ABSTRACT
The Artificial Gravity Bed Rest – European Space Agency (AGBRESA) study was the first joint bed rest study by ESA, DLR, and NASA that examined the effect of simulated weightlessness on the human body and assessed the potential benefits of artificial gravity as a countermeasure in an analog of long-duration spaceflight. In this study, we investigated the impact of simulated microgravity on the gut microbiome of 12 participants during a 60-day head-down tilt bed rest at the :envihab facilities. Over 60 days of simulated microgravity resulted in a mild change in the gut microbiome, with distinct microbial patterns and pathway expression in the feces of the countermeasure group compared to the microgravity simulation-only group. Additionally, we found that the countermeasure protocols selectively increased the abundance of beneficial short-chain fatty acids in the gut, such as acetate, butyrate, and propionate. Some physiological signatures also included the modulation of taxa reported to be either beneficial or opportunistic, indicating a mild adaptation in the microbiome network balance. Our results suggest that monitoring the gut microbial catalog along with pathway clustering and metabolite profiling is an informative synergistic strategy to determine health disturbances and the outcome of countermeasure protocols for future space missions.
Plain Language Summary
The future of spaceflight will involve missions beyond the International Space Station or the Moon and astronaut’s health will be challenged by a harsh space environment for longer periods. In the last decade, the intestine has gained importance in dictating overall physiology and we explore it as an additional indicator of health during our ground-based bed rest study simulating microgravity for 60 days. Through the analysis of fecal proteins, we compile the catalog of microbes colonizing the gut of the 12 participants along with the implicated biological activity of the proteins and another 9 lipid analytes. We found specific microbes associated with recovery or healthy status in our subjects to be increased during spaceflight countermeasure conditions and inverse observations in subjects subjected to perilous spaceflight simulation. Our approach improves the functional characterization of the gut by the use of noninvasive methodology correlating the microbial composition of human stool samples with physiological status.
Introduction
After several decades in low Earth orbit onboard the International Space Station, astronauts will embark on exploration missions beyond the Moon and be exposed to a harsh space environment affecting psychological and physiological performance and health.Citation1 Environmental stresses such as radiation, microgravity, poor nutrition as well as isolation, confinement, and sleep deprivation can collectively lead to immune dysfunction, anxiety, cognitive impairment, opportunistic infections, skin irritations, gastrointestinal dysfunction, and musculoskeletal as well as cardiopulmonary deconditioning.Citation2,Citation3 Changes in the human intestinal microbiome, which interacts with multiple physiological pathways, could mediate environmental influences and serve as a target for countermeasures.
The intestinal system harbors extremely rich and diverse environments.Citation4,Citation5 Gut microbes, especially probiotic strains, ferment complex carbohydrates and produce short-chain fatty acid (SCFA) metabolites like acetate, butyrate, and propionate. They play a vital role in maintaining intestinal homeostasis and have been associated with numerous health benefits. Acetate serves as an important energy source for various cells, including colonocytes, supporting gut barrier integrity. Butyrate acts as a key energy substrate for colonocytes and has anti-inflammatory effects. It enhances gut barrier function, modulates immune responses, and promotes the differentiation and apoptosis of colon cancer cells. Propionate regulates hepatic gluconeogenesis and lipid metabolism, contributing to metabolic health. Additionally, SCFAs can influence immune cell function and modulate inflammatory responses, thereby impacting immune health. SCFAs also play a role in regulating appetite, energy metabolism, and insulin sensitivity.Citation6 Yet, the shift to proteolytic fermentation upon depletion of these favored substrates,Citation7 when protein fermentation of branched-chain amino acids valine, leucine, and isoleucineCitation8, leads to the concomitant generation of other molecules that can damage intestinal cells and the environment such as ammonia, phenol, p-cresol, or biogenic amines.Citation9 Thus, variations in the gut’s microbial composition directly impact the bacterial fermentation that produces SCFA moieties.Citation10 The biochemical context of gut colonization as an emerging biomedical indicator has been explored in a few studies with a space flight background, where intestinal flora impacts well beyond the role of digestion as a source of nutrients and xenobiotic degradation.Citation3,Citation11–13
The Artificial Gravity Bed Rest European Space Agency (AGBRESA)Citation14–24 study tested whether daily gravitational force training on a short-arm centrifuge attenuates the physiological effects of weightlessness simulated through head-down tilt (HDT) bed rest in human beings. This was the first joint HDT bed rest study by the European Space Agency (ESA), the German Aerospace Center (DLR), and the National Aeronautics and Space Administration Agency (NASA) and took place at the :envihab facilities at the DLR Institute of Aerospace Medicine in Cologne, Germany. Akin to real weightlessness in space, HDT bed rest unloads the human body, thus, producing physiological deconditioning, including bone and muscular tissue loss. Moreover, HDT bed rest produces cephalad fluid shifts in the body, which are also observed in astronauts in space and contribute to spaceflight-associated neuro-ocular syndrome (SANS).Citation25
The AGBRESA study provided a unique opportunity to assess the gut microbiome in a controlled spaceflight analog and investigate the modulation of intestinal microbiota’s taxonomical and functional composition by means of metaproteomics. Additionally, microbe-derived short-chain fatty acids (SCFA) further influencing the gut microenvironment were quantified. This study aims to understand the gut as an indicator of health and whether it reflects the conditioning caused by microgravity simulation and the attempts of mitigation by treatment with centrifugal-based countermeasures.
Results
Profile of the participants of the AGBRESA study
The AGBRESA study evaluated the physiological response of 12 participants (8 males, 4 females, , Fig. S1) to a strict 60-day strict HDT protocol as well as the countermeasure (CM) strategy to offset the damage induced by HDT.Citation24 Specifically, participants were subjected to a strict 6° HDT (head-down tilt) position to replicate the microgravity environment on Earth by triggering fluid shifts in the body. A subset of participants underwent centrifugation protocols of 1–2 G using a short-arm centrifuge for 30 minutes, with two approaches: an intermittent protocol where participants rode the human centrifuge daily for 5 minutes, six consecutive times with a 3-minute interval (HDT & int CM), or had a cumulative, daily continuous 30 min treatment countermeasure protocol (HDT & cont CM).Citation14,Citation24 The primary objective of the study was to investigate the minimum daily duration of artificial gravity required to effectively mitigate deleterious physiological adaptations during 60 days of bed rest. The centrifugation process induces considerable blood pooling toward the feet and the maintain of cardiovascular homeostasis. However, prolonged exposure to the countermeasures could potentially lead to discomfort or pain.Citation24 The AGBRESA study was conducted in a highly controlled setting at the :envihab in Cologne and complied with all standardized NASA and ESA procedures. Participants resided in a research ward where variables such as room lighting, fluid intake, sodium intake, and macronutrient composition were tightly regulated. Caloric intake was individually adjusted to maintain body weight throughout the study. The study consisted of a 15-day baseline phase, 60 days of −6° HDT phase, and a recovery period. During the HDT phase, all daily activities were performed in the -6° HDT position.
Table 1. Characteristics of the participants in the second campaign of the AGBRESA study.
To characterize the gut microbiome and microenvironment of these participants, baseline stool samples were collected on the 5th day of the study (BDC-10). Further stool samples were obtained on the 10th, 30th, and 50th days of the HDT phase (HDT10, HDT30, HDT50) and once during the 9th day of the Recovery phase (R9). Blood analyses were also performed at corresponding time points. Participants reported various discomfort, not always related to the study, and medication was provided as needed (Table S1). These discomforts included headaches, nausea, respiratory congestions, mild viral infections, pre-syncope symptoms, heartburn, gastroesophageal reflux, ear infections, obstipation, dry eyes, eyelid infection, dysmenorrhea, pain at the biopsy site (biopsy obtained from the s soleus muscle to assess muscle strength in a different experimental initiative,Citation26 head and neck pain, back pain, knee, and heel pain. Medication such as anti-inflammatory, analgesic, anti-spasmodic, anti-histamine, intravenous hydration fluids, micro-enemas, mucolytics, decongestants, or eyedrops were administered to alleviate symptoms. Additional measures, such as physiotherapy, were also taken to prevent muscle contractures and alleviate muscle stiffness and back pain.
