ABSTRACT
Introduction
Oral hygiene instruction (OHI) is essential during periodontitis treatment. Various OHI approaches have been explored, including mobile apps.
Objective
To evaluate the mobile app-based OHI’s effect on periodontitis management by analyzing clinical parameters and subgingival microbiota.
Methods
Forty-four periodontitis patients were randomly assigned into two groups. The test group (n = 22) received scaling and root planing (SRP), OHI, and mobile app-based OHI, whereas the control group (n = 22) received SRP and OHI. Full mouth plaque score (FMPS), bleeding on probing (BOP) and probing pocket depth at the sampling sites (site-PPD) were assessed at baseline, one- and three-month visits. The 16S rRNA next-generation sequencing (NGS) was used to analyze subgingival plaque samples.
Results
Significant reduction in FMPS, BOP, and site-PPD at one- and three-month visits compared to baseline (p < 0.001) with no significant differences across groups (p > 0.05). In test groups, intra-group analysis showed better improvement in BOP and site-PPD (p < 0.05) than control. The diversity and composition of subgingival microbiota did not differ between groups or timepoints (p > 0.05).
Conclusions
Mobile app-based OHI showed no superior effects on improving clinical parameters and subgingival microbiota compared to conventional OHI. Further investigation into its long-term impact on periodontitis treatment is needed.
Introduction
Periodontitis is a chronic multifactorial inflammatory disease caused by dysbiotic plaque biofilms and characterized by progressive destruction of the tooth-supporting apparatus, leading to tooth loss if left untreated [Citation1,Citation2]. It affects 14% of adults worldwide (1.1 billion cases), comprising a significant global health issue [Citation3,Citation4]. The primary features of periodontitis include the loss of periodontal tissue support, manifested through clinical attachment loss (CAL) and radiographically assessed alveolar bone loss, the presence of periodontal pocketing, and gingival bleeding [Citation1,Citation2].
Periodontal treatment aims to restore periodontal health and involves a series of steps aimed at holistically managing patients and their periodontitis [Citation2,Citation5]. The initial phase of treatments is a cause-related therapy, including patient motivation to control plaque biofilm and risk factors, oral hygiene instructions (OHI), and professional mechanical instrumentation employing non-surgical treatment techniques [Citation2,Citation6]. Poor oral hygiene and compliance are significant risk factors for periodontitis, making OHI essential beyond professional treatment [Citation5,Citation7,Citation8].
Over the years, several approaches to OHI practices have been investigated, and using mobile apps is one of them. Health-related mobile apps (mHealth) have shown promise in influencing habits and behaviors positively [Citation9]. Recent systematic reviews suggest that mHealth can be an effective adjunct in managing gingivitis, acquiring oral health knowledge and improving oral hygiene [Citation10]. However, evidence of their effectiveness in periodontitis treatment outcomes is minimal, as most studies have focused on orthodontic patients without periodontitis. Thus, it is important to investigate the effectiveness of mobile app-based OHI in these patients and evaluate its impact on periodontal clinical parameters and the microbiota subgingival.
The subgingival microbiota plays a crucial role in the pathogenesis of periodontitis [Citation11,Citation12]. Dysbiosis in the subgingival biofilm can initiate chronic inflammation of the gingiva, potentially progressing to periodontitis [Citation13,Citation14]. Advances in DNA sequencing analysis have allowed for a more comprehensive study of the subgingival microbial community and have shown that periodontal treatment causes a widespread microbial shift in subgingival plaque, affecting the taxonomic composition (disease- and health-associated taxa) and microorganism interactions [Citation15–19]. Thus, assessing changes in the subgingival microbiota following OHI interventions could provide valuable insights.
Given these considerations, the primary objective of this randomized controlled trial is to assess the effectiveness of a mobile app-based OHI in periodontitis patients compared to conventional OHI alone. We hypothesize that the interventions will differ in improving periodontal clinical parameters and subgingival microbiota. This study aims to investigate changes in clinical parameters such as full mouth plaque score (FMPS), bleeding on probing (BOP), and probing depth, as well as subgingival microbiota diversity and composition following the intervention. This study intends to promote evidence-based strategies for managing periodontitis and maintaining good oral hygiene by comprehending the effect of mobile app-based OHI on clinical and microbiological outcomes.
Materials and methods
Study design and participant selection
This was a two-armed, parallel-group, double-blinded randomized controlled trial (RCT), with patients returning at one month and three months post-treatment. The study protocol was approved by the Dental Research Ethics Committee, Faculty of Dentistry, Universitas Indonesia, with letter number 01/Ethical Approval/FKGUI/I/2022 and protocol number 091241221. The trial was registered with registration number ISRCTN12409366 (https://doi.org/10.1186/ISRCTN12409366) and was conducted and reported in accordance with the Consolidated Standards of Reporting Trials (CONSORT) guidelines [Citation20].
The study population consisted of periodontitis patients who visited the Periodontics Clinic, Dental Teaching Hospital, Faculty of Dentistry, Universitas Indonesia, between September 2022 and December 2023. The study’s objectives, research flow, mobile app intervention, predicted advantages and disadvantages, and data confidentiality were all explained to participating patients. All study procedures began after the participant signed the consent form. The inclusion criteria were as follows: 1) female patients aged 25–60 years; 2) diagnosed with periodontitis (there is interdental CAL on at least two non-adjacent teeth or buccal or oral CAL ≥ 3 mm with pocketing >3 mm on at least two teeth) [Citation1,Citation21]; 3) had never received periodontal therapy during the previous six months; and 4) Android system-based smartphone users. The exclusion criteria were as follows: 1) systemic conditions that might affect the study (such as diabetes mellitus, cardiovascular diseases, blood disorders, etc.) [Citation22–25]; 2) currently taking medication from a health provider, especially for antibiotics, anticonvulsants, calcium channel blockers, and immunosuppressant drugs [Citation24,Citation26,Citation27]; 3) current smokers [Citation24,Citation28] and 4) pregnancy [Citation24,Citation29,Citation30]. All visits were carried out within the Periodontics Clinic, Dental Teaching Hospital, Faculty of Dentistry, Universitas Indonesia. Baseline data were gathered including age, education level, and periodontal clinical parameters ().
