ABSTRACT
Multidrug-resistant microorganisms have become a major public health concern around the world. The gut microbiome is a gold mine for bioactive compounds that protect the human body from pathogens. We used a multi-omics approach that integrated whole-genome sequencing (WGS) of 74 commensal gut microbiome isolates with metabolome analysis to discover their metabolic interaction with Salmonella and other antibiotic-resistant pathogens. We evaluated differences in the functional potential of these selected isolates based on WGS annotation profiles. Furthermore, the top altered metabolites in co-culture supernatants of selected commensal gut microbiome isolates were identified including a series of dipeptides and examined for their ability to prevent the growth of various antibiotic-resistant bacteria. Our results provide compelling evidence that the gut microbiome produces metabolites, including the compound class of dipeptides that can potentially be applied for anti-infection medication, especially against antibiotic-resistant pathogens. Our established pipeline for the discovery and validation of bioactive metabolites from the gut microbiome as novel candidates for multidrug-resistant infections represents a new avenue for the discovery of antimicrobial lead structures.
Introduction
Multi-drug resistant pathogens have become a critical public health burden worldwide.Citation1,Citation2 It is estimated that 70% of hospital-acquired infections are resistant to one or more antibiotics. Antibiotics account for 80% of the medications given to women during pregnancy and are administered to 35% of pregnant women in Western countries, which brings the potential risk of vertical transmittance of antibiotic resistance from mothers to their offspring.Citation3,Citation4 Pathogens such as Salmonella are a common cause of diarrhea and the leading cause of diarrheal disease-associated deaths.Citation5 The antimicrobial resistant ESKAPE pathogens (Enterococcus faecium, Staphylococcus aureus, Klebsiella pneumoniae, Acinetobacter baumannii, Pseudomonas aeruginosa, and Enterobacter) are reported as the major cause of life-threatening nosocomial infections throughout the world.Citation6,Citation7 In combination with improvements in antibiotic resistance measurement, the discovery of new treatment options to combat pathogens is urgently needed, especially for antibiotic-resistant bacteria. Identifying bioactive compounds from gut and environmental microbiomes that target antibiotic-resistant bacteria is an important new strategy.
Commensal bacteria constitute the normal and healthy microbiota that have co-evolved with humans and have essential roles in supplying nutrients and defending against pathogen invasion.Citation8,Citation9 Previous reports also demonstrated the strong effects of antibiotic treatment on microbial communities with a fundamental reduction in the richness and diversity of the gut microbiota.Citation10–12 Notably, disturbances of the healthy microbiome such as antibiotic treatment can lead to impaired colonization resistance and an increased susceptibility to new infections.Citation2,Citation13
The gut microbiome represents a widely unexplored rich reservoir for bioactive compounds capable of protecting the human body from pathogen invasion. Of particular interest is the discovery of two types of gut microbiome targets for defense against pathogens that include i) direct interactions of commensal bacteria with pathogens and ii) metabolites produced, excreted, or converted by gut bacteria. Fecal microbiota transplants (FMTs) are an effective treatment for Clostridioides difficile infections and are also a promising therapy option for other gastrointestinal diseases including irritable bowel syndrome and even neurological disorders such as Parkinson’s disease.Citation14–16 However, the content of fecal microbiota from donors is highly dynamic and FMT also brings a potential risk of donor-derived disease transmission.Citation17 More consistent microbial consortia with a defined amount of viable bacteria were developed to treat recurrent C. difficile infection and inflammatory bowel diseases (IBD) in clinical trials with promising results.Citation18–20 In our previous study, a large reduction of Salmonella Enteritidis growth was identified after the administration of anaerobically cultivated human intestinal microbiota (ACHIM).Citation10,Citation21,Citation22
While employing bacterial strains to reduce pathogenic growth is a powerful strategy, recent studies have demonstrated that microbiome-derived metabolites such as lipids, amino acids, and short-chain fatty acids (SCFAs) have multiple effects on interfering with pathogenic invasion and host signaling processes.Citation23–25 The impact of propionate, which is produced by commensal bacteria, was identified as altering Salmonella colonization.Citation26 Furthermore, succinate was investigated as a signaling metabolite for Salmonella virulence.Citation27 Antimicrobial peptides (AMPs) are reported to have significant efficacy against a wide spectrum of bacteria while causing little or no antimicrobial resistance and most bioactive antibiotics are derived from microorganisms as secondary metabolites.Citation28,Citation29 Multiple omics-based strategies with high-throughput screening, metagenomics, and metabolomics have also been developed for the biodiscovery of a new class of microbial natural products to counter antibiotic resistance.Citation30,Citation31
In this study, we employed a multi-omics strategy including the whole-genome sequencing (WGS) of commensal gut microbiome isolates, and state-of-the-art metabolome analysis to identify metabolic interaction of gut microbiome isolates with Salmonella. Our comparative genomic approach enabled the selection of a diverse subpopulation for the following functional assays. We identified the chemical structure for most of the top altered metabolites in the supernatant of Salmonella growth cultures compared to co-culture supernatants of the selected commensal gut microbiome isolates. The validated metabolites were further tested for their growth inhibition properties toward a group of antibiotic-resistant pathogens (S. Enteritidis, S. Typhimurium, S. aureus, Escherichia coli, and K. pneumoniae). Our results demonstrate a powerful example that the gut microbiome is a gold mine for bioactive products including previously unknown metabolites. Our reported strategy represents a pipeline for the discovery and in vivo validation of metabolites from the gut microbiome as novel potential interventions for multi-drug-resistant pathogens.
Results
Gut microbiota bacterial isolates exhibited substantial genomic diversity
Previous investigations into commensal gut microbiota cultures demonstrated their strong potential for the inhibition of Salmonella growth.Citation10 To determine which bacterial species play key roles in Salmonella inhibition, we generated a collection of 112 bacterial isolates from the ACHIM cultures and subjected them to WGS analysis. A bioinformatic examination of each isolate’s WGS library revealed that some of the isolates are genomically identical. In total, 74 ACHIM isolates with distinct genomes were found and cultured (). Assessing the pairwise Average Nucleotide Identity (ANI) of these 74 isolates demonstrated a broad diversity among the isolated population with values ranging from 76.8% to 97.8%.Obtaining the ANI percentage values for each unique isolate pair enabled the generation of an all-by-all similarity matrix based on calculated Pearson Correlation Coefficients, which resulted in the formation of 15 primary clades after hierarchical clustering (). WGS-based taxonomic classification of each isolate using the Lowest Common Ancestor (LCA) algorithm revealed that most terminal nodes comprising each ANI-derived clade represented organisms of the following species: Prevotella phocaeensis, P. oralis, Paraclostridium bifermentans, Clostridium perfringens, C. butyricum, Romboutsia litusburensis, and Eubacterium maltosivorans. (Table S1 and Table S2).
Figure 1. Gut microbiota isolate whole-genome sequencing (WGS) similarity and Salmonella co-culture experimental workflow.
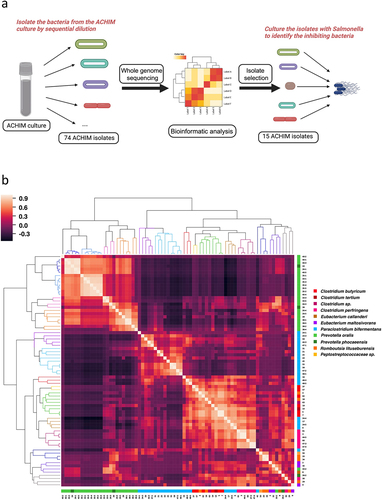
We selected 15 single representative isolates of each distinguished ANI-derived clade in downstream functional assays and delved deeper into their respective WGS data. Re-examination of the respective genomic distances among the selected isolates expressed in terms of the Jaccard Similarity Index (JSI) value between two MinHash genome sketches corroborated results from the ANI analysis.Citation32 However, while this analysis highlighted the genomic diversity of the selected subpopulation with JSI values ranging from zero to one, it also revealed distinct groups with substantial levels of genomic overlap (). Identification of a “best-match” reference organism from the Genome Taxonomy Database (GTDB) for each of the selected isolates showed the resemblance to P. phocaeensis SN19 (n = 4), P. bifermentans WYM (n = 4), C. perfringens CP-36 (n = 2), Clostridium sp. HMSC19A10 (n = 2), Romboutsia litusburensis DSM 797 (n = 1), and Eubacterium limosum SA11 (n = 2) ( and Fig. S1a).Citation33
Figure 2. Gut microbiota isolates selectively reduce Salmonella growth.
