ABSTRACT
Background: Although trauma exposure (TE) is a transdiagnostic risk factor for many psychiatric disorders, not everyone who experiences TE develops a psychiatric disorder. Resilience may explain this heterogeneity; thus, it is critical to understand the etiologic underpinnings of resilience.
Objective: The present study sought to examine the genetic underpinnings of psychiatric resilience using genome-wide association studies (GWAS), genome-wide complex trait analysis (GCTA), and polygenic risk score (PRS) analyses.
Method: Participants were 6,634 trauma exposed college students attending a diverse, public university in the Mid Atlantic. GWAS and GCTA analyses were conducted, and using GWAS summary statistics from large genetic consortia, PRS analyses examined the shared genetic risk between resilience and various phenotypes.
Results: Results demonstrate that nine single-nucleotide polymorphisms (SNPs) met the suggestive of significance threshold, heritability estimates for resilience were non-significant, and that there is genetic overlap between resilience and AD, as well as resilience and PTSD.
Conclusion: Mixed findings from the present study suggest additional research to elucidate the etiological underpinnings of resilience, ideally with larger samples less biased by variables such as heterogeneity (i.e. clinical vs. population based) and population stratification. Genetic investigations of resilience have the potential to elucidate the molecular bases of stress-related psychopathology, suggesting new avenues for prevention and intervention efforts.
HIGHLIGHTS
Resilience may explain the heterogeneity in outcomes following trauma exposure; thus, it is critical to understand the etiologic underpinnings of resilience.
The present study sought to examine the genetic underpinnings of psychiatric resilience using genome-wide association studies (GWAS), genome-wide complex trait analysis (GCTA), and polygenic risk score (PRS) analyses.
Results demonstrated shared genetic overlap between resilience and Alcohol Dependence, as well as resilience and PTSD.
Genetic investigations of resilience have the potential to elucidate the molecular bases of stress-related psychopathology, suggesting new avenues for prevention and intervention efforts.
Antecedentes: Aunque la exposición al trauma (ET) es un factor de riesgo transdiagnóstico para muchos trastornos psiquiátricos, no todas las personas que experimentan ET desarrollan un trastorno psiquiátrico. La resiliencia podría explicar esta heterogeneidad; por lo tanto, resulta crítico comprender los fundamentos etiológicos de la resiliencia.
Objetivo: El presente estudio buscó examinar los fundamentos genéticos de la resiliencia psiquiátrica utilizando un estudio de asociación del genoma completo (GWAS), el análisis de rasgo del genoma complejo (GCTA) y la prueba de puntuación de riesgo poligénico (PRS).
Método: Los participantes fueron 6.634 estudiantes universitarios expuestos a trauma que asistían a una universidad pública diversa ubicada en el Atlántico Medio. Se realizaron los análisis GWAS y GCTA y, utilizando resúmenes estadísticos del análisis GWAS de grandes consorcios genéticos, las pruebas PRS examinaron el riesgo genético compartido entre la resiliencia y varios fenotipos.
Resultados: Los resultados demuestran que nueve polimorfismos de nucleótido único (SNPs) cumplieron con el umbral de significación sugerente, las estimaciones de heredabilidad para la resiliencia fueron no significativas, y que existe una superposición genética entre la resiliencia y AD, así como entre la resiliencia y el TEPT.
Conclusión: Los hallazgos mixtos del presente estudio sugieren realizar investigación adicional para dilucidar los fundamentos etiológicos de la resiliencia, idealmente con muestras más grandes y con menor sesgo por variables como la heterogeneidad (por ejemplo, muestras clínicas versus poblacionales) y la estratificación de la población. Las investigaciones genéticas sobre la resiliencia tienen el potencial de dilucidar las bases moleculares de la psicopatología relacionada con el estrés, lo que sugiere nuevas vías para los esfuerzos relacionados con la prevención e intervención.
背景:虽然创伤暴露 (TE) 是许多精神障碍的跨诊断风险因素,但并不是每个经历过 TE 的人都会发展为精神障碍。 心理韧性可以解释这种异质性; 因此,了解心理韧性的病因学基础至关重要。
目的:本研究旨在使用全基因组关联研究 (GWAS)、全基因组复杂性状分析 (GCTA) 和多基因风险评分 (PRS) 分析来考查心理韧性的遗传基础。
方法:参与者是 6,634名曾就读于大西洋中部多元化公立大学的创伤暴露大学生。 进行了 GWAS 和 GCTA 分析,并使用来自大型遗传联盟的 GWAS 汇总统计数据, PRS 分析考查了心理韧性和各种表型之间的共享遗传风险。
结果:结果表明,9 个单核苷酸多态性 (SNP) 符合显著性阈值的提示,心理韧性的遗传力估计不显著,心理韧性与 AD 以及心理韧性与 PTSD 之间存在遗传重叠。
结论:本研究的混合结果表明需要开展更多研究来阐明心理韧性的病因学基础,理想情况下是更大的样本量,受异质性(即基于临床与基于人群)和人群分层等变量的偏差较小。 心理韧性的遗传研究有可能阐明应激相关精神疾病的分子学基础,为预防和干预工作提供新途径。
KEYWORDS:
PALABRAS CLAVE:
1. Introduction
Trauma exposure (TE) is common in the general population, with rates estimated at ∼70% (e.g. Benjet et al., Citation2016). TE is a transdiagnostic risk factor for the development of psychiatric disorders including, but not limited to, posttraumatic stress disorder ([PTSD], Rosellini et al., Citation2018) and alcohol use disorder (AUD; Boscarino, Adams, & Galea, Citation2006), which often co-occur following TE. Co-morbid PTSD and AUD are associated with increased symptom severity, greater healthcare utilization, and poorer treatment prognosis (Blanco et al., Citation2013; Ipser, et al., Citation2015; Shorter, et al. Citation2015). Although TE is both common and a transdiagnostic risk factor for the development of adverse psychiatric outcomes, the majority of people who experience TE do not develop a disorder (for a review see Galatzer-Levy et al., Citation2018).
Resilience has been proposed as partially explaining the observed heterogeneity in response to TE (for a review, see Windle, Citation2011). Although numerous definitions of resilience exist in the literature, the crux of the concept is the adaptation to and coping with environmental exposures (i.e. TE). In the present study, resilience was conceptualized as a discrepancy-based resilience variable wherein one’s lifetime TE is regressed on their reported anxiety and depressive symptoms (e.g. Amstadter et al., Citation2014, etc.) The residual is then considered to capture a quantitative metric of one’s level of resilience, with individuals endorsing relatively higher levels of TE and lower levels of symptoms having high levels of resilience (e.g. Amstadter et al., Citation2014). This work captures reported symptoms relative to one’s trauma load as resilience, with those having less symptoms at higher levels of TE showing more of an ability to ‘bounce back’ from these negative experiences. That is, we are using current symptoms of anxiety and depression relative to one’s trauma load as indicating one’s ability to maintain functioning and recover from stressful and traumatic events. Indeed, extant work has shown that this operationalization is separate from merely the absence of psychopathology (i.e. PTSD) following TE (Wolf et al., Citation2018).
In contextualizing the variable used in the present study with regards to the most commonly seen conceptualizations of resilience (i.e. trait, outcome, process), discrepancy-based resilience is most similar to a trait-based conceptualization. Resilience in the present study is theorized to be an interpersonal characteristic that has been shown to be both moderately heritable with the genetic and environmental influences stable over time (Amstadter et al., Citation2014). However, the genetic influences on discrepancy-based resilience do not account for 100% of the phenotype, and thus, the environment plays a critical role in influencing levels of discrepancy-based resilience.