The metaproteomic profiles show unique microbiota patterns in microgravity simulation and countermeasure application with comparable biodiversity scores
The fecal metaproteomic dataset of the 12 AGBRESA participants resulting from the analysis of the 60 nanoLC-MS/MS runs comprised 4,193,837 MS/MS spectra with an average of 69,897 ± 1,418 MS/MS obtained per sample. An average of 37 ± 4% spectra assigned to peptide sequences per sample led to the identification of a total of 58,894 proteins and 36,674 protein groups (Table S2). The Taxon-Spectrum Matches (TSMs) distribution by origin revealed a higher percentage of the microbial signal following 30- and 50-day microgravity simulation in stools of subjects experiencing int CM compared to those undergoing cont CM and controls (). Bacteria belonging to 10 different phyla dominated the microbial fraction of the metaproteomes (65 ± 5%, mean ± SD), followed by fungi of the Ascomycota phylum (5 ± 0.5%). Archaea represented 3 ± 0.3% of the signal, with Euryarchaeota being the most highly represented phylum ().
Figure 1. Metaproteomic output classification according to (a) source and (b) phylum. (c) alpha-diversity scores in inverse Simpson Index and (d) Principal component analysis (PCA) plotting. Green, red, and orange indicate subjects in HDT, HDT & cont CM, and HDT & int CM, respectively. Each point corresponds to one sample at one specific time point during the study. (e) heatmap representing the relative abundance of 70 genera clustered by individual samples of the AGBRESA participants.
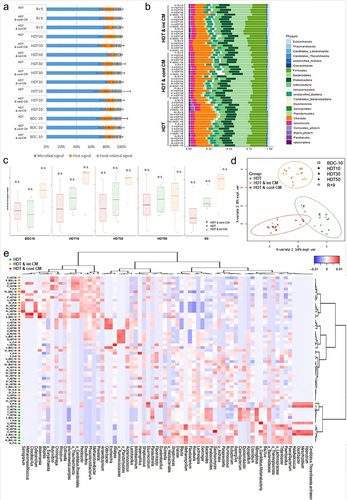
The Firmicutes/Bacteroidetes ratio (F/B ratio) quantification revealed no significant differences during HDT and HDT & CM protocols compared to baseline (Mann Whitney test, P < .05). Similarly, gut microbial biodiversity in AGBRESA participants (Tukey’s Honest Significant Difference) did not vary, even though HDT & int CM had slightly higher alpha diversity (Inverse Simpson Index scores) during the entire study except for time point HDT50 ().
According to the Bray-Curtis Index, the microbial composition based on the dissimilarity between samples did not cluster, indicating diversity between individuals was more substantial than the temporal dynamics of each subject’s microbiota during the study (Fig. S2). On the other hand, the partial least squares-discriminant analysis (PLS-DA) of metaproteomic profiles () revealed discriminative microbial signatures in each intervention group. Microgravity simulation alone (HDT) had the most noticeable impact on genera of the Clostridia class that tended to increase, with the Faecalibacterium genus showing the most important changes (, in green). A similar trend was found for the genera Paraprevotella, Bacteroides, Parabacteriodes, Roseburia, Gemmiger, Monoglobus, Mesorhizobium, Methanospirilium, Oscilibacter, Halorubrum, Dialaster, Halalkalicoccus, Flavobacterium, Candida, Saccharomyces, Azospirillum, Tidjanibacter, Bacillus, Blautia, Clostridium, Catenibacterium, Paenibacillus, Streptococcus, Aspergillus, Duodenibacillus and Eubacterium, which progressively increased in proportion. Conversely, the HDT treatment resulted in the decrease of genera Duodenibacillus, Elusimicrobium, and Streptomyces.
Moreover, the PLS-DA analysis of the CM groups also revealed trends regarding specific genera and their temporal dynamics (, in red). While the microbiota of subjects under cont CM revealed minimal remodeling of community structure, its signature during the recovery phase was extensively impacted compared with BDC-10. Here, HDT & cont CM predominantly resulted in decreased abundances of several genera, such as Methanobrevibacter, Prevotella, Phascolarctobacterium, Thaumarchaeota, Candida, Collinsella, Desulfovibrio, Pedobacter, Alistipes, Colletotrichum, Butyrivibrio, Haemophilus, Methanomassilicocus, Salinigranun, Cloacibacillus, Akkermansia, Rhizobium, Pedobacter, Kazachstania, and Haemophilus. Finally, the HDT & int CM treatment (, in orange) was distinctive by the increase of genera, including Dysosmobacter, Lachnospira, Bifidobacterium, Lactobacillus, Parasutterella, Acidaminococcus, Escherichia, Odoribacter, Sphingomonas, Acidianus, Halorussus, Megamonas, Gechnospira, Evtepia, Ruminococcus, and Bifidobacterium. In contrast, the genus Flavonifractor decreased in relative abundance during this treatment. Interestingly, the clustering of each of the fecal samples by the similarity of the microbiota profiles organized the microgravity simulation with both protocols of countermeasures in HDT & int CM and HDT & cont CM with an increased similarity between them and isolated the HDT condition alone in a different cluster. Outlier profiles from individuals Y and Q1 were observed at specific time points.
The metaproteomic profiles reveal a different impact on the functional dynamics, without altering bowel disease biomarker abundance
To investigate the functional dynamics of the microbial metaproteomic signatures captured during HDT and HDT & CM, proteins identified with a false discovery rate (FDR) of 1% were annotated, and their functions were assigned to 171 KEGG pathways (Table S3). The relative abundance of each pathway was calculated at the phylum level to discriminate different expression profiles without losing information from unambiguous assignments at a finer resolution.
Under HDT, dynamic functional changes (log2FC) were observed for the pathways related to drug resistance – antimicrobial, lipid and co-factors and vitamins metabolism of the Proteobacteria phylum (). Additionally, the substance dependence-related pathways of the Ascomycota also showed some dynamic alterations. On the contrary, pathways related to the terpenoids and polyketides metabolism of the Actinobacteria phylum as well as the metabolism of other amino acids of the Ascomycota were dynamically impacted also during HDT & cont CM. Pathways related to actinobacterial amino acid and glycan biosynthesis and metabolism were affected under both HDT and HDT & int CM. Verrucomicrobia pathways as those associated to transport and catabolism and lipid metabolism appeared to be mainly altered under the countermeasures ().
Figure 2. Heatmap with metaproteomic output assigned to KEGG pathway maps of (a) microbial and (b) Chordata phyla.
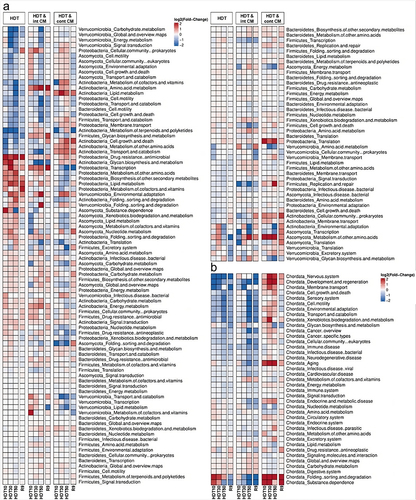
To investigate the dynamics of host-associated functions, proteins belonging to the host phylum Chordata were assigned to 39 KEGG pathways (Table S3). Under both HDT and HDT & int CM pathways such as development and regeneration, environmental adaptation, folding, sorting, and degradation were decreased (log2FC) (). Conversely, under HDT & cont CM the abundance of pathways such as aging, folding, sorting and degradation, and substance dependence increased, with the remodeling of the latter persisting during the recovery (R9).
Subsequently, we compared the relative abundances of known gut disease biomarkers for each of the 12 subjects (Fig. S2). Neither the HDT nor the CM expressively shifted the abundance of inflammatory bowel disease biomarkers EPX, beta-glucuronidase, immunoglobulins, Lactoferrin, or Calprotectin when compared with baseline measurements at BCD-10. However, certain individuals showed increased measurements compared to the remaining subjects of AGBRESA, specifically subjects W, N, P, X, or Z.
Centrifugation counteracts harmful SCFA adaptations in the gut microenvironment induced by HDT
To further expand the functional interpretation associated with microgravity simulation treatment and countermeasure application, we studied the abundance of fatty-acid metabolites. The principal component analysis (PCA) of SCFA moieties in the samples from AGBRESA participants did not reveal any difference among time points or conditions (). Nonetheless, the variance among groups tended to decrease within the CM conditions suggesting a convergence in metabolism and SCFA profiles.
