ABSTRACT
The role of the intratumoral microbiome in gastric cancer (GC) has not been comprehensively assessed. Here, we explored the relationship between the microbial community and GC prognosis and therapy efficacy. Several cancer-associated microbial characteristics were identified, including increased α-diversity, differential β-diversity, and decreased Helicobacter pylori abundance. After adjusting for clinical features, prognostic analysis revealed 2 phyla, 14 genera, and 5 species associated with the overall survival of patients with GC. Additionally, 2 phyla, 14 genera, and 6 species were associated with adjuvant chemotherapy (ACT) efficacy in patients with stage II – III GC. Furthermore, we classified GC microbiome structures into three microbial subtypes (MS1, MS2 and MS3) with distinguishing features. The MS1 subtype exhibited high immune activity and enrichment of microbiota related to immunotherapy and butyric acid-producing, as well as potential benefits in immunotherapy. MS2 featured the highest α-diversity and activation of the TFF pathway, MS3 was characterized by epithelial-mesenchymal transition and was associated with poor prognosis and reduced ACT efficacy. Collectively, the results of this study provide valuable insights into the microbial characteristics associated with GC prognosis and therapy efficacy.
Introduction
As detection technologies have advanced and our understanding of the tumor microenvironment (TME) has deepened, increasing evidence has confirmed the presence of microbiota within, and complex interactions with, tumors.Citation1,Citation2 Intratumoral microbiota may affect cancer development and progression through myriad mechanisms, such as reshaping the TME, promoting tumor metastasis, and influencing therapy efficacy.Citation3 However, the precise role that intratumoral microbiota have in cancer pathogenesis, remains unclear. Hence, elucidating the complex interactions between the microbiota, TME, and cancer cells can provide valuable insights into potential cancer therapies and aid the evaluation of therapy efficacy for individual patients.
Gastric cancer (GC) is a complex disease with multiple risk factors. In particular, the intratumoral microbiota has emerged as a potential risk factor. Historically, the human stomach was thought to serve as the exclusive habitat for Helicobacter pylori due to its acidity and other antimicrobial factors, making it unsuitable for other microorganisms. However, this view has recently been challenged as non-Helicobacter pylori-microbiota were found to impact GC progression by altering immune and metabolic homeostasis.Citation4–8 Moreover, several studies have extensively characterized the microbiota profiles of the stomach and GC, and those associated with GC development.Citation9–12 However, there remains a lack of research into the relationship between intratumoral microbiota and the clinical features, prognosis, and chemotherapy and immunotherapy efficacy in GC. Hence, the clinical translation of gut microbiota in GC research has been limited.
The rapid advancement of omics technologies has led to the molecular and genomic characterization of GC, representing a significant area of research. Indeed, new GC subtypes have been established based on genomic, transcriptomic, and proteomic analyses, improving the stratification of patient prognosis and advancing the development of targeted therapies.Citation13–15 However, our understanding of microbial subtypes (Msubtypes) remains limited. Current research has focused on individual pathogens or is constrained by small sample sizes and a lack of clinical outcome information. Hence, studies seeking to characterize the Msubtypes associated with GC and elucidate their clinical significance and molecular features, are greatly needed.
In this study, we conducted a comprehensive analysis combining microbiome (16S rRNA gene sequencing) data from 251 GC samples with paired noncancerous adjacent tissues (NATs), transcriptome data from 94 GC samples, and detailed clinical characteristics. Our study aimed to elucidate the different microbial characteristics among clinical subgroups and identify microbiota with independent prognostic significance for overall survival (OS) and adjuvant chemotherapy (ACT). Additionally, we performed microbiome-centered cluster analysis to identify subtypes with distinct molecular characteristics associated with GC prognosis and chemotherapy and immunotherapy efficacy. Taken together, our findings reveal the association between intratumoral microbiota and clinical outcomes in GC, providing valuable insights for precision medicine.
Results
GC intratumoral microbiome landscape
Primary GC samples and paired NATs were collected from 251 patients with GC. The median age of the patients was 62 years (range: 36–84 years). Of the GC samples, 11.6% were Epstein-Barr virus (EBV)-positive (n = 29), 30.7% were mismatch repair deficient (dMMR; n = 77), and 57% were proficient mismatch repair (pMMR; n = 150). Moreover, 12.4% of the GC samples were stage I (n = 31), 36.7% were stage II (n = 92), 50.2% were stage III (n = 126), and 0.8% were stage IV (n = 2). Additionally, 66.5% (n = 167) of the patients received ACT after surgery. All clinical information is summarized in Table S1 and presented in . Information related to quality control is summarized in Table S2. The rarefaction curve indicated that the microecological characteristics of GC and NAT reached saturation with increased sequencing depth ().
Figure 1. Intratumoral microbiome landscape of gastric cancer (GC). (a) Summary of the clinical characteristics of 251 patients with GC. (b) Rarefaction curve of GC and noncancerous adjacent tissues (NAT). (c) α-diversity difference between GC and NAT. (d) Principal coordinate analysis (PCoA) of the microbiome data for GC and NAT. (e) Differences in microbiota abundances between GC and NAT. (f) α-Diversity differences and PCoA among dMMR, EBV and pMMR subtypes. (g) Microbiota abundance differences among dMMR, EBV and pMMR subtypes. (h) Correlations between genera (top 20) and EBV load. The size of the circle represents the magnitude of the Spearman correlation coefficient.
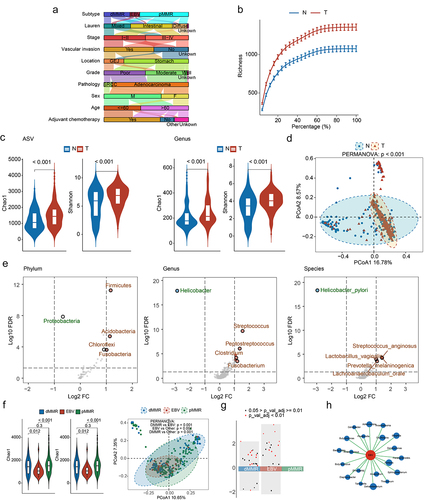
We evaluated the differences in the overall intratumoral microbial community structures between GC samples and NAT (251 pairs). Analyses of the GC and NAT genera profiles revealed a weak correlation (ρ = 0.08; Figure S1A). Moreover, GC samples exhibited increased α-diversity compared with those in NATs at both ASV and genus levels (). Furthermore, β-diversity analysis revealed a significant separation between GC and NAT samples (; PERMANOVA: p < 0.001). We also identified 16 phyla, 75 genera, and 34 species that were significantly enriched in GC compared with NAT (Adj p < 0.05; , Table S3–S5), including several well-established GC-associated bacteria such as Streptococcus, Prevotella, Fusobacterium, Parvimonas, and Lactobacillus.Citation16,Citation17 In addition, the Helicobacter genus and Helicobacter pylori species were predominant in NAT with markedly decreased abundance in the GC samples (Figure S1B). These findings highlight the distinct microbial ecologies between GC and NAT.
Considering the lack of consensus on the relationship between microbiota and clinical features, we compared the intratumoral microbial community structures of different clinical subgroups based on age, sex, pathology, tumor grade, location, vascular invasion, TNM stage, Lauren subtype, and molecular subtype. The results revealed that only the molecular subtype exhibited significant differences in microbial community structures ( and Figure S1C – S1D). Moreover, compared to pMMR subtype, both the EBV and dMMR subtypes showed a large number of different genera (). These findings suggest that dMMR and EBV subtypes have relatively specific microbial characteristics.