Given that TE is a transdiagnostic risk factor for the development of adverse psychiatric outcomes, developing interventions to promote a resilient response to TE is imperative. To properly do so, factors that influence one’s levels of resilience must be understood. The research base on the genetic influences on resilience is in its nascence (for a review see Ryan & Ryznar, Citation2022). As such, most of the knowledge about the genetic basis of resilience derives mainly from behavioural-genetic approaches (i.e. twin studies) which have demonstrated that resilience is moderately heritable (e.g. Amstadter et al., Citation2014; Wolf et al., Citation2018) and that heritability differs across the sexes (Amstadter et al., Citation2014; Boardman et al., Citation2008).
Twin studies have also examined shared genetic factors that contribute to the heritability of resilience and both internalizing and externalizing phenotypes. For example, Amstadter et al. (Citation2016) found that genetic influences on resilience accounted for 42% of the heritability of Major Depressive Disorder (MDD) and 61% of Generalized Anxiety Disorder (GAD), which was higher than for externalizing phenotypes (i.e. Alcohol Abuse/Dependence, 20%; Antisocial Personality Disorder, 18%; Amstadter et al., Citation2016). Similarly, Wolf et al. (Citation2018) demonstrated that PTSD and resilience were correlated at r = −0.59, and that 59% of the genetic correlation was attributable to a single genetic factor, with the remaining percentage attributable to a non-shared environmental factor, suggesting that genetic factors may contribute to a spectrum of traumatic stress response, reflecting resilience at one end and symptom severity at another.
There is a limited molecular literature on resilience, with the majority of these studies using a candidate gene approach (e.g. Azadmarzabadi et al., Citation2018; Bruenig et al., Citation2017). To date, there is only one genome-wide association study (GWAS) on resilience as defined by a self-report measure of perceived resilience (Stein et al., Citation2019). With a total of 11,492 European American (EA) participants, results revealed a genome-wide significant (GWS) locus on chromosome 4 in an intergenic region upstream to DCLK2 (doublecortin-like kinase 2) implicated in the promotion of survival and regeneration of injured neurons (Stein et al., Citation2019). At the GWS level, KLHL36 (Kelch like family member 36) was also associated with self-report levels of resilience. Additionally, a polymorphism near SLC15A5 (solute carrier family 15 member 5) met gene-wise genome significance when restricting analyses to those individuals endorsing high levels of deployment stress (N = 581).
Statistical-genetic innovations such as genome wide complex trait analysis (GCTA) and polygenic risk scores (PRS) allow for molecular-level investigations of psychiatric phenotypes. To date, only one study has applied GCTA and PRS in relation to resilience. Stein et al. (Citation2019) estimated the single-nucleotide polymorphism (SNP)-based heritability of self-assessed resilience (h2 = 0.16, SE = 0.050, p = 5.37 × 10−4). The authors also computed PRSs for self-reported resilience and did not find a significant correlation between self-assessed resilience and outcome-based resilience, as defined by a composite mental health outcome following deployment to Afghanistan though all self-report resilience scores were associated with higher odds for outcome-based resilience.
There is a need to better understand the molecular genetic underpinnings of resilience in order to inform therapeutic efforts for stress-related psychopathology. Not only is there a need to better understand these underpinnings, there is a need to examine them in diverse populations. As such, the present study leverages genome-wide data to better understand the molecular underpinnings of resilience, to estimate the molecular heritability of resilience, and to examine the genetic overlap between resilience with key outcomes (i.e. alcohol consumption, AUD, PTSD) that are seen following TE in EA and AA individuals. The first aim of the present study was to examine individual variants associated with resilience using GWAS, hypothesizing that there would be variants significantly associated with psychiatric resilience. Second, we examined the SNP-based h2 of resilience, hypothesizing that resilience would be minimally (e.g. 10–.20) heritable. Lastly, we examined the shared genetic risk between resilience and alcohol use phenotypes, PTSD, and subjective well-being with the hypothesis that there would be shared genetic risk between resilience and each of the subsequent phenotypes.
2. Methods
2.1. Participants
The sample included was leveraged from a larger cohort study at an urban, public university. At the time of this study, five cohorts (N = 12,365; ∼62% female, ∼50% European American [EA], ∼19% African American [AA], < 1% Native American, ∼6% Hispanic/Latino, ∼15% Asian, 6% Multi-racial, and <1% Native Hawaiian/Pacific Islander) which matriculated in the Fall 2011 (Cohort 1; N = 2,707), 2012 (Cohort 2; N = 2,481), 2013 (Cohort 3; N = 2,391), 2014 (Cohort 4; N = 2,310), and 2017 (Cohort 5; N = 2,476), had been enrolled in the study. The inclusion criterion required that participants endorsed experiencing TE at the baseline time-point (year-1 Fall, entry into college) variable (N = 6,634), provided genetic data, and identify as being of EA or AA ancestry. This study was approved by the university’s institutional review board.
2.2. Measures
2.2.1. Trauma exposure
Exposure to a TE was measured by an abbreviated version of the Life Events Checklist (LEC; Gray et al., Citation2004). The five-item LEC assesses exposure to a range of potentially-traumatic events, including exposure to a sexual assault, physical assault, natural disaster, motor vehicle accident, or other, unwanted sexual experience, with a ‘yes’ or ‘no’ response. At the baseline time-point (i.e. year-1 Fall), individuals report on lifetime types of traumas experienced before attending university. For the resilience variable, a sum score was used to capture the total load of TEs one experienced before attending university. In the current sample, participants endorsed an average of 1.75 traumatic events experienced before attending college (SD = 0.86, range: 0–4).
2.2.2. Internalizing symptoms
Internalizing symptoms were assessed at the baseline time-point using the Symptom Checklist-90 Short Version (SCL-27; Hardt & Gerbershagen, Citation2001). The assessment includes four items to assess depressive symptoms (e.g. ‘feeling low in energy or slowed down’; α = 0.89) and four items to assess anxiety symptoms (e.g. ‘nervousness or shakiness inside’; α = 0.85) over the past month. Responses were made on a Likert-type scale of 1 (not at all) to 5 (extremely). A sum score was computed, combining both depressive and anxiety symptoms, and used in the calculation of the resilience variable. For the present study, individuals, on average, reported a total anxiety and depressive symptom score of 15.8 (SD = 6.32, range: 8–40).
2.2.3. Resilience
The discrepancy-based measure of resilience utilized the phenotypic assessment of lifetime TE exposure and internalizing symptoms at baseline, modelled after other studies using a similar approach (e.g. Amstadter et al., Citation2014; Cusack et al., Citation2021). An individual’s total score on the SCL-27 was regressed onto their total LEC ‘lifetime’ sum (i.e. year 1 fall total endorsed TE range = 1–5). The residual (multiplied by −1 for ease of interpretation such that positive scores will represent degree of ‘resilient’ responding) was used to quantify resilience as the difference between a participant’s actual and expected functioning, based on the SCL sum score and LEC total, where higher scores represent greater levels of resilience.
2.3. DNA genotyping
For cohorts 1–3, samples were genotyped on the Axiom BioBank Array, Catalog Version 2. Cohort 4 samples were completed using the Smokescreen Genotyping Array at the Rutgers University Cell and DNA Repository (RUCDR) Infinite Biologics and cohort 5 was genotyped on the Affymetrix Axiom Precision Medicine Research Array. All cohorts were imputed from their separate arrays to a common 1000 Genomes platform.