Figure 3. Centrifugation countermeasure protocols produce favorable indicators on the gut microenvironments of the AGBRESA participants. (a) Principal component analysis of SCFA log2 fold changes to the baseline (BDC10) of each time point for each sample group with bar chart depicting the areas of calculated the 95% confidence ellipsis. (b) mean relative abundance of SCFAs to summed total abundance of SCFAs in the three treatment groups at each time point. (c) mean concentrations of SCFA converted to z-scores in the three treatment groups at each time point.
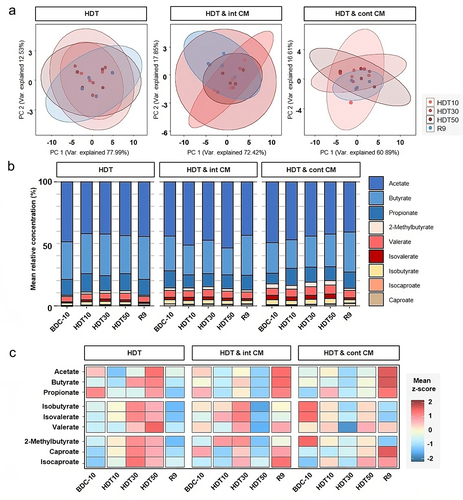
SCFA measurements, pooled by time point and condition (), revealed that acetate, butyrate, and propionate, known to exert beneficial effects,Citation27 made up above 85% of all SCFAs. While moieties diverged between samples, the cumulative amount was maintained over time. Valerate, isovalerate, and isobutyrate, labeled as putrefactive byproducts,Citation28 accounted for up to 10% of SCFAs and tended to increase with HDT and decline during recovery periods at R9. The response was particularly pronounced in the centrifugation groups. In addition, we further identified caproate, isocaproate, and 2-methylbutyrate, which accounted for less than 5% of SCFAs.
Finally, HDT alone potentiated the abundance of all SCFAs at HDT50 (). All SCFA values plummeted during the recovery period, suggesting physiological adaptation to a stress-inducing protocol. Conversely, both centrifugation CM modulated the response to HDT. HDT & int CM was associated with the reduction in all SCFAs at HDT50 and shifted the SCFA profiles toward beneficial acetate, butyrate, and propionate. Furthermore, in HDT & cont CM, the beneficial SCFAs peaked during the recovery period, whereas those described as putrefactive were at their lowest level.
The application of countermeasure protocols correlates SCFA moieties with lipid metabolism and immune system indicators and metabolic pathways
We then compared the trends in subclinical medical findings (Table S4) to SCFA profiles (). We observed associations during HDT & cont CM between Cholesterol, HDL, and LDL biomarkers with valerate, isovalerate and isobutyrate, suggesting that the decreased SCFAs occur with the reduction of lipid metabolism biomarkers. Furthermore, the increased levels of Creatine Kinase and LDH, muscle and tissue damage biomarkers, also correlated with butyrate rise. On the other hand, HDT & int CM showed, in most cases, negative associations between the levels of neutrophils and leukocytes and acetate, butyrate, propionate and valerate profiles, indicating that these SCFAs were inversely linked to the levels of systemic inflammatory biomarkers. Also, while ALP levels and butyrate, propionate, and valerate were negatively correlated, lipase and isobutyrate levels had a positive association.
Figure 4. Centrifugation countermeasure protocols correlate with blood biomarkers of lipid metabolism. Correlation analysis of concentration of SCFAs at all time points with clinical data for each treatment.
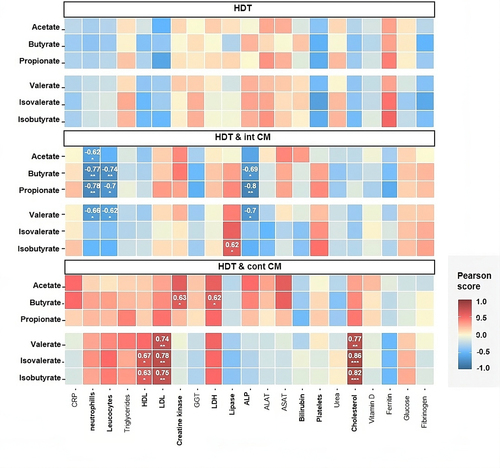
Similarly, the correlation between SCFA profiles and functional metaproteomics (Fig. S4) revealed consistent trends related to valerate, isovalerate, and isobutyrate and host-related pathways carbohydrate metabolism, digestive system, lipid metabolism and endocrine system and global overview maps. In microorganisms, carbohydrate metabolism, biosynthesis of other secondary metabolites, antineoplastic drug resistance and energy metabolism pathways correlated with the abundances of valerate, isovalerate, and isobutyrate. Conversely, acetate, butyrate, and propionate profiles did not display consistent correlation patterns with any KEGG pathway.
Discussion
Mild taxonomic adaptations of microbial communities occurred in the gut microbiome during microgravity simulation
The AGBRESA study analyzed the effects of simulated microgravity, which incorporated centrifugation countermeasures to aid physiological deconditioning. The gut has been considered a key organ dictating overall health, where the gut microbiome and its metabolites serve as an indicator of disease but are also a target of medical intervention.Citation29–31 Therefore, we aimed to study the gut microbiota and metabolites as potential health indicators during spaceflight.
Our metaproteomic analysis allowed for a taxonomical and functional interpretation by means of massive peptide sequencing.Citation32 The ecological analysis of the microbial data did not show significant differences between the diversity scores under the HDT with or without CM, highlighting that each individual’s microbiota has a unique adaptation response that is not coordinated with the stimulus. The distance between individuals was more substantial than the temporal dynamics of each subject’s microbiota, confirming that diversity scores may only be informative during drastic fluctuations associated with antibiotic intake or severe bowel disorders.Citation29,Citation30 Therefore, biodiversity scores of healthy subjects, like those selected for AGBRESA, would not illustrate the impact of mild stimuli.Citation31 Additionally, it is important to note that ecological notions alone may not be sufficient to retrieve functional interpretations,Citation33 as diversity indexes only provide a condensed evaluation of the ecosystem’s fitness and do not evaluate dysfunction, such as dysbiosis.
A closer examination of the microbiota temporal dynamics suggested the harm predicted with microgravity simulation by HDT would be associated with an increase of bacteria previously correlated with a sedentary lifestyle, such as the Paraprevotella genus and representatives of the Desulfovibrionaceae family.Citation34 Additionally, we observed an increase in the abundance of several genera of the Ruminococcaceae family and a decrease in those belonging to the Sutterellaceae family, both traits of healthy individuals. On the other hand, the benefits predicted with countermeasure protocols applied during HDT & CM were associated with a reduction of markers of inflammation such as Dialister, the temporal modulation of Sutterella abundance, and the increase of beneficial microbes such as Dysosmobacter and those identified in the Lachnospiraceae family.Citation35 These microorganisms belong to a commensal category, yet their nonpathogenic status does not render a healthy non-dysbiotic context.Citation36–38 Consistently, Turroni and colleagues found that during space travel, beneficial microbes such as Akkermansia and Ruminococcus decreased while opportunistic ones such as Parasutterella increased.Citation3 Likewise, Liu and colleagues did not report any variations in diversity during a short-term mission of 2 weeks, where an increase in Bacteroides was detected in agreement with our results, along with a decrease in probiotic Lactobacillus and Bifidobacterium.Citation12 Similarly, in the MARS500 project, the increased Bacteroides genus and a decrease in Faecalibacterium prausnitziiCitation3 were represented as a response to stress. Conversely, both countermeasure protocols presented many signatures known as protective by either decreasing opportunistic or disease-associated microbes or increasing beneficial probiotic microbes. However, the analysis was impacted by the strong interpersonal differences, as frequently denoted in the literature.Citation39,Citation40
The functional status of the gut is differentially impacted by simulated microgravity and countermeasure application
The functional interpretation of the gut is growing in importance due to the varying microbial composition found in different individuals, which can be influenced by factors such as culture, age, diet, birth delivery, pharmaceuticals or stress.Citation39,Citation40 We analyzed the functional status of the gut metabolites by mapping each phylum’s peptides to the respective functions within the KEGG pathway maps. During the study, we observed distinct changes in the microbiota functional profile. Specifically, we observed an increased expression of Proteobacteria antimicrobial drug resistance, lipid and cofactors and vitamins metabolisms during HDT treatment while under HDT & CM their profiles resembled the baseline expression. Interestingly, an increasing amount of studies identify this phylum as a possible microbial signature of disease.Citation41–43 Furthermore, under HDT & int CM, the glycan biosynthesis and amino acid metabolisms of the Actinobacteria phylum exhibited dynamic but persistent (R9) remodeling. Interestingly, the glycan metabolism strategy of this phylum is similar to that of Firmicutes.Citation44 Intriguingly, during HDT & int CM, the Firmicutes pathway was altered in the opposite direction, with the most substantial change observed at the recovery stage (R9). Additionally, the functional profile of the Ascomycota phylum was transiently altered, primarily under HDT & CM. This phylum is known to impact the gut microbiome of vulnerable patients with COVID-19Citation45 and other diseases, where its abundance and activity increase during harmful scenarios.Citation46
We looked into the biomarkers reported for severe inflammatory disease among the peptides identified in the AGBRESA samples indicating intestinal inflammation,Citation28 risk of occurrence of pro-carcinogens,Citation47 and the severity of inflammation.Citation48,Citation49 Overall, neither the microgravity simulation with HDT nor the countermeasure protocols significantly affected the abundance of severe inflammatory bowel disorder biomarkers, yet these values lack verification with antigen-specific methodology used for clinical diagnosis.