Table 1. Baseline Demographic and Periodontal Clinical Parameters.
Sample size calculations
The present study was designed to test continuous response variables in both independent control and test participants. The primary outcome for this study was the full mouth plaque score (FMPS) at the 1-month visit (1-month post-intervention). Secondary outcomes included FMPS at the 3-month visit, other periodontal clinical parameters (full mouth bleeding on probing [BOP] and probing pocket depth at the sampling site [site-PPD]) at the one- and three-month visits, as well as changes in subgingival microbiota diversity and composition at the same timepoints. The sample size was calculated using G*Power statistical software (version 3.1.9.7, Heinrich Heine Universität Düsseldorf, Germany) with an effect size of 0.89 from a previous study [Citation31], a significance level (α) of 0.05 and a power level of 0.8. This calculation indicated that each group needed at least 21 participants. To account for potential dropouts during the study period, we enrolled a total of 50 participants, with 25 in each group.
Clinical examinations and sampling site selection
All clinical data collections were carried out by four periodontology residents (M.R.P., M.M.P., V.H., and Y.S.). Inter-rater and intra-rater reliability among the four examiners was assessed using the intra-class correlation coefficient (ICC) for FMPS, BOP, and site-PPD. Prior to the main study, a calibration exercise involved the examiners assessing these measures with five participants. ICC values were 0.985 for FMPS, 0.999 for BOP, and 1 for site-PPD, indicating excellent agreement among the examiners for all measures.
At baseline, one- and three-month visits, clinical periodontal parameters, including FMPS: percentage of tooth sites revealing the presence of plaque [Citation32]; full mouth BOP: percentage of tooth sites with bleeding upon probing [Citation33]; and 6-point PPD (the distance from the gingival margin to the bottom of the pocket) charting [Citation34], were assessed using a periodontal probe (UNC-15, Hu-Friedy, Chicago, IL, USA) at six sites per tooth (mesiobuccal, buccal, distobuccal, mesiolingual, lingual, and distolingual), excluding third molars, with measurements rounded to the nearest millimeter. Following the assessment of PPD, the single site with the deepest PPD (PPD ≥ 5 mm on a tooth that was not deemed to have a hopeless prognosis [Citation35]) in each patient was selected as the sampling site, and then PPD, CAL, and BOP at those sampling sites were also recorded (referred to as site-PPD, site-CAL and site-BOP, respectively). The collected clinical data (FMPS, BOP, and PPD) were entered into the mobile apps via a back-end website by a data entry operator.
Randomization
Patients were randomized to one of the two treatment groups (test or control group) using simple randomization techniques (random number generator, based on odd or even numbers) by an independent researcher who was not involved in the clinical examination (A.K.R.). Each participant was assigned a unique identifier and subsequently categorized into either the test or control group depending on whether their identifier was odd or even. The treating clinician and the data entry operator were blinded to prevent observer bias.
Treatment and intervention
During the first visit (baseline), all participants received full mouth scaling and root planing (SRP), as well as conventional OHI (verbal OHI). Full-mouth SRP was performed in one session by four periodontology residents (M.R.P., M.M.P., V.H., and Y.S.) using ultrasonic instruments (Scaler UDS-J Woodpecker, Guilin Woodpecker Medical Instrument Co., Ltd., Guangxi, China). Scaling and root planing (SRP) was performed until no supra or subgingival plaque or calculus deposits were detectable by visual examination (direct observation) or by careful tactile examination of the root surface using a periodontal probe (UNC-15, Hu-Friedy, Chicago, IL, USA) [Citation34].
A mobile app-based OHI intervention was only implemented for participants who belonged to the test group. Participants in the test group were asked to download and install the app, which was available in the Google Play Store (Perio UI Care, Universitas Indonesia, Jakarta, Indonesia). Each participant then received a username and password that would be used to login to the app, and an independent researcher (A.K.R.) gave brief instructions and information on how to use the app. For each visit, clinical periodontal parameters, which were recorded, were displayed inside the app to ensure that the participants were aware of whether their periodontal condition had improved or deteriorated. Every day throughout the three-month intervention period, the participants receive push notifications as reminders and instructions to perform routine oral hygiene. These notifications contain detailed step-by-step instructions on proper tooth brushing technique and the use of complementary dental hygiene tools, such as interdental brushes and dental floss. The instructions are accompanied by visual aids, including sequential images illustrating the brushing technique, images of interdental brushes and dental floss, as well as visual demonstrations on their correct usage. Additionally, researchers can track whether participants have received notifications through the back-end website, allowing for monitoring of user engagement and follow-up if necessary. Participants can also access educational articles related to periodontal tissue health tailored to patient needs (based on diagnosis).
Subgingival plaque sampling
This was a single-site analysis, with sampling performed by two trained periodontology residents (M.R.P. and M.M.P.). This approach provided a detailed understanding of the microbiological changes occurring at individual sites [Citation16]. At the baseline visit, subgingival plaque was sampled from the single deepest PPD site that was selected in each patient immediately prior to the beginning of SRP. Subgingival plaques were re-collected from the same site at one- and three-month visits for re-evaluation. To collect samples, the tooth was isolated using a cotton roll, and the supragingival plaque was initially removed, then the subgingival plaque was harvested using a sterile Gracey curette (Hu-Friedy, Chicago, IL, USA). Samples were placed immediately into a sterile Eppendorf tube containing 1000 μL of phosphate-buffered saline and labeled. The tube was stored at −80°C until further analysis.