Currently, little is known regarding the association between EBV subtypes and microbiota. Hence, we conducted a transcriptome analysis of 94 tumor samples and aligned the data with the EBV genome to assess the viral load of each sample. As expected, the EBV subtypes exhibited higher EBV load. (Figure S1E). Further correlation analyses identified the top 20 genera positively correlated with the EBV load; Butyricicoccus was among these genera (). Interestingly, previous studies have reported a positive association between Butyricicoccus and EBV in the blood and plasma.Citation18 Furthermore, we observed a significant increase in the abundance of Butyricicoccus in the EBV subtype (Figure S1F). These results suggest a potential regulatory relationship between EBV and other microbiota in the EBV subtype.
Exploring the microbial taxa associated with GC OS and ACT
Increasing evidence has shown that intratumoral bacteria are associated with the prognosis of cancer patients.Citation19–21 However, the relationship between GC prognosis and the microbial profile remains unclear. Hence, we performed univariate Cox analysis to identify prognostic microbiota associated with patient OS at the phylum, genus, and species levels. Results identified 2 phyla, 23 genera, and 9 species associated with GC OS (Figure S2A). Furthermore, multivariable Cox regression was used to adjust for multiple clinical features, including age, sex, pathology, grade, location, vascular invasion, TNM stage, Lauren subtype, and molecular subtype, and to identify independent prognostic microbiota. Ultimately, 2 phyla, 14 genera, and 5 species were identified as independent prognostic microbiota for the OS of GC ().
Figure 2. Taxa associated with overall survival (OS) and adjuvant chemotherapy (ACT) after adjusting for clinical features. (a) Phyla, genera, and species associated with OS, after adjusting for clinical features. (b) Phyla, genera, and species associated with ACT, after adjusting for clinical features.
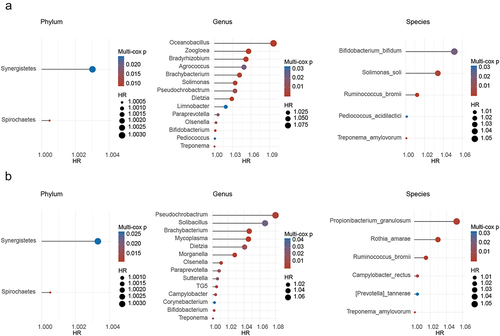
Considering that 5-fluorouracil-based ACT is the standard treatment for postoperative stage II and III GC, there is still a lack of awareness regarding the prognostic factors that influence the outcomes in this particular group.Citation22 Therefore, 2 phyla, 22 genera, and 12 species were identified to be associated with the OS of stage II – III GC receiving ACT (Figure S2B). After adjusting for clinical features, 2 phyla, 14 genera, and 6 species were considered the key microbiota influencing ACT efficacy ().
Identification of intratumoral microbial subtypes
The coexistence of microorganisms with anti-tumor and pro-tumor effects within the TME emphasizes the need to comprehensively evaluate the microbial characteristics rather than focusing on a single species. To gain a comprehensive understanding of the relationship between the intratumoral microbiome and GC outcomes, we identified three Msubtypes in the intratumoral microbial profiles (Figure S3). The MS1 subtype contained the most subtype-specific genera, including Parabacteroides, Ruminococcus, Butyricicoccus, and Lactobacillus. The MS2 subtype contained the most tumor-enriched genera, including Rhodoplanes, Nocardioides, Kaistobacter, and Pseudonocardia. Meanwhile, the MS3 subtype was characterized by Sphingobium, Delftia, Comamonas, and Stenotrophomonas. Delftia was associated with poor OS and poor ACT efficacy (). These three subtype categories were further supported by co-occurrence analysis of genera exhibiting strong positive correlations (Figure S4A).
Figure 3. Msubtypes and their correlation with clinical gastric cancer (GC) outcomes. (a) Heatmap of differentially abundant genera (Adj p < 0.05, Log2 FC > 2, and AUC > 0.7) among the Msubtypes. (b) Kaplan-Meier curve for overall survival (OS) among the Msubtypes. (c) α-Diversity differences and PCoA among the Msubtypes. (d) Co-abundance patterns among the genera of the Msubtypes.
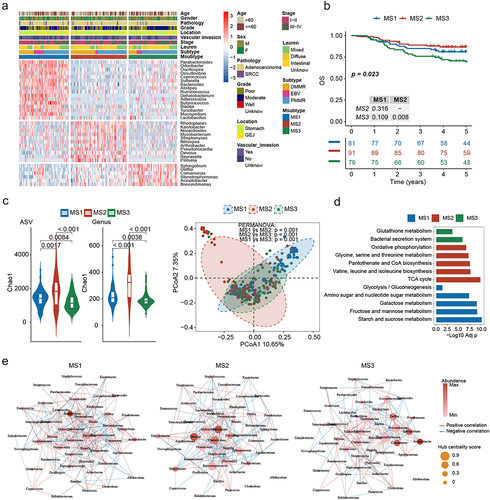
In the joint analysis with clinical features, EBV subtype was enriched within the MS1 subtype, dMMR subtype within the MS2 subtype, and pMMR subtype within the MS3 subtype. Moreover, no significant correlation was observed between the Msubtype and other clinical features (Table S6). Furthermore, survival analysis revealed that patients assigned to the MS3 subtype had the worst OS (). Additionally, the MS2 subtype had the highest α-diversity, and the MS3 subtype had the lowest. Meanwhile, the β-diversity analysis revealed that the microbial community exhibited phylogenetic differences within each subtype (PERMANOVA: p = 0.001; and Figure S4B).
To assess the metabolic differences between Msubtypes, we constructed a virtual metagenome for each sample’s microbiome using PICRUSt2 software. Several putative metacommunity-related functional shifts were identified between Msubtypes. More specifically, MS1 was characterized by the potential enrichment of carbohydrate metabolism pathways, MS2 exhibited increased potential for oxidative phosphorylation, TCA cycle, and amino acid metabolism, and MS3 might be associated with bacterial secretion system and glutathione metabolism (). To further investigate the variation patterns among the Msubtypes, we characterized the co-abundance patterns of each subtype. The MS1 subtype was characterized by the dominance of Halomonas and the hub genus Blautia. In contrast, MS2 was predominated by Burkholderia and the hub genus Chelativorans. The MS3 subtype was characterized by Acinetobacter and the hub genus Propionibacterium (). In total, 225 (MS1), 155 (MS2), and 145 (MS3) associations were identified (Figure S4C). Interestingly, the Msubtypes shared few associations, indicating significant changes in the symbiotic networks among the subtypes.
Multiple cohort analysis reveals that MS3 is associated with poor OS, RFS and poor chemotherapy efficacy
To further explore the biological characteristics of the three subtypes, we evaluated the transcriptome data for 94 samples. Gene Set Enrichment Analysis (GSEA) indicated that the MS1 subtype exhibited enrichment of numerous immune-related pathways, including PD1 signaling, suggesting its potential suitability for immunotherapy. The MS2 subtype was characterized by the TFF pathway, which plays an important role in maintaining the integrity of the gastrointestinal tract and promoting tissue repair. Meanwhile, the MS3 subtype was characterized by digestion and absorption, WNT signaling, and epithelial-mesenchymal transition (EMT; ). According to previous reports, Helicobacter pylori potentiates EMT in GC.Citation23,Citation24 Interestingly, we found that the MS3 subtype had the highest Helicobacter pylori abundance ().