Ancestry assignment was completed using principal component analysis with Mahalanobis distance calculations. Each participant was assigned to a 1000 Genomes Project (1KGP) population based on their minimum Mahalanobis distance. The samples were then collapsed into their respective super-population assignment, which were analysed separately and then meta-analysed. For the present study, only the AA and EA sub-samples were included, given that they were the two super populations with the highest number of individuals included. For a more detailed explanation of these methods, please see Peterson et al. (Citation2017).
The quality control pipeline excluded Off Target Variants found by SNPolisher samples missing >2% of genotypes and SNPs missing >5% of genotypes after sample filtering. Following QC removal, 560,138 variants remained for imputation. Filtering by Hardy-Weinberg (HWE), minor allele frequency (MAF), and relatedness were performed within the assigned super-populations.
2.4. Statistical analyses
GWAS. A GWAS was run using PLINK 2.0 (Chang et al., Citation2015) to identify variants associated with resilience, with ancestry PCs and sex included as covariates. The genomic inflation factor (λ) was estimated using R software (R Core Team, Citation2019), and the quantile-quantile (QQ) plots were used to determine and adjust for bulk inflation and excess false positives. False discovery rate (FDR) analyses were used to adjust for multiple testing in all analyses, and to determine what p-values are significant or suggestive of significance. Consistent with best practices suggested by the parent study workgroup (Webb et al., Citation2017), a threshold of 0.05 was used to determine significance. GWAS were conducted for the EA and AA sub-samples separately and subsequently meta-analysed using METAL (Willer et al., Citation2010), in order to account for low power due to small sample sizes.
GCTA. A GCTA was conducted to estimate the SNP-based heritability of resilience. GCTA estimates the heritability of a trait based off of the additive effect of all SNPs. This method creates a genetic relationship matrix based on SNPs for all individuals in the sample. The GRM is then used to predict phenotypic relatedness, resulting in an estimate of the variance in the trait that is due to each phenotype independently.
PRS. Summary statistics needed for generation of PRS scores came from large-scale genetic data that is publicly available to researchers (i.e. UK Biobank [alcohol consumption]; PGC-PTSD [PTSD]: PGC-SUD [AD]; SSGAC [well-being]; see for more details). To generate PRS, we:
Removed all ambiguous SNPs from the discovery GWAS (e.g. PGC-PTSD, UKBB, etc.)
Matched the discovery GWAS SNPS with 1000G phase 3 panel (n = 2,502) genotypes and target sample SNPs.
Linkage disequilibrium (LD) was corrected for by pruning variants nearby (500 kb) and in LD (r2 > 0.3) using 1000G reference panel genotypic information using the PLINK ‘prune’ method (i.e. keeping the SNPs with the lowest discovery GWAS p-value when pruning is needed) in a given region. We used 1000G for pruning instead of using the target sample genotypes in order to keep robust pruning for all discovery samples.
PRS were calculated using various p-value thresholds of the discovery GWAS in order to determine which p-value should be used in the prediction of resilience. PRS were generated using PRSice 2 (Choi & O'Reilly, Citation2019) at the following p-value thresholds: .001, .01, .05, .1, .2, .3, .4, .5, 1 using the target sample (i.e. Spit for Science) variant genotypes. The distribution of per-person polygenic risk was normalized by fitting to a standard normal distribution curve, to assist with interpretation of analyses.
Ancestry specific SNP-level summary statistics from each of the discovery datasets (AD, alcohol consumption, PTSD, well-being) were used to create score files using the GWAS data in the target sample to capture aggregate genetic risk. Each individual was scored for genetic risk for each phenotype by weighting risk alleles according to the natural log of the odds ratio from each of the discovery samples for specified p-value bins. Given that the optimal p-value is unknown a priori, PRS are calculated over a range of thresholds, and the association with the phenotype of interest tested for each. Once PRS were generated from the target dataset, the genetic overlap between resilience and each phenotype was determined using regression analyses, adjusting for sex and ancestry specific PCs. PRS analyses were conducted separately for EA and AA individuals. In other words, we employed PRS to examine cross-trait, aggregate genetic risk (i.e. does risk for PTSD calculated using summary statistics from the PGC-PTSD predict resilience in the target sample?).
3. Results
3.1. GWAS
Results were available for 3,379,382 markers for resilience (N = 6,634; EA = 4,594; AA = 2,040). Lambda values for resilience (λ = 0.977, SE = 6.04 × 10−6; ), were slightly below 1 indicating underinflation (i.e. p-values higher than would be expected by chance).
Figure 1. Manhattan Plot for Resilience (Meta-Analysed); Note: This figure plots the – log10(p) values of associations for resilience by chromosome. The red line represents genome-wide significance (p = 5 × 10−8), while the blue line indications a suggestive association threshold (p = 5 × 10−5).
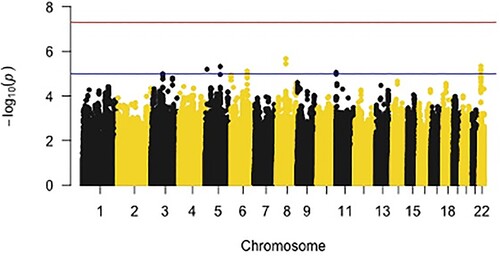
Figure 2. Quantile-quantile (QQ) plot for Meta-Analysed Findings; Note: The expected distribution of p-values is shown on the x-axis, while the observed distribution of p-values from GWAS of resilience is shown on the y-axis. All p-values are represented as – log10(P). The red and blue lines represent 95% confidence intervals.
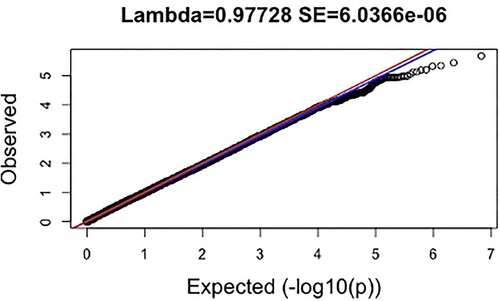
Findings from the meta-analysed GWAS demonstrated that zero SNPs met GWS, though nine passed the suggestive association threshold (p < 5 × 10−5; Figure and ; ). The largest number of suggestive SNPs from the GWAS (i.e. rs2018207 [z = 4.59, p = 4.53 × 10−6], rs7554264 [z = 4.48, p = 7.37 × 10−6], rs7290778 [z = −4.51, 4.64 × 10−6]) mapped onto the seizure related 6 homolog like (SEZ6L, chromosome 22) gene. Most of the SNPs nearby the SEZ6L gene were positively associated with resilience, meaning that increased copies of minor alleles for these SNPs were associated with higher levels of resilience. The LocusZoom plot of SEZ6L, demonstrated that two SNPs were in high LD (i.e. correlation between nearby variants) with the reference SNP (rs2018207).
Table 1. Summary information of SNPs meeting suggestive threshold (meta-analysed).
Two SNPs (rs10957272 [z = 4.74, p = 2.11 × 10−6], rs9969662 [z = −4.63, p = 3.60 × 10−6) were located in a cluster of genes (NKAIN3, GGH, TTPA, YTHDF3-AS1) found on chromosome 8. Findings suggest a mix of direction of influence with resilience such that rs10957272 was positively associated with resilience, indicating more copies of minor alleles as related to increased levels of resilience, and rs9969662 was negatively associated with resilience.
One SNP (rs74987153 [z = −4.48, p = 7.66 × 10−6) was located near the fyn related src family tyrosine kinase (FRK; chromosome 6) gene and one SNP (rs13162155 [z = −4.52, p = 6.31 × 10−6) was associated with the long intergenic non-protein coding RNA 2112 (LINC02112; chromosome 5) gene. Both of these SNPs were negatively related to resilience. Two SNPs (rs12719536 [z = −4.58, p = 4.75 × 10−6], rs6578251 [z = −4.45, p = 8.77 × 10−6]) were not associated with any one gene or cluster of genes on a region of chromosome 5 and 11 respectively.