DNA extraction and sequencing
DNA was extracted from subgingival plaque samples using the DNA Extraction Kit (InstaGeneTM Matrix, Bio-Rad, California, US). DNA concentration was determined using both NanoDrop spectrophotometers and Qubit fluorometers (Thermo Fisher Scientific, Waltham, MA, USA). The NGS platform, Nanopore sequencing (full-length sequence of 16s rRNA gene [V1-V9 regions]) (Oxford Nanopore Technology, Oxford, UK) [Citation36], was used for library preparation and sequencing. Nanopore sequencing was operated by MinKNOW software version 23.04.5 (Oxford Nanopore Technology, Oxford, UK). Base-calling was performed using Guppy version 6.5.7 (Oxford Nanopore Technology, Oxford, UK) with a high-accuracy model [Citation37]. The quality of FASTQ files was visualized using NanoPlot (Oxford Nanopore Technology, Oxford, UK), and quality filtering was performed using NanoFilt (Oxford Nanopore Technology, Oxford, UK) [Citation38,Citation39]. Filtered reads were classified using a centrifuge classifier (Thermo Fisher Scientific, Waltham, MA, USA). The bacteria and archaea index was built using the NCBI 16S RefSeq database (https://ftp.ncbi.nlm.nih.gov/refseq/TargetedLoci/). Downstream analysis and visualizations were performed using the R programming language (version 4.2.3, R Core Team, https://www.r-project.org/), with specific packages including Pavian (version 1.2.1, http://github.com/fbreitwieser/pavian) [Citation40], Krona Tools (version 2.8.1, https://github.com/marbl/Krona) [Citation41], and Vegan (version 2.6–6.1, https://github.com/vegandevs/vegan) [Citation42]. These open-source tools facilitated comprehensive analysis and visualization of the subgingival microbiota.
Statistical analysis
The clinical data were analyzed with SPSS software version 26 (IBM, Chicago, IL, USA) and visualized with GraphPad Prism software version 10.1.0 (GraphPad, Boston, MA, USA). At baseline, clinical data were presented as percentages, except for site-PPD and site-CAL, which were measured in millimeters. Variables analyzed for changes at one- and three-month visits included FMPS, BOP, and site-PPD. Descriptive statistics (e.g. median and Q1, Q3) were used to present those variables. The independent t-test was applied to compare the subjects’ means, while the chi-square test was employed to compare the subjects’ educational levels. The Shapiro–Wilk test was used to assess the normality of the data, revealing both normal and non-normal data distributions. For the analysis of inter-group comparisons (between two groups at each timepoint), the Mann-Whitney U test was utilized due to its suitability for non-parametric data. Exceptions to this were FMPS and BOP at baseline, which were analyzed using the independent t-test as they met the normality assumption. Intra-group comparisons (between timepoints within each group) were evaluated using the Friedman test with a post-hoc Wilcoxon Signed-Rank Test. A p-value <0.05 was considered statistically significant.
Analysis of the subgingival plaque microbiota was performed using the R programming language (version 4.2.3, R Core Team, https://www.r-project.org/). Alpha-diversity indices (observed species, Shannon, and abundance-based coverage estimator [ACE]) were calculated at the species level using the Vegan package in R (version 2.6–6.1, https://github.com/vegandevs/vegan) [Citation42]. Intra-group differences in α-diversity were assessed using the Wilcoxon signed-rank test. Beta-diversity was calculated using the Bray-Curtis dissimilarity metric (Vegan Package, version 2.6–6.1, https://github.com/vegandevs/vegan) [Citation42] and visualized via Principal Component Analysis (PCA) using ClustVis in R (version 0.10.2.1, http://biit.cs.ut.ee/clustvis/) [Citation43]. Inter-group differences in β-diversity were assessed using Analysis of Similarity (ANOSIM) (Vegan Package, version 2.6–6.1, https://github.com/vegandevs/vegan) [Citation42]. Microbiota composition at the species and genus levels was analyzed using the Mann-Whitney U test for inter-group analysis and the Wilcoxon Signed-Ranks test for intra-group analysis. All p-values were adjusted for multiple comparisons using the Benjamini–Hochberg correction. A p-value of <0.05 indicates statistical significance.
Results
A CONSORT flowchart reporting participants in this study is shown in . After screening, 50 participants fulfilling the inclusion criteria were recruited. At 3 months, a total of 6 participants were lost (dropouts). Three participants dropped out of the control group (two participants at the 1-month visit and the other one at the 3-month visit), and three participants from the test group dropped out at the 3-month visit. One participant from the control group chose to withdraw from the study due to personal reasons, while the other five participants were lost to follow-up. A total of 44 participants (22 participants per group) completed the study and were included in the final analysis.
presents the baseline demographic and periodontal clinical parameters of the study participants. No statistically significant differences (p > 0.05) were observed with respect to the mean age and education level between the two groups. The baseline clinical parameters were comparable between the test and control groups (p > 0.05).
Evaluation of periodontal clinical parameters
Comparisons of periodontal clinical parameters (FMPS, BOP, and site-PPD) between groups at baseline, one- and three-month visits are shown in . At re-evaluation, all clinical parameters were significantly improved in both groups (p < 0.001 for all clinical parameters between the three timepoints), with the test group having lower values than the control group. However, there was no significant difference in any parameters between groups (p > 0.05 for all).
Table 2. Comparison of Periodontal Clinical Parameters between groups at baseline, one- and three-month visits.
Post-hoc analysis of clinical parameters in the test group showed significant reductions in FMPS, BOP, and site-PPD after one- and three-months of using the mobile app compared to baseline (p < 0.001) (). Similar results were also seen in the control group (p < 0.001) (). There is no significant decrease in FMPS between one- and three-month visits in both groups (p > 0.05) (). Evaluation of BOP between one- and three-months showed no significant decrease in the test group (p > 0.05) (), while there was a significant increase in the control group (p < 0.05) (). A significant decrease in site-PPD of the test group was also observed between the one- and three-month visits (p < 0.05) (), whereas in the control group there was no significant reduction (p > 0.05) ().
Evaluation of subgingival microbiota diversity
The observed species, Shannon, and ACE indices (, Supplementary Table S1) were used to determine the diversity of the subgingival microbiota. Compared to baseline, there was an increase in the number of observed species, Shannon, and ACE indices within the test group at one- and three-month visits (). The increase indicates an increase in the diversity of microbiota species in the sample, but this difference was not statistically significant (p > 0.05). Similar results were seen in the control groups, with no significant changes in those indices at one- and three-month visits compared to baseline (p > 0.05). ()
Figure 4. Comparison of α-Diversity between Baseline, One-, and Three-month Visits in Test Group. The α-diversity Indices: (a) Observed species, (b) Shannon, and (c) Abundance-based Coverage Estimator (ACE).