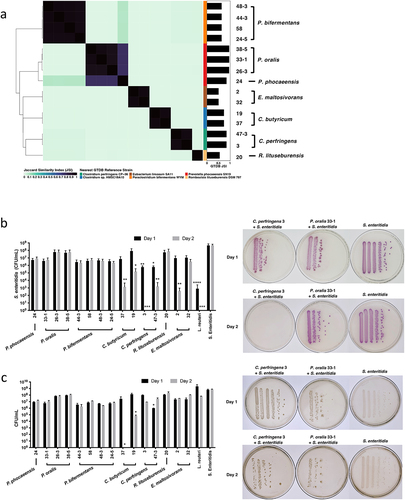
Gut microbiota isolates reduced Salmonella growth and exhibited genomic diversity
We cultured the 15 selected isolates with 106 CFU of Salmonella for 24 and 48 h. The co-cultures of two C. perfringens isolates (ACHIM 3 and 47–3) manifested significant inhibition of Salmonella growth within 24 h (Salmonella selective plates after co-culture: C. perfringens 3: 4.27 × 105 CFU/mL;C. perfringens 47–3: 7.60 × 105 CFU/mL; Control Salmonella: 6.63 × 108 CFU/mL) and demonstrated an even stronger inhibition on the second day (C. perfringens 3: 0 CFU/mL; C. perfringens 47–3: 2.67 × 103 CFU/mL; Control Salmonella: 6.23 × 108 CFU/mL) (). Two other isolates from C. butyricum (ACHIM 19 and 37) and one of the isolates from E. maltosivorans (ACHIM 2) also showed significant inhibition of Salmonella growth after 48 hours of co-culture (C. butyricum 19: 2.52 × 105 CFU/mL; C. butyricum 37: 2.33 × 103 CFU/mL; E. maltosivorans 2: 6.67 × 102 CFU/mL; and Control Salmonella to 6.23 × 108 CFU/mL) (). Salmonella co-cultured with isolates from P. phocaeensis, P. oralis, P. bifermentans, and R. lituseburensis did not yield significant differences compared to the control group ().
To follow the growth of the commensal gut microbes, we also cultured the bacteria on YCFA plates on which both gut microbes and Salmonella grew similarly. The growth of these total bacteria was unchanged except for C. butyricum 19 and C. butyricum 37, which had significantly decreased after 2 days in co-culture (YCFA plates after co-culture: Day 1: C. perfringens 3: 9.90 × 107 CFU/mL; C. perfringens 47–3: 1.01 × 106 CFU/mL; C. butyricum 19: 1.36 × 108 CFU/mL; C. butyricum 37: 2.65 × 107 CFU/mL; E. maltosivorans 2: 2.17 × 10Citation7 CFU/mL; Day 2: C. perfringens 3: 8.70 × 106 CFU/mL; C. perfringens 47–3: 2.69 × 107 CFU/mL; C. butyricum 19: 6.67 × 104 CFU/mL; C. butyricum 37: 0 CFU/mL; E. maltosivorans 2: 3.10 × 107 CFU/mL) ().
Results from these Salmonella co-culture assays yielded two phenotypic isolate groups: a “Kill” group comprised of isolates exhibiting the strongest Salmonella inhibition phenotypes (E. maltosivorans 2, C. perfringens 3, and C. butyricum 37) and a “No-Kill” group containing isolates that equally demonstrated the weakest growth inhibition (R. litusburensis 20, P. oralis 26–3, and P. oralis 38–5). By comparing the colony-forming units (CFUs) on YCFA plates with those on Salmonella-selective plates, we observed that ACHIM, isolated from the “Kill” group, including C. perfringens and E. maltosivorans, grew after co-culture (). We then selected four closely related strains, according to the ANI similarity matrix (), to the “Kill” and “No-Kill” strains under the same cluster. For the strains close to the “Kill” group, C. perfringens 61 and 44–5 showed similarly significant inhibition of S. enteritidis growth at 24 (C. perfringens 61: 3.78 × 105 CFU/mL isolate 61; C. perfringens 44–5: 3.70 × 105 CFU/mL) and 48 h (C. perfringens 61: 2.98 × 105 CFU/mL; C. perfringens 44–5: 3.40 × 105 CFU/mL), while the viability of the isolates themselves was not affected according to the YCFA plates at 24 (C. perfringens 61: 5.60 × 10Citation7 CFU/mL; C. perfringens 44–5: 4.53 × 107 CFU/mL isolate 44–5) and 48 h (C. perfringens 61: 6.04 × 107 CFU/mL; C. perfringens 44–5: 5.13 × 107 CFU/mL). Furthermore, in the close to ‘No-Kill’ group, the inhibition was either weaker or not significant. However, in both cases, the growth of the isolates themselves was significantly impaired at both 24 and 48 h (Figs. S1b-c).
We queried the 45,490 protein-coding genes predicted across the 15 gut microbiota isolate genomes using the Cluster of Orthologous Genes (COG) and Kyoto Encyclopedia of Genes and Genomes (KEGG) databases to survey the genetic underpinnings associated with their predicted metabolic capabilities.Citation34,Citation35 Principal coordinate analysis (PCoA) of functional profiles comprised of fractional abundances of genes with annotations to different COGs demonstrated a high degree of variation in functional potential among the subpopulation of ACHIM gut microbiota (Fig. S2a). This observed functional genomic diversity was underscored by results from similar analysis with KEGG pathway completion profiles generated for each of the 15 isolates (). PCoA analysis with COG fractional abundance and KEGG pathway completion profiles for isolates with established “Kill” and “No-Kill” Salmonella co-culture phenotypes revealed substantial variation in functional genomic potential between the two groups except isolate 20, which presented itself as an interloper with great distance from ‘No-Kill’ isolates 26–3 and 38–5 ( and Fig. S2a).
Figure 3. Evaluation of the functional genomic potentials among gut microbiota isolates.
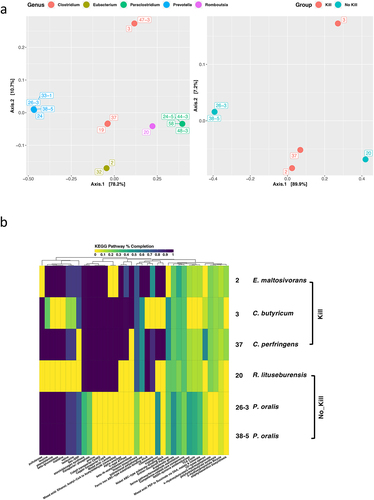
Constructing COG functional category and KEGG Pathway completion annotation summaries for the “Kill” and “No-Kill” isolates and observing profile similarities among them provided additional insight into differences in functional genomic potential across these two phenotypic groups ( and Fig. S2b). Both methods demonstrated two Prevotella isolates (26–3 and 38–5) with near-identical functional genomic capabilities, while the remaining four isolates exhibited numerous differences in COG abundance and KEGG pathway completion. The E. maltosivorans 2 genome exhibited higher frequencies of COG-category annotations in energy production and conversion such as V-type ATPase, coenzyme transport and metabolism including alpha-amylase, and K (transcription) relative to the other isolates ( and Fig. S2b). In addition, E. maltosivorans displayed the highest degree of KEGG pathway completion among all six of the isolates investigated. However, unlike E. maltosivorans 2, the Clostridium members of the “Kill” group exhibited the highest COG frequencies for carbohydrate transport and metabolism ( and Fig. S2b). Although the KEGG and COG profiles for both Clostridium isolates in the ‘Kill’ group showed high degrees of overlap, the R. lituseburensis 20 from the ‘Not-Kill’ group had quite a few similarities in KEGG pathways completion profile elements to those of the ‘Kill’ group isolates ( and Fig. S2b).
Metabolites unique in co-culture systems inhibiting Salmonella growth
To discover the bioactive metabolites that cause the inhibition at the molecular level, we performed a mass spectrometric metabolomic analysis to profile metabolites after coculturing these selected 15 gut microbial strains with Salmonella (). A monoculture of Salmonella and a YCFA culture were used as reference and control, respectively. The analysis was performed for each culture with biological triplicates. Principal component analysis (PCA) and heatmap clustering (top 25 mass spectrometric features based on one-way ANOVA) of the different bacterial strains revealed a clear difference between the species P. phocaeensis (A), P. bifermentans (B), and Rombustia (D) in comparison to the species Clostriduium sp. (C1), C. perfringens (C), and E. maltosivorans (E) (Fig. S2c). To identify metabolites that influence the growth of Salmonella, further targeted comparison was performed with the species that impaired Salmonella growth (E. maltosivorans 2, C. perfringens 3, C. butyricum 37, and L. reuteri) and bacteria that did not alter Salmonella growth (R. litusburensis 20, P. oralis 26–3, and P. oralis 38–5) (). The metabolite structures were validated through collision-induced fragmentation (CID). This structure identification process was performed for 214 features in the positive mode and 152 features in the negative mode mass spectrometry analysis (Table S3). Applied criteria were i) p-value <1.0 × 10−5 via t-test analysis; and ii) fold change > 10 to specifically focus on the highly altered metabolites. All obtained fragmentation spectra were compared with either experimental or computational mass spectrometry fragmentation libraries as well as our in-house library. Fragmentation spectra that did not match any of the previously available databases were uploaded into the computational tool SIRIUS for identification of the fragmentation fingerprint. The putative spectra were then either validated with chemical standards available in our in-house library or purchased. The final step of the metabolite structure validation was the comparison of the retention time of reference compounds with the natural metabolites in the bacterial cultures. After separate determination of the retention times, the chemical standard is utilized to verify the retention time in the biological matrix. Compounds with identical MS/MS fragmentation patterns and retention times were classified as confidence level 1 (Fig. S2d). In total, 100 molecules were identified and validated at the highest confidence levels with either authentic metabolite standards through co-injection experiments or comparison with databases for MS/MS fragmentation spectra.
Figure 4. Metabolites unique in co-culture systems inhibiting Salmonella growth.