Figure 4. MS3 is associated with poor overall survival (OS), regression-free survival (RFS), and poor chemotherapy efficacy. (a) GSEA of enriched pathways at the transcriptome level among Msubtypes. (b) Differences in Helicobacter pylori abundances among Msubtypes. (c) Kaplan-Meier curves for OS among the Msubtypes based on patients from the five transcriptome cohorts. (d) Kaplan-Meier curves for OS among the Msubtypes based on patients with stage II III gastric cancer (GC) who received adjuvant chemotherapy (ACT), from the three transcriptome cohorts. (e) Differences in response to neoadjuvant chemotherapy (NAC) among the Msubtypes, based on the published PUCH cohort data.
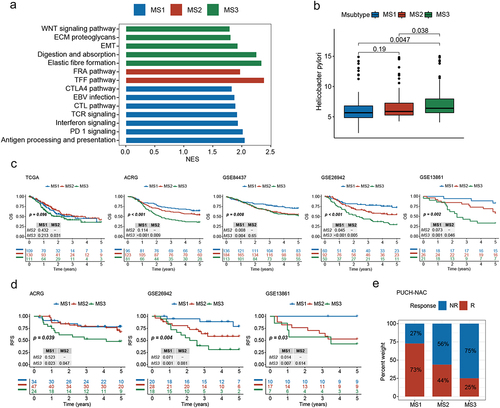
Immune infiltration analysis indicated that the MS1 subtype was dominated by CD8+ T cells (Figure S5A, Table S7), which may explain the better prognosis for patients with the MS1 subtype. We further determined whether the relationship between our subtypes and prognosis was universal by applying the Msubtypes to six published GC transcriptomic datasets (see Supplementary methods and Figure S5B). Survival analysis of multiple cohorts revealed that patients with the MS3 subtype had the worst OS and recurrence-free survival (RFS; and S5C). For patients with stage II – III GC who received ACT, the MS3 subtype was also associated with the worst OS and RFS ( and S5D). Moreover, the MS3 subtype exhibited resistance to neoadjuvant chemotherapy (NAC) in a cohort of patients at our center (). These results demonstrate the clinical relevance of the Msubtypes.
MS1 is associated with favorable efficacy of immunotherapy
The MS1 subtype is characterized by increased immune-related pathways and PD1 signaling, indicating its potential suitability for anti-PD1/PDL1 therapy. To further validate this result, we performed an integrative analysis of a curated list of 166 genes, including immune checkpoint genes and other known and emerging viable immunomodulatory targets (Table S8). The results showed that the expression of genes from all six immune-related categories was significantly upregulated in the MS1 subtype, including CD274 and IDO1 ( and Figure S6A).
Figure 5. MS1 is associated with favorable immunotherapy efficacy. (a) Differences in the expression of immune genes among the Msubtypes. (b) Differences in immunotherapy-associated mRNA signatures among the Msubtypes. (c) Differences in immunotherapy-associated microbiota signatures among Msubtypes. (d) Differences in CD8+ T cell-associated microbiota signatures among the Msubtypes. (e) Heatmap of butyric acid-producing genera among the Msubtypes. (f) Submap analysis of the three Msubtypes between our cohort and the response (R) and non-response (NR) subgroups of two gastric cancer (GC) cohorts who received immunotherapy.
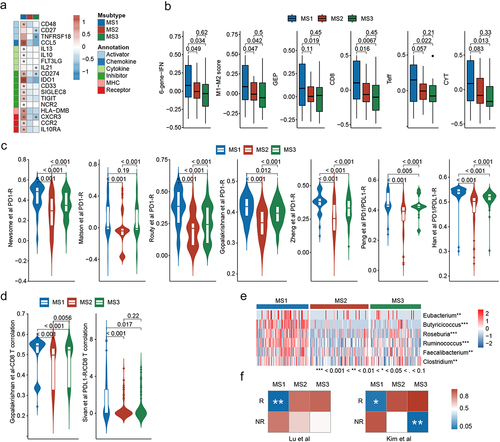
Considering that transcriptome and microbiome studies have aided the prediction of immunotherapy efficacy in various cancer types, we collected six mRNA signatures and nine microbiota signatures associated with immunotherapy response and CD8+ T cell infiltration (Table S9). The scores for these signatures were significantly higher in the MS1 subtype than the other subtypes in our cohort (). Further analysis showed that the MS1 subtype could effectively distinguish potential immunotherapy-responsive patients from non-responsive patients within the dMMR, EBV, and pMMR subtypes based on the microbiota signatures (Figure S6B). Intriguingly, several butyric acid-producing genera were significantly enriched in the MS1 subtype (); notably, butyric acid has been demonstrated to activate cytotoxic CD8+ T cells and enhance the effectiveness of anti-PD1 therapy.Citation25,Citation26
To further analyze the relationship between MS1 and immunotherapy, we performed a submap analysis between the three subtypes in our cohorts and the response (R) and non-response (NR) subgroups of the two GC cohorts receiving immunotherapy. The MS1 subtype was significantly associated with the responsive subgroups (), suggesting that the MS1 subtype may benefit from immunotherapy.
The regulation and shaping of tumor immunity are influenced by microbial microenvironments and the metabolites produced by microbesCitation27,Citation28 Currently, studies are establishing links between microbes and immune function.Citation29 The use of omics has accelerated the analyses of these relationships. Thus, to elucidate the association between microbes and immunity in these three Msubtypes, we applied the O2PLS model to perform a joint analysis of immune-related genes and microbial genera. The 15 most relevant immune genes and genera were identified for each Msubtype, which were used to construct networks. In the MS1 subtype, IDO1 and CD274 had the most associations with the microbiome, suggesting that they may be key targets for regulating the microbial microenvironment in this subtype. In the MS2 subtype, four genera exhibited extensive associations with immune genes, indicating that these genera might affect immunity in the MS2 subtype. However, the MS3 subtype lacked interactions between the immune system and microbiome (Figure S6C).
Discussion
In this study, we conducted intratumoral microbiome profiling of patients with GC. Our study sheds light on the relationship between the intratumoral microbiome and clinical features and outcomes of GC. We also identified three microbial subtypes with distinct microbial and genetic characteristics, offering potential guidance for personalized patient therapy. Moreover, the results of this study provide a valuable resource for further research into the clinical applications of the intratumoral microbiota.
Although Helicobacter pylori has been implicated in the malignant transformation, invasion, metastasis, and immune suppression of GC,Citation30 we found that the abundance of Helicobacter pylori was significantly reduced in GC compared to NAT and was not directly correlated with clinical outcomes. Meanwhile, the MS3 subtype, associated with the worst survival, had the highest Helicobacter pylori abundance. This suggests that Helicobacter pylori infection may play a complex role in GC initiation and progression.
While various omics studies have established a significant correlation between gut microbiota and cancer prognosis and treatment response,Citation31–33 research on intratumoral microbiota is limited. Our study addressed this issue and identified 2 phyla, 21 genera, and 8 species associated with OS or ACT efficacy in GC. These findings suggest that the microbiota composition can serve as a potential prognostic marker and may influence the efficacy of chemotherapy in GC. Interestingly, no beneficial microbiota associated with GC prognosis were found. Hence, from a translational perspective, the use of antibiotics rather than probiotics may improve GC prognosis. Indeed, antibiotics can inhibit the growth of GC cells and enhance the efficacy of chemotherapy for advanced GC.Citation34,Citation35 Our findings suggest the possibility that selective bacterial eradication or manipulation might improve prognosis. Although these findings require further validation, they provide insights into the potential clinical translation of the intratumoral microbiome in GC.