Then, we evaluated the profiles of microbially-produced SCFA metabolites. Even though the literature on the functional role of SCFAs is recent (reviewed inCitation50) it presents a clear link between SCFA levels and multiple GI diseases like Ulcerative colitis or Crohn’s diseaseCitation51 and non-GI diseases like autism, depression, Alzheimer’s or Parkinson’s.Citation52 In healthy individuals, SCFAs that exert beneficial effects are acetate, butyrate, and propionate,Citation53 and constitute the majority of the total SCFA in the AGBRESA participants. We observed that during HDT the proportion between the beneficial-putrefactive SCFAs slightly decreases but rises again to baseline levels during recovery, suggesting that HDT alone tends to potentiate the accumulation of putrefactive SCFAs. During HDT & int or cont CM, the ratio of beneficial-putrefactive SCFAs remains elevated compared to baseline (BDC10), indicating the stabilizing effects provided by centrifugation countermeasures. These results suggest that centrifugation CM promoted a healing environment and stabilized the gut metabolites for those eight participants compared to the four that were subjected to HDT only which had higher levels of amino acid deamination putrefactive products in the gutCitation27,Citation54 classified as maldigestion and malabsorption markers.
The quantitation of SCFA moieties objectively illustrates that the human subjects treated with HDT & CMs benefited from more protective gut metabolites than those in HDT alone. Comparing SCFA abundances to circulating blood biomarkers revealed that all analytes were within the reference interval for each physiological function. Nonetheless, HDT & cont CM showed associations between lipid metabolism analytes and valerate, isovalerate, and isobutyrate, suggesting that its decrease occurs with the reduction of these biomarkers. During HDT & int CM, we observed an inverse correlation in beneficial SCFAs with immune system markers and a direct correlation between lipid metabolism markers and putrefactive SCFAs. Additionally, there was a concerning correlation between increased levels of LDH and Creatine Kinase, signaling muscle or tissue damage, in the HDT & cont CM. This is likely associated with muscle biopsy damage performed for a different line of research within the AGBRESA study.
The gut microbiome and microenvironment as an indicator of health disturbances during space missions
Stressors beyond weightlessness can negatively impact the astronauts’ musculoskeletal system, ocular, immune functions, nervous system, and mental health.Citation1 Countermeasures post-spaceflight aid astronauts’ physiology to be reverted to healthy standards, preventing organism deteriorationCitation14. Within the AGBRESA study, while the centrifugal CMs were well tolerated in terms of cardiovascular loading and produced the same level of physical exertion, the int CM was better tolerated with less motion sickness than the cont CM.Citation14,Citation17 Both CM protocols mitigated the deterioration of muscle function like jumping power in malesCitation15 and improved attention and vigilance scores with superior cognitive performance.Citation17
Our findings also attest to the healthy status of the AGBRESA participants, a pre-selected study criterion, not comparable to profiles of severe bowel disease or spaceflight, making our analysis reflect the impact of a mild stimuli. In a 35-day-long study, 10 healthy males subjected to bedrest had significantly decreased stool frequency, and 60% had an onset of functional constipation.Citation55 Constipation is a danger factor dictating gut dysbiosis and is 16 times more likely to occur in bedridden patientsCitation56 (analogous to the model herein used for microgravity simulation). Stimuli slowing gut motility are connected to a high risk of constipation as it progressively reduces metabolic rateCitation55 while increasing the abundance of Pseudomonas aeruginosa, Campylobacter jejuniCitation57,Citation58 and methane-producing bacteriaCitation59 and reducing protective bacteria like Lactobacillus and Bifidobacterium.
The amount of data evaluating the effects of space-related stress or microgravity are very limited. Candela and colleagues showed that the gut microbial community dynamics, immune function, metabolic health, and psychological condition of six astronauts during the ground-based space simulation MARS500 project consistently indicate a stressed physiology and gut inflammation even when astronauts did not report any intestinal complaints.Citation60 Chen and colleagues studied seven humans under simulated microgravity, quantifying fecal Bifidobacterium and Lactobacillus, and gut microbial products in urine.Citation61 In Rhesus macaques, genera such as Klebsiella, Kluyvera, and Bifidobacterium were associated with immune dysfunction, while Oligella, Sporosarcina, Citrobacter, Weissella, and Myroides were linked to muscle atrophy under HDT.Citation62 Moreover, in mice, microgravity simulation led to a reduction in protective genera, namely Akkermansia and Bifidobacterium.Citation63 Yet, none of these studies assess the effect of countermeasures. On the other hand, the effect of countermeasures such as exercise training to offset spaceflight-induced multisystem deconditioning has recently been evaluated on a large cohort of astronauts on long-duration spaceflight missions.Citation64 While revealing substantial inter-individual heterogeneity in multisystem response, standard measures did not include endpoints related to the gut microbiota and microenvironment.
Thus, by revealing important information about an astronaut’s overall health and well-being, monitoring the gut microbiota and microenvironment could precede the suspicion, conscience, and reporting of disease and represent an invaluable data source to address health deterioration.
We acknowledge limitations in our study that warrant consideration. Firstly, the relatively low sample size may have restricted the statistical power to detect relevant effects accurately. As anticipated for other experiments within the AGBRESA study, data pooling across several bed rest studies, under standardized conditions and procedures, could help mitigate this limitation to a certain extent. Regarding the experimental setup, while we have found no evidence of systemic inflammation, we cannot entirely rule out the possibility of invasive muscle biopsy-related inflammation influencing the observed correlations between intestinal fatty acid profiles and inflammation onset. Furthermore, the medications administered to alleviate various discomforts may act as uncontrolled confounders and might contribute to the observed changes in the results. Yet, the compounds and ailment origin are highly variable and no clear trend can be correlated with any specific phenotype or gut profile.
Conclusions
The study of the gut microbiota and metabolites within AGBRESA provided valuable insights into the human physiology spectrum during simulated weightlessness. However, it is important to note that individuality is predominant in many aspects of our analysis. The limitations associated with a small sample size and significant interpersonal variation observed in the study suggest that further exploration is needed, including a larger sample size and a longer recovery duration. Additionally, the direct impact of diet was a central point during AGBRESA, reducing the uncertainty and ambiguity that nutritional studies typically comprise. However, the extrapolation of more benefits induced by countermeasures was limited by the short window and frequency of sampling. Fecal sampling has the advantage of being noninvasive and easily obtainable, enabling the comprehensive analysis of the gut microenvironment and signaling astronauts’ physiological and psychological health. Still, the definition of gut microbiome and microenvironment profiles as objective biomedical indicators is far from established. Future studies should consider following the strategies herein applied to stool samples from astronauts on mission. This will allow the terrestrial simulation to complement the physiological data of individuals subjected to harsher settings.