3.2. GCTA
Results from the GCTA are shown in . Neither the EA (h2 = .118, SE = .100, p = .12) nor AFR (h2 = .166, SE = .278, p = .28) analyses yielded significant heritability estimates and both produced notable standard error estimates (e.g. Visscher & Goddard, Citation2015), suggesting inadequate power .
Table 2. Findings from GCTA of resilience in both sub-samples.
Table 3. Overview of the genetic databases from which summary statistics for PRS analyses were derived.
3.3. PRS
Alcohol Dependence (AD). AD PRS from the PGC-SUD did not significantly predict resilience in the EA nor AA sub-sample. Results demonstrated that, for the EA sub-sample, model fit was optimized at a p-value of .20. Nagelkerke’s pseudo-R2 value showed that PRS at this threshold explained a maximum variance of 0.06% in resilience (b = 2.93, SE = 2.27, p = .19). Similar patterns were found when examining the ability of AD PRS to predict resilience among the AA sub-sample whereby model fit was optimized at a p-value of .17. PRS from the AA sub-sample did not significantly predict resilience (b = −19.25, SE = 14.10, p = .17) with AD explaining a maximum variance of 0.16%.
Alcohol Consumption. PRS analyses for alcohol consumption were used to predict resilience only in the EA sub-sample, given that summary statistics for consumption from the UK BioBank include EA individuals only. Polygenic risk for alcohol consumption significantly predicted resilience (b = −46.80, SE = 19.81, p < .05, see ), explaining 0.19% of the variance in resilience in the EA sample. The direction of effect is as expected with summary stats for alcohol consumption being negatively related to resilience.
PTSD. For the EA sub-sample, there was no significant association between PTSD PRS and resilience (b = −1.92, SE = 1.72, p = .10). In the AA sub-sample, there was a significant association between PTSD PRS and resilience (b = 2.94, SE = 0.02, p < .05), though in the opposite direction than would be expected such that PTSD-PRS were positively related to resilience. With p-values optimized at .02, PTSD PRS explained 0.42% of the variance in resilience.
Well-being. PRS for subjective well-being were calculated using summary statistics from the SSGAC (Turley et al., Citation2018). Subjective well-being was assessed via a combination of measures assessing life satisfaction and positive affect (Okbay et al., Citation2016; Turley et al., Citation2018). The ability of the well-being PRS to predict resilience was tested in the EA sub-sample, as summary statistics are not available for AA samples. For the EA sub-sample, results demonstrated that model fit was optimized at a p-value of .07. There was no significant association between PRS for well-being and resilience (b = 49.39, SE = 38.57, p = .20), with the R2 showing that PRS at this p-value threshold explained a maximum variance of 0.11% of resilience.
4. Discussion
The present paper had three primary aims. The first was to examine independent SNPs associated with resilience using GWAS, the second was to determine the SNP-based heritability of resilience using GCTA, and the third was to examine the shared, aggregate genetic risk between resilience and psychiatric phenotypes via PRS. All three aims, taken together, contribute to better elucidating the genetic underpinnings of resilience. Additionally, to the best of our knowledge, this is the first study to investigate the genetic underpinnings of resilience in both EA and AA populations. Results provide insight into the genetic etiology of resilience in diverse populations.
4.1. Aim 1: GWAS
The suggestive SNPs from the meta-analysed GWAS mapped onto three genes: SEZ6L, LINC02112, and FRK, and one cluster of genes on chromosome 8. The largest number of SNPs (n = 3) were associated with the SEZ6L gene which is a protein-coding gene that contributes to specialized endoplasmic reticulum functions in neurons (Gorlov et al., Citation2007). SEZ6L has not been examined in relation to resilience. However, the SEZ6L gene is located on chromosome 22 at what is a ‘hotspot’ for bipolar disorder (BD; Xu et al., Citation2013). Genetic variants on chromosome 22 are associated with additional psychiatric disorders such as schizophrenia (Pramparo et al., Citation2008), as well as seizures in both animal and human studies. The finding that the SNPs in the SEZ6L gene were inversely associated with resilience is intuitive in the context of the gene’s association with psychiatric disorders in that we would expect a gene whose variants confer risk for various psychiatric disorders to be inversely related to resilience.
Two SNPs were associated with a cluster of genes (NKAIN3, GGH, TTPA, YTHDF3-AS1) on chromosome 8 implicated in the metabolization and transport of various vitamins and minerals such as Vitamin E, folic acid (Vitamin B), sodium, and potassium. Although not yet studied in relation to resilience, the role of vitamin deficiencies in mental health, more broadly, has been established. In their study of vitamin deficiencies and mental health symptoms, Ramsey and Muskin (Citation2013) found that both Vitamin B and E deficiencies are implicated in the development of various psychiatric symptoms and diagnoses (i.e. MDD, BD) perhaps via immflamation pathways. It is well-established that both trauma and PTSD are linked with increased inflammation (for a review, see Kim, Lee, & Yoon, Citation2020). Symptoms of PTSD typically prompt activation of the threat response, leading to heightened physiological arousal, and subsequently increasing levels of oxidative stress and inflammation (Miller et al., Citation2018). This suggestive hit also aligns with current GWAS of PTSD which demonstrate significant hits for genes implicated in the immune system (e.g. Sheerin et al., Citation2017).
Two SNPs (rs74987153, rs13162155) were inversely related to resilience. These SNPs were associated with the LINC02112 and the FRK genes, respectively. The potential relation of FRK to resilience is unknown as research on this gene has focused on cancer (e.g. Zhang et al., Citation2016; Bagu et al., Citation2017). LINC02112 is an RNA gene that research has not found to be clinically significant for any physical nor mental health diagnosis. As such, replication and future investigation into this gene in the context of resilience is warranted.
Only one other GWAS of resilience has been conducted. Results from Stein et al. (Citation2019) demonstrate that among their sample of EA soldiers, for self-reported resilience, two genes were detected at GWS, doublecortin-like kinase 2 (DCLK2) and kelch-like family member 36 (KLHL36). For outcome-based resilience, only one SNP on chromosome 12 downstream from solute carrier family 15 member 5 (SLC15A5) demonstrated GWS. This SNP met GWS only in a sample of soldiers who were exposed to the highest level of deployment stress. The non-replication of key variants in the present study is likely due to sample size and characteristics (i.e. civilians vs. soldiers, ancestral diversity in the present sample).
Although only one GWAS of resilience has been conducted to date, there is a literature examining the genetic underpinnings of traits that may not require adversity to be present, as resilience does, but could be beneficial when adversity occurs. As discussed in their research agenda for psychological resilience, Choi et al., (Citation2019) review numerous GWAS examining such traits. For example, research has identified GWS loci associated with factors such as positive affect (Wingo et al., Citation2017), subjective well-being (Okbay et al., Citation2016; Turley et al., Citation2018), and IQ (Davies et al., Citation2011; Trampush et al., Citation2017). Notably, findings from Wingo et al. (Citation2017) report two SNPs significantly associated with positive affect that are located in a locus for LINC01221, a gene that is implicated in findings from the present study, suggesting continued research investigating this specific gene and region in relation to resilience.
