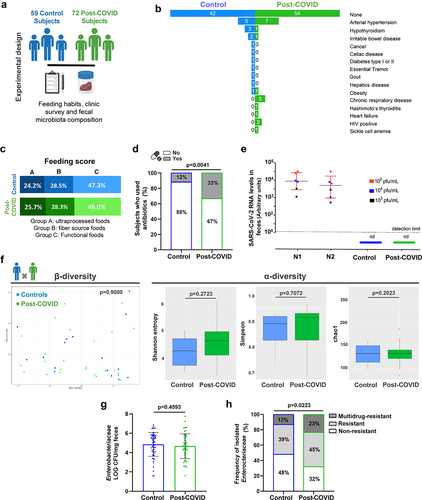
Table 1. Clinical and demographic characteristics of all human subjects in the study.
Table 2. Food characteristics used for the feeding score.
Gut microbiota composition was analyzed by 16S sequencing in 44 fecal samples, prioritizing the sampling of paired subjects from the same household (15 families) see samples workflow in (Figure S1B). We attempted to sample paired subjects from the same family because human-associated microbiota communities vary across individuals, but cohabiting family members share a similar microbiota.Citation16,Citation17 Analysis of the gut microbiota revealed similar profiles between individual samples from controls and post-COVID subjects (). β-Diversity (weighted UniFrac distances) and α-diversity metrics (Shannon, Simpson, and Chao1 indices) showed no significant differences in the gut microbiota between the groups, indicating similar taxonomic diversity ().
To explore the possible impact of SARS-CoV-2 infection on the gut microbiota beyond its composition, we evaluated the frequency of cultivable Enterobacteriaceae – due to their importance as pathobionts that serve as a reservoir of antimicrobial resistance genes of clinical interest, comparing the total number of colony-forming units (CFU) in the feces between controls and post-COVID subjects. Despite similar CFU numbers between the two groups (), post-COVID subjects had a higher percentage of Enterobacteriaceae strains with drug-resistant (45% DR) and multidrug-resistant (23% MDR) phenotypes when compared to controls (39% DR and 13% MDR) (). We identified an increased prevalence of antimicrobial resistance (AMR) Klebsiella spp. among all Enterobacteriaceae strains assessed in post-COVID subjects compared to controls (Figure S1C). The Klebsiella strains were predominantly resistant to quinolones (64%), aminoglycosides (100%), and sulfonamides (91%) (data not shown), which were not associated with intrinsic resistance in this bacterial genus. Overall, these findings show increased AMR in the Enterobacteriaceae community of the gut microbiota post-COVID, which might be partly due to the increased overuse of antibiotics treatment in this subject group but could also be a direct effect of SARS-CoV-2 infection, although the mechanisms remain unclear.
Post-COVID microbiota-induced alterations in the gut of microbiota humanized mice
To understand the direct contribution of post-COVID microbiota to the host, we performed human fecal microbiota transplantation (FMT) in germ-free mice. Fecal samples were harvested from controls and post-COVID volunteers (), prioritizing samples belonging to the same family (co-housing human fecal samples) (Figure S1B) to minimize differences in the gut microbiota caused by other environmental factors. FMT was performed for each human microbiota donor transfer to individual germ-free mice (Figure S2A). After FMT, mice were housed for 10–12 days to stabilize the human-derived microbiota. This protocol was based on a previous study by our group, where we observed reversal hyporesponsiveness to inflammatory stimuli in germ-free mice 7 days after fecal transplantation.Citation18–20 Nevertheless, there is no scientific consensus on the best methodology and timing of colonization for FMT in GF mice.
Figure 2. Control and post-COVID fecal microbiota transplant and effects on the gut of post-COVID mice. (a) experimental design: control (N = 8) and post-COVID (N = 14) mice received fresh feces from donors, and then analyzes of the gut microbiota and colon histology were performed 12 days after FMT. (b) 16S rRNA sequencing and comparison of the gut microbiota composition between human donor and mouse that received FMT. Principal Component analysis based on weighted Unifrac distances, a β-diversity index. α-diversity analysis based on Shannon, Simpson, and Chao1 indexes (donors N = 19; mice N = 19). (c) 16S rRNA sequencing of gut microbiota of control and post-COVID mice after FMT. β-diversity and α-diversity (N = 19). (d) Differential bacterial abundance in feces of control and post-COVID mice, Lachonospiraceae (p = .0300) (N = 19). (e) histological alterations in the large intestine in mice that received FMT. Black arrows indicate increases in Colonic lymphoid patches (N = 8). Red arrows Graphs showing the Colonic lymphoid patches Perimeter and the ratio between Goblet cells and epithelial cells in the colon. Statistical analysis: Wilcoxon test was used in B and C, PerMANOVA pairwise test was used in B and C, unpaired Student’s-t test was used in D, and Wald test was used in E. Data are shown as mean and standard deviation (SD). All results are representative of three independent experiments. See also Figure S2.
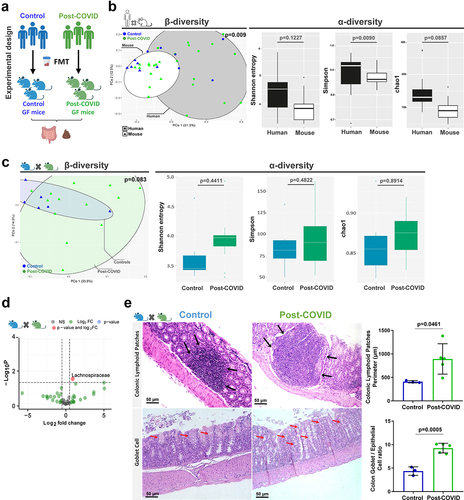
We evaluated human donor microbiota engraftment by first comparing the differences in β and α-diversity observed between fecal samples from human donors and recipient mice (). We analyzed engraftment levels by analyzing the percentage of bacterial groups from human donors retained in their respective recipient mice, we observed a level of engraftment between 45 and 75% and no difference between control and post-Covid groups (Figure S2B). During the engraftment, control and post-COVID mice presented similar mild diarrhea (commonly described during the first 5 days post-colonization) but without weight loss or any other observable alterations (data not shown). In addition to the differences between microbiota from human donors and recipient germ-free mice, transplanted humanized mice showed similarities between controls and post-COVID groups for both β- and α-diversity (). When comparing the composition and abundance of individual bacterial groups in the gut microbiota of transplanted humanized mice, the Lachnospiraceae family was significantly increased post-COVID compared to the control (). Although Lachnospiraceae were present in the inoculum of feces from donors, there were no differences between the control and post-COVID subjects (data not shown).
FMT can be used to demonstrate the direct effect of the gut microbiota on physiological processes. Therefore, we analyzed the impact of human microbiota in the gut of humanized microbiota (HM) mice compared to those that received feces from post-COVID versus control subject donors. HM mice that received feces from post-COVID subject donors did not show structural changes in the small intestine compared to controls but exhibited augmented cecal patches and an increase in goblet cells in the colon ().
To gain insights into the role of gut microbiota from post-COVID donors that may compromise intestinal homeostasis and influence systemic inflammation, we collected blood from human donors. We observed higher levels of the intestinal fatty acid-binding protein (I-FABP), a marker of epithelial damage (Figure S2C), suggesting disruption of gut epithelial barrier integrity in post-COVID subjects. However, this did not lead to increased translocation of gram-negative bacteria from the gut, because we did not observe differences in serum LPS levels between the groups (Figure S2D). Consistent with previous findings,Citation21 the levels of commensal microbial metabolites (acetate, propionate, butyrate) were reduced in the fecal samples of post-COVID subjects compared to their paired controls (Figure S2E-G). These findings suggest that post-COVID subjects had signs of intestinal epithelial injury with increased circulating I-FABP levels that might influence extraintestinal organs.
Post-COVID gut microbiota induces histological changes in the lung of humanized microbiota mice
To explore the systemic impact of post-COVID gut microbiota on extra-intestinal organs, we next assessed the effects of FMT on the lungs of recipient HM mice 12 days after transplantation (). We found foci of inflammatory infiltrates, mostly neutrophils, in both perivascular/peri-bronchial regions and increased histopathology scores in the lungs of mice receiving feces from post-COVID patients (). In addition, post-COVID mice showed increased expression of α-smooth muscle actin (α-SMA), indicative of physiological dysfunction in the lungs (). In addition, they had increased inflammatory cells in the bronchoalveolar fluid (BAL) compared to controls (). Cultivable Enterobacteriaceae in the BAL from post-COVID mice were found at higher levels than in control animals (), suggesting increased translocation of bacteria from the gut to the lung, which may account for the observed phenotype. Notably, SARS-CoV-2 nucleic acid was not detected in the lung tissues (), which corroborates the absence of the virus in the fecal samples used for the initial transplant (). We evaluated the levels of SCFAs in the feces of FMT mice and did not observe significant differences between controls and post-COVID mice ().