We employed a microbiota-based classification method to identify three GC subtypes, each with distinct molecular features that connect clinical and molecular characteristics. Specifically, we found that the MS1 subtype exhibited high immune activity and upregulated expression of multiple immune checkpoints, such as CD274 and IDO1. This suggests that immunotherapy may have potential benefits for patients with this subtype. This was supported further by the immune-related microbial features. Although these features were derived from the studies of the gut microbiome in patients undergoing immunotherapy, including two other GC-based studies,Citation36,Citation37 suggested that the intratumoral microbiome may originate from the gut microbiome.Citation38,Citation39 Interestingly, we observed the enrichment of butyric acid-producing genera in the MS1 subtype, indicating the potential enrichment of butyric acid. Butyric acid, an important short-chain fatty acid, plays a crucial role in immune regulation and gastrointestinal disorders.Citation40,Citation41 Recent studies have also indicated its positive impact on promoting CD8+ T cells activation and enhancing the efficacy of immunotherapy.Citation25,Citation26 The MS2 subtype featured the highest α-diversity and enrichment of the TFF pathway. The TFF pathway is associated with preserved gastric function, which may account for the relatively favorable prognosis of patients with this subtype. In contrast, the MS3 subtype was characterized by EMT features at the transcriptome level, which have been implicated in various aspects of tumor development, including tumor invasion, metastasis, cancer stemness, and therapy resistance.Citation42,Citation43 Additionally, microbiome analysis revealed the increased potential for glutathione metabolism that have been linked to platinum resistance.Citation44,Citation45 These features may contribute to the poor clinical prognosis and response to ACT observed in patients with the MS3 subtype. Our results emphasize the potential of the Msubtype as a potential application with a future opportunity for identification of correlated microbiotas to facilitate clinical decision-making. In addition, our findings suggest the possibility that selective bacterial eradication or manipulation might improve therapy efficacy. For instance, the oral administration of Bifidobacterium has the potential to enhance immunotherapy in melanoma.Citation46 In addition, transplanting the gut microbiota of chemotherapy-responsive mice can reverse chemotherapy resistance.Citation47
This study used a large independent cohort of microbiome samples as well as detailed clinical and therapy data to identify and elucidate the molecular characteristics and clinical significance of microbial subtypes. These findings were validated in multiple independent cohorts. Despite the strengths of this study, there were certain limitations. First, the sequencing resolution of the 16s rRNA was limited and did not allow for precise microbiota identification. Second, we observed a significant enrichment of butyric acid-producing genera in the MS1 subtype, suggesting a potential increase in butyric acid. However, this finding needs to be further validated by metabolite detection. Third, owing to the lack of research on intratumoral microbiota in GC and small sample sizes, as well as the lack of prognostic information, we were unable to identify an appropriate cohort of microbiome samples for external validation. Further research on the microbial function and interaction, together with improved sequencing techniques, are required to validate and expand upon these findings.
In conclusion, our study provides a comprehensive microbial perspective that enhances our understanding of GC. First, we identified the microbiota associated with GC prognosis and ACT efficacy. Notably, we discovered that the intratumoral microbiome pattern, referred to the Msubtype, is associated with OS as well as chemotherapy and immunotherapy efficacy in GC. Importantly, our study provides an integrated and extensive microbial resource for the further exploration of the pathogenesis of GC.
Material and methods
Clinical specimens of GC
GC samples were collected from the Peking University Cancer Hospital in China. None of the enrolled patients had received therapy before surgery, and all were followed up for five years after resection. After radical gastrectomy, resected tumor samples and NATs (>5 cm from the tumor edge) were obtained and snap-frozen in liquid nitrogen in less than 30 min after resection. Then the tissues were stored at − 80°C. To ensure the quality of tissues, routine histological evaluation was performed for each sample. All of the samples were obtained, stored, and transported in a sterile environment. Clinical information was collected for the patients, including age, sex, pathology, grade, location, vascular invasion, TNM stage, Lauren subtype, molecular subtype, and ACT information (Table S1). Patients who received ACT were defined as those who received at least one cycle of 5-fluorouracil-based ACT.Citation48 OS was defined as the time interval between surgery and death. This study was approved by the Research Ethics Committee of Peking University Cancer Hospital (2019KT111), and written informed consent was obtained from all patients.
dMMR status determination and EBV in situ hybridization
Immunohistochemistry (IHC) for MLH1, PMS2, MSH2, and MSH6 was performed as previously described to determine the deficient mismatch repair (dMMR) status.Citation49 The monoclonal antibodies used specific for MLH1 (Clone ES05, DAKO), MSH2 (Clone FE11, DAKO), PMS2 (Clone EP51, DAKO), and MSH6 (EP49, DAKO). Tumors with a negative stain for any of these markers were considered dMMR, and tumors with positive staining for all four were defined as proficient mismatch repair (pMMR).
Chromogenic in situ hybridization with EBV-encoded small RNA (EBER) was performed to detect EBV infection using fluorescein-labeled oligonucleotide probes (INFORMEBER Probe; Ventana). Specimens displaying EBER nuclear expression in > 20% of the tumor cells were considered EBER-positive.
Primary GC dataset collection
We collected five transcriptome datasets, TCGA-STAD (T = 375), GSE84437 (T = 433),Citation50 GSE62254/ACRG (T = 300),Citation14 GSE26942 (T = 217),Citation51 and GSE13861 (T = 90),Citation52 from the Gene Expression Omnibus (GEO) and The Cancer Genome Atlas (TCGA) databases; 1415 samples with clinical data were included in the study. The three cohorts ACRG, GSE26942, and GSE13861 contained information on ACT. In addition, we collected a transcriptome dataset of patients who responded to neoadjuvant chemotherapy (NAC) from our previous study.Citation53 Gene expression data was log2 transformed if necessary.
Patients with GC who received immunotherapy dataset collection
Two transcriptomic datasets with immunotherapy response information were included in our study: patients with GC (T = 45) treated with anti-PD1 therapyCitation54 and patients with GC (T = 24) treated with anti-PD1/PDL1/CTLA4 therapy.Citation55 Gene expression data in all datasets was log2- and z-score-transformed, if necessary.