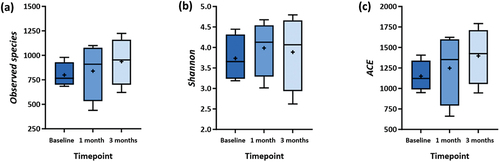
Figure 5. Comparison of α-Diversity between Baseline, One-, and Three-month Visits in Control Group. The α-diversity Indices: (a) Observed species, (b) Shannon, and (c) Abundance-based Coverage Estimator (ACE).
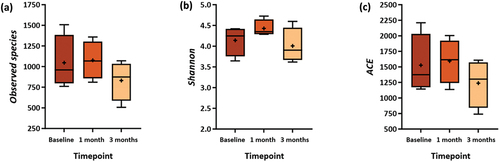
At the one- and three-month visits, the PCA plot showed overlap between the test and control groups (). Analysis of Similarities (ANOSIM) results between these two groups at the one-month visit showed R = −0.25, indicating a slight similarity between two groups, while at the three-month visit the value of R = 0.279 implied low similarity between groups. However, this difference was also not statistically significant (p > 0.05) ().
Evaluation of subgingival microbiota composition
show the top ten subgingival microbiota at the genus and species levels in both the test and control groups. At baseline, the subgingival microbiota composition in the test group was dominated by Prevotella (23.45%), Pseudomonas (19.83%), and Porphyromonas (12.30%). Meanwhile, the control group predominantly consisted of the genera Prevotella (22.66%), Neisseria (11.48%), and Porphyromonas (11.35%) (). Disease-associated species such as Prevotella intermedia (13.88%), Porphyromonas gingivalis (10.37%), and Anaeroglobus geminatus (4.92%) were found in the top three positions in the test group’s microbiota composition at baseline, whereas Prevotella oris (7.70%), Porphyromonas endodontalis (7.12%), and Hoylesella loescheii (5.57%) dominated the control group (). These genera and species showed no significant differences between groups at baseline (p > 0.05 for all).
Figure 7. Composition of Subgingival Microbiota at Genus Level.in (a) Test Group and (b) Control Group.
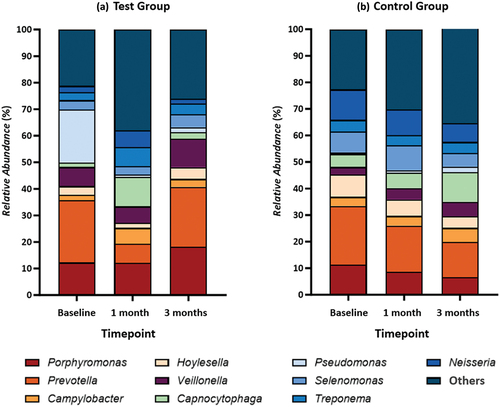
Figure 8. Composition of Subgingival Microbiota at Species Level.in (A) Test Group and (B) Control Group.
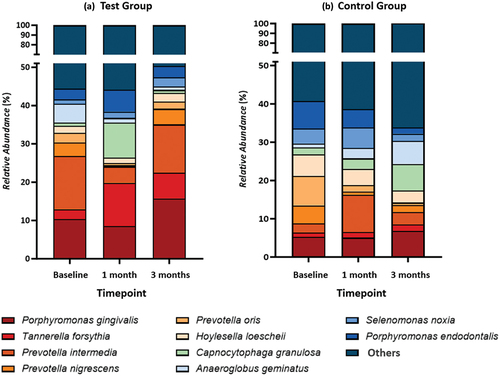
Investigating the changes in the proportion of subgingival microbiota composition following interventions, no significant differences were found at one- and three-month visits in both groups when compared to baseline (p > 0.05). However, there are some trends that could be observed. In the test group, Prevotella, Pseudomonas, and Porphyromonas decreased at the one-month visit but then increased at the three-month visit. Genera like Veillonela, Selenomonas, Hoylesella, and Capnocytophaga also showed a similar trend (). This trend also happens at the species level; disease-associated species like P. gingivalis, Prevotella intermedia, Prevotella nigrescens, Prevotella oris, and H. loescheii decreased at one-month visit but increased at three-month visit. Meanwhile, another periodontal pathogen, Tannerella forsythia (), showed the opposite pattern. In control groups, genera Prevotella, Neisseria, Porphyromonas, Hoylesella, and Treponema decreased over time, while Capnocytophaga, Campylobacter, Veillonella, and Pseudomonas increased (). At species level, the trend was similar to the test group; P. gingivalis and Prevotella nigrescens decreased at one-month visit but increased at three-month visit, whereas Prevotella intermedia showed the opposite. Species T. forsythia increased over time (). These genera and species at one- and three-month visits also showed no significant differences between groups (p > 0.05 for all).
Discussion
The most common barrier to patient adherence to OHI is the patient’s difficulty in understanding and recalling all the information that they have received [Citation44]. Various approaches based on behavioral sciences and communication skills have been proposed to improve and maintain patient adherence to OHI [Citation5]. Studies show that the use of mobile-based health applications (mHealth) is an effective approach to engaging patients in their health care, can be used as a behavior change instrument, including oral hygiene adherence, and may play an important part in the treatment of periodontitis patients [Citation10,Citation44].
This randomized controlled trial aimed to evaluate the impact of mobile app-based OHI on periodontitis patient management by analyzing periodontal clinical parameters, subgingival microbiota diversity, and composition. To the best of our knowledge, this is the first study to investigate the effect of mobile app-based OHI on both clinical parameters and subgingival microbiota. The study diagnosed periodontitis according to the 2017 AAP/EFP criteria [Citation1,Citation21]. During the baseline visit, a clinical assessment of the CAL was performed for diagnosis, and the CAL on the sampling site (site-CAL) was recorded. However, changes in CAL following the intervention were not analyzed. Additionally, participants were not categorized into specific stages or grades of periodontitis, as the initial phase of treatments, i.e. SRP and OHI, applies to all periodontitis patients. The study aimed to assess the app’s effectiveness for periodontitis patients in general. The study only focused on one gender (female) to eliminate potential confounding factors, such as hormonal differences, that might affect periodontal health and microbiota composition. Furthermore, females have a higher prevalence of periodontitis than males [Citation45] making them a significant demographic for this study. This approach aligns with previous research [Citation46], as this study is a continuation of earlier studies.