Methods
AGBRESA study design and sample preparation
The AGBRESA study – second campaign – took place at DLR Cologne in the :envihab facilities from 02.09.2019 to 19.02.2020. Each subject resided at the :envihab for 89 days, 60 of which were spent on head-down tilt – HDT – bed rest treatment. Prior to and following HDT, the participants were evaluated during a 15-day baseline data collection (BDC) stage and a 14-day recovery (R) phase. During HDT, the subjects were split into three subgroups, four were subjected to HDT treatment, four to HDT in addition to an intermittent protocol of countermeasure (HDT & int CM) of six times 5-minute centrifugation with 3-minute breaks, and the last four subjects were treated with a continuous (HDT & cont CM) 30-minute centrifugation protocol. Muscle tissue biopsy was performed twice on the lower extremities on each participant by trained medical staff for a different experiment within the bed rest campaign. Stool samples were recovered in DNA/RNA Shield transport and storage medium (#R1100, Zymo Research) according to manufacturer’s instructions from each of the 12 participants at five different time points: once during BDC-10, during HDT (at days 10, 30, and 50) and once during the recovery period R9 (Fig. S1), and the samples were then shipped on dry ice and stored at −80°C until further processing.
Human ethics statement
Subjects provided written, informed consent prior to study enrollment. The integrated AGBRESA protocol reflecting all experiments was approved by the ethics commission of the local medical association (number 2,018,143, Ärztekammer Nordrhein) in Düsseldorf, Germany, as well as the Federal Office for Radiation Protection (number Z 5–22464/2018–074-R-G, Bundesamt für Strahlenschutz). The NASA-initiated studies were separately approved by the NASA Institutional Review Board. The study was prospectively registered at the German Clinical Trials Register under number DRKS00015677.
Metaproteomic taxonomical and peptide-derived function analysis
Samples stored at −80°C in Zymo’s DNA/RNA Shield Fecal Collection Tube were thawed and homogenized before an aliquot of 1 mL of each sample was transferred to fresh tubes. Following precipitation of the proteins with chloroform-methanol,Citation65 the resulting pellet was resuspended in 180 μL LDS 1X (Lithium dodecyl sulfate) sample buffer (NP0007, InvitrogenTM, Thermo Fisher) supplemented with 5% beta-mercaptoethanol (vol/vol). Samples were incubated at 99°C for 5 min before 25 μL was loaded onto a NuPAGE 4–12% Bis-Tris gel, and proteins were subjected to short (5-min) SDS-PAGE migration. Proteins were stained for 5 min with Coomassie SimplyBlue SafeStain (LC6060, Thermo Fisher) prior to in-gel trypsin proteolysis with Trypsin Gold (V5280, Promega) using 0.011% ProteaseMAX surfactant (V2071, Promega), as described in.Citation66
Peptides were quantified and peptide volume to be injected for each fecal sample was normalized accordingly. Peptides were injected on a Q-Exactive HF mass spectrometer (#0726041, Thermo Fisher) connected with an UltiMate 3000 LC system (#5041.0010, Dionex-LC) and operated in data-dependent mode, as previously described.Citation67 The peptides were desalted on an Acclaim PepMap100 C18 precolumn (5 μm, 100 Å, 300 μm id × 5 mm), and then resolved on a nanoscale Acclaim PepMap 100 C18 column (3 μm, 100 Å, 75 μm id × 50 cm) with a 120-min gradient at a flow rate of 0.2 μL/min. The gradient was developed from 4 to 25% of [80% CH3CN, 0.1% formic acid] over 100 min, and then from 25 to 40% over 20 min. Peptides were analyzed during scan cycles initiated by a full scan of peptide ions in the ultra-high-field Orbitrap analyzer, followed by high-energy collisional dissociation and MS/MS scans on the 20 most abundant precursor ions (Top20 method). Full-scan mass spectra were acquired from m/z 350 to 1500 at a resolution of 60,000 with internal calibration activated on the m/z 445.12002 signal. During ion selection for MS/MS fragmentation and measurement, a 10-s dynamic-exclusion window was applied with an intensity threshold of 1.7 × 104. Only ions with positive charges 2+ and 3+ were considered. Precursor ions were isolated using a 0.7-m/z isolation window and activated with 27% normalized collision energy.
The Mascot Daemon 2.6.1 search engine (Matrix Science) was used to match MS/MS spectra to peptides in a multi-round search process. The search parameters were as follows: full trypsin specificity, maximum of two missed cleavages, mass tolerances of 5 ppm on the parent ion and 0.02 Da on the MS/MS, carbamidomethylated cysteine (+57.0215) as fixed modification, and oxidized methionine (+15.9949) and deamidation of asparagine and glutamine (+0.9848) as variable modifications. The NCBInr database (National Center for Biotechnology Information, NIH, Bethesda) was downloaded as https://ftp.ncbi.nlm.nih.gov/blast/db/FASTA/nr.gz (55 GB, downloaded on 2nd January 2020). Protein accession numbers were mapped to taxids as previously describedCitation68. Python SQLite databases were created to perform fast protein-to-taxid matching. The file prot.accession2taxid.gz, downloaded from https://ftp.ncbi.nlm.nih.gov/pub/taxonomy/accession2taxid was used to supplement databases. Files containing the NCBI taxonomy, names.dmp and nodes.dmp, were downloaded as https://ftp.ncbi.nlm.nih.gov/pub/taxonomy/taxdmp.zip to directly assign Peptide-spectrum matches (PSMs) to the “canonical” taxonomical levels (species, genus, family, order, class, phylum, and superkingdom) of the NCBI taxonomic tree. Mascot search rounds were performed as previously reportedCitation45 and taxonomies were identified as described.Citation69 The raw PSMs assigned to each taxon (hereafter TSMs, for Taxon-Spectrum Matches), the numbers of matching peptide sequences, specific peptide sequences, and corresponding specific PSMs were determined for the species, genus, family, order, class, phylum, and superkingdom “canonical” taxonomical levels. PSMs validated with a Mascot p-value of 0.05 during the second-round search were filtered using an FDR < 1% and subsequently used to infer peptide and protein identifications. Proteins were grouped if they shared at least one peptide. Label-free quantification was performed based on PSM counts for each protein, applying the principle of parsimony. For each phylum, spectral counts for all the proteins in a group were summed to assign abundance values to each protein group. Proteins were KEGG-annotated using the GhostKoala web service (https://www.kegg.jp/ghostkoala/) to match proteins to KEGG pathway maps. The spectrum count values for peptides mapped to a KEGG through protein mapping were summed to attribute an abundance value to each functional term. Peptide-to-taxon mapping was also performed to allow taxon-resolved functional quantification.
Count values from both taxonomic (number of TSMs) and functional data (spectrum counts) were scaled relative to their total in the sample. The R package metacoderCitation70 was used to visualize and manipulate community taxonomic diversity data. Pairwise alpha-diversity indices were calculated using the vegdist function from the vegan package in R. The plsda function in the mixOmics R package was used to introduce partial least squares discriminant analysis (PLS-DA) as a supervised model to reveal microbiota changes between groups, whilst its sparse version ((s)PLS-DA) was used to select variables that best discriminate between groups.Citation71 The coseq packageCitation72 was used to perform a co-expression analysis of the microbial and host-related functional profiles.
The relative abundance of proteins corresponding to known fecal biomarkers used for severe bowel disease was obtained by normalizing against the total number of spectra detected. These values were then plotted by each individual and timepoint.
Data availability
All data generated or analyzed during this study are included in this published article and its Supplementary Information files. The mass spectrometry and proteomics datasets acquired on fecal samples are available through the ProteomeXchange Consortium via the PRIDE partner repository (https://www.ebi.ac.uk/pride), under dataset identifiers P×D032387and 10.6019/PXD032387.
Short chain fatty acid (SCFA) quantitation
For SCFAs the method of Han et al. was modified.Citation73 Each fecal sample (100 mg) was mixed with 500 µL ACN:Water (1:1, v/v) and subsequently homogenized using a TissueLyser II (30 Hz, 10 min; Retsch Qiagen). After centrifugation (2 min, 14000 rpm) 38 µL were used for further derivatization. The feces supernatant was combined with 2 µL D7-butyric acid (2 mM) used as internal standard, 20 µL 3-nitrophenylhydrazine in 50% ACN (200 mM) and 20 µL N-(3-dimethylaminopropyl)-N‘-ethylcarbodiimide hydrochloride in 50% ACN with 6% pyridine (120 mM). Incubation of the mixture was done for 30 min at 40°C in a thermomixer (Eppendorf). SCFA standards of 2 µL were used as an external calibration curve for quantification, were diluted with 38 µL 100% ACN. Reagents references are the following: formic acid (#56302, Sigma Aldrich), acetonitrile (#1.00029.2500, Merck), 3-nitrophenylhydrazin hydrochloride (3 – NPH, #N21804, Sigma Aldrich), N-(3-dimethylaminopropyl)-N‘-ethylcarbodiimide hydrochloride (EDC) (#03450, Fluka), pyridin (#K44661928419, Merck), acetate (#1000631011, Merck), propionate (#81910, Sigma Aldrich), isobutyrate (#I1754, Sigma Aldrich), butyrate (#B103500, Sigma Aldrich), 2-methylbutyrate (#193070, Sigma Aldrich), isovalerate (#129542, Sigma Aldrich), valerate (# 240370, Sigma Aldrich), isocaproate (#277827, Sigma Aldrich), caproate (#21530, Sigma Aldrich), D7 butyric acid (#DLM-1508-5, Cambridge Isotopes Ltd).