4.2. Aim 2: GCTA
Very modest estimates of heritability were found in both the EUR (n = 2,371) and AFR (n = 908) sub-samples, large SEs rendered these estimates non-significant, likely due to a lack of power. When analysed by ancestral sub-groups our sample sizes are below the reported standard sample size needed to detect moderate heritability estimates (Visscher et al., Citation2014). We were also underpowered to detect sex differences, and there are sex differences in the heritability of resilience whereby it is thought to be higher among men as compared to women (Boardman et al., Citation2008). Sex is a potential confound and may also impact the significance of findings.
Our GCTA analyses are inconsistent with findings from twin studies for resilience whereby resilience has been found to be moderately heritable (e.g. Amstadter et al., Citation2014; Wolf et al., Citation2018). However, GCTA uses aggregate SNP data and as such, cannot account for variation due to rare variants when calculating heritability (Trzaskowski et al., Citation2014). Further, GCTA heritability estimates only capture the additive-genetic effects, whereby twin modelling heritability estimates can capture both additive and nonadditive effects (Plomin et al., Citation2013), resulting in higher heritability estimates for traits, broadly.
Although non-significant, findings from the present study are consistent with the one GCTA on resilience to date. Stein et al. (Citation2019) did not find a significant heritability estimate for outcome-based resilience. However, with a sample size 9x larger than the one used in the present study, they estimated the SNP-based heritability of self-assessed resilience to be 0.162 (SE = 0.05, p = 5.37 × 10−4) in an EA sample. Heritability for resilience may be larger within some groups, such as those with greater TE. Indeed, Stein et al. (Citation2019) conducted GCTA in a sample of deployed soldiers, who have experienced TE at higher rates than the college student sample used in the present study (Johnson et al., Citation2014). The degree of TE an individual experiences interacts with genetic influences to increase the heritability of resilience, similar findings from Wolf et al. (Citation2018) that demonstrated that PTSD is more heritable at higher levels of trauma exposure.
4.3. Aim 3: PRS
The current study used PRS analyses to assess the value of polygenic risk for AD, alcohol consumption, PTSD, and subjective well-being in predicting resilience.
AD. There was no significant association between the AD PRS and resilience for either sub-sample. AD PRS accounted for 0.06% and 0.16% of the variance in resilience in the EUR and AFR sub-samples, respectively. To date, there is no extant literature examining PRS of alcohol related construct(s) in predicting resilience. However, the multivariate twin literature demonstrates that genetic influences on AUD and resilience are moderately correlated, with estimates around 57% (Long et al., Citation2017). As findings from the present study are inconsistent with the twin literature, it suggests that null findings are likely due to being underpowered. Indeed, when examining the sample sizes for the EUR and AFR sub-samples from the PGC-SUD, there were 8,233 cases in the EUR sub-sample, and 2,917 in the AFR sub-sample, as compared to, for example, 23,185 and 4,363 cases of PTSD respectively.
Alcohol Consumption. Findings demonstrate that alcohol consumption PRS are significantly associated with resilience in the EUR sub-group, explaining 0.19% of the variance in resilience. Although this R2 estimate may not seem substantial, it is consistent with the variance explained by PRS for alcohol consumption in the literature more broadly (e.g. 0.11% in drinking behaviours; Mies et al., Citation2018). The direction of the PRS effect on resilience was as expected such that polygenic risk for consumption was inversely associated with resilience. Although the R2 estimate is consistent with estimates in the literature more broadly, PRS from the present study explain much less than 1% of the variance in resilience. Given that analyses in the present study are notably underpowered, it is plausible that there is shared-genetic risk driving alcohol consumption and resilience that is not captured here.
To our knowledge, this is the first study to use PRS to examine the correlation between the genetic propensity for alcohol consumption and resilience. Most of the extant multivariate genetic literature has instead focused on the genetic correlation between resilience, trauma exposure, and PTSD in relation to AUD symptoms and AD (e.g. Sheerin et al., Citation2021; Long et al., Citation2017; Sartor et al., Citation2011). The self-medication literature largely supports the relationship between PTSD and AUD symptoms (e.g. Gaher et al., Citation2014), and provides less support for the relationship between PTSD and alcohol consumption (e.g. Possemato et al., Citation2015). Although the present study did not find a significant relationship between AD and resilience, the discovery sample size for the alcohol consumption summary statistics is significantly larger than that for AD, perhaps explaining discrepant findings.
PTSD. PTSD PRS were significantly associated with resilience in the AFR sub-sample accounting for 0.43% of the variance in resilience. However, PTSD PRS and resilience were positively associated, meaning that PRS for PTSD is linked to an increase in ‘risk’ for resilience (b = 2.94, SE = 2.59, p < .05). Although to date, no research has examined PRS for PTSD as predicting resilience, the direction of this finding contradicts the phenotypic literature which supports resilience as inversely related to PTSD symptoms and diagnostic status (e.g. Bensimon, 2012), with some researchers defining resilience as a lack of PTSD symptoms (e.g. Bonanno et al., Citation2007). The multivariate twin literature also provides support for the genetic propensity for PTSD as negatively related to the genetic propensity for resilience (e.g. r = −0.59; Wolf et al., Citation2018). One plausible explanation for the direction of this effect is based on the fact that those identifying as Black/African-American experience greater cumulative trauma exposure given systemic racism (e.g. Stevens-Watkins et al., Citation2014). As such, these individuals are required to ‘persevere through’ constantly, meaning that their genetic risk for PTSD is related to increased levels of resilience through increased trauma exposure, and required ‘perseverance’. It is also possible that the effect estimate was made positive via effects of natural selection whereby effect alleles occur at a higher frequency than non-effect alleles on average, thereby resulting in a PRS with a mean positive value (Choi & O'Reilly, Citation2019).
The discrepant findings between the EA and AA sub-samples is consistent with the literature on PTSD reporting racial differences in lifetime prevalence rates of PTSD (Black and/or African American [8.7%], non-Latino whites [7.4%]; Roberts et al., Citation2011). Assuming that the direction of the significant association of PTSD PRS and resilience is an artifact, it follows that, perhaps genetic risk for PTSD influences rates of PTSD in Blacks through impacting the genetic capability for resilience, but not for those identifying as white. However, using a genetic basis to explain higher rates of PTSD in Blacks is problematic due to the fact that although Black individuals report experiencing traumatic events at lower rates than white individuals (Roberts et al., Citation2011) these individuals exist in a society that is systemically racist. Although racial discrimination events are not yet considered Criterion A traumas, they have been shown to influence PTSD symptoms (Pieterse et al., Citation2010).
Subjective Well-Being. PRS were not significantly associated with resilience. This finding is inconsistent with extant research that examines the relation between resilience and well-being both phenotypically and genotypically. Well-being has been shown to be positively correlated with resilience on a phenotypic level (e.g. Bajaj & Pande, Citation2016; Satici, Citation2016). In their recent study using PRS to examine the common underlying genetic factors of well-being and resilience, de Vries et al., (Citation2021) found that PRS for well-being was a significant predictor of resilience, explaining 1.4–1.8% of the variance. The discrepancy between these findings is likely explained by sample size differences, whereby de Vries and colleagues included 10,876 participants and the present study included only 4,594.
4.4. Limitations and future directions
Findings should be considered in the context of a number of limitations. The largest limitation of all analyses conducted is low statistical power due to sample size. For example, with GWAS, the ability to detect less-common variants, increases with sample size (Teo, Citation2008). Although a larger sample size produces an increased number of ‘true positives’, false positives are also less likely to be found as sample size increases (Marigorta et al., Citation2018) given that power to detect a true effect is increased. Thus, another limitation related to sample size for the current study is the increased likelihood of suggestive hits being false positives.