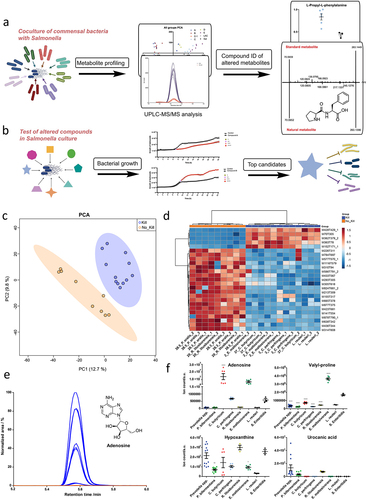
To illustrate our results, the top 25 mass spectrometric features in positive mode analysis are depicted in . We anticipated identifying the most affected metabolic differences between the two groups, as exemplified by adenosine (). The isolates of the same species demonstrate a highly similar metabolite pattern. To obtain metabolites structures at the highest confidence level, compounds of interest were identified with authentic synthetic standards including adenosine, adenine, agmatine, phenylalanine, glutamine, guanine, and the dipeptides Leu-Pro, Val-Pro, Leu-Thr, Pro-Phe, Try-Pro, and Tyr-Pro. Dipeptides are not a common compound class in metabolomic analysis and it is important to identify the correct order/sequence of the dipeptide for the follow-up bioactivity assays. We have determined the order of the two amino acids based on their specific fragmentation as previously reported by us.Citation36 These molecules were present at significantly higher levels in at least one of the Salmonella-depleted co-cultures compared to the co-cultures with unaltered Salmonella ( and Fig. S3, and Table S4 and Table S5). We have also included dipeptides in this list that were found to be significantly increased in the L. reuteri culture as these may also represent bioactive compounds. The levels of hypoxanthine, urocanic acid, and xanthurenic acid were found to be reduced in the co-cultures with reduced Salmonella growth (Fig. S3). Hypoxanthine is an intermediate of the purine degradation pathway, which is utilized by the microbiome as a substrate and is thus known as an important metabolite for metabolic microbial interaction.Citation37,Citation38 Urocanic acid is a metabolite of histidine degradation, which has been suggested as a potential signaling molecule and a cue for bacterial pathogenesis.Citation39 Xanthurenic acid is a quinoline carboxylic acid and part of the tyrosine catabolism pathway. Minor antimicrobial activity has been reported for this compound for E. coli and B. subtilis.Citation40
Adenosine and adenine potently inhibit multiple antibiotic resistance pathogens
After testing dose-dependent inhibition for adenosine and phenylalanine, 1 mmol/L was chosen to validate the findings on significantly changed metabolites (Fig. S4a-b). Salmonella was cultured with the top metabolites that were significantly changed (both increased and decreased) in the co-culture systems when comparing the Salmonella-depleted co-cultures and those without Salmonella inhibition. The growth of bacteria showed that among the tested compounds, adenosine and adenine demonstrated strong inhibition of Salmonella growth after 8 hours of culture (adenosine vs. control: 6.32 × 107 CFU/mL to 2.83 × 108 CFU/mL; adenine vs. control: 6.40 × 107 × 105 CFU/mL to 6.63 × 108 CFU/mL) (). Other metabolites were increased in the Salmonella-depleted co-cultures including agmatine, phenylalanine, glutamine, guanine, and decreased in the Salmonella-depleted co-cultures, including hypoxanthine, urocanic acid, and xanthurenic acid did not significantly affect Salmonella growth (Fig. S4c-i).
Figure 5. Adenosine and adenine are potent inhibitors of multiple-antibiotic-resistant pathogens.
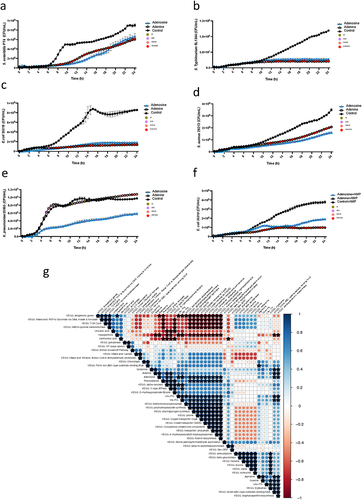
To further examine the potential inhibition of adenosine and adenine, we co-cultured adenosine and adenine with several other common pathogens including antibiotic-sensitive strain K. pneumoniae 25955, and multiple drug-resistant pathogens S. Typhimurium SL1344 (resistance to Streptomycin and Rifampin), S. aureus ATCC 29213 (resistance to Ciprofloxacin, Clindamycin, Gentamicin, and Neomycin), E. coli ST131 (resistance to Ampicillin, Cefotaxime, Ciprofloxacin, nalidixic acid, and Cefpodoxime), and E. coli 35218 (resistance to Ampicillin and Chloramphenicol).Citation41,Citation42,Citation43,Citation44,Citation45 The growth of bacteria revealed that both adenosine and adenine completely inhibit the growth of S. Typhimurium SL1344 and E. coli 35218 in 24 h of co-culture (). Notably, the inhibition is strain-specific, as both adenosine and adenine did influence the growth of E. coli ST131. However, the inhibition effect on E. coli ST131 disappeared after 13 h (Fig. S4j). The growth of S. aureus was reduced but not completely inhibited by either adenosine or adenine, while only adenosine led to partial inhibition of K. pneumoniae growth (). Further investigation of the synergistic potential of adenosine or adenine with a minimum inhibitory concentration (MIC) breakpoint level for ampicillin (32 µg/mL) on E. coli 35218 and E. coli ST131 was further tested ( and S4k). However, the addition of adenosine or adenine together with ampicillin did not increase the susceptivity of the pathogens E. coli 35218 and E. coli ST131. A similar concentration of adenosine and adenine did not significantly inhibit the representative gut microbiome isolates (Fig. S4l-m).
The correlation analysis between the top altered compounds and the KEGG pathways identified through WGS analysis revealed potential connections with the inhibition function. For example, both Adenine and Adenosine are associated with alpha-amylase, V-type ATPase, Nickel ABC-type substrate-binding NikA, and staphyloaxanthin biosynthesis pathways. Additionally, Adenosine is specifically linked to 4-Hydroxybutyrate/3-hydroxypropionate, thiamin biosynthesis, transporter: phosphate, Cobalt transporter CbiMQ, Copper transporter CopA, proline, and starch/glycogen synthesis ( and Table S6).
Opposing roles of dipeptides on Salmonella growth
Among the top metabolite candidates, we identified several dipeptides including Val-Pro, Trp-Pro, Pro-Phe, Tyr-Pro, Leu-Pro, and Leu-Thr that were significantly higher in the supernatant from the Salmonella-depleted co-cultures or the L. reuteri co-culture (positive control) compared to the co-cultures with unaltered Salmonella (, Fig. S3j-k, Table S3, and Table S7). These dipeptides have not been well characterized before, especially on their potential function on infection. To investigate their potential roles, we supplemented Salmonella with synthetic dipeptides and monitored the Salmonella growth for 24 h. Interestingly, Val-Pro and Trp-Pro demonstrated inhibition of Salmonella growth after 24 h, while Pro-Phe and Tyr-Pro demonstrated the opposite function that boosted Salmonella growth starting at 8-h Salmonella (). Tyr-Pro showed the strongest growth promotion among all the tested compounds. No significant difference was found for related other dipeptides (Fig. S4n-o). These findings suggest that the identified dipeptides may have opposing functions in Salmonella infection, with some such as Val-Pro and Trp-Pro potentially secreted by commensal bacteria to combat Salmonella, and others such as Pro-Phe and Tyr-Pro produced by Salmonella as a defense mechanism. The study also highlights the complexity of the interplay between commensal bacteria and pathogens in the gut, as different dipeptides may have different effects on bacterial growth. Further investigation is needed to fully elucidate the functions of these dipeptides in infections and their broader implications for gut health.
Figure 6. Dipeptides demonstrated the opposite function in Salmonella growth.
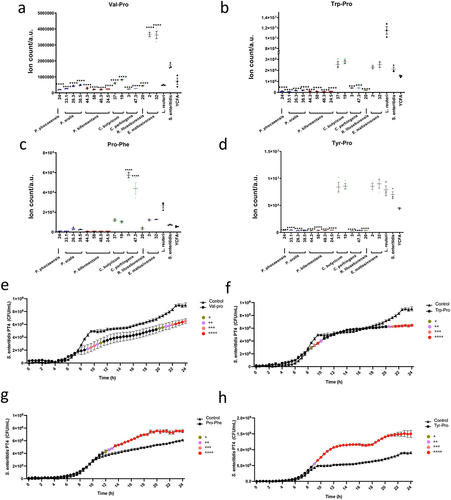
Methods
Bacterial strain isolations
The anaerobically cultivated human intestinal microbiota (ACHIM) culture is a cultivation of a healthy adult donor’s gut microbiota in vitro. ACHIM culture is stable at the species level and had been tested pathogen-free and applied in clinical trials.Citation10,Citation21 Our recent study observed that ACHIM significantly reduced Salmonella growth.Citation10 To identify the specific active bacteria, single isolates were collected by sequential dilution of the ACHIM culture on Deutsche Sammlung von Mikroorganismen und Zellkulturen (DSMZ) modified Yeast Casitone Fatty Acids (YCFA) medium agar plates after 48 h of anaerobic incubation. Isolated gut commensal colonies were then anaerobically cultured in fresh modified YCFA liquid medium for these experiments.
