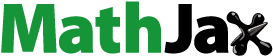
ABSTRACT
Background: Analysis of dynamic (temporal) networks allows an identification of important targets of treatment.
Objective: This study examined the dynamic network of symptoms in patients diagnosed with post-traumatic stress disorder (PTSD) during exposure therapy.
Method: Patients (n = 65) were randomized to either standard prolonged exposure, which includes imaginal exposure to the traumatic memory, or modified prolonged exposure, where imagery re-scripting of the memory replaced imaginal exposure, in a 10-week residential program. They completed a measure of DSM-IV PTSD symptoms weekly. The multilevel vector autoregressive (mlVAR) model was used to analyse the data, producing a temporal (dynamic), contemporaneous, and between-person network.
Results: Physiological reactivity to reminders in a given week was positively related to distress reactivity and to flashbacks the subsequent week. Hypervigilance one week was positively related to startle response and external avoidance the subsequent week. In addition, sleep problems were positively predicted by previous week internal avoidance and negatively predicted by previous week flashbacks. Hypervigilance and physiological reactivation had the highest out-strength, indicating that they were the most predictive of other symptoms.
Conclusions: The present within-person results make a preliminaryrect basis for the recommendation to monitor and facilitate change in physiological reactivation and hypervigilance in the treatment of PTSD. Future studies should examine the replicability of our temporal PTSD network and also include causal variables beyond symptoms.
HIGHLIGHTS
• Dynamic networks of PTSD symptoms may reveal useful targets of treatment.• Hypervigilance and physiological reactivity to reminders were most predictive.• Physiological reactivity predicted subsequent distress reactivity and flashbacks.• Hypervigilance predicted subsequent startle response and external avoidance.
Objetivo: Este estudio examinó la red dinámica de síntomas en pacientes diagnosticados con trastorno de estrés postraumático (TEPT) durante la terapia de exposición.
Método: Los pacientes (n = 65) se asignaron al azar a una exposición prolongada estándar, que incluye la exposición imaginaria al recuerdo traumático, o la exposición prolongada modificada, donde la re-escritura imaginaria del recuerdo reemplazó a la exposición imaginaria, en un programa residencial de 10 semanas. Ellos completaron una medición de los síntomas del TEPT según DSM-IV semanalmente. El modelo de vectores autorregresivos multinivel (mlVAR) se usó para analizar los datos, produciendo una red temporal (dinámica), contemporánea y entre personas.
Resultados: La reactividad fisiológica a los recordatorios en una semana determinada se relacionó positivamente con la reactividad de angustia y con los flashbacks de la semana siguiente. La hipervigilancia de una semana se relacionó positivamente con la respuesta de sobresalto y la evitación externa la semana siguiente. Además, los problemas de sueño fueron predichos positivamente por la evitación interna de la semana anterior y predichos negativamente por los flashbacks de la semana anterior. La hipervigilancia y la reactivación fisiológica tuvieron la mayor resistencia, lo que indica que fueron los más predictivos de otros síntomas.
Conclusiones: Los resultados actuales intra-sujeto constituyen una base preliminar para la recomendación de monitorear y facilitar el cambio en la reactivación fisiológica y la hipervigilancia en el tratamiento del trastorno de estrés postraumático. Los estudios futuros deberían examinar la replicabilidad de nuestra red temporal de TEPT y también incluir variables causales más allá de los síntomas.
目的:本研究检查了暴露治疗期间创伤后应激障碍(PTSD)患者的症状动态网络。
方法:在10周的住院计划中,患者(n = 65)被随机分配到标准的延长暴露疗法,其中包括想象性暴露于创伤记忆或改变后的延长暴露,其中图像重写记忆取代了图像暴露。他们每周完成一系列DSM-IV PTSD症状的测评。多水平向量自回归(mlVAR)模型用于分析数据,产生时间(动态),同期和被试间网络。
结果:在一周内对提醒线索的生理反应性与遇险反应性和随后一周的闪回呈正相关。一周的过度警惕与随后一周的惊吓反应和外部回避呈正相关。此外,睡眠问题通过前一周的内部回避得到了积极的预测,并且在前一周的倒叙中得到了负面的预测。过度警觉和生理恢复具有最高的强度,表明它们是其他症状中最具预测性的。
结论:生理性再激活和高警觉的变化可以作为PTSD治疗中监测和促进的靶点,目前的被试间结果为其提供了初步支持基础。未来的研究应该考察我们的PTSD时间网络的可重复性,和症状以外的因果变量。
1. Introduction
Post-traumatic stress disorder (PTSD) is a debilitating condition that has been found to affect about 7% of the population during the course of life (Kessler et al., Citation2005). Several trauma-focused cognitive behavioural treatments have proved to be effective (NICE, Citation2005). However, on average 46% of the completers have been found not to reliably improve (Bradley, Greene, Russ, Dutra, & Westen, Citation2005) from the most widely documented treatment, prolonged exposure (PE; Foa, Hembree, & Rothbaum, Citation2007). A progress toward optimizing PTSD treatment would be to examine how existing PTSD treatments work. Identification of central mechanisms of change could provide a basis for more focused, reliable and effective interventions.