In addition to sample size, phenotype of interest is an important variable to consider in the context of power. Sham and Purcell (Citation2014) note that most diseases have a complex genetic architecture involving various risk loci and environmental factors, but that this level of complexity differs based on the disease. Resilience is unique in that it is influenced by many contextual factors and thus requiring a larger sample size. Another challenge for the molecular literature on resilience is that it is defined in many different ways across the literature. For example, Stein et al. (Citation2019) study focused on self-report resilience whereas the present study used a discrepancy-based resilience phenotype. While the discrepancy-based resilience phenotype is a more refined phenotype, it requires precise measurement of both adversity and subsequent symptoms, both of which are likely influenced by genetic factors.
Follow-up studies are needed with adequate sample sizes for detecting GWS SNPs for resilience. Future research would also benefit from consensus in the field with regards to how to best measure resilience, in terms of both adversity exposure and the outcome variable. This consensus coupled with large, genetic consortia data on resilience would allow for the detection of GWS SNPs for resilience. The detection of GWS SNPs that are associated with resilience will further elucidate the molecular underpinnings of stress-related psychopathology, and specifically, will suggest variants that contribute to health and well-being, as opposed to disease. Identifying SNPs associated with resilience will allow for the development of targeted therapies, including psychotherapy and pharmacotherapy, that directly target the resilience-promoting pathways implicated via GWAS.
The Spit for Science Working Group: Director: Karen Chartier Co-Director: Ananda Amstadter. Past Founding Director: Danielle M. Dick. Registry management: Emily Lilley, Renolda Gelzinis, Anne Morris. Data cleaning and management: Katie Bountress, Amy E. Adkins, Nathaniel Thomas, Zoe Neale, Kimberly Pedersen, Thomas Bannard & Seung B. Cho. Data collection: Amy E. Adkins, Kimberly Pedersen, Peter Barr, Holly Byers, Erin C. Berenz, Erin Caraway, Seung B. Cho, James S. Clifford, Megan Cooke, Elizabeth Do, Alexis C. Edwards, Neeru Goyal, Laura M. Hack, Lisa J. Halberstadt, Sage Hawn, Sally Kuo, Emily Lasko, Jennifer Lend, Mackenzie Lind, Elizabeth Long, Alexandra Martelli, Jacquelyn L. Meyers, Kerry Mitchell, Ashlee Moore, Arden Moscati, Aashir Nasim, Zoe Neale, Jill Opalesky, Cassie Overstreet, A. Christian Pais, Kimberly Pedersen, Tarah Raldiris, Jessica Salvatore, Jeanne Savage, Rebecca Smith, David Sosnowski, Jinni Su, Nathaniel Thomas, Chloe Walker, Marcie Walsh, Teresa Willoughby, Madison Woodroof & Jia Yan. Genotypic data processing and cleaning: Cuie Sun, Brandon Wormley, Brien Riley, Fazil Aliev, Roseann Peterson & Bradley T. Webb.
Acknowledgments
We would like to thank Dr. Danielle Dick for founding and directing the Spit for Science Registry from 2011-2022, and the Spit for Science participants for making this study a success, as well as the many University faculty, students, and staff who contributed to the design and implementation of the project.
Disclosure statement
No potential conflict of interest was reported by the author(s).
Data availability statement
Data sharing is not applicable to this article as no new data were created or analysed in this study.
Additional information
Funding
References
- Amstadter, A. B., Maes, H. H., Sheerin, C. M., Myers, J. M., & Kendler, K. S. (2016). The relationship between genetic and environmental influences on resilience and on common internalizing and externalizing psychiatric disorders. Social Psychiatry and Psychiatric Epidemiology, 51(5), 669–678. https://doi.org/10.1007/s00127-015-1163-6
- Amstadter, A. B., Myers, J. M., & Kendler, K. S. (2014). Psychiatric resilience: Longitudinal twin study. British Journal of Psychiatry, 205(4), 275–280. https://doi.org/10.1192/bjp.bp.113.130906
- Azadmarzabadi, E., Haghighatfard, A., & Mohammadi, A. (2018). Low resilience to stress is associated with candidate gene expression alterations in the dopaminergic signalling pathway. Psychogeriatrics, 18(3), 190–201. https://doi.org/10.1111/psyg.12312
- Bagu, E. T., Miah, S., Dai, C., Spriggs, T., Ogunbolude, Y., Beaton, E., … Lukong, K. E. (2017). Repression of Fyn-related kinase in breast cancer cells is associated with promoter site-specific CpG methylation. Oncotarget, 8(7), 11442. doi: 10.18632/oncotarget.14546
- Bajaj, B., & Pande, N. (2016). Mediating role of resilience in the impact of mindfulness on life satisfaction and affect as indices of subjective well-being. Personality and Individual Differences, 93, 63–67. doi: 10.1016/j.paid.2015.09.005
- Benjet, C., Bromet, E., Karam, E., Kessler, R., McLaughlin, K., Ruscio, A., Shahly, V., Stein, D. J., Petukhova, M., Hill, E., Alonso, J., Atwoli, L., Bunting, B., Bruffaerts, R., Caldas-de-Almeida, J. M., de Girolamo, G., Florescu, S., Gureje, O., Huang, Y., … Koenen, K. (2016). The epidemiology of traumatic event exposure worldwide: Results from the world mental health survey consortium. Psychological Medicine, 46(2), 327–343. https://doi.org/10.1017/S0033291715001981
- Blanco, C., Xu, Y., Brady, K., Pérez-Fuentes, G., Okuda, M., & Wang, S. (2013). Comorbidity of posttraumatic stress disorder with alcohol dependence among US adults: Results from National Epidemiological Survey on Alcohol and Related Conditions. Drug and Alcohol Dependence, 132(3), 630–638. doi: 10.1016/j.drugalcdep.2013.04.016
- Boardman, J. D., Blalock, C. L., & Button, T. M. (2008). Sex differences in the heritability of resilience. Twin Research and Human Genetics, 11(1), 12–27. https://doi.org/10.1375/twin.11.1.12
- Bonanno, G. A., Galea, S., Bucciarelli, A., & Vlahov, D. (2007). What predicts psychological resilience after disaster? The role of demographics, resources, and life stress. Journal of Consulting and Clinical Psychology, 75(5), 671. doi: 10.1037/0022-006X.75.5.671
- Boscarino, J. A., Adams, R. E., & Galea, S. (2006). Alcohol use in New York after the terrorist attacks: a study of the effects of psychological trauma on drinking behavior. Addictive Behaviors, 31(4), 606–621. doi: 10.1016/j.addbeh.2005.05.035
- Chang, C. C., Chow, C. C., Tellier, L. C., Vattikuti, S., Purcell, S. M., & Lee, J. J. (2015). Second-generation PLINK: rising to the challenge of larger and richer datasets. Gigascience, 4(1), s13742–015. doi: 10.1186/s13742-015-0047-8
- Bruenig, D., Morris, C. P., Mehta, D., Harvey, W., Lawford, B., Young, R. M., & Voisey, J. (2017). Nitric oxide pathway genes (NOS1AP and NOS1) are involved in PTSD severity, depression, anxiety, stress and resilience. Gene, 625, 42–48. https://doi.org/10.1016/j.gene.2017.04.048
- Choi, S. W., & O'Reilly, P. F. (2019). PRSice-2: Polygenic risk score software for biobank-scale data. Gigascience, 8(7), giz082. doi: 10.1093/gigascience/giz082
- Choi, K. W., Stein, M. B., Dunn, E. C., Koenen, K. C., & Smoller, J. W. (2019). Genomics and psychological resilience: a research agenda. Molecular psychiatry, 24(12), 1770–1778. doi: 10.1038/s41380-019-0457-6
- Clarke, T. K., Adams, M. J., Davies, G., Howard, D. M., Hall, L. S., Padmanabhan, S., … McIntosh, A. M. (2017). Genome-wide association study of alcohol consumption and genetic overlap with other health-related traits in UK Biobank (N= 112 117). Molecular psychiatry, 22(10), 1376–1384. doi: 10.1038/mp.2017.153
- Davies, G., Tenesa, A., Payton, A. et al. (2011). Genome-wide association studies establish that human intelligence is highly heritable and polygenic. Mol Psychiatry, 16, 996–1005. https://doi.org/10.1038/mp.2011.85
- Cusack, S. E., Bountress, K. E., Sheerin, C. M., Dick, D. M., Amstadter, A. B., & Spit for Science Work Group. (2021). The longitudinal buffering effects of resilience on alcohol use outcomes. Psychological Trauma: Theory, Research, Practice, and Policy.