S. enteritidis PT4 (Salmonella) was cultured in Luria-Bertani (LB) broth aerobically, and Lactobacillus reuteri DSM 17,938 was cultured in DeMan, Rogosa, and Sharpe (MRS) medium anaerobically before the commensal bacteria-Salmonella co- culture assay. The co-cultured of Salmonella with gut commensal isolates or L. reuteri as the positive control was carried out in DSMZ modified YCFA medium anaerobically. Bacteria for metabolite function assays (S. Typhimurium SL1344, S. aureus ATCC 29,213, E. coli ST131, E. coli ATCC 35,218, and K. pneumoniae ATCC 25,955) were cultured aerobically in M9 minimal medium (glucose 0.4%) with 1 mmol/L metabolite compound.
Bacterial isolate WGS and bioinformatics analysis
The genomic DNA of each gut commensal bacterial isolate was extracted using the DNAeasy Blood & Tissue Kit (Qiagen, Germany) according to the standard protocols provided. DNA sequencing library preparation and DNA sequencing were performed following the protocols and standard pipelines for the Illumina MiSeq platform to generate 2 × 150 bp paired-end reads (Illumina, California, USA). FASTQ read pairs for each isolate WGS library were processed using the BACTpipe 2.6.0 workflow.Citation46 Reads that passed quality assessment using BBDuk (http://sourceforge.net/projects/bbmap/) were subsequently screened for contaminants using Mash Screen.Citation47 The surviving reads were then assembled into draft genomes using Shovill (https://github.com/tseemann/shovill). Pairwise comparisons of all isolate WGS assemblies for average nucleotide identity (ANI) were performed using the ‘sketch’ and ‘comparesketch’ scripts of the BBMap software package (http://sourceforge.net/projects/bbmap/). A genome similarity matrix based on pairwise ANI values was created by assessing the Pearson correlation coefficient for all ANI scores of unique isolate pairs using the ‘corr’ method of the Python module Pandas,Citation48 followed by plotting with the ‘clustermap’ method from Seaborn.Citation49
Taxonomic inference for each isolate based on WGS nucleotide composition was carried out using Sourmash 4.2.2.Citation50 Briefly, MinHash sketches made for each isolate WGS assembly with a k-mer size of 31 and scaled setting of 2000 were queried against subjects in the genome taxonomy database (GTDB) r202.Citation33 The ‘search’ sub-command was used to identify the ‘best-match’ between a subject in GTDB r202 (https://sourmash.readthedocs.io/en/latest/databases.html) and each ACHIM isolate WGS sketch. The ‘lca classify’ sub-command was used to generate taxonomic ranks for each of the 74 ACHIM isolates using the k-31 version of GTDB r202. The all-by-all pairwise comparisons of the Jaccard Similarity Index values between ACHIM isolate WGS MinHash sketches performed with the ‘compare’ subcommand were visualized in R 4.1 with the Complex Heatmap 2.10.0 package.Citation51
Standardized bioinformatic pipelines for gene prediction using Prodigal 2.6.3.Citation52 and corresponding functional annotation of protein-coding sequences (CDS) were implemented on ACHIM isolate WGS assemblies using automated workflows comprised within Bactopia 2.0.2.Citation53 The multi-fasta amino acid sequence files generated by Prokka 1.14.6Citation54 were used as inputs for eggnog-mapper v2,Citation34 which was run with default settings using Diamond 2.0.11.Citation55 Annotations from the Clusters of Orthologous Genes (COG) databaseCitation56 were summarized into functional profiles for each isolate in R using functions from the Tidyverse 1.3.1 package.Citation57 Adapted from previously reported methods, the fractional genomic abundances of each eggNOG orthologous group (OG) of genesCitation58 present within isolate genomes were calculated in R and used to generate a sample-wise feature table.Citation59 All COG functional profiles were filtered to keep only those present in all samples being analyzed for a given analysis. Functional distances based on eggNOG-OG abundances were determined by ordination with the R package Phyloseq using the PCoA method and Bray-Curtis dissimilarity.Citation60
KEGG Pathway analysis of amino acid sequences generated by Prokka for each isolate was carried out using Kofamscan 1.3.0 with a default score threshold setting for KEGG Ortholog profile HMM assignment.Citation61 KEGG-Decoder was used to assess KEGG pathway completion for each isolate.Citation62 KEGG pathway completion results were filtered in R with 2 conditions based on the Salmonella “Kill” phenotype: (1) keep pathways with non-zero values in at least 2 out of 3 ‘Kill’ isolates and zero values in all ‘No_Kill’ isolates, and (2) keep pathways with zero values in at least 2 out of 3 ‘No_Kill’ isolates and non-zero values in at least 1 ‘Kill’ isolate. Correlation analysis between KEGG pathways and significant metabolite abundances was performed in R using the corrplot 0.92 package. Significant metabolite abundances used in correlation analyses consisted of mean values (n = 3). Correlation matrices and p-value estimation were carried out using the non-parametric Spearman rank correlation method. Significant correlations had estimated p ≤ 0.05.
Commensal bacteria-Salmonella co-culture assay
Considering that the infectious dose of Salmonella enteritidis is approximately 1.5 × 104 CFU/g, in our earlier publication, we employed 106 CFU in a 10ml culture to ensure the establishment of infection, which could be monitored in our ACHIM system. To maintain consistency with our previous publications for comparison, a dosage of 106 CFU was utilized in the current experiment.Citation10 Each commensal gut isolate (1 optical density (OD) in 1 mL experiment volume) was co-cultured with 10Citation6 CFU (1 × 106 CFU/mL in 1 mL experiment volume) of Salmonella in culture tubes with modified YCFA liquid medium and incubated for 24 and 48 h anaerobically. Salmonella without commensal bacteria and Salmonella co-cultured with L. reuteri were used as controls.Citation63 To quantify the bacteria growth, sequentially diluted co-culture was inoculated both on Salmonella-selective agar plates to get the Salmonella CFU and on YCFA plates to get the total bacteria number that could be cultured in the mix. The plates were cultured at 37°C for 48 h, aerobically for Salmonella-selective agar plates and anaerobically for YCFA plates as published.Citation10 Bacteria numbers were counted from each condition and calculated by dilution factors. All experiments were repeated three times with triplicates. The co-culture assays performed with the isolates close to ‘Kill’ and ‘No_Kill’ followed the same steps and were repeated twice with duplicates.
Metabolite extraction and ultraperformance liquid chromatography-tandem mass spectrometry (UPLC-MS/MS) analysis
Bacteria supernatants were filtered through a 0.2 µm filter before four times the volume of methanol (Fisher Scientific, USA) was added to precipitate large cell components and proteins. The solution was stored at −80°C until the metabolite extraction. An aliquot of the mixture (500 µL) was centrifuged at 13,500 rpm for 5 min (Eppendorf, USA) before the supernatant was dried under vacuum (SpeedVac – Eppendorf, USA) and re-dissolved in 100 µL of a 5% acetonitrile solution in water. Metabolites were analyzed by ultrahigh-performance liquid chromatography coupled with mass spectrometry (UPLC-MS) and identified through MS/MS fragmentation analysis, which differ among the three time points (Acquity UPLC system connected to a Synapt G2 Q-TOF mass spectrometer (Waters Corporation, USA)). The system was controlled through the MassLynx software package v 4.1 (Waters Corporation, USA). The metabolites were separated on an Acquity UPLC® HSS T3 column (1.8 µm, 100 × 2.1 mm) (Waters Corporation, USA). The mobile phase comprised 0.1% formic acid in MilliQ water (A) plus 0.1% formic acid in LC-MS grade methanol (B). The mobile phase gradient was set as follows: 0–2 min, 0% B; 2–15 min, 0–100% B; 15–18 min, 100% B; 18–20 min, 100–0% B; 20–25 min, 0% B, with the flow rate at 0.3 mL/min and column temperature at 40°C. The samples were transferred into the q-TOF via positive electrospray ionization. The capillary voltage was 2.50 kV and the cone voltage was set as 40 V. The source temperature was kept at 100°C, with the cone gas flow at 50 L/min and the desolvation gas flow at 600 L/h. The instrument was operated in MSE mode, with the scan range at m/z = 50–1200 and the scan time at 0.3 s. The instrument was calibrated using a solution of sodium formate (0.5 mM in 2-propanol: water, 90:10, v/v) and lock mass correction was done by using a solution of leucine-encephalin (2 ng/µL in acetonitrile: 0.1% formic acid in the water, 50:50, v/v) at an injection rate of 30 s.
Functional assays of metabolite activity
To identify the effect of metabolite compound on pathogen growth, 1 × 106 CFU/mL pathogen bacterial strain was cultured in 100 µL M9 minimal medium with 1 mmol/L metabolite compound in a 96-well plate format and incubated at 37°C for 24 h. The growth of bacteria was detected by the SpectraMax® i3× Multi-Mode Microplate Reader with absorbance at 600 nm (OD600) every 30 min. The pathogens cultured in 100 µL of M9 minimal medium without additional compounds was considered as control. The synergistic effects of the metabolites and the antibiotics were tested by co-culture Salmonella with the resisted antibiotics and metabolites in M9 medium for 24 h. To test the gut microbiome isolates, two commensal strains that exhibited significant inhibitory functions were cultured in 100 µL of YCFA medium under anaerobic conditions, as these strains did not grow under pathogenic conditions. A 1:100 dilution of overnight culture with OD = 1, supplemented with 1 mmol/L adenosine or adenine, was used as the starting concentration. Each experimental condition was repeated at least three times. Data were visualized by GraphPad Prism 9.