The resulting derivative was diluted 1:50 in 10% ACN. For measurement, 10 µL of each sample were injected onto the UltiMate 3000 HPLC system (ThermoFisher) coupled online to a QTRAP® 5000 mass spectrometer (Sciex). SFCA were separated on an Acquity UPLC BEH C18 column (1.7 µm, 2.1 × 100 mm) with H2O +0.01% formic acid and ACN + 0.01% formic acid as mobile phases A and B, respectively. Constant flow rate was set to 0.35 mL and column oven was set to 40°C. The chromatographic gradient was as follows: 0–2 min at 15% B, 2–15 min 15–50% B, 15–16 min 100% B, 16–17 min 100–15% B, 17–20 min 15% B. Spectral data were obtained in negative ionization mode. For identification and quantitation, a scheduled multiple reaction monitoring (MRM) methodCitation73 was used with specific transitions for every SCFA. Peak areas of all samples and standards for linear regression were determined in Analyst® Software (v. 1.6.2, AB Sciex) and areas for single SCFAs were exported. Normalization and statistics were performed with in-house written R scripts.
Author contribution
Conception and design of experiments were planned by EM, PFM, URK, JA and RM; sample processing and characterization was carried by LG, SBH, GM, OL, NJ, BE; data analysis and compilation was conducted by ARN, LG, SBH, AH; writing of the manuscript was performed by ARN, LG; further contributions such as discussions, comments, proofreading to the manuscript were provided by SBH, AH, FSA, YL, BE, MVB, EM, PFM, CEH, JJ, URK, JA, RM. All authors improved the manuscript by their specific input and also approved the final version of the manuscript.
Supplemental Material
Download Zip (23.8 MB)Acknowledgments
We would like to thank the AGBRESA team of technicians, engineers, nurses and medical staff that participated in the daily routine of the study as well as the participants for their cooperation, the DLR centrifuge team, and DLR study team for their efforts and extend our appreciation to NASA and ESA for their collaboration. Also, we thank Nikea J. Ulrich for proofreading our manuscript. Finally, a special display of gratitude to Katharina Runzheimer, Erika Muratov, and Andrea Schröder from the Institute of Aerospace Medicine at DLR for all the precious discussions about their daily experiences of the AGBRESA enterprise.
Disclosure statement
No potential conflict of interest was reported by the author(s).
Supplementary material
Supplemental data for this article can be accessed online at https://doi.org/10.1080/19490976.2023.2259033
Additional information
Funding
References
- Tesei D, Jewczynko A, Lynch A, Urbaniak C. Understanding the complexities and changes of the astronaut microbiome for successful long-duration space missions. Life. 2022 Mar;12(4):495. doi:10.3390/life12040495.
- Garrett-Bakelman FE, Darshi M, Green SJ, Gur RC, Lin L, Macias BR, McKenna MJ, Meydan C, Mishra T, Nasrini J, et al. The NASA twins study: a multidimensional analysis of a year-long human spaceflight. Sci. 2019;364(6436)eaau8650. doi:10.1126/science.aaw7086.
- Turroni S, Magnani M, KC P, Lesnik P, Vidal H, Heer M. Gut microbiome and space travelers’ health: state of the art and possible pro/prebiotic strategies for long-term space missions. Front Physiol. 2020 Sep 08;11:1135. Frontiers Media S.A. doi: 10.3389/fphys.2020.553929.
- Vuong HE, Yano JM, Fung TC, Hsiao EY. The microbiome and host behavior. Annu Rev Neurosci. 2017;40(1):21–18. doi:10.1146/annurev-neuro-072116-031347.
- Sender R, Fuchs S, Milo R. Revised estimates for the number of human and bacteria cells in the body. PLoS Biol. 2016 Aug;14(8):e1002533. doi:10.1371/journal.pbio.1002533.
- Vitetta L, Bambling M, Alford H. The gastrointestinal tract microbiome, probiotics, and mood. Inflammopharmacology. 2014;22(6):333–339. doi:10.1007/s10787-014-0216-x.
- Flint HJ, Scott KP, Duncan SH, Louis P, Forano E. Microbial degradation of complex carbohydrates in the gut. Gut Microbes. 2012 Jul;3(4):289–306. doi:10.4161/gmic.19897.
- Macfarlane GT, Gibson GR, Beatty E, Cummings JH. Estimation of short-chain fatty acid production from protein by human intestinal bacteria based on branched-chain fatty acid measurements. FEMS Microbiol Ecol. 1992 Dec;10(2):81–88. doi:10.1111/j.1574-6941.1992.tb00002.x.
- Aguirre M, Eck A, Koenen ME, Savelkoul PHM, Budding AE, Venema K. Diet drives quick changes in the metabolic activity and composition of human gut microbiota in a validated in vitro gut model. Res Microbiol. 2016 Feb;167(2):114–125. doi:10.1016/j.resmic.2015.09.006.
- Koh A, De Vadder F, Kovatcheva-Datchary P, Bäckhed F. From dietary fiber to host physiology: short-chain fatty acids as key bacterial metabolites. Cell. 2016 Jun 02;165(6):1332–1345. Cell Press. doi:10.1016/j.cell.2016.05.041.
- Jiang P, Green SJ, Chlipala GE, Turek FW, Vitaterna MH. Reproducible changes in the gut microbiome suggest a shift in microbial and host metabolism during spaceflight. Microbiome. 2019 Aug;7(1):1–18. doi:10.1186/s40168-019-0724-4.
- Liu Z, Luo G, Du R, Sun W, Li J, Lan H, Chen P, Yuan X, Cao D, Li Y, et al. Effects of spaceflight on the composition and function of the human gut microbiota. Gut Microbes. 2020 Jul;11(4):807–819. doi:10.1080/19490976.2019.1710091.
- Garrett-Bakelman FE, Darshi M, Green SJ, Gur RC, Lin L, Macias BR, McKenna MJ, Meydan C, Mishra T, Nasrini J, et al. The NASA twins study: a multidimensional analysis of a year-long human spaceflight. Sci. 2019 Apr;364(6436). doi:10.1126/science.aaw7086.
- Frett T, Green DA, Mulder E, Noppe A, Arz M, Pustowalow W, Petrat G, Tegtbur U, Jordan J. Tolerability of daily intermittent or continuous short-arm centrifugation during 60-day 6o head down bed rest (AGBRESA study). PloS One. 2020 Sep;15(9):e0239228. doi:10.1371/journal.pone.0239228.
- Kramer A, Venegas-Carro M, Zange J, Sies W, Maffiuletti NA, Gruber M, Degens H, Moreno-Villanueva M, Mulder E. Daily 30-min exposure to artificial gravity during 60 days of bed rest does not maintain aerobic exercise capacity but mitigates some deteriorations of muscle function: results from the AGBRESA RCT. Eur J Appl Physiol. 2021 Jul;121(7):2015–2026. doi:10.1007/s00421-021-04673-w.
- De Martino E, Salomoni SE, Hodges PW, Hides J, Lindsay K, Debuse D, Winnard A, Elliott J, Hoggarth M, Beard D, et al. Intermittent short-arm centrifugation is a partially effective countermeasure against upright balance deterioration following 60-day head-down tilt bed rest. J Appl Physiol. 2021 Aug;131(2):689–701. doi:10.1152/japplphysiol.00180.2021.
- Tays GD, McGregor HR, Lee JK, Beltran N, Kofman IS, De Dios YE, Mulder E, Bloomberg JJ, Mulavara AP, Wood SJ, et al. The effects of 30 minutes of artificial gravity on cognitive and sensorimotor performance in a spaceflight analog environment. Front Neural Circuits. 2022 Mar;16:9. doi:10.3389/fncir.2022.784280.