- de Vries, L. P., Baselmans, B. M., Luykx, J. J., de Zeeuw, E. L., Minică, C. C., de Geus, E. J., … Bartels, M. (2021). Genetic evidence for a large overlap and potential bidirectional causal effects between resilience and well-being. Neurobiology of Stress, 14, 100315. doi: 10.1016/j.ynstr.2021.100315
- Gaher, R. M., Simons, J. S., Hahn, A. M., Hofman, N. L., Hansen, J., & Buchkoski, J. (2014). An experience sampling study of PTSD and alcohol-related problems. Psychology of addictive behaviors, 28(4), 1013. doi: 10.1037/a0037257
- Galatzer-Levy, I. R., Huang, S. H., & Bonanno, G. A. (2018). Trajectories of resilience and dysfunction following potential trauma: A review and statistical evaluation. Clinical psychology review, 63, 41–55. doi: 10.1016/j.cpr.2018.05.008
- Gorlov, I. P., Meyer, P., Liloglou, T., Myles, J., Boettger, M. B., Cassidy, A., … Amos, C. I. (2007). Seizure 6-like (SEZ6L) gene and risk for lung cancer. Cancer research, 67(17), 8406–8411. doi: 10.1158/0008-5472.CAN-06-4784
- Gray, M. J., Litz, B. T., Hsu, J. L., & Lombardo, T. W. (2004). Psychometric properties of the life events checklist. Assessment, 11(4), 330–341. https://doi.org/10.1177/1073191104269954
- Hardt, J., & Gerbershagen, H. U. (2001). Cross-validation of the SCL-27: A short psychometric screening instrument for chronic pain patients. European Journal of Pain, 5(2), 187–197. https://doi.org/10.1053/eujp.2001.0231
- Ipser, J. C., Wilson, D., Akindipe, T. O., Sager, C., & Stein, D. J. (2015). Pharmacotherapy for anxiety and comorbid alcohol use disorders. Cochrane Database of Systematic Reviews, (1).
- Johnson, M. C., Graceffo, J. M., Hayes, J. A., & Locke, B. D. (2014). Examining treatment-seeking college students with and without military experience and trauma histories. Journal of College Counseling, 17(3), 260–270. doi: 10.1002/j.2161-1882.2014.00062.x
- Kim, T. D., Lee, S., & Yoon, S. (2020). Inflammation in post-traumatic stress disorder (PTSD): a review of potential correlates of PTSD with a neurological perspective. Antioxidants, 9(2), 107. doi: 10.3390/antiox9020107
- Long, E. C., Lönn, S. L., Ji, J., Lichtenstein, P., Sundquist, J., Sundquist, K., & Kendler, K. S. (2017). Resilience and risk for alcohol use disorders: a Swedish twin study. Alcoholism: Clinical and Experimental Research, 41(1), 149–155. doi: 10.1111/acer.13274
- Marigorta, U. M., Rodríguez, J. A., Gibson, G., & Navarro, A. (2018). Replicability and prediction: Lessons and challenges from GWAS. Trends in Genetics, 34(7), 504–517. https://doi.org/10.1016/j.tig.2018.03.005
- Mies, G. W., Verweij, K. J. H., Treur, J. L., Ligthart, L., Fedko, I. O., Hottenga, J. J., Willemsen, G., Bartels, M., Boomsma, D. I., & Vink, J. M. (2018). Polygenic risk for alcohol consumption and its association with alcohol-related phenotypes: Do stress and life satisfaction moderate these relationships? Drug and Alcohol Dependence, 183, 7–12. https://doi.org/10.1016/j.drugalcdep.2017.10.018
- Miller, M. W., Lin, A. P., Wolf, E. J., & Miller, D. R. (2018). Oxidative stress, inflammation, and neuroprogression in chronic PTSD. Harvard Review of Psychiatry, 26(2), 57–69. https://doi.org/10.1097/HRP.0000000000000167
- Nievergelt, C. M., Maihofer, A. X., Klengel, T., Atkinson, E. G., Chen, C. Y., Choi, K. W., … Stevens, J. S. (2019). International meta-analysis of PTSD genome-wide association studies identifies sex-and ancestry-specific genetic risk loci. Nature Communications, 10(1), 4558. doi: 10.1038/s41467-019-12576-w
- Okbay, A., Beauchamp, J. P., Fontana, M. A., Lee, J. J., Pers, T. H., Rietveld, C. A., … Rustichini, A. (2016). Genome-wide association study identifies 74 loci associated with educational attainment. Nature, 533(7604), 539–542. doi: 10.1038/nature17671
- Peterson, R. E., Edwards, A. C., Bacanu, S. A., Dick, D. M., Kendler, K. S., & Webb, B. T. (2017). The utility of empirically assigning ancestry groups in cross-population genetic studies of addiction. The American Journal on Addictions, 26(5), 494–501 doi: 10.1111/ajad.12586
- Pieterse, A. L., Carter, R. T., Evans, S. A., & Walter, R. A. (2010). An exploratory examination of the associations among racial and ethnic discrimination, racial climate, and trauma-related symptoms in a college student population. Journal of Counseling Psychology, 57(3), 255. doi: 10.1037/a0020040
- Plomin, R., Haworth, C. M., Meaburn, E. L., Price, T. S., Wellcome Trust Case Control Consortium 2, & Davis, O. S. (2013). Common DNA markers can account for more than half of the genetic influence on cognitive abilities. Psychological Science, 24(4), 562–568.
- Possemato, K., Maisto, S. A., Wade, M., Barrie, K., McKenzie, S., Lantinga, L. J., & Ouimette, P. (2015). Ecological momentary assessment of PTSD symptoms and alcohol use in combat veterans. Psychology of Addictive Behaviors, 29(4), 894. doi: 10.1037/adb0000129
- Pramparo, T., De Gregori, M., Gimelli, S., Ciccone, R., Frondizi, D., Liehr, T., Pellacani, S., Masi, G., Brovedani, P., Zuffardi, O., & Guerrini, R. (2008). A 7 Mb duplication at 22q13 in a girl with bipolar disorder and hippocampal malformation. American Journal of Medical Genetics Part A, 146A(13), 1754–1760. https://doi.org/10.1002/ajmg.a.32326
- R Core Team. (2019). R: A Language and Environment for Statistical Computing. R Foundation for Statistical Computing. URL http://www.R-project.org/.
- Ramsey, D., & Muskin, P. R. (2013). Vitamin deficiencies and mental health: How are they linked. Current Psychiatry, 12(1), 37–43.