There were no significant differences in periodontal clinical parameters between the two groups at baseline, indicating their comparability. At one- and three-month visits, both groups showed significant reductions in FMPS, BOP, and PPD at sampling sites (site-PPD) compared to baseline. These outcomes align with the study by Williams et al. [Citation8], which reported an improvement in periodontal clinical parameters following initial therapy, regardless of the OHI method. Studies have shown that scaling and root planing are effective in reducing plaque, PPD, and clinical inflammation, as indicated by BOP values [Citation47–49].
The current study showed no significant differences in clinical parameters (FMPS, BOP, and site-PPD) between participants who used the mobile app-based OHI and those who did not at any timepoints. However, intra-group analysis (between timepoints) indicated varying trends (). Participants in the test group showed better improvement in gingival inflammation (measured by BOP values) and PPD at the sampling sites compared to the control group, particularly evident between the one- and three-month visits. This could be due to participants’ repetitive education and notifications regarding OHI, which motivated them to improve their oral hygiene practices and enhance treatment outcomes. This aligns with the previous study reporting that the mobile app (‘Perio UI Care’) is effective in improving patients’ cognitive and psychomotor factors related to oral hygiene practices [Citation46]. Moreover, smartphones’ accessibility enables patients to easily obtain information anytime and anywhere, enhancing their convenience and autonomy [Citation10]. These findings are consistent with studies by Fernández et al. [Citation50], and Toniazzo et al. [Citation10], which emphasize the potential benefits of mHealth (mobile apps and text messages) interventions in improving clinical outcomes compared to conventional face-to-face strategies.
Unlike the study by Hartono et al. [Citation46], which evaluated the use of the mobile app in periodontal disease patients (mainly gingivitis), the absence of significant differences in clinical parameters in the present study may be due to the irreversible nature of periodontal tissue destruction in periodontitis patients. In gingivitis, the inflammatory conditions are reversible, and patients can recover to a healthy condition after treatment and routine plaque control [Citation24,Citation51,Citation52]. Moreover, advanced periodontitis cases (stage III or IV) typically have soft and hard tissue defects that can only be corrected surgically [Citation47]. Based on the 3-month re-evaluations in both groups, there is a need for continued active periodontal treatment, including potential surgical interventions, emphasizing the ongoing challenges in managing periodontitis.
In line with the clinical condition, no significant difference in microbiota diversity was observed between groups and across timepoints. These findings contrast with a study by Nath et al. [Citation53], which reported a decrease in the diversity of subgingival microbiota following periodontal treatments. The debate over changes in microbiota diversity post-treatment persists [Citation28,Citation54], most likely due to the complex interplay between pathogenic and commensal species. Following therapy, the decrease in pathogenic species alongside an increase in commensal species complicates the assessment of post-treatment microbiota diversity [Citation28].
Although no significant differences were found, there were increasing trends in diversity at one- and three-month visits compared to baseline in the test group. This trend could be related to decreasing PPD at the sampling sites following the intervention. Kirst et al. [Citation55] found that shallower pockets (6 mm) had higher diversity and species richness than deeper pockets (>7–8 mm). These findings support Van Dyke et al.’s [Citation18] hypothesis regarding the pathophysiology of periodontitis. In the early stages of periodontitis, polymicrobial diversity increases, but as the disease progresses, diversity decreases, with anaerobic microbial species dominating in the periodontal pockets [Citation56].
The anaerobic Gram-negative genera Prevotella and Porphyromonas were predominant in the subgingival microbial composition at baseline for both groups. At species level, P. gingivalis and T. forsythia, the key pathogens in periodontitis and part of the red complex bacteria (Socransky’s bacteria complexes), were also present alongside the orange complex species Prevotella intermedia and Prevotella nigrescens, which act as catalysts or ‘bridging species’ for more pathogenic red complexes [Citation18,Citation34,Citation57]. These findings are consistent with previous studies suggesting the significant roles of these complexes in periodontitis development [Citation28]. There was also Anaeroglobus geminatus, an anaerobic Gram-negative species that has been linked to Prevotella and might contribute to microbiota shifts toward periodontitis [Citation58]. Bao et al. [Citation58] found that A. geminatus increased the abundance of Prevotella intermedia and altered the protein expression of biofilm communities, potentially enhancing the pathogenicity of subgingival biofilms, though further functional confirmation is needed.
No significant differences in microbiota composition (genus and species level) were observed following interventions in both groups. This finding aligns with previous studies stating that periodontitis-associated microbiota can persist in some patients despite improvements in clinical parameters after treatment [Citation54,Citation59]. The trend of the recovery of certain species, such as P. gingivalis, Prevotella intermedia, Prevotella nigrescens, Prevotella oris, and H. loescheii, to baseline levels at the three-month re-evaluation is consistent with other studies. Johnston et al. [Citation16] observed drastic changes in microbiota composition from baseline to day 1 post-instrumentation and remained stable until day 7. By day 90, some samples showed a resurgence of disease-associated species (entire recovery), although this condition tends not to be associated with post-treatment clinical outcomes [Citation16]. Other studies have also reported shifts in subgingival microbiota abundance following treatment and a gradual return to baseline (pre-treatment) levels after 12 weeks [Citation11,Citation17].