16S rRNA gene sequencing
A total of 251 GC tissue pairs were selected for microbiological analysis. Total genomic DNA samples were extracted using the OMEGA Soil DNA Kit (M5635–02) (OmegaBio-Tek, Norcross, GA, USA), following the manufacturer’s instructions, and stored at −20°C for further analysis. The quantity and quality of extracted DNA were measured using a NanoDrop NC2000 spectrophotometer (Thermo Fisher Scientific, Waltham, MA, USA) and agarose gel electrophoresis, respectively. PCR amplification of the bacterial 16S rRNA gene V3-V4 region was performed using the forward primer 338F (5’-ACTCCTACGGGAGGCAGCA-3’) and the reverse primer 806 R (5’-GGACTACHVGGGTWTCTAAT-3’). Sample-specific 7-bp barcodes were incorporated into the primers for multiplex sequencing. The PCR components contained 5 μl of buffer (5×), 0.25 μl of Fast pfu DNA Polymerase (5 U/μl), 2 μl (2.5 mM) of dNTPs, 1 μl (10 uM) of the forward and reverse primers, 1 μl of DNA Template, and 14.75 μl of ddH2O. Thermal cycling consisted of initial denaturation at 98°C for 5 min, followed by 25 cycles of denaturation at 98°C for 30 s, annealing at 52°C for 30 s, and extension at 72°C for 45 s, with a final extension cycle for 5 min at 72°C. The PCR amplicons were purified using Vazyme VAHTSTM DNA Clean Beads (Vazyme, Nanjing, China) and quantified using a Quant-iT PicoGreen dsDNA Assay Kit (Invitrogen, Carlsbad, CA, USA). After the individual quantification step, the amplicons were pooled in equal amounts, and pair-end 2 × 250bp sequencing was performed using the Illumina NovaSeq platform with the NovaSeq 6000 SP Reagent Kit (500 cycles) at Shanghai Personal Biotechnology Co., Ltd (Shanghai, China).
16S rRNA data analysis
Sequence data analyses were performed using QIIME2.Citation56 Taxonomic information for each ASV is obtained by aligning it with reference sequences in the Greengenes database. Alpha diversity indices were calculated using the ASV table in QIIME2. Beta diversity analysis was performed to assess the structural variation of microbial communities across samples using Bray-Curtis metrics and was analyzed via principal coordinate analysis (PCoA). The significance of differential microbiota among groups was assessed using PERMANOVA. SparCCCitation57 was used to compute the co-occurrence between microbiota. The network was visualized and analyzed using the iGraph package. To measure and identify the hub microbiota in the network, Kleinberg’s hub centrality scores were calculated for each network and scaled across all networks. O2PLS modeling was then employed to delineate the relationships between microbiota and immune-related genes; the main immune-related genes and core microbial genera with strong correlations were selected. Microbial functions were predicted using PICRUSt2Citation58 in the Kyoto Encyclopedia of Genes and Genomes (KEGG, https://www.kegg.jp/) database.
RNA extraction
A total of 94 tumors were analyzed using RNA-seq. Total RNA was isolated using Trizol reagent (Invitrogen Life Technologies), and the concentration, quality, and integrity of RNA were determined using a NanoDrop spectrophotometer (Thermo Scientific). Three micrograms of RNA were used as the input material for RNA sample preparation. Sequencing libraries were generated by first purifying mRNA from the total RNA using poly T oligo-attached magnetic beads. Fragmentation was performed using divalent cations at elevated temperatures in the Illumina proprietary fragmentation buffer. Then, first-strand cDNA was synthesized using random oligonucleotides and SuperScript II reverse transcriptase. Second-strand cDNA synthesis was subsequently performed using DNA polymerase I and RNase H. The remaining overhangs were converted into blunt ends via exonuclease/polymerase activities, and the enzymes were removed. After adenylation of the 3′ ends of the DNA fragments, Illumina PE adapter oligonucleotides were ligated to prepare them for hybridization. To select 400–500 bp cDNA fragments, library fragments were purified using the AMPure XP system (Beckman Coulter, Beverly, CA, USA). DNA fragments with ligated adaptor molecules at both ends were selectively enriched using the Illumina PCR Primer Cocktail in a 15 cycle PCR reaction. The products were purified (AMPure XP system) and quantified using an Agilent High-Sensitivity DNA Assay on a Bioanalyzer 2100 system (Agilent Technologies). The library was sequenced using a NovaSeq 6000 platform (Illumina).
RNA-Seq data analysis
Quality control and raw data processing were performed using FastQC (v0.11.9) and Cutadapt (v1.15).Citation59 The reads were aligned to the reference genome (hg38) using HISAT2 (v2.0.5).Citation60 HTSeq (0.9.1)Citation61 was applied to obtain FPKM. The FPKM values were transformed into TPM values. Gene expression was then log2-transformed. The EBV reference genome was downloaded from https://www.ncbi.nlm.nih.gov/datasets/genome/GCA_027943465.1/and the EBV genome was mapped using the STAR software (v2.7.9a),Citation62 generating the read counts for the EBV genome. The EBV load of each sample was defined as the total read counts of the EBV genome.
Deconvolution of GC transcriptome data using the MCP-counter
To characterize the immune characteristics of the GC transcriptome, the abundance of eight immune cells (T cells, CD8+ T cells, cytotoxic lymphocytes, NKs, B cells, monocytes, dendritic cells, and neutrophils) and two stromal cells (endothelial cells and CAFs) were estimated using the MCP-counter.Citation63
Consensus clustering analysis
Microbial genera that were expressed in more than 60% of the patient samples were selected for clustering analysis. Consensus clustering was conducted using the ConsensusClusterPlus package with the Pearson correlation as the distance measure.Citation64 The consensus matrix for k = 3 showed clear separation between the subtypes. In addition, the consensus CDF and delta plots showed that the relative change in the area under the cumulative distribution function curve increased the most from two to three subtypes, whereas the others exhibited no significant increase. Furthermore, the three subtypes were significantly associated with patient survival (Figure S3). Taken together, the Msubtypes were defined using consensus clustering with k = 3. Subtype-specific genera were defined as those that were highly enriched in a specific subtype (Adj p-value <0.05, Log2 FC > 2, and AUC > 0.7).
Functional enrichment analysis
To further analyze biological characteristics of different subtypes, we performed gene set enrichment analysis (GSEA) to identify enriched pathways from the KEGG, Biocarta, Reactome, PID, Wikipathways, and Hallmarker databases associated with the Msubtypes with at least 10 overlapping genes. GSEA was performed on the log2 (FC) values from the differential abundance analysis between Msubtypes and others in the transcriptome. Pathways with an FDR < 0.1 and a normalized enrichment score (NES) > 0 were considered significantly enriched.
GC subtype classification and prediction evaluation
We used the support vector machine (SVM)Citation65 algorithm to divide samples from the published transcriptome cohorts into subtypes. The SVM algorithm was performed using e1071 package. A transcriptome dataset was used as the training set to train the model, and 10-fold cross-validation was performed to evaluate the accuracy of the model.
Subgroup correlation analysis of independent cohorts
An unsupervised subclass mapping method (SubMap) (GenePattern module “SubMap”) (https://cloud.genepattern.org) was used to identify similar subgroups between independent cohorts despite their clinical differences.Citation66
Quantification and statistical analysis
Statistical analyses were performed using R (v. 4.2.1) unless otherwise stated. Standard statistical tests were used to analyze data from different groups. For categorical variables, Fisher’s exact test was applied, while continuous variables were evaluated via Wilcoxon rank-sum test to compare differences between two groups. The Spearman’s rank correlation coefficient was used to evaluate the correlations between two continuous variables. For survival analysis, the Kaplan-Meier method and log-rank test were used to compare survival distribution. Additionally, Cox analysis was applied to evaluate the correlation between survival and different variables and to calculate hazard ratio (HR) values. All statistical tests were two-sided, and p < 0.05 was considered statistically significant.