- Roberts, A. L., Gilman, S. E., Breslau, J., Breslau, N., & Koenen, K. C. (2011). Race/ethnic differences in exposure to traumatic events, development of post-traumatic stress disorder, and treatment-seeking for post-traumatic stress disorder in the United States. Psychological medicine, 41(1), 71–83. doi: 10.1017/S0033291710000401
- Rosellini, A. J., Dussaillant, F., Zubizarreta, J. R., Kessler, R. C., & Rose, S. (2018). Predicting posttraumatic stress disorder following a natural disaster. Journal of Psychiatric Research, 96, 15–22. doi: 10.1016/j.jpsychires.2017.09.010
- Ryan, M., & Ryznar, R. (2022). The molecular basis of resilience: a narrative review. Frontiers in Psychiatry, 13. doi: 10.3389/fpsyt.2022.856998
- Sartor, C. E., McCutcheon, V. V., Pommer, N. E., Nelson, E. C., Grant, J. D., Duncan, A. E., … Heath, A. C. (2011). Common genetic and environmental contributions to post-traumatic stress disorder and alcohol dependence in young women. Psychological Medicine, 41(7), 1497–1505. doi: 10.1017/S0033291710002072
- Satici, S. A. (2016). Psychological vulnerability, resilience, and subjective well-being: The mediating role of hope. Personality and Individual Differences, 102, 68–73. doi: 10.1016/j.paid.2016.06.057
- Sham, P. C., & Purcell, S. M. (2014). Statistical power and significance testing in large-scale genetic studies. Nature Reviews Genetics, 15(5), 335–346. doi: 10.1038/nrg3706
- Sheerin, C. M., Lind, M. J., Bountress, K. E., Nugent, N. R., & Amstadter, A. B. (2017). The genetics and epigenetics of PTSD: overview, recent advances, and future directions. Current Opinion in Psychology, 14, 5–11. doi: 10.1016/j.copsyc.2016.09.003
- Sheerin, C. M., Bustamante, D., Bountress, K. E., Cusack, S. E., Aggen, S. H., Kendler, K. S., & Amstadter, A. B. (2021). Psychiatric resilience and alcohol resistance: A twin study of genetic correlation and Sex differences. Behavior Genetics, 35(2), 1–12. https://doi.org/10.1002/da.22700
- Shorter, D., Hsieh, J., & Kosten, T. R. (2015). Pharmacologic management of comorbid post-traumatic stress disorder and addictions. The American Journal on Addictions, 24(8), 705–712. doi: 10.1111/ajad.12306
- Stein, M. B., Choi, K. W., Jain, S., Campbell-Sills, L., Chen, C. Y., Gelernter, J., He, F., Heeringa, S. G., Maihofer, A. X., Nievergelt, C., Nock, M. K., Ripke, S., Sun, X., Kessler, R. C., Smoller, J. W., & Ursano, R. J. (2019). Genome-wide analyses of psychological resilience in U.S. Army soldiers. American Journal of Medical Genetics Part B: Neuropsychiatric Genetics, 180(5), 310–319. https://doi.org/10.1002/ajmg.b.32730
- Stevens-Watkins, D., Perry, B., Pullen, E., Jewell, J., & Oser, C. B. (2014). Examining the associations of racism, sexism, and stressful life events on psychological distress among African-American women. Cultural Diversity and Ethnic Minority Psychology, 20(4), 561. doi: 10.1037/a0036700
- Trampush, J. W., Yang, M. L. Z., Yu, J., Knowles, E., Davies, G., Liewald, D. C., … & Lencz, T. (2017). GWAS meta-analysis reveals novel loci and genetic correlates for general cognitive function: a report from the COGENT consortium. Molecular Psychiatry, 22(3), 336–345. doi: 10.1038/mp.2016.244
- Trzaskowski, M., Yang, J., Visscher, P. M., & Plomin, R. (2014). DNA evidence for strong genetic stability and increasing heritability of intelligence from age 7 to 12. Molecular Psychiatry, 19(3), 380–384. doi: 10.1038/mp.2012.191
- Teo, Y. Y. (2008). Common statistical issues in genome-wide association studies: a review on power, data quality control, genotype calling and population structure. Current Opinion in Lipidology, 19(2), 133–143. doi: 10.1097/MOL.0b013e3282f5dd77
- Turley, P., Walters, R. K., Maghzian, O., Okbay, A., Lee, J. J., Fontana, M. A., Nguyen-Viet, T. A., Wedow, R., Zacher, M., Furlotte, N. A., Magnusson, P., Oskarsson, S., Johannesson, M., Visscher, P. M., Laibson, D., Cesarini, D., Neale, B. M., & Benjamin, D. J. (2018). Multi-trait analysis of genome-wide association summary statistics using MTAG. Nature Genetics, 50(2), 229–237. https://doi.org/10.1038/s41588-017-0009-4
- Visscher, P. M., & Goddard, M. E. (2015). A general unified framework to assess the sampling variance of heritability estimates using pedigree or marker-based relationships. Genetics, 199(1), 223–232. https://doi.org/10.1534/genetics.114.171017
- Visscher, P. M., Hemani, G., Vinkhuyzen, A. A., Chen, G. B., Lee, S. H., Wray, N. R., … Yang, J. (2014). Statistical power to detect genetic (co) variance of complex traits using SNP data in unrelated samples. PLoS Genetics, 10(4), e1004269. doi: 10.1371/journal.pgen.1004269
- Walters, R. K., Polimanti, R., Johnson, E. C., McClintick, J. N., Adams, M. J., Adkins, A. E., … Porjesz, B. (2018). Transancestral GWAS of alcohol dependence reveals common genetic underpinnings with psychiatric disorders. Nature Neuroscience, 21(12), 1656–1669. doi: 10.1038/s41593-018-0275-1
- Webb, B. T., Edwards, A. C., Wolen, A. R., Salvatore, J. E., Aliev, F., Riley, B. P., … Adkins, A. (2017). Molecular genetic influences on normative and problematic alcohol use in a population-based sample of college students. Frontiers in Genetics, 8, 30. https://doi.org/10.3389/fgene.2017.00030
- Willer, C. J., Li, Y., & Abecasis, G. R. (2010). METAL: fast and efficient meta-analysis of genomewide association scans. Bioinformatics, 26(17), 2190–2191. doi: 10.1093/bioinformatics/btq340
- Windle, G. (2011). What is resilience? A review and concept analysis. Reviews in Clinical Gerontology, 21(2), 152–169. doi: 10.1017/S0959259810000420
- Wingo, A. P., Briscione, M., Norrholm, S. D., Jovanovic, T., McCullough, S. A., Skelton, K., & Bradley, B. (2017). Psychological resilience is associated with more intact social functioning in veterans with post-traumatic stress disorder and depression. Psychiatry Research, 249, 206–211 doi: 10.1016/j.psychres.2017.01.022
- Xu, C., Mullersman, J. E., Wang, L., Su, B. B., Mao, C., Posada, Y., … Wang, K. S. (2013). Polymorphisms in seizure 6-like gene are associated with bipolar disorder I: evidence of gene× gender interaction. Journal of Affective Disorders, 145(1), 95–99. doi: 10.1016/j.jad.2012.07.017
- Wolf, E. J., Miller, M. W., Sullivan, D. R., Amstadter, A. B., Mitchell, K. S., Goldberg, J., & Magruder, K. M. (2018). A classical twin study of PTSD symptoms and resilience: Evidence for a single spectrum of vulnerability to traumatic stress. Depression and Anxiety, 35(2), 132–139. https://doi.org/10.1002/da.22712
- Zhang, X., Li, F., & Zhu, L. (2016). Fyn-related kinase expression predicts favorable prognosis in patients with cervical cancer and suppresses malignant progression by regulating migration and invasion. Biomedicine & Pharmacotherapy, 84, 270–276. doi: 10.1016/j.biopha.2016.09.042