Several factors are known to influence changes in the oral microbiota and may partly explain these results. Divaris et al. [Citation60] suggested genetic susceptibility to colonization by red complex species, indicating that host genetics play a significant role in microbiota composition alterations. Johnston et al. [Citation16], propose that each patient may have their own ‘dysbiosis threshold’. Moreover, the persistence of periodontal pockets post-treatment, albeit reduced, allows recolonization of disease-associated species and biofilm development, contributing to the chronic nature of periodontitis [Citation16]. The significance of the microbiota’s response to the absence of clinical signs of disease remains unclear. However, a high abundance of disease-associated species may predict future disease progression. This may explain why periodontitis patients have a higher risk of recurrence than healthy periodontal patients, despite effective periodontal treatment [Citation16,Citation17]. This finding highlights the critical importance of supportive periodontal therapy (maintenance phase/phase IV) in preserving periodontal health (in intact or reduced periodontium) achieved through active periodontal treatment. This phase involves comprehensive OHI and regular recall visits, customized to the patient’s needs, to ensure the continued maintenance of periodontal health treatment outcomes periodontitis patients [Citation2,Citation34].
This study found no significant differences in microbiota composition between groups, which aligns with the beta diversity analysis. This outcome is consistent with the re-evaluation of clinical parameters, suggesting that the interventions did not lead to substantial changes in the microbial composition or clinical outcomes. There are some limitations of this study that should be considered when interpreting the findings. The lack of a healthy control group limits the ability to compare the post-intervention microbiota and clinical parameters with pristine periodontal health, which could have provided further insight into the interventions’ efficacy. Despite controlling the potential confounding factor by only including female participants, this study did not collect data on the menopause status of participants, potentially impacting the periodontium condition and influencing the outcomes [Citation61]. The short duration of the study’s follow-up also might limit the understanding of the long-term effect of the mobile app-based OHI on periodontal health. Further investigation with longer follow-up periods and a broader participant profile, including both gender and health controls, may provide a more comprehensive understanding of the effects of mobile-app interventions. Additionally, recently introduced compounds and techniques have shown significant influence on the oral environment. Future research could explore the combined effect of mobile app interventions with other preventive therapies, such as Ozone [Citation62], photobiomodulation [Citation63], and probiotics [Citation64], to better understand their collective impact on oral microbiota.
Conclusions
While the test group showed promising trends of improvement in clinical parameters, particularly between one- and three-month visits, the lack of statistically significant differences in clinical outcomes, microbiota diversity, and composition between groups suggests that mobile app-based OHI may not provide substantial short-term advantages over conventional OHI. However, the observed improvements in the app-utilizing group warrant further investigation into potential long-term benefits. Future research with longer follow-up periods and a more diverse participant profile is necessary to fully assess the efficacy of mobile app-based OHI interventions on periodontitis treatment.
Author contribution
M.R.P., M.M.P., B.S., and A.W. designed the study and performed the analysis. V.H., Y.S., and A.K.R., collected the sample and designed the apps. F.M.T. and M.R.C.T.L. validated the result and study. B.S. and A.W. supervised the study. M.R.P. and B.S., wrote the manuscript. All authors have reviewed and agreed to the manuscript.
Ethical statements
The ethical statement was approved by the Dental Research Ethics Committee, Faculty of Dentistry, Universitas Indonesia, with letter number: 01/Ethical Approval/FKGUI/I/2022 and protocol number: 091241221.
Supplemental Material
Download (21.5 KB)Acknowledgments
The authors thank Universitas Indonesia for supporting the publication’s APC.
Disclosure statement
No potential conflict of interest was reported by the author(s).
Supplementary material
Supplemental data for this article can be accessed online at https://doi.org/10.1080/20002297.2024.2372206
Additional information
Funding
References
- Papapanou PN, Sanz M, Buduneli N, et al. Periodontitis: consensus report of workgroup 2 of the 2017 world workshop on the classification of periodontal and peri-implant diseases and conditions. J Periodontol. 2018;89(S1):S173–S182. doi: 10.1002/JPER.17-0721
- Sanz M, Herrera D, Kebschull M, et al. Treatment of stage I–III periodontitis—The EFP S3 level clinical practice guideline. J Clin Periodontol. 2020;47(S22):4–14. doi: 10.1111/jcpe.13290
- Chen MX, Zhong YJ, Dong QQ, et al. Global, regional, and national burden of severe periodontitis, 1990–2019: an analysis of the Global Burden of Disease Study 2019. J Clin Periodontol. 2021;48(9):1165–1188. doi: 10.1111/jcpe.13506
- Tonetti MS, Jepsen S, Jin L, et al. Impact of the global burden of periodontal diseases on health, nutrition and wellbeing of mankind: a call for global action. J Clin Periodontol. 2017;44(5):456–462. doi: 10.1111/jcpe.12732
- Carra MC, Detzen L, Kitzmann J, et al. Promoting behavioural changes to improve oral hygiene in patients with periodontal diseases: a systematic review. In: J. Clin. Periodontol. Vol. 47, Issue S22, pp. Blackwell Munksgaard; 2020. p. 72–89. doi: 10.1111/jcpe.13234