Figure 3. Post-COVID gut microbiota induce lung alterations in HM mice. (a) experimental design: germ-free mice received fresh feces from control (N = 8) or post-COVID (N = 14) donors and lung tissue and bronchoalveolar lavage were assessed 12 days after FMT. (b) H&E staining: the histopathological lung alterations induced by FMT to HM GF mice. Graph showing the histopathological score of airways, vascular and parenchymal inflammation in control and post-COVID mice lungs. Arrowheads indicate lung airways. Asterisks indicate inflammatory infiltrates. Scale bar: 50 µm. 20X objective (N = 22). α-SMA immune-staining: lung samples from HM GF mice and graph showing the morphometrical analysis of muscular layer changes. Ten images of the muscular layer of each animal were acquired with a 40X objective. Arrowheads indicate the immunostained area (N = 10). (c) total number of cells in bronchoalveolar lavage (N = 19). (d) cultivable Enterobacteriaceae load in bronchoalveolar lavage (N = 19). (e) RT-qPCR for SARS-CoV-2 in the lungs of control and post-COVID mice (N = 22). (f) paired analysis of feces acetate, propionate, and butyrate levels in control and post-COVID HM GF mice (N = 16). Statistical analysis: unpaired Student’s t-test was used in B, C and D. Wilcoxon matched-pairs signed rank test was used in F. Data are shown as mean and standard deviation (SD). All results are representative of three independent experiments.
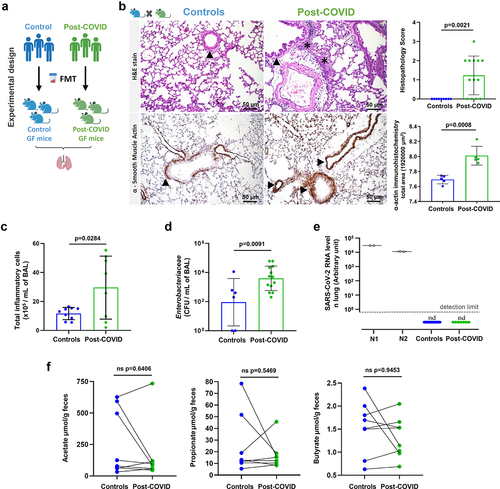
Overall, our data indicate that transfer of fecal samples from post-COVID subjects induced lung inflammation in recipient mice in the absence of SARS-CoV-2, suggesting that the intestinal microbiota modified by COVID-19 causes this phenotype.
Fecal transplantation from post-COVID human volunteers to HM mice impairs host pulmonary defense
We hypothesized that the consequences of post-COVID fecal transplant-induced lung alterations could further impact host defense and favor secondary infections. To validate this hypothesis, we intranasally infected HM mice with a multidrug-resistant strain of Klebsiella pneumonia (Kp) (). We compared Kp lung infection in control versus post-COVID mice and observed higher pathological changes in the perivascular, peri-bronchial, and parenchymal regions characterized by emphysema-like areas in the lungs of infected post-COVID mice (). These changes were associated with intense inflammatory cell infiltration in the BAL of these mice compared to the infected control mice (). Despite this, the recruitment of inflammatory cells to the lungs was inefficient for the clearance of the bacteria because we harvested similar CFU levels of Kp in the lungs of both control and post-COVID-infected mice (). We also observed an increase in Enterobacteriaceae CFU numbers in the blood of both post-COVID Kp-infected and non-infected (vehicle) mice suggesting systemically translocation of gram-negative bacteria in post-COVID mice (). Our group and others have demonstrated that acetate, a gut microbiota metabolite, contributes to the control of pulmonary infection induced by the pathogen Klebsiella pneumonia in mice.Citation22–24 Accordingly, we observed reduced serum acetate levels in post-COVID Kp-infected mice compared to those in post-COVID non-infected (vehicle) mice (). When we correlated the fecal levels of SCFAs in paired mice, we did not observe significant differences in non-infected (vehicle) control mice and their paired Kp-infected mice (). However, we noted a propionate reduction in the fecal samples of post-COVID mice Kp-infected compared to their non-infected (vehicle) post-COVID donor-paired mice ().
Figure 4. FMT from post-COVID patients impacts the gut-lung axis and increases susceptibility to K. pneumoniae B31 lung infection. (a) experimental design: germ-free mice received fresh feces from control or post-COVID donors and were infected with K. pneumoniae B31 (K. pneumoniae: control N = 13, post-COVID N = 15) or received saline (vehicle), and lung tissue, bronchoalveolar lavage and serum SCFAs levels were assessed. (b) histological alterations in the lung of post-COVID mice infected by K. pneumoniae B31 and a graph showing the histopathological score of the airway, vascular and parenchymal inflammation in control and post-COVID mice lungs (N = 28). Asterisks indicate inflammatory infiltrates. Hash marks areas of emphysema. Scale bar: 50 μm. 20X and 40X objective. (c) total number of inflammatory cells in bronchoalveolar lavage (BAL) (N = 28). (d) total numbers of Enterobacteriaceae in BAL (N = 28) and (e) blood (N = 28). (f) serum acetate levels (mmol.L−1) in vehicle and K. pneumonia-infected HM mice (N = 33). (g) paired analysis of fecal acetate, propionate, and butyrate levels between vehicle and infected mice that received feces from the same control donor (N = 14). (h) paired analysis of fecal acetate, propionate, and butyrate levels between vehicle and infected mice that received feces from the same post-COVID donor (N = 30). Statistical analysis: unpaired Student’s t-test was used in B and C, Mann-Whitney test was used in D. Two-way ANOVA with Tukey’s tests was used in E and F. Wilcoxon matched pairs signed rank test was used in G and H. Data are shown as mean and standard deviation (SD). All results are representative of three independent experiments.
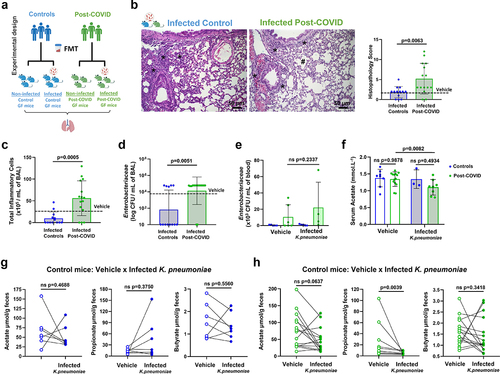
Overall, our data indicate that transplantation of post-COVID feces affected the lungs of recipient mice in the absence of SARS-CoV-2 and contributed to the impairment of the host lung defense against bacterial infection.
Post-COVID gut microbiota induces memory impairment and hippocampus changes and can be partially reversed with probiotics
In addition to lung alterations, accumulating reports have found that post-COVID sequelae are commonly associated with brain dysfunction.Citation12–25–Citation27 To further explore the causal effects of gut microbiota on brain alterations, HM germ-free mice that received FMT from post-COVID and control subjects were subjected to cognitive behavioral tests (). Post-COVID mice showed memory impairment in the recognition and location tests compared to control mice (). Control mice showed significantly more interaction with a novel object in the cage than post-COVID mice. Based on these phenotypes, we analyzed inflammatory markers in the hippocampus of post-COVID mice, which could indicate a possible connection with neuroinflammation. We observed increased mRNA expression of the pro-inflammatory cytokine tnf but lower levels of neuroprotective factors such as bdnf and psd-95 in post-COVID mice than in controls (). Together, these results suggest that alterations in gut microbiota and inflammation caused by COVID-19 can directly cause changes in brain cognition.
Figure 5. FMT from post-COVID patients induces cognitive alterations in HM mice. (a) experimental design: germ-free mice received fresh feces from control (N = 14) or post-COVID (N = 15) donors, and underwent cognition (object location and recognition) tests nine days later. Following behavioral analysis, their hippocampus was subjected to mRNA expression. (b) percentage of exploration time for the new object (N) or the one remaining unmoved (O) in the location test relative to a total exploration time (N = 29) 9 days after FMT. Quantification of the expression, by RT-qPCR, of (c) TNF (N = 13), (d) BDNF (N = 13), and (e) PSD95 (N = 13) in the hippocampus 12 days after FMT. Statistical analysis: One sample t test against the hypothetical value of 50% and unpaired Student’s t test was used in B. Data are shown as mean and standard deviation (SD). All results are representative of two independent experiments.
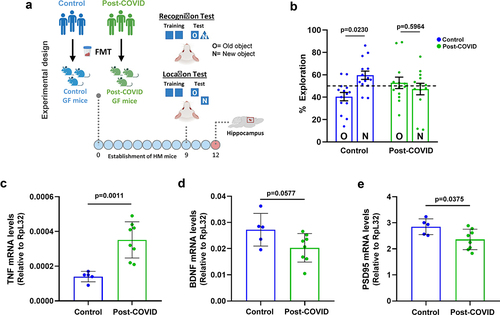
To further investigate and test the potential of microbiota-based interventions as targets to prevent memory impairment induced by direct coronavirus infection, we used a β-coronavirus murine model of lung infection.Citation28 This experimental murine model of mouse hepatitis virus (MHV-3) mimics human COVID-19 inflammatory manifestations in mice, and we also observed that infected animals had memory impairment using the object location test. Using this model, we tested the potential of microbiome-based interventions by administering the probiotic Bifidobacterium longum 51A (). The particularity of choosing the probiotic B. longum 51A strain is the ability to produce high levels of SCFAs, which has beneficial effects in controlling host inflammation, particularly in the lung as previously demonstrated by our group.Citation23,Citation29 Treatment with B. longum 51A prevented memory impairment induced by MHV-3 infection in mice (). The probiotic treatment also reduced the weight loss caused by the murine coronavirus infection (). There is no alteration in the total numbers and/or differentiation of any inflammatory cells in both probiotic-treated bronchoalveolar fluid (BAL) in either probiotic-treated or not in MHV-3 infected mice (). Notably, treatment with B. longum 51A to non-infected mice per si increased inflammatory infiltration of cells in the BAL (). Still, treatment with B. longum 51A reduced the lung tissue inflammation characterized by a reduction in the foci of inflammation in perivascular and peribronchial regions induced by MHV-3 infection, as shown in the histopathology, and measured by inflammatory score (). To test the effect of the SCFA, acetate, on MHV-3 condition, we treated mice orally with acetate (Figure S6). However, we did not find any decrease in the symptoms while observing minor protection in the survival MHV-3-infected mice treated with acetate (Figure S6A).