Mechanistic studies of PTSD have so far adhered to a latent variable approach. That is, both PTSD and mechanism variables have been treated as latent constructs, referring to underlying entities causing observable symptoms or indicators (Edwards & Bagozzi, Citation2000). Because latent entities cannot be observed and measured directly, sum-scores of their indicators are used to estimate the level of a latent variable. Using this approach, Zalta et al. (Citation2014) found that bi-weekly change in negative cognitions associated with PTSD positively predicted subsequent PTSD symptom change. Using an ecological momentary assessment (EMS) approach, Short, Allan, and Schmidt (Citation2017) found that within-person variations in quality and efficiency of sleep-predicted variations in PTSD symptom severity. However, the latent variable perspective adopted in these studies has several limitations. First, the assumption that symptoms are only passive effects and have no causal interactions among each other are untenable. For instance, intrusive re-experiencing as a response to trauma reminders tends to strengthen avoidance of those reminders. Second, the focus on latent entities leads to global and not very specific and actionable clinical recommendations (e.g., ‘reduction of belief in trauma-related negative cognitions will improve PTSD’).
An alternative network approach to psychopathology treats disorder constructs as referring to a network of causally interacting symptoms (Borsboom, Citation2017; McNally, Citation2016). Instead of symptoms being viewed as passive effects of underlying entities, they are taken to be autonomous causes of each other. In this view, a clinical disorder such as PTSD is maintained by the causal connections between external triggers, symptoms and other relevant observable variables. As mechanisms can be defined as activities and processes explaining the connections between the elements of the network, a first step in mechanism research according to a network perspective is to establish what the connections among symptoms are. Most network studies of PTSD to date have examined cross-sectional networks (see Greene, Gelkopf, Epskamp, & Fried, Citation2018, for an overview), which are less relevant for identifying dynamic interactions of symptoms over time. Only one study has explored the temporal dynamics of PTSD symptoms, using intensive longitudinal data collected twice a day in a general population sample during a period of conflict (Greene et al., Citation2018). The authors analysed the data with multilevel network analysis, producing three types of networks: temporal network, contemporaneous network, and between-person network. From a dynamic perspective, the temporal and contemporaneous networks usually carry most information. The temporal network depicts the lagged associations of symptoms from 1 point of time to the next within patients. The residuals of the temporal analysis are used to construct the contemporaneous network, that is, the relationships between symptoms at the same occasion. Thus, both networks reflect within-person relationships and is therefore of particular relevance for therapy theories and the study of mechanisms of maintenance and change. This is because the mechanisms depicted in such theories concern within-person relationships, that is, how variables (e.g., catastrophic interpretations of bodily sensations and panic attacks) causally interact within patients over time. Consequently, it is also these two types of networks that provide clinical implications. In particular, nodes with high outstrength in the temporal network are targets for potentially effective interventions as changes in such nodes are likely to propagate through the network. For this network, Greene et al. found that exaggerated startle response had the far greatest out-strength. This centrality measure represents the number of significant temporal connections extending out from the symptom, excluding autocorrelations. The symptoms with the highest in-strength centrality – the extent to which symptoms are influenced by other symptoms in the network at the previous measurement – were sleep disturbance and loss of interest. However, no study has examined the temporal network of symptoms among PTSD patients, either within or outside treatment.