Author contributions
J.F.J., H.J.W. and X.F.X. conceived the study. X.F.X. contributed to biospecimen processing. G.J.W., H.J.W., X.J. and W.J.J. analyzed the data. G.J.W., X.F.X., X.J. and L.M. contributed to collection of clinical samples and data and critical review of data. G.J.W., H.J.W., and H.J.W. drafted the manuscript. J.F.J., H.J.W., H.J.W., X.F.X., X.J. and G.J.W. critically revised the manuscript. J.F.J., H.J.W., H.J.W., X.J., X.F.X., T.W., Y.Z., W.J.J., L.M. and G.J.W. supervised the study. J.F.J., T.W., H.J.W., and Y.Z., obtained funding. All authors read and approved the final manuscript.
Supplemental Material
Download Zip (1.8 MB)Disclosure statement
No potential conflict of interest was reported by the author(s).
Data availability statement
The sequencing data generated by this study is available through the Genome Sequence Archive for Human (GSA-Human): HRA005570 (16s rRNA, https://ngdc.cncb.ac.cn/gsa-human/submit/hra/subHRA007973/finishedOverview.) and HRA005478 (RNAseq, https://ngdc.cncb.ac.cn/gsa-human/submit/hra/subHRA007857/finishedOverview).
Supplementary material
Supplemental data for this article can be accessed online at https://doi.org/10.1080/19490976.2024.2369336
Additional information
Funding
References
- Wong-Rolle A, Wei HK, Zhao C, Jin C. Unexpected guests in the tumor microenvironment: microbiome in cancer. Protein Cell. 2021;12(5):426–17. doi:10.1007/s13238-020-00813-8.
- Cogdill AP, Gaudreau PO, Arora R, Gopalakrishnan V, Wargo JA. The impact of intratumoral and gastrointestinal microbiota on systemic cancer therapy. Trends Immunol. 2018;39(11):900–920. doi:10.1016/j.it.2018.09.007.
- Ferrari V, Rescigno M. The intratumoral microbiota: friend or foe? Trends Cancer. 2023;9(6):472–479. doi:10.1016/j.trecan.2023.03.005.
- Lertpiriyapong K, Whary MT, Muthupalani S, Lofgren JL, Gamazon ER, Feng Y, Ge Z, Wang TC, Fox JG. Gastric colonisation with a restricted commensal microbiota replicates the promotion of neoplastic lesions by diverse intestinal microbiota in the Helicobacter pylori INS-GAS mouse model of gastric carcinogenesis. Gut. 2014;63(1):54–63. doi:10.1136/gutjnl-2013-305178.
- Ling Z, Shao L, Liu X, Cheng Y, Yan C, Mei Y, Ji F, Liu X. Regulatory t cells and plasmacytoid dendritic cells within the tumor microenvironment in gastric cancer are correlated with gastric microbiota dysbiosis: A preliminary study. Front Immunol. 2019;10:533. doi:10.3389/fimmu.2019.00533.
- Ahmetlić F, Riedel T, Hömberg N, Bauer V, Trautwein N, Geishauser A, Sparwasser T, Stevanović S, Röcken M, Mocikat R. Regulatory t cells in an endogenous mouse lymphoma recognize specific antigen peptides and contribute to immune escape. Cancer Immunol Res. 2019;7(4):600–608. doi:10.1158/2326-6066.Cir-18-0419.
- Li Q, Wu W, Gong D, Shang R, Wang J, Yu H. Propionibacterium acnes overabundance in gastric cancer promote M2 polarization of macrophages via a TLR4/PI3K/Akt signaling. Gastric Cancer. 2021;24(6):1242–1253. doi:10.1007/s10120-021-01202-8.
- Hwang CH, Lee NK, Paik HD. The anti-cancer potential of heat-killed lactobacillus brevis KU15176 upon AGS cell lines through intrinsic apoptosis pathway. Int J Mol Sci. 2022;23(8):4073. doi:10.3390/ijms23084073.
- Bik EM, Eckburg PB, Gill SR, Nelson KE, Purdom EA, Francois F, Perez-Perez G, Blaser MJ, Relman DA. Molecular analysis of the bacterial microbiota in the human stomach. Proc Natl Acad Sci USA. 2006;103:732–737. doi:10.1073/pnas.0506655103.
- Yu G, Hu N, Wang L, Wang C, Han XY, Humphry M, Ravel J, Abnet CC, Taylor PR, Goldstein AM. Gastric microbiota features associated with cancer risk factors and clinical outcomes: A pilot study in gastric cardia cancer patients from Shanxi, China. Int J Cancer. 2017;141(1):45–51. doi:10.1002/ijc.30700.
- Aviles-Jimenez F, Vazquez-Jimenez F, Medrano-Guzman R, Mantilla A, Torres J. Stomach microbiota composition varies between patients with non-atrophic gastritis and patients with intestinal type of gastric cancer. Sci Rep. 2014;4(1):4202. doi:10.1038/srep04202.
- Liu X, Shao L, Liu X, Ji F, Mei Y, Cheng Y, Liu F, Yan C, Li L, Ling Z. Alterations of gastric mucosal microbiota across different stomach microhabitats in a cohort of 276 patients with gastric cancer. EBioMedicine. 2019;40:336–348. doi:10.1016/j.ebiom.2018.12.034.
- Comprehensive molecular characterization of gastric adenocarcinoma. Nature. 2014;513(7517):202–209. doi:10.1038/nature13480.
- Cristescu R, Lee J, Nebozhyn M, Kim KM, Ting JC, Wong SS, Liu J, Yue YG, Wang J, Yu K, et al. Molecular analysis of gastric cancer identifies subtypes associated with distinct clinical outcomes. Nat Med. 2015;21(5):449–456. doi:10.1038/nm.3850.
- Ge S, Xia X, Ding C, Zhen B, Zhou Q, Feng J, Yuan J, Chen R, Li Y, Ge Z, et al. A proteomic landscape of diffuse-type gastric cancer. Nat Commun. 2018;9(1):1012. doi:10.1038/s41467-018-03121-2.
- Dai D, Yang Y, Yu J, Dang T, Qin W, Teng L, Ye J, Jiang H. Interactions between gastric microbiota and metabolites in gastric cancer. Cell Death Disease. 2021;12(12):1104. doi:10.1038/s41419-021-04396-y.
- Coker OO, Dai Z, Nie Y, Zhao G, Cao L, Nakatsu G, Wu WK, Wong SH, Chen Z, Sung JJY, et al. Mucosal microbiome dysbiosis in gastric carcinogenesis. Gut. 2018;67(6):1024–1032. doi:10.1136/gutjnl-2017-314281.
- Xia W, Liu L, Shi N, Zhang C, Tang A, He G. Epstein Barr virus infection in tree shrews alters the composition of gut microbiota and metabolome profile. Virol J. 2023;20(1):177. doi:10.1186/s12985-023-02147-3.
- Neuzillet C, Marchais M, Vacher S, Hilmi M, Schnitzler A, Meseure D, Leclere R, Lecerf C, Dubot C, Jeannot E, et al. Prognostic value of intratumoral Fusobacterium nucleatum and association with immune-related gene expression in oral squamous cell carcinoma patients. Sci Rep. 2021;11(1):7870. doi:10.1038/s41598-021-86816-9.
- Zhu G, Su H, Johnson CH, Khan SA, Kluger H, Lu L. Intratumour microbiome associated with the infiltration of cytotoxic CD8+ T cells and patient survival in cutaneous melanoma. Eur J Cancer. 2021;151:25–34. doi:10.1016/j.ejca.2021.03.053.