Figure 6. The mouse model of MHV-3 infection showed memory impairment in object recognition and location tests, and treatment with B. longum 51A reversed the cognitive alterations. (a) experimental design: C57BL/6 non-infected and MHV-3 infected and treated with probiotic B. longum 51A (vehicle: non-infected N = 4; MHV-3 infected N = 7; B. longum 51A: non-infected N = 4; MHV-3 infected N = 5), and subjected to behavioral (object location and recognition) tests 4 days later. (b) percentage of exploration time for the new object (N) or the one remaining unmoved (O) in the location test relative to a total exploration time (N = 20). (c) Body mass over time was measured daily throughout the experiment. (# significant main effect of MHV infection) (N = 29). (d) total number of inflammatory cells in bronchoalveolar lavage (BAL) (N = 29). Differential inflammatory cells number in bronchoalveolar lavage (BAL) (N = 29). (e) H&E staining: histological alterations in the lung of MHV-3 infected mice and treated with probiotic B. longum 51A. Graph showing the histopathological score of the airway, vascular and parenchymal inflammation in control and post-COVID mice lungs (N = 29). Arrowheads indicate lung airways. Asterisks indicate inflammatory infiltrates. Scale bar: 50 μm. 20X objective. Statistical analysis: One sample t-test against the hypothetical value of 50% was used in B. Three-way repeated measures ANOVA with Tukey’s test was used in C. Two-way ANOVA with Student-Newman-Keuls test was used in D and E. Data are shown as mean and standard deviation (SD).
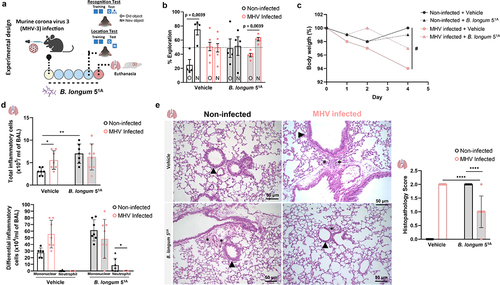
Altogether, our data indicate that alterations in the gut microbiota contribute to the post-COVID disruption of hippocampal function, leading to cognitive impairment, which might be prevented or attenuated via microbiome-based interventions.
Discussion
Among pathophysiological responses triggered by SARS-CoV-2 infection, several studies showed associations between gastrointestinal symptoms and altered gut microbiota in COVID-19 during and after the infection.Citation12–30−Citation33 However, our study is the first to show a causal effect of gut microbiota alterations on post-COVID sequelae. Our findings confirm previous data that SARS-CoV-2 infection is associated with the spread of antimicrobial resistance in the gut microbiota.Citation34Citation35Citation36Citation37–Citation34Citation39 In fact, we observed an increase in AMR Enterobacteriaceae in the gut microbiota of post-COVID individuals who were either asymptomatic or had mild symptoms of COVID-19. This is especially surprising, as COVID-19-related AMR spread has been mainly associated with moderate/severe cases and hospitalized individuals.Citation38 Although the widespread use of antimicrobials during COVID-19 and overuse between our post-COVID and control volunteers may explain the increased AMR spread,Citation40–43 we cannot exclude the direct impact of SARS-CoV-2 infection on increasing AMR through the microbiome. Notably, we found a predominant increase in AMR Klebsiella sp. in post-COVID gut microbiota subjects. This bacterium belongs to the ESKAPE group (Enterococcus faecium, Staphylococcus aureus, Klebsiella pneumoniae, Acinetobacter baumannii, Pseudomonas aeruginosa, and Enterobacter species) of AMR pathogenic bacteria that are responsible for most nosocomial pneumonia in hospitalized patients with COVID-19.Citation44,Citation45 Moreover, post-COVID mice had worse lung injury, which compromised the lung immune response to K. pneumoniae B31 infection. Therefore, post-COVID microbiota transfer may have contributed to the impairment of the pulmonary immune system, inducing a greater susceptibility to infections caused by pathogens/pathobionts.Citation46–49 Lung microbiota disruption is another relevant factor associated with pulmonary alterations observed in post-COVID mice is lung microbiota disruption.Citation50 The lung microbiota is known to be susceptible to gut microbiota alterations, highlighting the relevance of the gut-lung axis.Citation50,Citation51 Although we did not evaluate the lung microbiota as a whole, we found increased cultivable Enterobacteriaceae in the lungs and pulmonary tissue damage in mice with post-COVID gut microbiota, but not in the controls. These findings raise questions about the components in the fecal samples that induce such alterations after transplantation and which mechanisms are involved in the gut-lung connection.
The next step in our investigation was to deepen our understanding of the effects triggered by post-COVID microbiota by exploring other host tissues. Therefore, the connection between the gut and brain is well established,Citation52 and most recently, it has been described as the lung-brain axis.Citation53,Citation54 Our finding that post-COVID microbiota can induce memory impairment in transplanted mice suggests a possible connection with the neurological outcomes of post-acute COVID-19 in humans.Citation55,Citation56 Although our study did not assess the role of the lung microbiota in neurological disorders, clinical studies have shown differences in the lung microbiota between cognitively impaired individuals versus controls.Citation57 Previous studies suggested that even minimal alterations in the lung microbiome can affect the central nervous system, although significant changes in gut microbiota were necessary.Citation58 Thus, lung alterations induced by the microbiota in post-COVID human donors in post-COVID mice may be associated with neurological outcomes.
Gut microbiome production of metabolites, particularly short-chain fatty acids (SCFA), is an effective mechanism that supports the gut-lung and gut-brain axis.Citation14,Citation59 SCFAs are also known to play a role in the development of the immune system and lung mucosal function, thereby protecting against infections and pulmonary damage.Citation59 Furthermore, SCFA can also modulate blood-brain barrier integrity and inflammatory responses in microglia,Citation58 and clinical and experimental studies have associated reduced SCFA levels with Alzheimer’s disease.Citation60 We observed reduced acetate, propionate, and butyrate levels in the feces of post-COVID donor subjects and decreased acetate levels in post-COVID Kp-infected mice. Thus, this decrease in SCFA levels could affect the gut-lung and gut-brain connections and help explain neurological sequelae and the increased susceptibility to pulmonary coinfections observed post-COVID.
We also observed apparent differences in the systemic levels of host factors, such as I-FABP, indicating a loss of intestinal homeostasis in post-COVID subjects compared with controls. Indeed, I-FABP is a relevant prognostic biomarker that is positively correlated with a worse prognosis in COVID-19.Citation61 Thus, although no significant differences in α- and β-diversity were observed in the gut microbiota of post-COVID and control subjects, functional changes were observed in both patients and mice. Notably, enrichment of the Lachnospiraceae family in post-COVID mice is corroborated by previous findings, where subjects with COVID-19 had a higher prevalence of this group of bacteria.Citation62,Citation63
We observed increased TNF expression in the hippocampus of mice with post-COVID gut microbiota, suggesting neuroinflammatory responses in the central nervous system. A similar effect was observed in a colitis model, where systemic-driven hippocampal TNF expression was associated with memory impairment, which was abolished upon restoration of the gut microbiotaCitation64. Furthermore, we found reduced expression of the neuroplasticity markers BDNF and PSD-95 in the hippocampi of post-COVID mice. This impairment in neuroplasticity was previously observed when feces from transgenic Alzheimer’s disease mice transferred their cognitive phenotype to recipient mice.Citation65
As a proof-of-concept for the potential use of microbiome-based approaches for post-COVID sequelae, we used a probiotic strain of B. longum 51A to assess a mouse model of coronavirus infection. Here, we used the probiotic B. longum 51A isolated from the gut microbiota of healthy children, which can produce high levels of SCFAs and has beneficial effects in controlling inflammation at a systemic level, especially in the lung.Citation23,Citation29,Citation66 We observed neuroprotective effects of B. longum 51A which prevented memory impairment after lung infection with a murine coronavirus. Although the effects of B. longum 51A should be explored further, these data suggest that therapies targeting the gut microbiota are promising approaches for treating post-acute COVID-19 consequences.Citation67
Our study indicates a direct connection between altered gut microbiota and post-COVID symptoms.Citation15 However, some limitations of this study should be considered. First, our study had a relatively small sample size and compared humans that were not perfectly age- and sex-matched. Other factors, such as gut viromes, were not assessed in our study but were associated with clinical outcomes of COVID-19.Citation8,Citation68–70 Finally, SARS-CoV-2 antigens can persist for long periods in the intestine, feces, and gut tissues, boosting immune responses that may fuel post-COVID symptoms.Citation71 However, we did not detect SARS-CoV-2 in the feces of donors or lungs of humanized microbiota mice.
Collectively, our results suggest a direct connection between the long-term effects of COVID-19 and alterations in the gut microbiota after the clearance of SARS-CoV-2 infection. Our findings emphasize the need to define how the gut microbiota is affected by SARS-CoV-2 infection, even in individuals who do not have severe symptoms. This is especially important, given the increased AMR in the gut microbiota of patients previously infected with SARS-CoV-2. AMR has spread at alarming rates, and the effects of the COVID-19 pandemic on the gut microbiota resistome could have a major additional contribution.