- Möstl S, Orter S, Hoffmann F, Bachler M, Hametner B, Wassertheurer S, Rabineau J, Mulder E, Johannes B, Jordan J, et al. Limited effect of 60-days strict head down tilt bed rest on vascular aging. Front Physiol. 2021 May;12:765. doi:10.3389/fphys.2021.685473.
- De Martino E, Hides J, Elliott JM, Hoggarth MA, Zange J, Lindsay K, Debuse D, Winnard A, Beard D, Cook JA, et al. Intramuscular lipid concentration increased in localized regions of the lumbar muscles following 60 day bedrest. The Spine Journal. 2022 Apr;22(4):616–628. doi:10.1016/j.spinee.2021.11.007.
- Tran V, De Martino E, Hides J, Cable G, Elliott JM, Hoggarth M, Zange J, Lindsay K, Debuse D, Winnard A, et al. Gluteal muscle atrophy and increased intramuscular lipid concentration are not mitigated by daily artificial gravity Following 60-Day head-down tilt bed rest. Front Physiol. 2021 Nov;12:1945. doi:10.3389/fphys.2021.745811.
- Kramer A, Venegas-Carro M, Mulder E, Lee JK, Moreno-Villanueva M, Bürkle A, Gruber M. Cardiorespiratory and neuromuscular demand of daily centrifugation: results from the 60-day AGBRESA bed rest study. Front Physiol. 2020 Sep;11:1155. doi:10.3389/fphys.2020.562377.
- Attias J, Grassi A, Bosutti A, Ganse B, Degens H, Drey M. Head-down tilt bed rest with or without artificial gravity is not associated with motor unit remodeling. Eur J Appl Physiol. 2020 Nov;120(11):2407–2415. doi:10.1007/s00421-020-04458-7.
- Ganse B, Bosutti A, Drey M, Degens H. Sixty days of head-down tilt bed rest with or without artificial gravity do not affect the neuromuscular secretome. Exp Cell Res. 2021 Feb;399(2):112463. doi:10.1016/j.yexcr.2020.112463.
- Clément G, Rittweger J, Nitsche A, Doering W, Frings-Meuthen P, Hand O, Frett T, Noppe A, Paulke F, Lecheler L, et al. Assessing the effects of artificial gravity in an analog of long-duration spaceflight: the protocol and implementation of the AGBRESA bed rest study. Front Physiol, 2022 Sep;13:976926. doi:10.3389/fphys.2022.976926.
- Laurie SS, Greenwald SH, Marshall‐Goebel K, Pardon LP, Gupta A, Lee SMC, Stern C, Sangi‐Haghpeykar H, Macias BR, Bershad EM, et al. Optic disc edema and chorioretinal folds develop during strict 6° head-down tilt bed rest with or without artificial gravity. Physiol Rep. 2021 Aug;9(15):e14977. doi:10.14814/phy2.14977.
- Thot GK, Berwanger C, Mulder E, Lee JK, Lichterfeld Y, Ganse B, Giakoumaki I, Degens H, Duran I, Schönau E, et al. Effects of long-term immobilisation on endomysium of the soleus muscle in humans. Exp Physiol. 2021;106(10):2038–2045. doi:10.1113/EP089734.
- Oliphant K, Allen-Vercoe E. Macronutrient metabolism by the human gut microbiome: major fermentation by-products and their impact on host health. Microbiome. 2019;7(1):1–15. doi:10.1186/s40168-019-0704-8.
- Chen L, Reynolds C, David R, Peace Brewer A. Development of an Index score for intestinal inflammation-associated dysbiosis using real-world stool test results. Dig Dis Sci. 2020 Apr;65(4):1111–1124. doi:10.1007/s10620-019-05828-8.
- Ashton JJ, Beattie RM, Ennis S, Cleary DW. Analysis and interpretation of the human microbiome. Inflamm Bowel Dis. 2016;22(7):1713–1722. doi:10.1097/MIB.0000000000000809.
- Birg A, Ritz NL, Lin HC. The unknown effect of antibiotic-induced dysbiosis on the gut microbiota. Elsevier Inc; 2019. doi:10.1016/B978-0-12-815249-2.00020-8.
- Scepanovic P, Hodel F, Mondot S, Partula V, Byrd A, Hammer C, Alanio C, Bergstedt J, Patin E, Touvier M, et al. A comprehensive assessment of demographic, environmental, and host genetic associations with gut microbiome diversity in healthy individuals. Microbiome. 2019 Sep;7(1):130. doi:10.1186/S40168-019-0747-X/FIGURES/5.
- Van Den Bossche T, Arntzen MØ, Becher D, Benndorf D, Eijsink VGH, Henry C, Jagtap PD, Jehmlich N, Juste C, Kunath BJ, et al. The metaproteomics initiative: a coordinated approach for propelling the functional characterization of microbiomes. Microbiome. 2021 Dec 01;9(1):1–4. BioMed Central Ltd. doi:10.1186/s40168-021-01176-w.
- Daly AJ, Baetens JM, De Baets B. Ecological diversity: measuring the unmeasurable. Mathematics. 2018;6(7):119. doi:10.3390/math6070119.
- Bressa C, Bailén-Andrino M, Pérez-Santiago J, González-Soltero R, Pérez M, Montalvo-Lominchar MG, Maté-Muñoz JL, Domínguez R, Moreno D, Larrosa M, et al. Differences in gut microbiota profile between women with active lifestyle and sedentary women. PloS One. 2017 Feb;12(2):e0171352. doi:10.1371/journal.pone.0171352.
- Vacca M, Celano G, Calabrese FM, Portincasa P, Gobbetti M, De Angelis M. The controversial role of human gut lachnospiraceae. Microorganisms. 2020 Apr;8(4):573. doi:10.3390/microorganisms8040573.
- Obata T, Goto Y, Kunisawa J, Sato S, Sakamoto M, Setoyama H, Matsuki T, Nonaka K, Shibata N, Gohda M, et al. Indigenous opportunistic bacteria inhabit mammalian gut-associated lymphoid tissues and share a mucosal antibody-mediated symbiosis. Proc Natl Acad Sci USA. 2010 Apr;107(16):7419–7424. doi:10.1073/PNAS.1001061107.
- Rath S, Rud T, Karch A, Pieper DH, Vital M. Pathogenic functions of host microbiota. Microbiome. 2018 Sep;6(1):1–13. doi:10.1186/s40168-018-0542-0.
- Stevens EJ, Bates KA, King KC, Bliska JB. Host microbiota can facilitate pathogen infection. PLoS Pathog. 2021 May;17(5):e1009514. doi:10.1371/JOURNAL.PPAT.1009514.
- Lloyd-Price J, Abu-Ali G, Huttenhower C. The healthy human microbiome. Genome Med. 2016 Apr 27;8(1):1–11. BioMed Central Ltd. doi:10.1186/s13073-016-0307-y.
- Yatsunenko T, Rey FE, Manary MJ, Trehan I, Dominguez-Bello MG, Contreras M, Magris M, Hidalgo G, Baldassano RN, Anokhin AP, et al. Human gut microbiome viewed across age and geography. Nature. 2012 Jun 14;486(7402):222–227. Nature Publishing Group. doi:10.1038/nature11053.
- Rizzatti G, Lopetuso LR, Gibiino G, Binda C, Gasbarrini A. Proteobacteria: a common factor in human diseases. BioMed Res Int. 2017;2017:1–7. doi:10.1155/2017/9351507.
- Bradley PH, Pollard KS. Proteobacteria explain significant functional variability in the human gut microbiome. Microbiome. 2017;5(1):1–23. doi:10.1186/s40168-017-0244-z.
- Shin NR, Whon TW, Bae JW. Proteobacteria: microbial signature of dysbiosis in gut microbiota. Trends Biotechnol. 2015;33(9):496–503. doi:10.1016/j.tibtech.2015.06.011.
- Ndeh D, Gilbert HJ. Biochemistry of complex glycan depolymerisation by the human gut microbiota. FEMS Microbiol Rev. 2018;42(2):146–164. doi:10.1093/femsre/fuy002.
- Grenga L, Pible O, Miotello G, Culotta K, Ruat S, Roncato M-A, Gas F, Bellanger L, Claret P-G, Dunyach‐Remy C, et al. Taxonomical and functional changes in COVID-19 faecal microbiome could be related to SARS-CoV-2 faecal load. Environ Microbiol. 2022;24(9):4299–4316. doi:10.1111/1462-2920.16028.