Statistical data analyses
The difference in bacterial growth among different co-culture systems of ACHIM isolates and Salmonella were compared by the Kruskal–Wallis H test with post hoc tests and Dunn’s multiple-comparison test and visualized with GraphPad Prism software. The growth of Salmonella with metabolites in 24 h was compared to that of the control group with only Salmonella growing in culture medium by two-way ANOVA with post hoc test Šídák’s multiple comparisons test. The obtained UPLC-MS data comparing the different time points were analyzed using the XCMS software package under R (version 3.3.0) to perform peak detection, alignment, peak filling, and integration.Citation64 Metaboanalyst was used for generation of the principal component analysis charts and heatmaps. Data were autoscaled before uni- and multivariate analysis.Citation65
Discussion
The discovery of bioactive metabolites produced by the commensal microbiome is a new and promising strategy with multiple opportunities to identify unknown antimicrobial compounds that inhibit pathogenic infection. This is of great importance, as the impending crisis of antibiotic-resistant bacteria that threatens millions of lives, especially those of pregnant women, infants, and people with weakened immune systems, and generates an enormous clinical and societal burden. Towards this goal, we have initially isolated over 100 commensal bacterial strains from a gut microbiota that demonsttated inhibition of Salmonella growth.Citation10 We carried out whole-genome sequencing on all these bacteria and evaluated representative strains for their inhibition effect on Salmonella growth. The metabolomic analysis revealed that adenosine, adenine, agmatine, phenylalanine, glutamine, guanine, and several dipeptides, i.e., Leu-Pro, Val-Pro, Leu-Thr, Pro-Phe, Try-Pro, and Tyr-Pro, demonstrated significantly higher levels in these co-culture systems inhibiting Salmonella growth than bacteria that did not lead to any inhibition. Further validation with adenosine and adenine revealed direct inhibition of Salmonella growth. This observation was confirmed with multiple antibiotic-resistant pathogens. Overall, our study provides a powerful strategy for identifying and screening gut microbiome-derived metabolites against pathogens, especially multidrug-resistant bacterial strains.
All isolated strains from the in vitro commensal gut microbiota culture belong to the Firmicutes or Bacteroidetes phyla, which are from the two major phyla in the gut that represent about 90% of the gut microbiota.Citation66,Citation67 Many isolates from the Firmicutes phylum belong to the Clostridium genera, which usually represents 95% of the Firmicutes phylum.Citation66 P. phocaeensis is the only species we isolated from the Bacteroidetes phylum. We further identified C. perfringens, C. butyricum, and E. maltosivorans but not P. phocaeensis, P. oralis, P. bifermentans, and R. litusburensis, isolates with strong inhibitory effects on Salmonella growth. This is in line with others showing that the spore-forming Clostridia species protected neonatal mice from S. typhimurium infection and a combination of Enterobacteriaceae and spore-forming bacteria from Firmicutes phylum conferred colonization resistance to S. enteritidis.Citation68,Citation69
Our metabolomics-based investigation of co-culture samples identified previously unknown bioactive microbiome-derived metabolites. Notably, similar to earlier publications, we identified that adenosine and adenine, both are components of purine metabolism, directly inhibit Salmonella growth.Citation70,Citation71 Nucleosides and their derivatives have been reported to show antibacterial activity by inhibiting cell-wall peptidoglycan biosynthesis and some bacterial enzymes, such as purine nucleoside phosphorylases and DNA ligases.Citation72,Citation73 Adenosine is highly abundant in the intestine and is estimated to be 5 mmol/L in the lumen of a normal human intestine and may reach 6 mmol/L in an inflamed intestine.Citation74,Citation75 Increased adenosine signaling suppresses excessive inflammatory reactions and protects against immune-mediated damage.Citation76 Adenosine has also been reported to have a significant antibacterial effect against oral Streptococci.Citation77 Adenosine analogues having cyclopentyl or deoxyribose groups in the NCitation6−position exhibited good antimicrobial properties.Citation72 Moreover, several adenosine analogues were reported to have antibacterial activity, such as S. aureus.Citation72,Citation78 Our correlation analysis between metabolites and WGS also demonstrated multiple potential pathways that these compounds could potentially affect. Interestingly, our data demonstrate that adenosine has a more broad-spectrum effect on several pathogens including multi-drug resistance strains S. aureus and several gram-negative bacteria: S. enteritidis, S. Typhimurium, K. pneumoniae, and E. coli 35218.
Adenine is a biosyntheitic precursor of adenosine.Citation79 Adenine can also be formed by partial degradation of adenosine with de-glycosylation and has been reported to regulate the immunological functions of lymphocytes and restrain the inflammatory reactions induced by lipopolysaccharide (LPS).Citation80–83 Multiple adenine derivatives have been reported to engage in antibacterial activity against both gram-positive and gram-negative bacteria.Citation84–86 Our experiments support these findings and demonstrate a significant inhibitory effect of adenine on the growth of S. aureus, S. enteritidis, S. Typhimurium, and E. coli 35,218. However, adenine is not an ideal drug for infection clearance because a chronic low-dose adenine diet in rats has been widely used to establish chronic kidney disease models.Citation87,Citation88 Notably, both adenosine and adenine inhibited the growth of E. coli 35218 but not extended-spectrum β-lactamase (ESBL)-producing bacteria E. coli ST131. Moreover, synergistic effects have been reported for exogenous supplementation of metabolites that effectively improved antibiotic treatments against multidrug-resistant pathogens.Citation89,Citation90 Integrated biochemical screening and network modeling suggested that antibiotic-induced intracellular adenine limitation would increase ATP demand, leading to elevated central metabolism activity and enhanced antibiotic lethality.Citation91 However, no synergistic effects of adenosine or adenine with ampicillin on ampicillin-resistant E. coli strains were observed in our study.
Dipeptides comprise a class of metabolites that have most commonly been described as downstream or degradation products of bigger proteins. Recently, two different studies have reported that the three dipeptides, i.e., Tyr-Leu, Phe-Leu, and Try-Leu, possess anxiolytic properties.Citation92,Citation93 The relevance of dipeptides has increased within the era of the microbiome, as several of these metabolites are produced or can influence microbiota or bacterial growth. Hsueh et al. have described the correlation between the levels of several dipeptides with interleukin-8 (IL-8) expression in the treatment of patients with bacterial pneumonia.Citation94 Another publication details the effect of basic and bulky hydrophobic dipeptides in the acceleration of protein degradation through interaction with the ubiquitination sites.Citation95 Additionally, the importance of regulating fecal microbiome was demonstrated in an increased level of the genus Prevotella in the human feces as both dipeptides promoted the growth of these bacteria.Citation96 Most of the dipeptides have not been well studied although they are commonly found in the intestines. Herein, we identified and further investigated the six dipeptides (Val-Pro, Trp-Pro, Pro-Phe, Tyr-Pro, Leu-Pro, and Leu-Thr), and evaluated their impact on Salmonella growth. Our data demonstrates that Val-Pro and Trp-Pro exhibited weak yet significant inhibition on Salmonella after 24 h, while Pro-Phe, Tyr-Pro promoted the growth of Salmonella. These novel observations shed light on the potential mechanisms and new bioactive metabolites involved in the clearance of Salmonella infections and host defense mechanisms through unknown gut microbiome-pathogen interaction.
In conclusion, our study provides an interdisciplinary pipeline for the discovery of bioactive antimicrobial metabolites produced by the gut microbiome against pathogens. Strains from C. perfringens, C. butyricum, and E. maltosivorans were identified as the key microbes to inhibit Salmonella growth. Adenosine and adenine were discovered to directly inhibit the growth of pathogens including several multi-drug-resistant pathogens. We also demonstrated novel dipeptide features which suggest potential interactions and signaling between gut microbes and pathogens. We believe that our described powerful pipeline can be utilized for investigation of additional strains for discovery of metabolites with promising potential as new microbiome-derived drugs against antibiotic-resistant pathogens.
Contributors
Conception and design: D.G. and J.D.
Sample collection and development of methodology: L.C., M.S.P.C., D.G. and J.D.
Acquisition of data: L.C., M.S.P.C., F.R.G., E.J., Å.S. and E.L.N.
Analysis and interpretation of data: L.C., M.S.P.C., S.M.H., F.R.G., I.T, F.B., D.G. and J.D.
Writing of the manuscript: L.C., M.S.P.C., F.R.G., I.T, D.G. and J.D.
Review and/or revision of the manuscript: All authors.
Data and materials availability
All the data generated from this study are included in this paper. Code used to analyze data and generate figures is available on GitHub (https://github.com/ctmrbio/achim_smh1). The WGS sequencing data for the isolates are available in the European Nucleotide Archive (ENA) database under accession project number PRJEB59984.
Supplemental Material
Download Zip (13 MB)Acknowledgments
Ferring Phamaceuticals funded the center where this project was carried out. We thank all the support from the Centre for Translational Microbiome Research (CTMR), Karolinska Institutet, Sweden.
Disclosure statement
No potential conflict of interest was reported by the author(s).