Materials and methods
Study design
This cross-sectional study consisted of two steps:1. Fresh feces and blood were collected from post-COVID and health volunteers (control), and a survey consisting of clinical symptoms during COVID-19, medication use, and lifestyle questions was administered (Figure S1A); 2. We developed humanized microbiota (HM) mice by performing individual FMT from these donors into GF-recipient mice (Figure S2F). For the FMT, samples of subjects (Control and Post-COVID) from the same household (n = 6 families; in total n = 12 members) were prioritized, where one was post-COVID and the other was not infected (Control). Additionally, random samples of post-COVID individuals (n = 8) were added to the experimental group, totaling 19 samples. In addition to the second stage of the study, an experimental pulmonary infection induced by the multidrug-resistant Klebsiella pneumoniae B31 strain was performed ().
Study subjects and sample collection
Seventy-two post-COVID volunteers (after nucleic acid amplification test [NAAT] or an antigen test confirmed SARS-CoV-2 infection) and fifty-nine control volunteers (SARS-CoV-2 NAAT or antigen and IgG/IgM negative) were included (Figure S1A). In the post-COVID group, sixty-four volunteers were symptomatic (collections were performed 1 to 4 months after infection), and eight were asymptomatic (classified after antigen test). All volunteers, controls, and post-COVID were between 15 and 60 years of age. The clinical spectrum of disease severity (mild and moderate) in post-COVID and the volunteers was classified according to the NIH COVID-19 Treatment Guidelines (National Institutes of Health, 2022). Vaccination for SARS-CoV-2 and positive serological test results at the time of sample collection were excluded. Samples were collected between October 2020 and April 2021 from Belo Horizonte, Minas Gerais, Brazil. Fecal samples were collected in a sterile container and immediately stored in a home refrigerator (4°C °C) for 12 h prior to analysis and FMT in the laboratory. All subjects consented to participate in this study with the approval of the Ethics Committee on Human Research of Universidade Federal de Minas Gerais (COEP) protocol 4.615.698.
SARS-CoV-2 load in fecal samples
RNA extraction from fecal, human, and mouse samples was adapted from a previously published protocol.Citation72 Briefly, fecal samples were diluted 1:5 (w:v) in guanidine, homogenized, and clarified by centrifugation (4,000xg, 20 min, 4°C). Viral RNA was purified using the QIAamp Viral RNA Mini Kit, following the manufacturer’s instructions. RT-qPCR for SARS-CoV-2 was performed using the one-step RT-qPCR Master Mix according to the CDC USA protocol (CDC, 2020), and primers for N1 and N2 (cat. no. 10006770) on a QuantStudio™ 7 Flex Real-Time PCR System platform (Applied Biosystems, USA). For analysis, amplification values of N1 or N2 viral targets with threshold cycle (Ct) below 40.0 were considered positive for SARS-CoV-2, and above 40 or indeterminate were considered undetectable, and the relative concentrations were expressed in arbitrary units. Fecal samples spiked with inactivated SARS-CoV-2 (stock titer 6.7 × 106 PFU/mL) were used as a positive control at different dilutions.
Gut microbiota composition analysis
DNA extraction of fecal samples – human and mouse – stored at -70°C was performed using QIAamp DNA Stool Mini Kits (Qiagen, USA) according to the manufacturer’s instructions. DNA was used as a template in the PCR amplicon, targeting the V3 and V4 hypervariable regions of the bacterial 16S rRNA gene. The Illumina 16S Metagenomic Sequencing Library Preparation protocol was used to prepare a 16S rRNA gene library. The 16S library was quantified using the Qubit dsDNA HS Assay Kit (Invitrogen, USA) and checked using a 2100 Bioanalyzer Instrument (Agilent Technologies, USA). The sample pool (4 nM) library was diluted to a final concentration of 8 pM and added to 20% (v/v) of 8 pM PhiX DNA (Illumina, USA), following the Illumina guidelines. Sequencing was performed using the Miseq reagent kit v3 (600 cycles) on the Illumina MiSeq platform and 2 × 300 bp (MSC v2.4), according to the manufacturer’s instructions (Illumina, USA). 16S rRNA gene sequence data were processed using the QIIME2 pipeline.Citation73 First, sequenced reads were denoised with DADA2 and then processed by VSEARCHCitation74 to filter chimeras and perform de novo clustering of valid sequences into OTUs with 97% sequence similarity. Next, MAFFT Fasttree was used to conduct phylogenetic analysis based on OTUs. α- and β-diversity were analyzed using the core-metrics-phylogenetic method built into QIIME2, and the Shannon and Simpson diversity indices and Chao1 were calculated; for β-diversity, Bray-Curtis and weighted UniFrac distances were calculated. OTUs were taxonomically classified using Naive Bayes classifiers trained with Silva v. 138, with 97% sequence similarity to full-length OTUs. Differential abundance was calculated using the ANCOM.Citation75 A two-sided alternative Wilcoxon test was performed for alpha diversity parameters, while pairwise Permanova was performed for beta diversity parameters. The differential bacterial abundance was calculated using DESeq2Citation76 and plotted as a volcano plot using the EnhancedVolcano package.Citation77 The bacterial families with log2 Fold-change >0,5 and P-value ≤0.05, were considered statistically different between conditions. Statistical analyses were performed using the R statistical software environment (The R Foundation, Austria). The 16S rRNA gene amplicon sequencing library produced in this study was deposited in the NCBI SRA database under the project number PRJNA843134.
Enterobacteriaceae identification and antimicrobial resistance test
Fresh fecal samples were homogenized (100 mg for each 1 mL of sterile 0.9% saline) and serially diluted (1:10). Subsequently, different dilutions were plated on MacConkey agar (Sigma, Germany) and incubated for 24 h at 37°C under aerobic conditions. The colonies were counted, and the data were expressed as the log10 of colony-forming units (CFU) per milligram of feces. Enterobacteriaceae colonies with different morphologies were isolated from the MacConkey agar. Pure colonies were suspended in sterile 0.9% saline at a 1.5 × 108 CFU/mL concentration according to the 0.5 McFarland standard. Then, a sterile swab was soaked in the bacterial solution and inoculated by spreading on Mueller-Hinton agar plates (140 × 15 mm) (Merck, USA). After 15 minutes, a dispenser (Thermo Scientific, Remel™, USA) with 12 discs (Thermo Scientific, Oxoid™, USA) referring to β-lactam (amoxicillin-clavulanic acid, cephalosporin, ertapenem, meropenem, imipenem), aminoglycosides (amikacin, streptomycin, gentamicin), quinolones (ciprofloxacin, levofloxacin, norfloxacin), sulfonamide and folate inhibitors (sulfamethoxazole-trimethoprim) and macrolides (azithromycin) antibiotics were added to the inoculated plates. The plates were incubated at 37°C for 24 h. The presence or absence of bacterial growth inhibition zones was observed and measured to determine the resistance profile in: sensitive, intermediate, or resistant, according to the CLSI M100Ed31 guidelines. The resistance phenotype was determined according to the number of antimicrobial classes in which each strain presented resistance: resistant (1–2 antimicrobials) or multidrug-resistant (≥3 antimicrobials). Identification of Enterobacteriaceae strains was performed by Matrix Associated Laser Desorption-Ionization – Time of Flight (MALDI-TOF), using the FlexControl MicroFlex LT mass spectrometer (Brunker Daltonics, USA) as described before.Citation78 Before identification, calibration was performed using the Escherichia coli DH5α test standard (Brunker Daltonics, USA).
Laboratory animals
Male and female germ-free Swiss/NIH mice derived from a GF nucleus (Taconic Farms, USA), with ~ 8-weeks-old were used. They were maintained in flexible plastic isolators (Standard Safety Equipment Co., USA) using classical gnotobiology techniques at the Gnotobiology Laboratory of the Federal University of Minas Gerais (UFMG), Minas Gerais, Brazil, under controlled conditions (26°C, 12 h light/dark cycle) with steam sterilized food (Nuvilab, Brazil) and sterile water ad libitum. For the FMT experiments, germ-free mice were kept individually in sterile microisolator cages (UNO Roestvaststaal B.V., Netherlands) throughout the experiment to avoid cross-contamination and ensure the individual feces donor phenotype for the respective HM mice. Also, Male and female C57BL/6J mice, aged ~8 weeks old, obtained from the UFMG animal facility, were kept in plastic cages (Alesco, Monte Mor, Brazil) in a room with controlled conditions (26°C, 12 h light/dark cycle) with steam sterilized food (Nuvilab, Brazil) and sterile water ad libitum. All mouse procedures were performed in accordance with the guidelines of the Guide for the Care and Use of Laboratory Animals of the Brazilian National Council of Animal Experimentation (http://www.cobea.org.br/) and the Brazilian Federal Law 11.794 (October 8, 2008). The animal study was reviewed and approved by The Institutional Committee for Animal Ethics of the Federal University of Minas Gerais (protocol no. CEUA/UFMG 281/2020 and 55/2021).