- Ma J, Gnanasekar A, Lee A, Li WT, Haas M, Wang-Rodriguez J, Chang EY, Rajasekaran M, Ongkeko WM. Influence of intratumor microbiome on clinical outcome and immune processes in prostate cancer. Cancers (Basel). 2020;12(9). doi:10.3390/cancers12092524.
- Aoyama T, Yoshikawa T, Watanabe T, Hayashi T, Ogata T, Cho H, Tsuburaya A. Macroscopic tumor size as an independent prognostic factor for stage II/III gastric cancer patients who underwent D2 gastrectomy followed by adjuvant chemotherapy with S-1. Gastric Cancer. 2011;14(3):274–278. doi:10.1007/s10120-011-0038-0.
- Brandt S, Kwok T, Hartig R, König W, Backert S. NF-κB activation and potentiation of proinflammatory responses by the Helicobacter pylori CagA protein. Proc Natl Acad Sci USA. 2005;102(26):9300–9305. doi:10.1073/pnas.0409873102.
- Yin Y, Grabowska AM, Clarke PA, Whelband E, Robinson K, Argent RH, Tobias A, Kumari R, Atherton JC, Watson SA. Helicobacter pylori potentiates epithelial: mesenchymal transition in gastric cancer: links to soluble HB-EGF, gastrin and matrix metalloproteinase-7. Gut. 2010;59(8):1037–1045. doi:10.1136/gut.2009.199794.
- Zhu X, Li K, Liu G, Wu R, Zhang Y, Wang S, Xu M, Lu L, Li P. Microbial metabolite butyrate promotes anti-PD-1 antitumor efficacy by modulating T cell receptor signaling of cytotoxic CD8 T cell. Gut Microbes. 2023;15(2):2249143. doi:10.1080/19490976.2023.2249143.
- He Y, Fu L, Li Y, Wang W, Gong M, Zhang J, Dong X, Huang J, Wang Q, Mackay CR, et al. Gut microbial metabolites facilitate anticancer therapy efficacy by modulating cytotoxic CD8+ T cell immunity. Cell Metab. 2021;33(5):988–1000.e1007. doi:10.1016/j.cmet.2021.03.002.
- Ma J, Huang L, Hu D, Zeng S, Han Y, Shen H. The role of the tumor microbe microenvironment in the tumor immune microenvironment: bystander, activator, or inhibitor? J Exp Clin Cancer Res. 2021;40(1):327. doi:10.1186/s13046-021-02128-w.
- Wan M, Ding Y, Li Z, Wang X, Xu M. Metabolic manipulation of the tumour immune microenvironment. Immunology. 2022;165(3):290–300. doi:10.1111/imm.13444.
- Dorrestein PC, Mazmanian SK, Knight R. Finding the missing links among metabolites, microbes, and the host. Immunity. 2014;40(6):824–832. doi:10.1016/j.immuni.2014.05.015.
- Navashenaq JG, Shabgah AG, Banach M, Jamialahmadi T, Penson PE, Johnston TP, Sahebkar A. The interaction of Helicobacter pylori with cancer immunomodulatory stromal cells: New insight into gastric cancer pathogenesis. Semin Cancer Biol. 2022;86:951–959. doi:10.1016/j.semcancer.2021.09.014.
- Teng H, Wang Y, Sui X, Fan J, Li S, Lei X, Shi C, Sun W, Song M, Wang H, et al. Gut microbiota-mediated nucleotide synthesis attenuates the response to neoadjuvant chemoradiotherapy in rectal cancer. Cancer Cell. 2023;41(1):124–138.e126. doi:10.1016/j.ccell.2022.11.013.
- Yi Y, Shen L, Shi W, Xia F, Zhang H, Wang Y, Zhang J, Wang Y, Sun X, Zhang Z, et al. Gut Microbiome Components Predict Response to Neoadjuvant Chemoradiotherapy in Patients with Locally Advanced Rectal Cancer: A Prospective, Longitudinal Study. Clin Cancer Res. 2021;27:1329–1340. doi:10.1158/1078-0432.Ccr-20-3445.
- Qiu B, Xi Y, Liu F, Li Y, Xie X, Guo J, Guo S, Wu Y, Wu L, Liang T, et al. Gut Microbiome Is Associated with the Response to Chemoradiotherapy in Patients with Non-small Cell Lung Cancer. Int J Radiat Oncol Biol Phys. 2023;115:407–418. doi:10.1016/j.ijrobp.2022.07.032.
- Tang C, Yang L, Jiang X, Xu C, Wang M, Wang Q, Zhou Z, Xiang Z, Cui H. Antibiotic drug tigecycline inhibited cell proliferation and induced autophagy in gastric cancer cells. Biochem Biophys Res Commun. 2014;446(1):105–112. doi:10.1016/j.bbrc.2014.02.043.
- Imai H, Saijo K, Komine K, Ueta R, Numakura R, Wakayama S, Umegaki S, Hiraide S, Kawamura Y, Kasahara Y, et al. Antibiotic Treatment Improves the Efficacy of Oxaliplatin-Based Therapy as First-Line Chemotherapy for Patients with Advanced Gastric Cancer: A Retrospective Study. Cancer Manag Res. 2022;14:1259–1266. doi:10.2147/cmar.S353432.
- Han Z, Cheng S, Dai D, Kou Y, Zhang X, Li F, Yin X, Ji J, Zhang Z, Wang X, et al. The gut microbiome affects response of treatments in HER2-negative advanced gastric cancer. Clin Transl Med. 2023;13(7):e1312. doi:10.1002/ctm2.1312.
- Peng Z, Cheng S, Kou Y, Wang Z, Jin R, Hu H, Zhang X, Gong JF, Li J, Lu M, et al. The Gut Microbiome Is Associated with Clinical Response to Anti–PD-1/PD-L1 Immunotherapy in Gastrointestinal Cancer. Cancer Immunol Res. 2020;8(10):1251–1261. doi:10.1158/2326-6066.Cir-19-1014.
- Xie Y, Xie F, Zhou X, Zhang L, Yang B, Huang J, Wang F, Yan H, Zeng L, Zhang L, et al. Microbiota in Tumors: From Understanding to Application. Adv Sci (Weinh). 2022;9(21):e2200470. doi:10.1002/advs.202200470.
- Yang L, Li A, Wang Y, Zhang Y. Intratumoral microbiota: roles in cancer initiation, development and therapeutic efficacy. Signal Transduct Target Ther. 2023;8(1):35. doi:10.1038/s41392-022-01304-4.
- Niccolai E, Di Pilato V, Nannini G, Baldi S, Russo E, Zucchi E, Martinelli I, Menicatti M, Bartolucci G, Mandrioli J, et al. The Gut Microbiota-Immunity Axis in ALS: A Role in Deciphering Disease Heterogeneity? Biomedicines. 2021;9(7):753. doi:10.3390/biomedicines9070753.
- Baldi S, Menicatti M, Nannini G, Niccolai E, Russo E, Ricci F, Pallecchi M, Romano F, Pedone M, Poli G, et al. Free Fatty Acids Signature in Human Intestinal Disorders: Significant Association between Butyric Acid and Celiac Disease. Nutrients. 2021;13(3):742. doi:10.3390/nu13030742.
- Zhang J, Hu Z, Horta CA, Yang J. Regulation of epithelial-mesenchymal transition by tumor microenvironmental signals and its implication in cancer therapeutics. Semin Cancer Biol. 2023;88:46–66. doi:10.1016/j.semcancer.2022.12.002.