Supplementary material
Supplemental data for this article can be accessed online at https://doi.org/10.1080/19490976.2024.2356275
Additional information
Funding
References
- Kabeerdass N, Krishnamoorthy S, Anbazhagan M, Srinivasan R, Nachimuthu S, Rajendran M, Mathanmohun M. Screening, detection and antimicrobial susceptibility of multi-drug resistant pathogens from the clinical specimens. Mater Today: Proc. 2021;47:461–22. doi:10.1016/j.matpr.2021.05.018.
- Santacroce L, Di Domenico M, Montagnani M, Jirillo E. Antibiotic resistance and microbiota response. Curr Pharm Des. 2023;29(5):356–364. doi:10.2174/1381612829666221219093450.
- Samarra A, Esteban-Torres M, Cabrera-Rubio R, Bernabeu M, Arboleya S, Gueimonde M, Collado MC. Maternal-infant antibiotic resistance genes transference: what do we know? Gut Microbes. 2023;15(1):2194797. doi:10.1080/19490976.2023.2194797.
- Ding Y, Jiang X, Wu J, Wang Y, Zhao L, Pan Y, Xi Y, Zhao G, Li Z, Zhang L. Synergistic horizontal transfer of antibiotic resistance genes and transposons in the infant gut microbial genome. mSphere. 2024;9(1):e0060823. doi:10.1128/msphere.00608-23.
- Troeger C, Forouzanfar M, Rao PC, Khalil I, Brown A, Reiner RC, Fullman N, Thompson RL, Abajobir A, Ahmed M. et al. Estimates of global, regional, and national morbidity, mortality, and aetiologies of diarrhoeal diseases: a systematic analysis for the global burden of disease study 2015. Lancet Infect Dis. 2017;17(9):909–948. doi:10.1016/S1473-3099(17)30276-1.
- De Oliveira DMP, Forde BM, Kidd TJ, Harris PNA, Schembri MA, Beatson SA, Paterson DL, Walker MJ. Antimicrobial resistance in ESKAPE pathogens. Clin Microbiol Rev. 2020;33(3). doi:10.1128/CMR.00181-19.
- Botelho J, Cazares A, Schulenburg H. The ESKAPE mobilome contributes to the spread of antimicrobial resistance and CRISPR-mediated conflict between mobile genetic elements. Nucleic Acids Res. 2023;51(1):236–252. doi:10.1093/nar/gkac1220.
- Ducarmon QR, Zwittink RD, Hornung BVH, van Schaik W, Young VB, Kuijper EJ. Gut microbiota and colonization resistance against bacterial enteric infection. Microbiol Mol Biol Rev. 2019;83(3). doi:10.1128/MMBR.00007-19.
- Zheng Y, Hunt RL, Villaruz AE, Fisher EL, Liu R, Liu Q, Cheung GYC, Li M, Otto M. Commensal Staphylococcus epidermidis contributes to skin barrier homeostasis by generating protective ceramides. Cell Host Microbe. 2022;30(3):301–313.e9. doi:10.1016/j.chom.2022.01.004.
- Hu YOO, Hugerth LW, Bengtsson C, Alisjahbana A, Seifert M, Kamal A, Sjöling Å, Midtvedt T, Norin E, Du J. et al. Bacteriophages synergize with the gut microbial community to combat Salmonella. mSystems. 2018;3(5). doi:10.1128/mSystems.00119-18.
- Ramirez J, Guarner F, Bustos Fernandez L, Maruy A, Sdepanian VL, Cohen H. Antibiotics as major disruptors of gut microbiota. Front Cell Infect Microbiol. 2020;10:572912. doi:10.3389/fcimb.2020.572912.
- Yip AYG, King OG, Omelchenko O, Kurkimat S, Horrocks V, Mostyn P, Danckert N, Ghani R, Satta G, Jauneikaite E. et al. Antibiotics promote intestinal growth of carbapenem-resistant Enterobacteriaceae by enriching nutrients and depleting microbial metabolites. Nat Commun. 2023;14(1):5094. doi:10.1038/s41467-023-40872-z.
- Kim S, Covington A, Pamer EG. The intestinal microbiota: Antibiotics, colonization resistance, and enteric pathogens. Immunol Rev. 2017;279(1):90–105. doi:10.1111/imr.12563.
- Yadegar A, Pakpoor S, Ibrahim FF, Nabavi-Rad A, Cook L, Walter J, Seekatz AM, Wong K, Monaghan TM, Kao D. Beneficial effects of fecal microbiota transplantation in recurrent Clostridioides difficile infection. Cell Host Microbe. 2023;31(5):695–711. doi:10.1016/j.chom.2023.03.019.
- Lopetuso LR, Deleu S, Godny L, Petito V, Puca P, Facciotti F, Sokol H, Ianiro G, Masucci L, Abreu M. et al. The first international Rome consensus conference on gut microbiota and faecal microbiota transplantation in inflammatory bowel disease. Gut. 2023;72(9):1642–1650. doi:10.1136/gutjnl-2023-329948.
- Cheng Y, Tan G, Zhu Q, Wang C, Ruan G, Ying S, Qie J, Hu X, Xiao Z, Xu F. et al. Efficacy of fecal microbiota transplantation in patients with Parkinson’s disease: clinical trial results from a randomized, placebo-controlled design. Gut Microbes. 2023;15(2):2284247. doi:10.1080/19490976.2023.2284247.
- Wang J-W, Kuo C-H, Kuo F-C, Wang Y-K, Hsu W-H, Yu F-J, Hu H-M, Hsu P-I, Wang J-Y, Wu D-C. Fecal microbiota transplantation: Review and update. J Formosan Med Assoc. 2019;118(Suppl 1):S23–31. doi:10.1016/j.jfma.2018.08.011.
- Gerding DN, Meyer T, Lee C, Cohen SH, Murthy UK, Poirier A, Van Schooneveld TC, Pardi DS, Ramos A, Barron MA. et al. Administration of spores of nontoxigenic Clostridium difficile strain M3 for prevention of recurrent C. difficile infection: a randomized clinical trial. JAMA. 2015;313(17):1719–1727. doi:10.1001/jama.2015.3725.
- van der Lelie D, Oka A, Taghavi S, Umeno J, Fan T-J, Merrell KE, Watson SD, Ouellette L, Liu B, Awoniyi M. et al. Rationally designed bacterial consortia to treat chronic immune-mediated colitis and restore intestinal homeostasis. Nat Commun. 2021;12(1):3105. doi:10.1038/s41467-021-23460-x.
- Benno P, Dahlgren A-L, Befrits R, Norin E, Hellström PM, Midtvedt T. From IBS to DBS: the dysbiotic bowel syndrome. J Investig Med High Impact Case Rep. 2016;4(2):2324709616648458. doi:10.1177/2324709616648458.
- Jorup-Rönström C, Håkanson A, Sandell S, Edvinsson O, Midtvedt T, Persson A-K, Norin E. Fecal transplant against relapsing Clostridium difficile-associated diarrhea in 32 patients. Scand J Gastroenterol. 2012;47(5):548–552. doi:10.3109/00365521.2012.672587.
- El Hage Chehade N, Ghoneim S, Shah S, Chahine A, Mourad FH, Francis FF, Binion DG, Farraye FA, Hashash JG. Efficacy of fecal microbiota transplantation in the treatment of active ulcerative colitis: A systematic review and meta-analysis of double-blind randomized controlled trials. Inflamm Bowel Dis. 2023;29(5):808–817. doi:10.1093/ibd/izac135.
- Thomas RM, Jobin C. Microbiota in pancreatic health and disease: the next frontier in microbiome research. Nat Rev Gastroenterol Hepatol. 2020;17(1):53–64. doi:10.1038/s41575-019-0242-7.
- Wang P, Wu, P.F., Wang H-J, Liao F, Wang F, Chen J-G. Gut microbiome-derived ammonia modulates stress vulnerability in the host. Nat Metab. 2023;5(11):1986–2001. doi:10.1038/s42255-023-00909-5.
- Zarour HM. Microbiome-derived metabolites counteract tumor-induced immunosuppression and boost immune checkpoint blockade. Cell Metab. 2022;34(12):1903–1905. doi:10.1016/j.cmet.2022.11.010.
- Jacobson A, Lam L, Rajendram M, Tamburini F, Honeycutt J, Pham T, Van Treuren W, Pruss K, Stabler SR, Lugo K. et al. A gut commensal-produced metabolite mediates colonization resistance to Salmonella infection. Cell Host Microbe. 2018;24(2):296–307.e7. doi:10.1016/j.chom.2018.07.002.
- Rosenberg G, Yehezkel D, Hoffman D, Mattioli CC, Fremder M, Ben-Arosh H, Vainman L, Nissani N, Hen-Avivi S, Brenner S. et al. Host succinate is an activation signal for Salmonella virulence during intracellular infection. Science. 2021;371(6527):400–405. doi:10.1126/science.aba8026.
- Li W, Separovic F, O’Brien-Simpson NM, Wade JD. Chemically modified and conjugated antimicrobial peptides against superbugs. Chem Soc Rev. 2021;50(8):4932–4973. doi:10.1039/d0cs01026j.
- Awad M, EL–Shahed HYIK, Aziz R, Sarmidi MR, El–Enshasy HA. Antibiotics as microbial secondary metabolites: production and application. J Teknol. 2013;59(1). doi:10.11113/jt.v59.1593.