Human fecal microbiota transplant to GF mice
Fresh fecal samples were used for FMT, and samples from members of the same household were prioritized (n = 6 families; n = 12 members). In addition, randomized samples of post-COVID subjects were included (n = 8), totaling 19 samples (Figure S2F). Each sample was weighed and resuspended in 0.9% sterile saline (NaCl) solution (100 mg/mL). FMT was performed individually, and each animal received a sample from an individual donor volunteer. The fecal transfer was not sex-matched, but the proportion of male and female animals was the same in groups receiving fecal transfer from control or post-COVID subjects. A 100 μL aliquot was used for oral gavage of GF mice, with the same concentration and volume of feces and bacteria in all animals. After nine days, the experiments were performed to ensure a stable human microbiota population of GF mice when we were able to transfer human bacteria to GF mice, according to our group.Citation18,Citation79
Histopathology and immunohistochemistry
Intestine and lung tissues from HM mice were collected and processed. For the intestinal morphometric analysis, images (20X objective) were acquired from hematoxylin and eosin (H&E) staining for quantification of the colonic lymphoid patches and globet cells, and the absolute number of colon globet cells per epithelial ratio and perimeter of lymphoid patches in the controls compared to post-COVID mice. For lung analysis, the inflammatory score was determined using hematoxylin and eosin (H&E)-stained slides, and airway, vascular, and parenchymal inflammation were evaluated, as previously described.Citation80,Citation81 For immunohistochemical analysis, lung tissue slides from control and post-COVID HM mice were immunostained. Briefly, the slides were incubated with primary anti-α-actin antibody (1:500) (DAKO, USA) overnight at 4°C. The primary antibodies were detected using an anti-mouse/anti-rabbit detection system (Novolink Polymer Detection System; Leica Biosystems, UK) according to the manufacturer’s instructions. The sections were counterstained with diluted Harris Hematoxylin solution and permanently mounted using Entellan (Merck, USA). For the morphometric analysis, images (20X objective) were acquired from immunolabeled α-actin to quantify the muscle layer of the lung section. For intestine and lung morphometric analyses, we used the ImageJ 1.52 program (NIH, USA). All analyses were performed under a light microscope by a pathologist blinded to the experiment.
Bronchoalveolar lavage collection and analysis
After anesthetizing and euthanizing the mice (ketamine/xylazine, 180 and 12 mg/kg, respectively), bronchoalveolar lavage (BAL) was performed by inserting and collecting 1 mL of sterile phosphate-buffered saline (PBS) through a 20-gauge catheter in a 1-mL syringe. The Enterobacteriaceae quantification was quantified by plating an aliquot (100 µL) in MacConkey medium and incubating under aerobic conditions for 24 h at 37°C. Colonies were counted and expressed as CFU per mL of BAL. For airway inflammatory cell counts, the remaining BAL was centrifuged and resuspended in 100 µL of saline, and total leukocytes were quantified by Neubauer chamber counting.
Klebsiella pneumoniae infection
K. pneumoniae B31, a clinical isolate with an AMR profile,Citation82 was kindly provided by Prof. Vasco Azevedo, Laboratory of Cellular and Molecular Genetics at ICB/UFMG. Intratracheal infection was induced as previously described.Citation23 Briefly, anesthetized animals were exposed to the trachea and 25 μL of the suspension containing 1 × 10Citation6 CFU/mL of K. pneumoniae B31, or sterile saline for vehicle control animals, was administered with a 26-gauge needle.
Short-chain fatty acid (SCFA) assays
Human and mouse fecal samples were suspended in 1% phosphoric acid (1:6 weight:volume) (Merck, USA), vortexed, and centrifuged (20,000xg, 30 min, 4°C). Supernatants were filtered (0.22 µm) and injected directly into an HPLC system with an ionic exchange resin column 300 × 7.8 mm (Sigma, Germany) at 30°C with a Micro-Guard cation H+ cartridge (Sigma, Germany) and detector set at 210 nm. The flow rate was 0.5 mL/min for 35 min, changed to 0.7 mL/min until the end of the 55 min chromatographic run. Serum samples were diluted in formic acid (1 mol.L−1), and internal standard, 2-ethyl-butyric acid 1 mol.L−1, (Sigma, Germany) was added, in proportions 5:5:1 respectively, followed by vortexing and centrifugation (12,000xg, 30 min, 4°C). Next, supernatants were injected into a Gas Chromatograph – FID (Agilent, USA), with an HP-FFAP column 19091F–105 (Agilent, USA), 50 m × 0.20 mm × 0.33 µm, and the detector set at 240°C. chromatographic conditions were 60°C for 0.5 minutes, heating at 8°C.min−1 to 180°C for 1 min, with a new heating rate of 20°C.min−1 at 240°C for 7 min. The total run time was 26.5 minutes. Seven-point external calibration curves were adopted to quantify fecal and serum samples using analytical-grade SCFA (Sigma, Germany) as standards.
Mouse behavioral tests
The tests were performed in a 30 (w) × 30 (d) × 45 (h) cm arena, where each animal was allowed to freely explore for 5 min. The next day, the mice were subjected to a 5 min training session, during which they were placed at the center of the arena in the presence of two identical objects. The time spent exploring each object was then recorded. The test session was performed after 30 min by replacing one of the two identical objects with a new one in the object recognition paradigm or moving the training object to a new location in the new object location paradigm.Citation83 The results were expressed as a percentage of time exploring each object or location, old (O) or new (N), concerning the total exploration time during the test session.
Mouse hippocampus analysis
Total RNA from the hippocampus of post-COVID HM mice and controls was extracted using TRIzol reagent (Thermo Scientific, USA). cDNAs was synthesized and subjected to qPCR using Power SYBR Green Master Mix kits following the manufacturer’s instructions on the QuantStudio™ 7 Flex real-time PCR system platform (Applied Biosystems, USA). Primer sequences used are listed in . Gene expression changes were determined by the 2–ΔCt method using ribosomal protein L32 for normalization.
Table 3. Primer sequences for RT-qPCR.
Mouse hepatitis virus-3 (MHV-3) infection
The β-coronavirus mouse hepatitis virus MHV-3 (3 × 10Citation1 PFU), propagated in L929 cells or sterile saline for sham controls, was used to intranasally infect C57BL/6J mice as previously described.Citation28 Some groups were treated with B. longum 51A. The probiotic bacterium B. longum 51A, from the culture collection of the Laboratory of Biotherapeutic Agents at ICB/UFMG was isolated from fecal samples of healthy children and cultivated as previously described.Citation23,Citation84 Briefly, mice were treated by oral gavage of a single 100 μL dose of suspension containing 1 × 10Citation9 CFU/mL B. longum 51A or sterile saline every 48 h during the infection period.Citation23 Four days after infection, behavioral tests were performed.
Intestinal fatty acid-binding protein (I-FABP) assays
I-FABP was quantified by enzyme-linked immunosorbent assay (ELISA) in accordance with the manufacturer’s instructions (R&D Systems, USA) and as previously described.Citation61
Statistical analysis
Statistical analyses were performed using GraphPad Prism7 (GraphPad Software, USA) and R software v.4.2.2 (R Core Team, 2013). Graphs were produced GraphPad Prism7, Microsoft PowerBi (Microsoft Corporation, USA), or R software v.4.2.2. Data normality and homoscedasticity were tested using the Shapiro-Wilk and Levene tests, respectively. Data with a normal distribution were evaluated by Student’s t test paired or unpaired, and one-way, two-way, or three-way analysis of variance (ANOVA). Following significant ANOVAs, a post-hoc test was performed according to the coefficient of variation (CV): Tukey (CV ≤ 15%), Student’s Newman-Keuls (CV 15–30%), and Duncan (CV > 30%). Non-parametric tests were applied to data that did not show normal distribution, using Mann-Whitney, Kruskal – Wallis post-hoc tests, or Wilcoxon matched-pairs signed rank test. Log-rank (Mantel – Cox) test was used for survival analysis. Categorical data were analyzed using Fisher’s exact test, chi-square test, or Wald test, depending on the experimental design. Data are presented as mean ± standard deviation (SD).
Author Contributions
Study conception, methodology, supervision, project administration and funding acquisition: A.T.V.
Investigation: V.M., D.F.E., M.F.R., C.S.C., D.A.A., M.A., J.M., E.C.M., V.M.R., T.G.C., L.S.B.L., J.C.P., M.A., G.S.Z., B.J.S., V.C.C., E.S.R., I.G., and C.C.G.
Formal analysis and interpretation of the data: V. M., M. F. R., D. F. E., C. S. C., E. R. G. R. A, I. S. L., D. A. A., T. G. C., G. D. C., and D. M. S.
Data curation: V.M., E.R.G.R.A, and I.S.L.
Resources: F.S.M., J.T.M., F.M.R., L.C.A., M.M.T., V.V.C., R.S.S.
Writing – original draft: V.M., A.T.V., M.F.R., D.F.E.
Writing – review & editing: J.T.M., P.M.H., M.M.T., and A.T.V.
Visualization: V.M., C.S.C. A.T.V., and M.F.R.
Supplemental Material
Download MS Excel (11.5 KB)Supplemental Material
Download MS Word (705.1 KB)Acknowledgments
Prof. Vasco Azevedo provided the K. pneumonia B31 clinical isolate, Prof. Vivian Vasconcelos Costa and Prof. Mauro Martins Teixeira provided the mouse hepatitis virus MHV-3, and Prof. Flaviano S. Martins provided the probiotic strain B. longum 51A. Daniela M. Nolasco and Prof. Leda Quercia Vieira for technical support; Eduardo Zimmer, Andreza F. de Bem, and Ana Maria Caetano de Faria for their valuable discussions.
Disclosure statement
No potential conflict of interest was reported by the author(s).
Data availability statement
The 16S rRNA gene libraries produced in this study were deposited in the NCBI SRA database under the project number PRJNA843134. The data are available upon request from the corresponding author.