- Fragkioudakis I, Riggio MP, Apatzidou DA. Understanding the microbial components of periodontal diseases and periodontal treatment-induced microbiological shifts. J Med Microbiol. 2020;70(1). doi: 10.1099/JMM.0.001247
- Lertpimonchai A, Rattanasiri S, Arj-Ong Vallibhakara S, et al. The association between oral hygiene and periodontitis: a systematic review and meta-analysis. Int Dent J. 2017;67(6):332–343. doi: 10.1111/idj.12317
- Williams KA, Mithani S, Sadeghi G, et al. Effectiveness of oral hygiene instructions given in computer-assisted format versus a self-care instructor. Dent J (Basel) J. 6(1). 2018;6(1):2. doi: 10.3390/dj6010002
- Underwood B, Birdsall J, Kay E. The use of a mobile app to motivate evidence-based oral hygiene behaviour. Br Dent J. 2015;219(4):E2. doi: 10.1038/sj.bdj.2015.660
- Toniazzo MP, Nodari D, Muniz FWMG, et al. Effect of mHealth in improving oral hygiene: a systematic review with meta-analysis. J Clin Periodontol. 2019;46(3):297–309. doi: 10.1111/jcpe.13083
- Belstrøm D, Grande MA, Sembler-Møller ML, et al. Influence of periodontal treatment on subgingival and salivary microbiotas. J Periodontol. 2018;89(5):531–539. doi: 10.1002/JPER.17-0377
- Diaz PI, Hoare A, Hong B-Y. Subgingival microbiome shifts and community dynamics in periodontal diseases. CDA J. 2016;44(7):421–435. doi: 10.1080/19424396.2016.12221035
- Hajishengallis G, Lamont RJ. Beyond the red complex and into more complexity: the polymicrobial synergy and dysbiosis (PSD) model of periodontal disease etiology. Mol Oral Microbiol. 2012;27(6):409–419. doi: 10.1111/j.2041-1014.2012.00663.x
- Könönen E, Gursoy M, Gursoy UK. Periodontitis: a multifaceted disease of tooth-supporting tissues. J Clin Med. 2019;8 doi: 10.3390/jcm8081135
- Johnston W, Rosier BT, Artacho A, et al. Mechanical biofilm disruption causes microbial and immunological shifts in periodontitis patients. doi: 10.1038/s41598-021-89002-z
- Johnston W, Rosier BT, Carda-Diéguez M, et al. Longitudinal changes in subgingival biofilm composition following periodontal treatment. J Periodontol. 2023;94(9):1065–1077. doi: 10.1002/JPER.22-0749
- Lu H, Zhao Y, Feng X, et al. Microbiome in maintained periodontitis and its shift over a single maintenance interval of 3 months. J Clin Periodontol. 2019;46(11):1094–1104. doi: 10.1111/jcpe.13177
- R core team. R: a language and environment for statistical computing. Vienna, Austria: R Foundation for Statistical Computing; 2021. Available from: https://www.R-project.org/
- Zhang Y, Qi Y, Lo ECM, et al. Using next-generation sequencing to detect oral microbiome change following periodontal interventions: a systematic review. Oral Dis. 2021;27(5):1073–1089). doi: 10.1111/odi.13405
- Schulz KF, Altman DG, Moher D. CONSORT 2010 Statement: updated guidelines for reporting parallel group randomised trials. BMC Med. 2010;8(1):18. http://www.biomedcentral.com/1741-7015/8/18
- Tonetti MS, Greenwell H, Kornman KS. Staging and grading of periodontitis: Framework and proposal of a new classification and case definition. J Periodontol. 2018;89(February):S159–S172. doi: 10.1002/JPER.18-0006
- Bouchard P, Carra MC, Boillot A, et al. Risk factors in periodontology: a conceptual framework. J Clin Periodontol. 2017;44(2):125–131. doi: 10.1111/jcpe.12650
- Chapple ILC, Genco R. Diabetes and periodontal diseases: consensus report of the Joint EFP/AAP Workshop on Periodontitis and Systemic Diseases. J Periodontol. 2013;84(4S). doi: 10.1902/jop.2013.1340011
- Chapple ILC, Mealey BL, Van Dyke TE, et al. Periodontal health and gingival diseases and conditions on an intact and a reduced periodontium: Consensus report of workgroup 1 of the 2017 world workshop on the classification of periodontal and peri-implant diseases and conditions. J Periodontol. 2018;89(S1):S74–S84. doi: 10.1002/JPER.17-0719
- Hajishengallis G. Periodontitis: From microbial immune subversion to systemic inflammation. Nat Rev Immunol. 2015;15(1):30–44. doi: 10.1038/nri3785
- Chatzopoulos GS, Jiang Z, Marka N, et al. Relationship of medication intake and systemic conditions with periodontitis: a retrospective study. J Pers Med. 2023;13(10):1480. doi: 10.3390/jpm13101480
- Kopra E, Lahdentausta L, Pietiäinen M, et al. Systemic antibiotics influence periodontal parameters and oral microbiota, but not serological markers. Front Cell Infect Microbiol. 2021;11. doi: 10.3389/fcimb.2021.774665
- Zhang J, Yu J, Dou J, et al. The impact of smoking on subgingival plaque and the development of periodontitis: a literature review. Front Oral Health.Vol. (2021;2. doi: 10.3389/froh.2021.751099
- Jang H, Patoine A, Wu TT, et al. Oral microflora and pregnancy: a systematic review and meta-analysis. Sci Rep. 2021;11(1). doi: 10.1038/s41598-021-96495-1
- Ye C, Kapila Y. Oral microbiome shifts during pregnancy and adverse pregnancy outcomes: hormonal and immunologic changes at play. Periodontol. 2000;87(1):276–281. John Wiley and Sons Inc. doi: 10.1111/prd.12386
- Alkadhi OH, Zahid MN, Almanea RS, et al. The effect of using mobile applications for improving oral hygiene in patients with orthodontic fixed appliances: a randomised controlled trial. J Orthods. 2017;44(3):157–163. doi: 10.1080/14653125.2017.1346746
- Iorio-Siciliano V, Ramaglia L, Isola G, et al. Changes in clinical parameters following adjunctive local sodium hypochlorite gel in minimally invasive nonsurgical therapy (MINST) of periodontal pockets: a 6-month randomized controlled clinical trial. Clin Oral Investig. 2021;25(9):5331–5340. doi: 10.1007/s00784-021-03841-8
- Lang NP, Bartold PM. Periodontal health. J Periodontol. 2018;89(S1):S9–S16. doi: 10.1002/JPER.16-0517
- Newman MG, Takei H, Klokkevold PR, et al. Newman and carranza’s clinical periodontology. 13th ed. Philadelphia (PA): Elsevier Health Sciences; 2018.