- Chandra Mohana N, Yashavantha Rao HC, Rakshith D, Mithun PR, Nuthan BR, Satish S. Omics based approach for biodiscovery of microbial natural products in antibiotic resistance era. J Genet Eng Biotechnol. 2018;16(1):1–8. doi:10.1016/j.jgeb.2018.01.006.
- Francine P. Systems biology: New insight into antibiotic resistance. Microorganisms. 2022;10(12):2362. doi:10.3390/microorganisms10122362.
- Pierce NT, Irber L, Reiter T, Brooks P, Brown CT. Large-scale sequence comparisons with sourmash. F1000Res. 2019;8:1006. doi:10.12688/f1000research.19675.1.
- Parks DH, Chuvochina M, Rinke C, Mussig AJ, Chaumeil P-A, Hugenholtz P. GTDB: an ongoing census of bacterial and archaeal diversity through a phylogenetically consistent, rank normalized and complete genome-based taxonomy. Nucleic Acids Res. 2022;50(D1):D785–D794. doi:10.1093/nar/gkab776.
- Cantalapiedra CP, Hernández-Plaza A, Letunic I, Bork P, Huerta-Cepas J, Tamura K. eggNOG-mapper v2: Functional annotation, orthology assignments, and domain prediction at the metagenomic scale. Mol Biol Evol. 2021;38(12):5825–5829. doi:10.1093/molbev/msab293.
- Kanehisa M, Goto S. KEGG: Kyoto encyclopedia of genes and genomes. Nucleic Acids Res. 2000;28(1):27–30. doi:10.1093/nar/28.1.27.
- Vallianatou T, Bèchet NB, Correia MSP, Lundgaard I, Globisch D. Regional brain analysis of modified amino acids and dipeptides during the sleep/wake cycle. Metabolites. 2021;12(1):21. doi:10.3390/metabo12010021.
- Mars RAT, Yang Y, Ward T, Houtti M, Priya S, Lekatz HR, Tang X, Sun Z, Kalari KR, Korem T. et al. Longitudinal multi-omics reveals subset-specific mechanisms underlying irritable bowel syndrome. Cell. 2020;182(6):1460–1473.e17. doi:10.1016/j.cell.2020.08.007.
- Xiao L, Liu Q, Luo M, Xiong L. Gut microbiota-derived metabolites in irritable bowel syndrome. Front Cell Infect Microbiol. 2021;11:729346. doi:10.3389/fcimb.2021.729346.
- Zhang X-X, Ritchie SR, Rainey PB. Urocanate as a potential signaling molecule for bacterial recognition of eukaryotic hosts. Cell Mol Life Sci. 2014;71(4):541–547. doi:10.1007/s00018-013-1527-6.
- Fujiwara M, Kono N, Hirayama A, Malay AD, Nakamura H, Ohtoshi R, Numata K, Tomita M, Arakawa K. Xanthurenic acid is the main pigment of trichonephila clavata gold dragline silk. Biomolecules. 2021;11(4):563. doi:10.3390/biom11040563.
- Bailey AM, Paulsen IT, Piddock LJ. RamA Confers Multidrug Resistance in Salmonella enterica via Increased Expression of acrB, Which Is Inhibited by Chlorpromazine. Antimicrob Agents Chemother. 2008;52(10):3604–3611. doi:10.1128/AAC.00661-08.
- Olsson L. Detection of synergistic activity of antibiotics in Klebsiella Pneumoniae using MALDI-TOF MS. 2015. https://www.semanticscholar.org/paper/Detection-of-synergistic-activity-of-antibiotics-in-Olsson/889f7d0c80057eee95529ee95fe0707bf8cc0b7f.
- Wacher-Rodarte M del, Trejo-Muñúzuri TP, Montiel-Aguirre JF, Drago-Serrano ME, Gutiérrez-Lucas RL, Castañeda-Sánchez JI, Sainz-Espuñes T. Antibiotic resistance and multidrug-resistant efflux pumps expression in lactic acid bacteria isolated from pozol, a nonalcoholic Mayan maize fermented beverage. Food Science & Nutrition. 2016;4(3):423–430. doi:10.1002/fsn3.304.
- Paulshus E, Thorell K, Guzman-Otazo J, Joffre E, Colque P, Kühn I, Möllby R, Sørum H, Sjöling Å. Repeated Isolation of Extended-Spectrum-β-Lactamase-Positive Escherichia coli Sequence Types 648 and 131 from Community Wastewater Indicates that Sewage Systems Are Important Sources of Emerging Clones of Antibiotic-Resistant Bacteria. Antimicrob Agents Chemother. 2019;63(9). doi:10.1128/AAC.00823-19.
- Clinical and Laboratory Standards Institute (CLSI). Performance Standards for Antimicrobial Susceptibility Testing. CLSI supplement M100. USA: Clinical and Laboratory Standards Institute; 2022. https://clsi.org/media/2663/m100ed29_sample.pdf.
- Rudbeck E, Sharma A, Kirangwa J, Hu Y, Álvarez-Carretero S, Boulund F, Thorell K. ctmrbio/BACTpipe: BACTpipe v3.1.0. Zenodo. 2021. doi:10.5281/zenodo.4742358.
- Ondov BD, Starrett GJ, Sappington A, Kostic A, Koren S, Buck CB, Phillippy AM. Mash Screen: high-throughput sequence containment estimation for genome discovery. Genome Biol. 2019;20(1):232. doi:10.1186/s13059-019-1841-x.
- McKinney W. Pandas: a foundational python library for data analysis and statistics. Python High Perform Sci Comput. 2011;14(9):1–9.
- Waskom M. Seaborn: statistical data visualization. JOSS. 2021;6(60):3021. doi:10.21105/joss.03021.
- Titus Brown C, Irber L. sourmash: a library for MinHash sketching of DNA. JOSS. 2016;1(5):27. doi:10.21105/joss.00027.
- Gu Z, Eils R, Schlesner M. Complex heatmaps reveal patterns and correlations in multidimensional genomic data. Bioinformatics. 2016;32(18):2847–2849. doi:10.1093/bioinformatics/btw313.
- Hyatt D, Chen G-L, Locascio PF, Land ML, Larimer FW, Hauser LJ. Prodigal: prokaryotic gene recognition and translation initiation site identification. BMC Bioinformatics. 2010;11(1):119. doi:10.1186/1471-2105-11-119.
- Petit RA, Read TD, Segata N. Bactopia: a flexible pipeline for complete analysis of bacterial genomes. mSystems. 2020;5(4). doi:10.1128/mSystems.00190-20.
- Seemann T. Prokka: rapid prokaryotic genome annotation. Bioinformatics. 2014;30(14):2068–2069. doi:10.1093/bioinformatics/btu153.
- Buchfink B, Reuter K, Drost H-G. Sensitive protein alignments at tree-of-life scale using DIAMOND. Nat Methods. 2021;18(4):366–368. doi:10.1038/s41592-021-01101-x.
- Tatusov RL, Galperin MY, Natale DA, Koonin EV. The COG database: a tool for genome-scale analysis of protein functions and evolution. Nucleic Acids Res. 2000;28(1):33–36. doi:10.1093/nar/28.1.33.
- Wickham H, Averick M, Bryan J, Chang W, McGowan L, François R, Grolemund G, Hayes A, Henry L, Hester J. et al. Welcome to the tidyverse. JOSS. 2019;4(43):1686. doi:10.21105/joss.01686.
- Huerta-Cepas J, Szklarczyk D, Heller D, Hernández-Plaza A, Forslund SK, Cook H, Mende DR, Letunic I, Rattei T, Jensen LJ. et al. eggNOG 5.0: a hierarchical, functionally and phylogenetically annotated orthology resource based on 5090 organisms and 2502 viruses. Nucleic Acids Res. 2019;47(D1):D309–D314. doi:10.1093/nar/gky1085.
- Li Y, Wang M, Sun Z-Z, Xie B-B. Comparative genomic insights into the taxonomic classification, diversity, and secondary metabolic potentials of kitasatospora, a genus closely related to streptomyces. Front Microbiol. 2021;12:683814. doi:10.3389/fmicb.2021.683814.
- McMurdie PJ, Holmes S, Watson M. phyloseq: an R package for reproducible interactive analysis and graphics of microbiome census data. PLoS One. 2013;8(4):e61217. doi:10.1371/journal.pone.0061217.
- Aramaki T, Blanc-Mathieu R, Endo H, Ohkubo K, Kanehisa M, Goto S, Ogata H. KofamKOALA: KEGG Ortholog assignment based on profile HMM and adaptive score threshold. Bioinformatics. 2020;36(7):2251–2252. doi:10.1093/bioinformatics/btz859.
- Graham ED, Heidelberg JF, Tully BJ. Potential for primary productivity in a globally-distributed bacterial phototroph. Isme J. 2018;12(7):1861–1866. doi:10.1038/s41396-018-0091-3.
- Shi S, Qi Z, Sheng T, Tu J, Shao Y, Qi K. Antagonistic trait of Lactobacillus reuteri S5 against Salmonella enteritidis and assessment of its potential probiotic characteristics. Microb Pathog. 2019;137:103773. doi:10.1016/j.micpath.2019.103773.
- Smith CA, Want EJ, O’Maille G, Abagyan R, Siuzdak G. XCMS: processing mass spectrometry data for metabolite profiling using nonlinear peak alignment, matching, and identification. Anal Chem. 2006;78(3):779–787. doi:10.1021/ac051437y.