Supplementary material
Supplemental data for this article can be accessed online at https://doi.org/10.1080/19490976.2023.2249146
Additional information
Funding
References
- World Health Organization. COVID-19 weekly epidemiological update, 16 March 2023. 134th ed. 2023. https://apps.who.int/iris/handle/10665/366534.
- Augustin M, Schommers P, Stecher M, Dewald F, Gieselmann L, Gruell H, Horn C, Vanshylla K, Di Cristanziano V, Osebold L. Post-COVID syndrome in non-hospitalised patients with COVID-19: a longitudinal prospective cohort study. Lancet Reg Heal. 2021;6:100122. doi:10.1016/j.lanepe.2021.100122.
- Lopez-Leon S, Wegman-Ostrosky T, Perelman C, Sepulveda R, Rebolledo PA, Cuapio A, Villapol S. More than 50 long-term effects of COVID-19: a systematic review and meta-analysis. Sci Rep. 2021;11(1):16144. doi:10.1038/s41598-021-95565-8.
- Subramanian A, Nirantharakumar K, Hughes S, Myles P, Williams T, Gokhale KM, Taverner T, Chandan JS, Brown K, Simms-Williams N. Symptoms and risk factors for long COVID in non-hospitalized adults. Nat Med. 2022;28(8):1706–22. doi:10.1038/s41591-022-01909-w.
- Hikmet F, Méar L, Edvinsson Å, Micke P, Uhlén M, Lindskog C. The protein expression profile of ACE2 in human tissues. Mol Syst Biol. 2020;16:e9610. doi:10.15252/msb.20209610.
- Sencio V, Gallerand A, Gomes Machado M, Deruyter L, Heumel S, Soulard D, Barthelemy J, Cuinat C, Vieira AT, Barthelemy A, et al. Influenza virus infection impairs the gut’s barrier properties and favors secondary enteric bacterial infection through reduced production of short-chain fatty acids. Infect Immun. 2021;89(9):e0073420. doi:10.1128/IAI.00734-20.
- Yeoh YK, Zuo T, Lui GY, Zhang F, Liu Q, Li AY, Chung AC, Cheung CP, Tso EY, Fung KS, et al. Gut microbiota composition reflects disease severity and dysfunctional immune responses in patients with COVID-19. Gut [Internet]. 2021;70(4):698–706. doi:10.1136/gutjnl-2020-323020.
- Zuo T, Liu Q, Zhang F, Lui GCY, Tso EYK, Yeoh YK, Chen Z, Boon SS, Chan FKL, Chan PKS, et al. Depicting SARS-CoV-2 faecal viral activity in association with gut microbiota composition in patients with COVID-19. Gut. 2021;70:276–284. doi:10.1136/gutjnl-2020-322294.
- Chen Y, Gu S, Chen Y, Lu H, Shi D, Guo J, Wu WR, Yang Y, Li Y, Xu KJ, et al. Six-month follow-up of gut microbiota richness in patients with COVID-19. Gut. 2022;71(1):222–225. doi:10.1136/gutjnl-2021-324090.
- Cullen BR. MicroRNAs as mediators of viral evasion of the immune system. Nat Immunol. 2013;14(3):205–210. doi:10.1038/ni.2537.
- Wu Y, Cheng X, Jiang G, Tang H, Ming S, Tang L, Lu J, Guo C, Shan H, Huang X. Altered oral and gut microbiota and its association with SARS-CoV-2 viral load in COVID-19 patients during hospitalization. Npj Biofilms Microbio [Internet]. 2021;7(1):61. doi:10.1038/s41522-021-00232-5.
- Liu Q, Mak JWY, Su Q, Yeoh YK, Lui GCY, Ng SSS, Zhang F, Li AYL, Lu W, Hui DSC, et al. Gut microbiota dynamics in a prospective cohort of patients with post-acute COVID-19 syndrome. Gut [Internet]. 2022;71(3):544–552. doi:10.1136/gutjnl-2021-325989.
- Zollner A, Koch R, Jukic A, Pfister A, Meyer M, Rössler A, Kimpel J, Adolph TE, Tilg H. Postacute COVID-19 is characterized by gut viral antigen persistence in inflammatory bowel diseases. Gastroenterol [Internet]. 2022;163(2):495–506.e8. doi:10.1053/j.gastro.2022.04.037.
- Bowerman KL, Rehman SF, Vaughan A, Lachner N, Budden KF, Kim RY, Wood DLA, Gellatly SL, Shukla SD, Wood LG, et al. Disease-associated gut microbiome and metabolome changes in patients with chronic obstructive pulmonary disease. Nat Commun [Internet]. 2020;11(1):5886. doi:10.1038/s41467-020-19701-0.
- Fernández-de-Las-Peñas C, Palacios-Ceña D, Gómez-Mayordomo V, Cuadrado ML, Florencio LL. Defining post-COVID symptoms (post-acute COVID, long COVID, persistent post-COVID): an integrative classification. Int J Environ Res Public Health. 2021;18(5):18. doi:10.3390/ijerph18052621.
- Caugant DA, Levin BR, Selander RK. Distribution of multilocus genotypes of escherichia coli within and between host families. J Hyg (Lond). 1984;92(3):377–384. doi:10.1017/S0022172400064597.
- Song SJ, Lauber C, Costello EK, Lozupone CA, Humphrey G, Berg-Lyons D, Caporaso JG, Knights D, Clemente JC, Nakielny S, et al. Cohabiting family members share microbiota with one another and with their dogs. Elife. 2013;2. doi:10.7554/eLife.00458.
- Souza DG, Vieira AT, Soares AC, Pinho V, Nicoli JR, Vieira LQ, Teixeira MM. The essential role of the intestinal microbiota in facilitating acute inflammatory responses. J Immunol. 2004;173(6):4137–4146. doi:10.4049/jimmunol.173.6.4137.
- M C CC, L NL, C M F, J L G, D A, C G, G C, S H P, C M, F S M, et al. Comparing the effects of acute alcohol consumption in germ-free and conventional mice: the role of the gut microbiota. BMC Microbiol. 2014;14(1):240. doi:10.1186/s12866-014-0240-4.
- Rungue M, Melo V, Martins D, Campos PC, Leles G, Galvão I, Mendes V, Aganetti M, Pedersen Á, Assis NRG, et al. NLRP6-associated host microbiota composition impacts in the intestinal barrier to systemic dissemination of brucella abortus. PLoS Negl Trop Dis. 2021;15(2):e0009171. doi:10.1371/journal.pntd.0009171.
- Zhang F, Wan Y, Zuo T, Yeoh YK, Liu Q, Zhang L, Zhan H, Lu W, Xu W, Lui GCY, et al. Prolonged impairment of short-chain fatty acid and L-Isoleucine biosynthesis in gut microbiome in patients with COVID-19. Gastroenterol. 2022;162(2):548–561.e4. doi:10.1053/j.gastro.2021.10.013.
- Galvão I, Tavares LP, Corrêa RO, Fachi JL, Rocha VM, Rungue M, Garcia CC, Cassali G, Ferreira CM, Martins FS, et al. The metabolic sensor GPR43 receptor plays a role in the control of Klebsiella pneumoniae infection in the lung. Front Immunol. 2018;9. http://journal.frontiersin.org/article/10.3389/fimmu.2018.00142/full.
- Vieira AT, Rocha VM, Tavares L, Garcia CC, Teixeira MM, Oliveira SC, Cassali GD, Gamba C, Martins FS, Nicoli JR. Control of klebsiella pneumoniae pulmonary infection and immunomodulation by oral treatment with the commensal probiotic bifidobacterium longum 51A. Microbes Infect. 2016;18(3):180–189. doi:10.1016/j.micinf.2015.10.008.
- Wu T, Xu F, Su C, Li H, Lv N, Liu Y, Gao Y, Lan Y, Li J. Alterations in the gut microbiome and cecal metabolome during klebsiella pneumoniae-induced pneumosepsis. Front Immunol. 2020;11:1331. doi:10.3389/fimmu.2020.01331.
- Douaud G, Lee S, Alfaro-Almagro F, Arthofer C, Wang C, McCarthy P, Lange F, Andersson JLR, Griffanti L, Duff E. SARS-CoV-2 is associated with changes in brain structure in UK biobank. Nature. 2022;604(7907):697–707. doi:10.1038/s41586-022-04569-5.
- Hartung TJ, Neumann C, Bahmer T, Chaplinskaya-Sobol I, Endres M, Geritz J, Haeusler KG, Heuschmann PU, Hildesheim H, Hinz A. Fatigue and cognitive impairment after COVID-19: a prospective multicentre study. EClinicalMed. 2022;53:101651. doi:10.1016/j.eclinm.2022.101651.
- Heine J, Schwichtenberg K, Hartung TJ, Rekers S, Chien C, Boesl F, Rust R, Hohenfeld C, Bungenberg J, Costa AS. Structural brain changes in patients with post-COVID fatigue: a prospective observational study. Eclinicalmed. 2023;58. doi:10.1016/j.eclinm.2023.101874.
- Andrade SP, Campolina-Silva GH, Queiroz-Junior CM, de Oliveira LC, de Lacerda L, Gaggino JCP, de Souza FRO, de Meira Chaves I, Passos IB, Teixeira DC, et al. A biosafety level 2 mouse model for studying betacoronavirus-induced acute lung damage and systemic manifestations. J Virol. 2021;95(22). doi:10.1128/JVI.01276-21.