- Kwok V, Caton JG. Prognosis revisited: a system for assigning periodontal prognosis. J Periodontol. 2007;78(11):2063–2071. doi: 10.1902/jop.2007.070210
- Bahram M, Anslan S, Hildebrand F, et al. Newly designed 16S rRNA metabarcoding primers amplify diverse and novel archaeal taxa from the environment. Environ Microbiol Rep. 2019;11(4):487–494. doi: 10.1111/1758-2229.12684
- Wick RR, Judd LM, Holt KE. Performance of neural network basecalling tools for Oxford Nanopore sequencing. Genome Biol. 2019;20(1). doi: 10.1186/s13059-019-1727-y
- De Coster W, D’Hert S, Schultz DT, et al. NanoPack: Visualizing and processing long-read sequencing data. Bioinformatics. 2018;34(15):2666–2669. doi: 10.1093/bioinformatics/bty149
- Nygaard AB, Tunsjø HS, Meisal R, et al. A preliminary study on the potential of Nanopore MinION and Illumina MiSeq 16S rRNA gene sequencing to characterize building-dust microbiomes. Sci Rep. 2020;10(1):3209. doi: 10.1038/s41598-020-59771-0
- Breitwieser FP, Salzberg SL, Schwartz R. Pavian: Interactive analysis of metagenomics data for microbiome studies and pathogen identification. Bioinformatics. 2020;36(4):1303–1304. doi: 10.1093/bioinformatics/btz715
- Ondov BD, Bergman NH, Phillippy AM. Interactive metagenomic visualization in a web browser. BMC Bioinformatics. 2011;12(1). doi: 10.1186/1471-2105-12-385
- Oksanen J, Simpson GL, Blanchet FG, et al. Vegan: community ecology package. R Package Version 2.4–3. 2017. https://CRAN.R-project.org/package=vegan
- Metsalu T, Vilo J. ClustVis: a web tool for visualizing clustering of multivariate data using Principal Component Analysis and heatmap. Nucleic Acids Res. 2015;43(W1):W566–W570. doi: 10.1093/nar/gkv468
- Chang WJ, Wang YL, Chang YH, et al. Effectiveness of an app-based mobile intervention for precision oral self-care in patients with periodontitis from initial therapy to re-evaluation. Appl Sci. 2021;11(9):4229. doi: 10.3390/app11094229
- Kementerian Kesehatan RI Badan Penelitian dan Pengembangan Kesehatan. Laporan Nasional RISKESDAS 2018. 2018.
- Hartono V, Setiadharma Y, Rizany AK, et al. Mobile application-based support for periodontal treatment improves clinical, cognitive, and psychomotor outcomes: a randomized controlled trial study. Dent J. 2024;12(3):63. doi: 10.3390/dj12030063
- Cobb CM, Sottosanti JS. A re-evaluation of scaling and root planing. J Periodontol. 2021;92(10):1370–1378. doi: 10.1002/JPER.20-0839
- Deas DE, Moritz AJ, Sagun RS, et al. Scaling and root planing vs. conservative surgery in the treatment of chronic periodontitis. Periodontol. 2000;71(1):128–139. doi: 10.1111/prd.12114
- Needleman I, Nibali L, Di Iorio A. Professional mechanical plaque removal for prevention of periodontal diseases in adults – systematic review update. J Clin Periodontol. 2015;421):S12–S35. doi: 10.1111/jcpe.12341
- Fernández CE, Maturana CA, Coloma SI, et al. Teledentistry and mHealth for promotion and prevention of oral health: a systematic review and meta-analysis. J Dent Res. 2021;100(9):914–927. doi: 10.1177/00220345211003828
- Murakami S, Mealey BL, Mariotti A, et al. Dental plaque–induced gingival conditions. J Periodontol. 2018;89(S1):S17–S27. doi: 10.1002/JPER.17-0095
- Tonetti MS, Eickholz P, Loos BG, et al. Principles in prevention of periodontal diseases: Consensus report of group 1 of the 11th European Workshop on Periodontology on effective prevention of periodontal and peri-implant diseases. J Clin Periodontol. 2015;42(S16):S5–S11. doi: 10.1111/jcpe.12368
- Nath S, Pulikkotil SJ, Weyrich L, et al. Effect of periodontal interventions on characteristics of the periodontal microbial profile: a systematic review and meta-analysis. Microorganisms. 2022;10(8):1582. doi: 10.3390/microorganisms10081582
- Chen C, Hemme C, Beleno J, et al. Oral microbiota of periodontal health and disease and their changes after nonsurgical periodontal therapy. Isme J. 2018;12(5):1210–1224. doi: 10.1038/s41396-017-0037-1
- Kirst ME, Li EC, Alfant B, et al. Dysbiosis and alterations in predicted functions of the subgingival microbiome in chronic periodontitis. Appl Environ Microbiol. 2015;81(2):783–793. doi: 10.1128/AEM.02712-14
- Van Dyke TE, Bartold PM, Reynolds EC. The aexus between periodontal inflammation and dysbiosis. doi: 10.3389/fimmu.2020.00511
- Zhang Y, Zhen M, Zhan Y, et al. Population-genomic insights into variation in Prevotella intermedia and Prevotella nigrescens isolates and its association with periodontal disease. Front Cell Infect Microbiol. 2017;7(SEP). doi: 10.3389/fcimb.2017.00409
- Bao K, Bostanci N, Thurnheer T, et al. Proteomic shifts in multi-species oral biofilms caused by anaeroglobus geminates. Sci Rep. 2017;7(1). doi: 10.1038/s41598-017-04594-9
- Schwarzberg K, Le R, Bharti B, et al. The personal human oral microbiome obscures the effects of treatment on periodontal disease. PLOS ONE. 2014;9(1):e86708. doi: 10.1371/journal.pone.0086708
- Divaris K, Monda KL, North KE, et al. Genome-wide association study of periodontal pathogen colonization. J Dent Res. 2012;91(7_suppl):S21–S28. doi: 10.1177/0022034512447951
- Bhardwaj A, Bhardwaj S. Effect of menopause on women′s periodontium. J Midlife Health. 2012;3(1):5. doi: 10.4103/0976-7800.98810
- Scribante A, Gallo S, Pascadopoli M, et al. Ozonized gels vs chlorhexidine in non-surgical periodontal treatment: a randomized clinical trial. Oral Dis. 2023. doi: 10.1111/odi.14829
- Elbay M, Elbay ÜŞ, Kaya E, et al. Effects of photobiomodulation with different application parameters on injection pain in children: a randomized clinical trial. JOCPD. 2023;47(4):54–62. doi: 10.22514/jocpd.2023.035
- Butera A, Maiorani C, Gallo S, et al. Evaluation of adjuvant systems in non-surgical peri-implant treatment: a literature review. Healthcare (Switz). 2022;10(5):886. doi: 10.3390/healthcare10050886