- Douglas GL, DeKerlegand D, Dlouhy H, Dumont-Leblond N, Fields E, Heer M, Krieger S, Mehta S, Rooney BV, Torralba MG, et al. Impact of diet on human nutrition, immune response, gut microbiome, and cognition in an isolated and confined mission environment. Science Reports. 2022 Dec;12(1):1–22. doi:10.1038/s41598-022-21927-5.
- Siddiqui I, Majid H, Abid S. Update on clinical and research application of fecal biomarkers for gastrointestinal diseases. World J Gastrointest Pharmacol Ther. 2017;8(1):39. doi:10.4292/WJGPT.V8.I1.39.
- Sherwood RA. Faecal markers of gastrointestinal inflammation. J Clin Pathol. 2012 Nov;65(11):981–985. doi:10.1136/jclinpath-2012-200901.
- Fu Y, Wang L, Xie C, Zou K, Tu L, Yan W, Hou X. Comparison of non-invasive biomarkers faecal BAFF, calprotectin and FOBT in discriminating IBS from IBD and evaluation of intestinal inflammation. Sci Rep. 2017 Dec;7(1):2669. doi:10.1038/s41598-017-02835-5
- Deleu S, Machiels K, Raes J, Verbeke K, Vermeire S. Short chain fatty acids and its producing organisms: an overlooked therapy for IBD? EBioMedicine. 2021 Apr;66:103293. doi:10.1016/J.EBIOM.2021.103293.
- Venegas DP, De la Fuente MK, Landskron G, González MJ, Quera R, Dijkstra G, Harmsen HJM, Faber KN, Hermoso MA. Short chain fatty acids (SCFAs)mediated gut epithelial and immune regulation and its relevance for inflammatory bowel diseases. Front Immunol. 2019;10(MAR):277. Frontiers Media S.A. doi:10.3389/fimmu.2019.00277.
- Silva YP, Bernardi A, Frozza RL. The role of short-chain fatty acids from gut microbiota in gut-Brain communication. Front Endocrinol. 2020 Jan 31;11:25. Frontiers Media S.A. doi: 10.3389/fendo.2020.00025.
- Den Besten G, Van Eunen K, Groen AK, Venema K, Reijngoud DJ, Bakker BM. The role of short-chain fatty acids in the interplay between diet, gut microbiota, and host energy metabolism. J Lipid Res. 2013 Sep;54(9):2325–2340. doi:10.1194/jlr.R036012.
- Feng W, Ao H, Peng C. Gut microbiota, short-chain fatty acids, and herbal medicines. Front Pharmacol. 2018 Nov 23;9(NOV):1354. Frontiers Media S.A. doi:10.3389/fphar.2018.01354.
- Iovino P, Chiarioni G, Bilancio G, Cirillo M, Mekjavic IB, Pisot R, Ciacci C. New onset of constipation during long-term physical inactivity: a proof-of-concept study on the Immobility-induced bowel changes. PloS One. 2013 Aug;8(8):e72608. doi:10.1371/journal.pone.0072608.
- Di Saverio S, Tugnoli G, Orlandi PE, Casali M, Catena F, Biscardi A, Pillay O, Baldoni F. A 73-year-old man with long-term immobility presenting with abdominal pain. PLoS Med. 2009 Jul;6(7):e1000092. doi:10.1371/journal.pmed.1000092.
- Gerritsen J, Smidt H, Rijkers GT, De Vos WM. Intestinal microbiota in human health and disease: the impact of probiotics. Genes Nutr. 2011 Aug 27;6(3):209–240. BioMed Central. doi:10.1007/s12263-011-0229-7.
- Kirgizov IV, Sukhorukov AM, Dudarev VA, Istomin AA. Hemostasis in children with dysbacteriosis in chronic constipation. Clin Appl Thromb Hemost. 2001 Oct;7(4):335–338. doi:10.1177/107602960100700416.
- Zhao Y, Yu YB. Intestinal microbiota and chronic constipation. SpringerPlus. 2016 Dec 01;5(1):1130. SpringerOpen. doi:10.1186/s40064-016-2821-1.
- Candela M, Biagi E, Soverini M, Consolandi C, Quercia S, Severgnini M, Peano C, Turroni S, Rampelli S, Pozzilli P, et al. Modulation of gut microbiota dysbioses in type 2 diabetic patients by macrobiotic ma-pi 2 diet. Br J Nutr. 2016 Jul;116(1):80–93. doi:10.1017/S0007114516001045.
- Chen P, Yu Y, Tan C, Liu H, Wu F, Li H, Huang J, Dong H, Wan Y, Chen X, et al. Human metabolic responses to microgravity simulated in a 45-day 6° head-down tilt bed rest (HDBR) experiment. Anal Methods. 2016;8(22):4334–4344. doi:10.1039/c6ay00644b.
- Zhang P, Shao L, Zhang J, Wang L, Pang X, Tao W, Fan G, Peng L, Kan G, Li W, et al. Microbiota–muscle/immune interactions in rhesus macaque under simulated microgravity revealed by integrated multi‐omics analysis. JCSM Rapid Commun. 2022;5(2):212–225. doi:10.1002/rco2.60.
- Wang Y, Zhao W, Shi J, Wang J, Hao J, Pang X, Huang X, Chen X, Li Y, Jin R, et al. Intestinal microbiota contributes to altered glucose metabolism in simulated microgravity mouse model. FASEB J. 2019;33(9):10140–10151. doi:10.1096/fj.201900238RR.
- Scott JM, Feiveson AH, English KL, Spector ER, Sibonga JD, Dillon EL, Ploutz-Snyder L, Everett ME. Effects of exercise countermeasures on multisystem function in long duration spaceflight astronauts. NPJ Microgravity. 2023;9(1):11. doi:10.1038/s41526-023-00256-5.
- Wessel D, Flügge UI. A method for the quantitative recovery of protein in dilute solution in the presence of detergents and lipids. Anal Biochem. 1984;138(1):141–143. doi:10.1016/0003-2697(84)90782-6.
- Hartmann EM, Allain F, Gaillard JC, Pible O, Armengaud J. Taking the shortcut for high-throughput shotgun proteomic analysis of bacteria. Methods Mol Biol. 2014;1197:275–285. doi:10.1007/978-1-4939-1261-2_16.
- Klein G, Mathé C, Biola-Clier M, Devineau S, Drouineau E, Hatem E, Marichal L, Alonso B, Gaillard J-C, Lagniel G, et al. RNA-binding proteins are a major target of silica nanoparticles in cell extracts. Nanotoxicology. 2016 Nov;10(10):1555–1564. doi:10.1080/17435390.2016.1244299.
- Pible O, Allain F, Jouffret V, Culotta K, Miotello G, Armengaud J. Estimating relative biomasses of organisms in microbiota using ‘phylopeptidomics,’. Microbiome. 2020 Mar;8(1):30. doi:10.1186/s40168-020-00797-x.
- Jouffret V, Miotello G, Culotta K, Ayrault S, Pible O, Armengaud J. Increasing the power of interpretation for soil metaproteomics data. Microbiome. 2021 Dec;9(1):195. doi:10.1186/s40168-021-01139-1.
- Foster ZSL, Sharpton TJ, Grünwald NJ, Poisot T. Metacoder: an R package for visualization and manipulation of community taxonomic diversity data. PLoS Comput Biol. 2017 Feb;13(2):e1005404. doi:10.1371/journal.pcbi.1005404.
- Rohart F, Gautier B, Singh A, Lê Cao K-A, Schneidman D. mixOmics: an R package for ‘omics feature selection and multiple data integration. PLoS Comput Biol. 2017 Nov;13(11):e1005752. doi:10.1371/journal.pcbi.1005752.
- Rau A, Maugis-Rabusseau C. Transformation and model choice for RNA-seq co-expression analysis. Brief Bioinform. 2018 May;19(3):425–436. doi:10.1093/bib/bbw128.
- Han J, Lin K, Sequeira C, Borchers CH. An isotope-labeled chemical derivatization method for the quantitation of short-chain fatty acids in human feces by liquid chromatography-tandem mass spectrometry. Anal Chim Acta. 2015 Jan;854:86–94. doi:10.1016/j.aca.2014.11.015.