- da Silva JGV, Vieira AT, Sousa TJ, Viana MVC, Parise D, Sampaio B, da Silva AL, de Jesus LCL, de Carvalho PKRML, de Castro Oliveira L, et al. Comparative genomics and in silico gene evaluation involved in the probiotic potential of Bifidobacterium longum 51A. Gene. 2021;795:145781. doi:10.1016/j.gene.2021.145781.
- Xu E, Xie Y, Al-Aly Z. Long-term gastrointestinal outcomes of COVID-19. Nat Commun [Internet]. 2023;14(1):983. doi:10.1038/s41467-023-36223-7.
- Livanos AE, Jha D, Cossarini F, Gonzalez-Reiche AS, Tokuyama M, Aydillo T, Parigi TL, Ladinsky MS, Ramos I, Dunleavy K, et al. Intestinal host response to SARS-CoV-2 infection and COVID-19 outcomes in patients with gastrointestinal symptoms. Gastroenterol [Internet]. 2021;160(7):2435–2450.e34. doi:10.1053/j.gastro.2021.02.056.
- Merad M, Blish CA, Sallusto F, Iwasaki A. The immunology and immunopathology of COVID-19. Science (80-) [Internet]. 2022;375(6585):1122–1127. doi:10.1126/science.abm8108.
- Wu Y, Cheng X, Jiang G, Tang H, Ming S, Tang L, Lu J, Guo C, Shan H, Huang X. Author correction: altered oral and gut microbiota and its association with SARS-CoV-2 viral load in COVID-19 patients during hospitalization. Npj Biofilms Microbio [Internet]. 2021;7(1):90. doi:10.1038/s41522-021-00262-z.
- Langford BJ, So M, Simeonova M, Leung V, Lo J, Kan T, Raybardhan S, Sapin ME, Mponponsuo K, Farrell A. Antimicrobial resistance in patients with COVID-19: a systematic review and meta-analysis. Lancet Microbe. 2022. doi:10.2139/ssrn.4099404.
- Langford BJ, Soucy JP, Leung V, So M, Kwan ATH, Portnoff JS, Bertagnolio S, Raybardhan S, MacFadden D, Daneman N. Antibiotic resistance associated with the COVID-19 pandemic: a systematic review and meta-analysis. Clin Microbiol Infect 2022;29(3):302–309. doi: 10.1016/j.cmi.2022.12.006.
- de Nies L, Galata V, Martin-Gallausiaux C, Despotovic M, Busi SB, Snoeck CJ, Delacour L, Budagavi DP, Laczny CC, Habier J, et al. Altered infective competence of the human gut microbiome in COVID-19. Microbiome. 2023;11(1):46. doi:10.1186/s40168-023-01472-7.
- Bernard-Raichon L, Venzon M, Klein J, Axelrad JE, Zhang C, Sullivan AP, Hussey GA, Casanovas-Massana A, Noval MG, Valero-Jimenez AM, et al. Gut microbiome dysbiosis in antibiotic-treated COVID-19 patients is associated with microbial translocation and bacteremia. Nat Commun [Internet]. 2022;13(1):5926. doi:10.1038/s41467-022-33395-6.
- Kariyawasam RM, Julien DA, Jelinski DC, Larose SL, Rennert-May E, Conly JM, Dingle TC, Chen JZ, Tyrrell GJ, Ronksley PE, et al. Antimicrobial resistance (AMR) in COVID-19 patients: a systematic review and meta-analysis (November 2019–June 2021). Antimicrob Resist Infect Control [Internet]. 2022;11(1):45. doi:10.1186/s13756-022-01085-z.
- López-Jácome LE, Fernández-Rodríguez D, Franco-Cendejas R, Camacho-Ortiz A, Morfin-Otero MDR, Rodríguez-Noriega E, Ponce-de-León A, Ortiz-Brizuela E, Rojas-Larios F, Velázquez-Acosta MDC, et al. Increment antimicrobial resistance during the COVID-19 pandemic: results from the invifar network. Microb Drug Resist. 2022;28(3):338–345. doi:10.1089/mdr.2021.0231.
- Furlan L, Caramelli B. The regrettable story of the “covid kit” and the “early treatment of Covid-19” in Brazil. Lancet Reg Health Am. 2021;4:100089. doi:10.1016/j.lana.2021.100089.
- Jeon K, Jeong S, Lee N, Park MJ, Song W, Kim HS, Kim HS, Kim JS. Impact of COVID-19 on antimicrobial consumption and spread of multidrug-resistance in bacterial infections. Antibiotic. 2022;11(4):11. doi:10.3390/antibiotics11040535.
- Karami Z, Knoop BT, Dofferhoff ASM, Blaauw MJT, Janssen NA, van Apeldoorn M, Kerckhoffs APM, van de Maat JS, Hoogerwerf JJ, ten Oever J. Few bacterial co-infections but frequent empiric antibiotic use in the early phase of hospitalized patients with COVID-19: results from a multicentre retrospective cohort study in the Netherlands. Infect Dis. 2021;53(2):102–110. doi:10.1080/23744235.2020.1839672.
- Van Laethem J, Wuyts S, Van Laere S, Koulalis J, Colman M, Moretti M, Seyler L, De Waele E, Pierard D, Lacor P, et al. Antibiotic prescriptions in the context of suspected bacterial respiratory tract superinfections in the COVID-19 era: a retrospective quantitative analysis of antibiotic consumption and identification of antibiotic prescription drivers. Intern Emerg Med [Internet]. 2022;17(1):141–151. doi:10.1007/s11739-021-02790-0.
- Bazaid AS, Barnawi H, Qanash H, Alsaif G, Aldarhami A, Gattan H, Alharbi B, Alrashidi A, Al-Soud WA, Moussa S, et al. Bacterial coinfection and antibiotic resistance profiles among hospitalised COVID-19 patients. Microorgan. 2022;10(3):495. doi:10.3390/microorganisms10030495.
- Russell CD, Fairfield CJ, Drake TM, Turtle L, Seaton RA, Wootton DG, Sigfrid L, Harrison EM, Docherty AB, de Silva TI, et al. Co-infections, secondary infections, and antimicrobial use in patients hospitalised with COVID-19 during the first pandemic wave from the ISARIC WHO CCP-UK study: a multicentre, prospective cohort study. The Lancet Microbe [Internet]. 2021;2(8):e354–65. doi:10.1016/S2666-5247(21)00090-2.
- Arcari G, Raponi G, Sacco F, Bibbolino G, Di Lella FM, Alessandri F, Coletti M, Trancassini M, Deales A, Pugliese F, et al. Klebsiella pneumoniae infections in COVID-19 patients: a 2-month retrospective analysis in an Italian hospital. Int J Antimicrob Agents. 2021;57(1):106245. doi:10.1016/j.ijantimicag.2020.106245.
- Chong WH, Saha BK, Ramani A, Chopra A. State-of-the-art review of secondary pulmonary infections in patients with COVID-19 pneumonia. Infection [Internet]. 2021;49(4):591–605. doi:10.1007/s15010-021-01602-z.
- Gerver SM, Guy R, Wilson K, Thelwall S, Nsonwu O, Rooney G, Brown CS, Muller-Pebody B, Hope R, Hall V. National surveillance of bacterial and fungal coinfection and secondary infection in COVID-19 patients in England: lessons from the first wave. Clin Microbiol Infect. 2021;27(11):1658–1665. doi:10.1016/j.cmi.2021.05.040.
- Protonotariou E, Mantzana P, Meletis G, Tychala A, Kassomenaki A, Vasilaki O, Kagkalou G, Gkeka I, Archonti M, Kati S, et al. Microbiological characteristics of bacteremias among COVID-19 hospitalized patients in a tertiary referral hospital in Northern Greece during the second epidemic wave. FEMS Microbes [Internet]. 2022;2. doi:10.1093/femsmc/xtab021/6448474.
- Zheng D, Liwinski T, Elinav E. Interaction between microbiota and immunity in health and disease. Cell Res [Internet]. 2020;30(6):492–506. doi:10.1038/s41422-020-0332-7.
- Jang YO, Kim OH, Kim SJ, Lee SH, Yun S, Lim SE, Yoo HJ, Shin Y, Lee SW. High-fiber diets attenuate emphysema development via modulation of gut microbiota and metabolism. Sci Rep. 2021;11(1):7008. doi:10.1038/s41598-021-86404-x.
- Sampson TR, Mazmanian SK. Control of brain development, function, and behavior by the microbiome. Cell Host & Microbe. 2015;17(5):565–576. doi:10.1016/j.chom.2015.04.011.
- Bajinka O, Simbilyabo L, Tan Y, Jabang J, Saleem SA. Lung-brain axis. Crit Rev Microbiol [Internet]. 2022;48(3):257–269. doi:10.1080/1040841X.2021.1960483.
- Hosang L, Canals RC, van der Flier FJ, Hollensteiner J, Daniel R, Flügel A, Odoardi F. The lung microbiome regulates brain autoimmunity. Nature [Internet]. 2022;603(7899):138–144. doi:10.1038/s41586-022-04427-4.
- Monje M, Iwasaki A. The neurobiology of long COVID. Neuron [Internet]. 2022;110(21):3484–3496. doi:10.1016/j.neuron.2022.10.006.
- Taquet M, Geddes JR, Husain M, Luciano S, Harrison PJ. 6-month neurological and psychiatric outcomes in 236 379 survivors of COVID-19: a retrospective cohort study using electronic health records. The Lancet Psych [Internet]. 2021;8(5):416–427. doi:10.1016/S2215-0366(21)00084-5.