The purpose of the present exploratory study was to examine the dynamic network of symptoms in patients diagnosed with PTSD during exposure therapy. The results may shed light on which PTSD symptoms are promising targets for clinical interventions. The patients were randomly assigned to receive either PE, which includes imaginal exposure (IE), or modified PE, where imagery rescripting (IR; Smucker, Citation2005) replaced IE as the imagery component of PE in a 10-week residential program. The outcome of the two treatments is reported elsewhere, showing few differences between them at post-treatment and one-year follow-up (Langkaas et al., Citation2017).
2. Methods
2.1. Participants
Participants were recruited from patients admitted for treatment of PTSD at a National clinic (Langkaas et al., Citation2017). The clinic offers residential treatment for non-psychotic patients seeking specialized treatment not available locally or for patients who have not responded adequately to local treatment or standard outpatient care. The inclusion criteria for being admitted for PTSD treatment (regulated by the clinic) were (a) having a presenting problem corresponding to diagnostic criteria (DSM-IV) for PTSD, (b) PTSD identified as the primary diagnosis in need of treatment, (c) age between 18 and 67 years, and (d) accepting withdrawal of all psychotropic medication (referred patients usually have received medication without effect). Exclusion criteria (regulated by the clinic) were (a) experiencing the ongoing threat of harm (e.g. still in an abusive relationship), (b) currently psychotic, (c) currently suicidal, (d) current active substance abuse, or (d) having extensive dissociative symptoms. The inclusion and exclusion criteria from the clinic were also used for the study (registered in Clinical Trials NCT00891098). From December 2008 to November 2010, 71 patients were assessed and admitted for PTSD treatment at the clinic. The study was approved by the Norwegian Regional Ethics Committees for Medical and Health Research Ethics (REC) and the patients gave informed consent after the procedure had been fully explained. All who consented were assessed for PTSD again by the research staff and all were confirmed to meet diagnostic criteria. Those who did not consent received treatment without participating in the study. Of the 67 patients randomly allocated to treatment condition, two lost their eligibility after randomization and were removed from the analysis. The intention-to-treat (ITT) sample thus consisted of 65 patients. Sixty-one patients (94%) completed allocated treatment as planned.
The ITT sample consisted of 38 women (58%) and 27 men (42%). The mean age was 45.2 years (SD = 9.7) and the mean length of time since the index trauma were 17.5 years (SD = 13.3). The most prevalent index traumas among the 38 women were nonsexual assault by a familiar person (n = 12; 31.6%), sexual assault by a familiar person (n = 9; 23.7%), and sexual assault by a stranger (n = 8; 21.1%). Among the 27 men, war-related trauma was most frequent (n = 7; 25.9%), followed by assault by a familiar person (n = 6; 22.2%) and accidents (n = 4; 14.8%). Over half the index traumas were prolonged events (a threatening situation not resolved within one day) or repeated traumas (n = 36; 55.4%).
Among the 65 patients, 61 (94%) met criteria for additional DSM-IV Axis I disorders. Most prevalent were other anxiety disorders (n = 54; 83%), mood disorders (n = 50; 77%) and alcohol/substance disorders (n = 19; 29%). Twenty-seven (42%) patients met criteria for at least one Axis II disorder, Cluster C disorders being most prevalent (n = 21; 32%). More details about the methods and sample are reported elsewhere (Langkaas et al., Citation2017).
2.2. Measure
The PTSD Symptom Scale-Self-Report (PSS-SR; Foa, Riggs, Dancu, & Rothbaum, Citation1993) is a self-report measure consisting of 17 items corresponding to the DSM-IV PTSD symptoms. This measure is usually rated for the last week, but the rating period was shortened to the last three days in this study. The frequency part of the criteria was changed correspondingly (0 = not at all, 1 = 1 time/sometimes, 2 = 2 times/half of the time, 3 = 3 or more times/almost always.
2.3. Procedure
The PSS-SR was completed every Monday morning. The patients were asked to base their ratings on their experiences during the last three days.
2.4. Experimental design and treatment
The modified PE manual included the same treatment elements as PE manual (Foa et al., Citation2007), except for imagery interventions, where the modified PE condition followed the procedure described in the IR treatment manual (Smucker, Citation2005).