- Pang Z, Chong J, Zhou G, de Lima Morais DA, Chang L, Barrette M, Gauthier C, Jacques P-É, Li S, Xia J. MetaboAnalyst 5.0: narrowing the gap between raw spectra and functional insights. Nucleic Acids Res. 2021;49(W1):W388–W396. doi:10.1093/nar/gkab382.
- Arumugam M, Raes J, Pelletier E, Le Paslier D, Yamada T, Mende DR, Fernandes GR, Tap J, Bruls T, Batto J-M. et al. Enterotypes of the human gut microbiome. Nature. 2011;473(7346):174–180. doi:10.1038/nature09944.
- Groisman EA, Han W, Krypotou E. Advancing the fitness of gut commensal bacteria. Science. 2023;382(6672):766–768. doi:10.1126/science.adh9165.
- Kim Y-G, Sakamoto K, Seo S-U, Pickard JM, Gillilland MG, Pudlo NA, Hoostal M, Li X, Wang TD, Feehley T. et al. Neonatal acquisition of Clostridia species protects against colonization by bacterial pathogens. Science. 2017;356(6335):315–319. doi:10.1126/science.aag2029.
- Litvak Y, Mon KKZ, Nguyen H, Chanthavixay G, Liou M, Velazquez EM, Kutter L, Alcantara MA, Byndloss MX, Tiffany CR. et al. Commensal enterobacteriaceae protect against salmonella colonization through oxygen competition. Cell Host Microbe. 2019;25(1):128–139.e5. doi:10.1016/j.chom.2018.12.003.
- Xi H, Schneider BL, Reitzer L. Purine catabolism in Escherichia coli and function of xanthine dehydrogenase in purine salvage. J Bacteriol. 2000;182(19):5332–5341. doi:10.1128/JB.182.19.5332-5341.2000.
- Kilstrup M, Hammer K, Ruhdal Jensen P, Martinussen J. Nucleotide metabolism and its control in lactic acid bacteria. FEMS Microbiol Rev. 2005;29(3):555–590. doi:10.1016/j.fmrre.2005.04.006.
- Vitali LA, Petrelli D, Lambertucci C, Prenna M, Volpini R, Cristalli G. In vitro antibacterial activity of different adenosine analogues. J Med Microbiol. 2012;61(Pt 4):525–528. doi:10.1099/jmm.0.038174-0.
- Mills SD, Eakin AE, Buurman ET, Newman JV, Gao N, Huynh H, Johnson KD, Lahiri S, Shapiro AB, Walkup GK. et al. Novel Bacterial NAD + -Dependent DNA Ligase Inhibitors with Broad-Spectrum Activity and Antibacterial Efficacy In Vivo. Antimicrob Agents Chemother. 2011;55(3):1088–1096. doi:10.1128/AAC.01181-10.
- Ye JH, Rajendran VM. Adenosine: an immune modulator of inflammatory bowel diseases. World J Gastroenterol. 2009;15(36):4491–4498. doi:10.3748/wjg.15.4491.
- Kimura Y, Turner JR, Braasch DA, Buddington RK. Lumenal adenosine and AMP rapidly increase glucose transport by intact small intestine. Am J Physiol Gastrointest Liver Physiol. 2005;289(6):G1007–14. doi:10.1152/ajpgi.00085.2005.
- D’Antongiovanni V, Fornai M, Pellegrini C, Benvenuti L, Blandizzi C, Antonioli L. The adenosine system at the crossroads of intestinal inflammation and neoplasia. Int J Mol Sci. 2020;21(14):5089. doi:10.3390/ijms21145089.
- Al-Mashhadane FA, Hamdon SM, Aljader GH. The activity of adenosine on some oral bacteria: An in vitro study. J Pharmaceutical Sci Res. 2019;11(5):2019–2021.
- Srivastava R, Bhargava A, Singh RK. Synthesis and antimicrobial activity of some novel nucleoside analogues of adenosine and 1,3-dideazaadenosine. Bioorg Med Chem Lett. 2007;17(22):6239–6244. doi:10.1016/j.bmcl.2007.09.028.
- Hall J, Frenguelli BG. The combination of ribose and adenine promotes adenosine release and attenuates the intensity and frequency of epileptiform activity in hippocampal slices: Evidence for the rapid depletion of cellular ATP during electrographic seizures. J Neurochem. 2018;147(2):178–189. doi:10.1111/jnc.14543.
- Hosoi T, Ino S, Ohnishi F, Todoroki K, Yoshii M, Kakimoto M, Müller CE, Ozawa K. Mechanisms of the action of adenine on anti-allergic effects in mast cells. Immun Inflamm Dis. 2018;6(1):97–105. doi:10.1002/iid3.200.
- Silwal P, Shin K, Choi S, Kang SW, Park JB, Lee H-J, Koo S-J, Chung K-H, Namgung U, Lim K. et al. Adenine suppresses IgE-mediated mast cell activation. Mol Immunol. 2015;65(2):242–249. doi:10.1016/j.molimm.2015.01.021.
- Silwal P, Lim K, Heo J-Y, Park JI, Namgung U, Park S-K. Adenine attenuates lipopolysaccharide-induced inflammatory reactions. Korean J Physiol Pharmacol. 2018;22(4):379–389. doi:10.4196/kjpp.2018.22.4.379.
- Bizzarri BM, Fanelli A, Kapralov M, Krasavin E, Saladino R. Meteorite-catalyzed intermolecular trans-glycosylation produces nucleosides under proton beam irradiation. RSC Adv. 2021;11(31):19258–19264. doi:10.1039/d1ra02379a.
- Abdel-Monem RA, El-Sayed AA, Abdelhamid AE, Rabie ST. Adenine functionalized antibacterialPVC with both photo and thermal stability. J Vinyl Addit Technol. 2021;27(3):555–566. doi:10.1002/vnl.21827.
- Kinali-Demirci S, Idil O, Disli A, Demirci S. Adenine derivatives for regenerable antibacterial surface applications based on A−T base pairing. ChemistrySelect. 2020;5(32):10128–10134. doi:10.1002/slct.202002238.
- Sowrirajan S, Elangovan N, Chinnamani T, Vijayakumar G, Kolochi T. Antibacterial and antifungal activities on derivatives of adenine. Int J Adv Multidiscip Res. 2018;5(5):30–37. doi:10.22192/ijamr.2018.05.05.004.
- Yokozawa T, Zheng PD, Oura H, Koizumi F. Animal model of adenine-induced chronic renal failure in rats. Nephron. 1986;44(3):230–234. doi:10.1159/000183992.
- Diwan V, Brown L, Gobe GC. Adenine-induced chronic kidney disease in rats. Nephrology (Carlton). 2018;23(1):5–11. doi:10.1111/nep.13180.
- Peng B, Su Y-B, Li H, Han Y, Guo C, Tian Y-M, Peng X-X. Exogenous alanine and/or glucose plus kanamycin kills antibiotic-resistant bacteria. Cell Metab. 2015;21(2):249–262. doi:10.1016/j.cmet.2015.01.008.
- Liu Y, Li R, Xiao X, Wang Z. Bacterial metabolism-inspired molecules to modulate antibiotic efficacy. J Antimicrob Chemother. 2019;74(12):3409–3417. doi:10.1093/jac/dkz230.
- Yang JH, Wright SN, Hamblin M, McCloskey D, Alcantar MA, Schrübbers L, Lopatkin AJ, Satish S, Nili A, Palsson BO. et al. A white-box machine learning approach for revealing antibiotic mechanisms of action. Cell. 2019;177(6):1649–1661.e9. doi:10.1016/j.cell.2019.04.016.
- Kanegawa N, Suzuki C, Ohinata K. Dipeptide Tyr-Leu (YL) exhibits anxiolytic-like activity after oral administration via activating serotonin 5-HT1A, dopamine D1 and GABAA receptors in mice. FEBS Lett. 2010;584(3):599–604. doi:10.1016/j.febslet.2009.12.008.
- Mizushige T, Kanegawa N, Yamada A, Ota A, Kanamoto R, Ohinata K. Aromatic amino acid-leucine dipeptides exhibit anxiolytic-like activity in young mice. Neurosci Lett. 2013;543:126–129. doi:10.1016/j.neulet.2013.03.043.
- Hsueh P-C, Wu K-A, Yang C-Y, Hsu C-W, Wang C-L, Hung C-M, Chen Y-T, Yu J-S, Wu C-C. Metabolomic profiling of parapneumonic effusion reveals a regulatory role of dipeptides in interleukin-8 production in neutrophil-like cells. Anal Chim Acta. 2020;1128:238–250. doi:10.1016/j.aca.2020.06.022.
- Du F, Navarro-Garcia F, Xia Z, Tasaki T, Varshavsky A. Pairs of dipeptides synergistically activate the binding of substrate by ubiquitin ligase through dissociation of its autoinhibitory domain. Proc Natl Acad Sci USA. 2002;99(22):14110–14115. doi:10.1073/pnas.172527399.
- Sperk M, Ambikan AT, Ray S, Singh K, Mikaeloff F, Diez RC, Narayanan A, Vesterbacka J, Nowak P, Sönnerborg A. et al. Fecal metabolome signature in the HIV-1 elite control phenotype: enrichment of dipeptides acts as an HIV-1 antagonist but a prevotella agonist. J Virol. 2021;95(18):e0047921. doi:10.1128/JVI.00479-21.