- Yang I, Arthur RA, Zhao L, Clark J, Hu Y, Corwin EJ, Lah J. The oral microbiome and inflammation in mild cognitive impairment. Exp Gerontol. 2021;147:111273. doi:10.1016/j.exger.2021.111273.
- Erny D, Hrabě de Angelis AL, Jaitin D, Wieghofer P, Staszewski O, David E, Keren-Shaul H, Mahlakoiv T, Jakobshagen K, Buch T, et al. Host microbiota constantly control maturation and function of microglia in the CNS. Nat Neurosci [Internet]. 2015;18(7):965–977. doi:10.1038/nn.4030.
- Dang AT, Marsland BJ. Microbes, metabolites, and the gut–lung axis. Mucosal Immunol [Internet]. 2019;12(4):843–850. doi:10.1038/s41385-019-0160-6.
- Doifode T, Giridharan VV, Generoso JS, Bhatti G, Collodel A, Schulz PE, Forlenza OV, Barichello T. The impact of the microbiota-gut-brain axis on Alzheimer’s disease pathophysiology. Pharmacol Res. 2021;164:105314. doi:10.1016/j.phrs.2020.105314.
- Saia RS, Giusti H, Luis-Silva F, Pedroso K, Auxiliadora-Martins M, Morejón KML, Degiovani AM, Cadelca MR, Basile-Filho A. Clinical investigation of intestinal fatty acid-binding protein (I-FABP) as a biomarker of SARS-CoV-2 infection. Int J Infect Dis [Internet]. 2021;113:82–86. doi:10.1016/j.ijid.2021.09.051.
- Mańkowska-Wierzbicka D, Zuraszek J, Wierzbicka A, Gabryel M, Mahadea D, Baturo A, Zakerska-Banaszak O, Slomski R, Skrzypczak-Zielinska M, Dobrowolska A. Alterations in gut microbiota composition in patients with COVID-19: a pilot study of whole hypervariable 16S rRNA gene sequencing. Biomed. 2023;11(2):11. doi:10.3390/biomedicines11020367.
- Al Bataineh MT, Henschel A, Mousa M, Daou M, Waasia F, Kannout H, Khalili M, Kayasseh MA, Alkhajeh A, Uddin M. Gut microbiota interplay with COVID-19 reveals links to host lipid metabolism among middle eastern populations. Front Microbiol. 2021;12:12. doi:10.3389/fmicb.2021.761067.
- Jang SE, Lim SM, Jeong JJ, Jang HM, Lee HJ, Han MJ, Kim DH. Gastrointestinal inflammation by gut microbiota disturbance induces memory impairment in mice. Mucosal Immunol. 2018;11(2):369–379. doi:10.1038/mi.2017.49.
- Kim N, Jeon SH, Ju IG, Gee MS, Do J, Oh MS, Lee JK. Transplantation of gut microbiota derived from alzheimer’s disease mouse model impairs memory function and neurogenesis in C57BL/6 mice. Brain Behav Immun [Internet]. 2021;98:357–365. doi:10.1016/j.bbi.2021.09.002.
- Vieira AT, Galvão I, Amaral FA, Teixeira MM, Nicoli JR, Martins FS. Oral treatment with bifidobacterium longum 5 1A reduced inflammation in a murine experimental model of gout. Benef Microbes [Internet]. 2015;6(6):799–806. doi:10.3920/BM2015.0015.
- Alharbi KS, Singh Y, Hassan Almalki W, Rawat S, Afzal O, Alfawaz Altamimi AS, Kazmi I, Al-Abbasi FA, Alzarea SI, Singh SK, et al. Gut microbiota disruption in COVID-19 or post-COVID illness association with severity biomarkers: a possible role of pre/pro-biotics in manipulating microflora. Chem Biol Interact. 2022;358:109898. doi:10.1016/j.cbi.2022.109898.
- Mukhopadhya I, Segal JP, Carding SR, Hart AL, Hold GL. The gut virome: the “missing link” between gut bacteria and host immunity? Therap Adv Gastroenterol. 2019;12:1756284819836620. doi:10.1177/1756284819836620.
- Yuan L, Hensley C, Mahsoub HM, Ramesh AK, Zhou P. Microbiota in viral infection and disease in humans and farm animals. Prog Mol Biol Transl Sci. 2020;171:15–60. doi:10.1016/bs.pmbts.2020.04.005.
- Lu ZH, Zhou HW, Wu WK, Fu T, Yan M, He Z, Sun SW, Ji ZH, Shao Z. Alterations in the composition of intestinal DNA virome in patients with COVID-19 [Internet]. Front Cell Infect Microbiol. 2021;11:11. doi:10.3389/fcimb.2021.790422.
- Natarajan A, Zlitni S, Brooks EF, Vance SE, Dahlen A, Hedlin H, Park RM, Han A, Schmidtke DT, Verma R, et al. Gastrointestinal symptoms and fecal shedding of SARS-CoV-2 RNA suggest prolonged gastrointestinal infection. Med [Internet]. 2022;3(6):371–387.e9. doi:10.1016/j.medj.2022.04.001.
- Coryell MP, Iakiviak M, Pereira N, Murugkar PP, Rippe J, Williams DB, Heald-Sargent T, Sanchez-Pinto LN, Chavez J, Hastie JL, et al. A method for detection of SARS-CoV-2 RNA in healthy human stool: a validation study. Lancet Microbe. 2021;2(6):e259–66. doi:10.1016/S2666-5247(21)00059-8.
- Bolyen E, Rideout JR, Dillon MR, Bokulich NA, Abnet CC, Al-Ghalith GA, Alexander H, Alm EJ, Arumugam M, Asnicar F, et al. Reproducible, interactive, scalable and extensible microbiome data science using QIIME 2. Nat Biotechnol. 2019;37(8):852–857. doi:10.1038/s41587-019-0209-9.
- Rognes T, Flouri T, Nichols B, Quince C, Mahé F. VSEARCH: a versatile open source tool for metagenomics. PeerJ. 2016;4:e2584. doi:10.7717/peerj.2584.
- Quast C, Pruesse E, Yilmaz P, Gerken J, Schweer T, Yarza P, Peplies J, Glöckner FO. The SILVA ribosomal RNA gene database project: improved data processing and web-based tools. Nucleic Acids Res. 2012;41(D1):D590–6. doi:10.1093/nar/gks1219.
- Love M, Huber W, Anders S. Moderated estimation of fold change and dispersion for RNA-seq data with DESeq2. Genome Biol. 2014;15(12):10–1186. doi:10.1186/s13059-014-0550-8.
- Blighe K, Sharmila Rana ML. Enhanced volcano: publication-ready volcano plots with enhanced colouring and labeling. R Packag Version 1. 2019. https://bioconductor.org/packages/devel/bioc/vignettes/EnhancedVolcano/inst/doc/EnhancedVolcano.html.
- Assis GBN, Pereira FL, Zegarra AU, Tavares GC, Leal CA, Figueiredo HCP. Use of MALDI-TOF mass spectrometry for the fast identification of gram-positive fish pathogens. Front Microbiol. 2017;8. doi:10.3389/fmicb.2017.01492.
- Vieira AT, Macia L, Galvão I, Martins FS, Canesso MCC, Amaral FA, Garcia CC, Maslowski KM, De Leon E, Shim D, et al. A role for gut microbiota and the metabolite-sensing receptor GPR43 in a murine model of gout. Arthritis & Rheumatol. 2015;67(6):1646–1656. doi:10.1002/art.39107.
- Garcia CC, Russo RC, Guabiraba R, Fagundes CT, Polidoro RB, Tavares LP, Salgado APC, Cassali GD, Sousa LP, Machado AV, et al. Platelet-activating factor receptor plays a role in lung injury and death caused by influenza a in mice. PLoS Pathog. 2010;6(11):e1001171. doi:10.1371/journal.ppat.1001171.
- Horvat JC, Beagley KW, Wade MA, Preston JA, Hansbro NG, Hickey DK, Kaiko GE, Gibson PG, Foster PS, Hansbro PM. Neonatal chlamydial infection induces mixed T-cell responses that drive allergic airway disease. Am J Respir Crit Care Med. 2007;176(6):556–564. doi:10.1164/rccm.200607-1005OC.
- Profeta R, Seyffert N, Tiwari S, Viana MVC, Jaiswal AK, Caetano AC, Bücker DH, Tavares de Oliveira L, Santos R, Gala-Garcia A, et al. Comparative genomics with a multidrug-resistant Klebsiella pneumoniae isolate reveals the panorama of unexplored diversity in Northeast Brazil. Gene. 2021;772:145386. doi:10.1016/j.gene.2020.145386.
- Melo HM, Seixas da Silva GS, Sant’ana MR, Teixeira CVL, Clarke JR, Miya Coreixas VS, de Melo BC, Fortuna JTS, Forny-Germano L, Ledo JH, et al. Palmitate is increased in the cerebrospinal fluid of humans with obesity and induces memory impairment in mice via pro-inflammatory TNF-α. Cell Rep. 2020;30(7):2180–2194.e8. doi:10.1016/j.celrep.2020.01.072.
- Santos SS, Miranda VC, Trindade LM, Cardoso VN, Reis DC, Cassali GD, Nicoli JR, Cara DC, Martins FS. Bifidobacterium longum subsp. longum 51A attenuates signs of inflammation in a murine model of food allergy. Probiotics Antimicrob Proteins [Internet]. 2021;15(1):63–73. doi:10.1007/s12602-021-09846-9.