- Lambert AW, Weinberg RA. Linking EMT programmes to normal and neoplastic epithelial stem cells. Nat Rev Cancer. 2021;21(5):325–338. doi:10.1038/s41568-021-00332-6.
- Shi Y, Wang Y, Huang W, Wang Y, Wang R, Yuan Y. Integration of metabolomics and transcriptomics to reveal metabolic characteristics and key targets associated with cisplatin resistance in nonsmall cell lung cancer. J Proteome Res. 2019;18(9):3259–3267. doi:10.1021/acs.jproteome.9b00209.
- Zheng ZG, Xu H, Suo SS, Xu XL, Ni MW, Gu LH, Chen W, Wang LY, Zhao Y, Tian B, et al. The Essential Role of H19 Contributing to Cisplatin Resistance by Regulating Glutathione Metabolism in High-Grade Serous Ovarian Cancer. Sci Rep. 2016;6(1):26093. doi:10.1038/srep26093.
- Sivan A, Corrales L, Hubert N, Williams JB, Aquino-Michaels K, Earley ZM, Benyamin FW, Lei YM, Jabri B, Alegre ML, et al. Commensal Bifidobacterium promotes antitumor immunity and facilitates anti–PD-L1 efficacy. Science. 2015;350(6264):1084–1089. doi:10.1126/science.aac4255.
- Tintelnot J, Xu Y, Lesker TR, Schönlein M, Konczalla L, Giannou AD, Pelczar P, Kylies D, Puelles VG, Bielecka AA, et al. Microbiota-derived 3-IAA influences chemotherapy efficacy in pancreatic cancer. Nature. 2023;615(7950):168–174. doi:10.1038/s41586-023-05728-y.
- Wang JT, Li H, Zhang H, Chen YF, Cao YF, Li RC, Lin C, Wei YC, Xiang XN, Fang HJ, et al. Intratumoral IL17-producing cells infiltration correlate with antitumor immune contexture and improved response to adjuvant chemotherapy in gastric cancer. Ann Oncol. 2019;30(2):266–273. doi:10.1093/annonc/mdy505.
- van der Sluis K, van Sandick Jw, van Dieren Jm, Vollebergh MA, Grootscholten C, van den Berg Jg, Snaebjornsson P, Hartemink KJ, Veenhof A, Chalabi M, et al. The clinical impact of testing for biomarkers in gastric cancer patients: a real-world cohort. Histopathology. 2023;82(6):826–836. doi:10.1111/his.14869.
- Yoon SJ, Park J, Shin Y, Choi Y, Park SW, Kang SG, Son HY, Huh YM. Deconvolution of diffuse gastric cancer and the suppression of CD34 on the BALB/c nude mice model. BMC Cancer. 2020;20(1):314. doi:10.1186/s12885-020-06814-4.
- Oh SC, Sohn BH, Cheong JH, Kim SB, Lee JE, Park KC, Lee SH, Park JL, Park YY, Lee HS, et al. Clinical and genomic landscape of gastric cancer with a mesenchymal phenotype. Nat Commun. 2018;9(1):1777. doi:10.1038/s41467-018-04179-8.
- Lim JY, Yoon SO, Hong SW, Kim JW, Choi SH, Cho JY. Thioredoxin and thioredoxin-interacting protein as prognostic markers for gastric cancer recurrence. World J Gastroenterol. 2012;18(39):5581–5588. doi:10.3748/wjg.v18.i39.5581.
- Li Z, Gao X, Peng X, May Chen MJ, Li Z, Wei B, Wen X, Wei B, Dong Y, Bu Z, et al. Multi-omics characterization of molecular features of gastric cancer correlated with response to neoadjuvant chemotherapy. Sci Adv. 2020;6(9):eaay4211. doi:10.1126/sciadv.aay4211.
- Kim ST, Cristescu R, Bass AJ, Kim KM, Odegaard JI, Kim K, Liu XQ, Sher X, Jung H, Lee M, et al. Comprehensive molecular characterization of clinical responses to PD-1 inhibition in metastatic gastric cancer. Nat Med. 2018;24(9):1449–1458. doi:10.1038/s41591-018-0101-z.
- Lu Z, Chen H, Jiao X, Zhou W, Han W, Li S, Liu C, Gong J, Li J, Zhang X, et al. Prediction of immune checkpoint inhibition with immune oncology-related gene expression in gastrointestinal cancer using a machine learning classifier. J Immunother Cancer. 2020;8(2):8. doi:10.1136/jitc-2020-000631.
- Bolyen E, Rideout JR, Dillon MR, Bokulich NA, Abnet CC, Al-Ghalith GA, Alexander H, Alm EJ, Arumugam M, Asnicar F, et al. Reproducible, interactive, scalable and extensible microbiome data science using QIIME 2. Nat Biotechnol. 2019;37(8):852–857. doi:10.1038/s41587-019-0209-9.
- Friedman J, Alm EJ, von Mering C. Inferring correlation networks from genomic survey data. PLOS Comput Biol. 2012;8(9):e1002687. doi:10.1371/journal.pcbi.1002687.
- Langille MG, Zaneveld J, Caporaso JG, McDonald D, Knights D, Reyes JA, Clemente JC, Burkepile DE, Vega Thurber RL, Knight R, et al. Predictive functional profiling of microbial communities using 16S rRNA marker gene sequences. Nat Biotechnol. 2013;31(9):814–821. doi:10.1038/nbt.2676.
- MJEj M. Cutadapt removes adapter sequences from high-throughput sequencing reads2011. EMBnet Journal. 2011;17(1):10–12. doi:10.14806/ej.17.1.200.
- Kim D, Paggi JM, Park C, Bennett C, Salzberg SL. Graph-based genome alignment and genotyping with HISAT2 and HISAT-genotype. Nat Biotechnol. 2019;37(8):907–915. doi:10.1038/s41587-019-0201-4.
- Anders S, Pyl PT, Huber W. HTSeq—a Python framework to work with high-throughput sequencing data. Bioinformatics. 2015;31(2):166–169. doi:10.1093/bioinformatics/btu638.
- Dobin A, Davis CA, Schlesinger F, Drenkow J, Zaleski C, Jha S, Batut P, Chaisson M, Gingeras TR. STAR: ultrafast universal RNA-seq aligner. Bioinformatics. 2013;29(1):15–21. doi:10.1093/bioinformatics/bts635.
- Becht E, Giraldo NA, Lacroix L, Buttard B, Elarouci N, Petitprez F, Selves J, Laurent-Puig P, Sautès-Fridman C, Fridman WH, et al. Estimating the population abundance of tissue-infiltrating immune and stromal cell populations using gene expression. Genome Biol. 2016;17(1):218. doi:10.1186/s13059-016-1070-5.
- Wilkerson MD, Hayes DN. ConsensusClusterPlus: a class discovery tool with confidence assessments and item tracking. Bioinformatics. 2010;26(12):1572–1573. doi:10.1093/bioinformatics/btq170.
- Guyon I, Weston J, Barnhill S, VJMl V. Gene selection for cancer classification using support vector machines2002. Mach Learn. 2002;46(1/3):389–422. doi:10.1023/A:1012487302797.
- Hoshida Y, Brunet JP, Tamayo P, Golub TR, Mesirov JP, Hofmann O. Subclass mapping: identifying common subtypes in independent disease data sets. PLOS One. 2007;2(11):e1195. doi:10.1371/journal.pone.0001195.