Both treatments consisted of 10 sessions lasting between 90 and 120 min and were delivered over 10 weeks. The first two sessions were identical, involving education about trauma and overcoming PTSD and planning in vivo exposure assignments. Imagery interventions were part of sessions 3 to 10. Between each session, patients were given homework assignments to work on every day, consisting of in vivo exposure assignments and listening to audio recordings of the last session.
Treatment was delivered by two experienced therapists. The clinical staff also consisted of four psychiatric nurses assisting the patients in treatment assignments between sessions. The staff had a minimum of 10 years’ experience of delivering CBT for anxiety disorders and all had received CBT training and certification provided by the Norwegian Association for Cognitive Therapy.
2.5. Treatment fidelity
The therapists and clinical staff were trained and supervised by Mervin R. Smucker in delivering IR and Elizabeth A. Hembree in delivering PE. All patient sessions were videotaped for use in supervision and treatment fidelity checks. The therapists received weekly supervision during the trial.
On a 0 to 1 scale, the mean adherence ratings across 48 rated cases were .77 (SD = .18) for PE and .86 (SD = .19) for modified PE. On a 1 to 5 scale, mean adequacy ratings were 2.96 (SD = 1.30) for PE and 3.76 (SD = 1.27) for IR, corresponding to levels of satisfactory and close to good, respectively.
2.6. Statistical analysis
To analyse our longitudinal symptom data, we used the multilevel vector autoregressive (mlVAR) model, which is currently the most often used method to analyse multivariate time series in multiple subjects (Bringmann et al., Citation2013; Epskamp, Waldorp, Mõttus, & Borsboom, Citation2018). In time series data, consecutive responses are not likely to be independent (e.g., sleep problems at one-time point predict sleep problems at the next), thus violating a typical statistical assumption. The autoregressive (AR) part of mlVAR accounts for this time dependency within an individual by regressing a variable at time t on a lagged (measured at the previous time point, t-1) version of that same variable. The VAR model is a multivariate extension of the AR model. In VAR, variables are regressed on a lagged version of the same variable and all other variables of the multivariate set. Finally, the multilevel (ml) extension of the VAR allows the modelling of time dynamics across individuals. In mlVAR, each subject is assumed to have their own VAR model, and the VAR parameters are assumed to vary randomly across individuals. The average parameters represent the fixed effects, and particular individuals’ deviations from these fixed effects are called random effects. The fixed effects inform of the average intraindividual effects. The variances and covariances of the random effects tell about individual differences.
For our analysis, the limited sample size and power of the study made us reduce the number of symptom variables analysed. Of the 17 Diagnostic and Statistical Manual, version IV (DSM-IV; American Psychiatric Association, Citation1994) symptoms rated on our measure, we selected the eight items deemed most important by the developers of the more narrow International Classification of Diseases, version 11 (ICD-11; First, Reed, Hyman, & Saxena, Citation2015) criteria for PTSD, that is, distressing dreams, flashbacks, distress reactivity, physiological reactivity, internal avoidance, external avoidance, hypervigilance, and exaggerated startle response. Because sleep problems have been found to predict the sum of other PTSD symptoms on a within-person level (Short et al., Citation2017), it was included as the ninth symptom studied.
The mlVAR presupposes that the time series for each subject are stationary, that is, that their means and variances-covariances are stable over time. However, during treatment, the aim is to reduce to symptoms, which also happen in most patients. To make the time series of symptoms trend stationary, we detrended the data. That is, each person’s time series was regressed on time and the residuals were saved for further analyses. Then, we used the Kwiatkowski-Phillips-Schmidt-Shin (KPSS; Kwiatkowski, Phillips, Schmidt, & Shin, Citation1992) test to examine the level stationarity of these residuals, that is, whether they had a stable variance-covariance structure over time. The test was conducted separately for each of the 65 patients and the 9 symptoms variables per patient using the R packages tseries 0.10–43. The KPSS test indicated all the time-series was level stationary. Next, the person-mean of each symptom across assessment was computed and these means and the residuals computed in the first step were summed. Thus, the resulting score represents both a person’s mean for a symptom over time and the time-specific deviations from this mean, controlled for any person-specific linear time trend.
The predictors of the subjects’ VAR models are by default person-mean centred to disentangle within-person from between-person processes. Thus, a within-person (WP) component of the predictors is singled out. Because fitting the mlVAR directly to the multivariate time series is challenging due to the high number of parameters to estimate, a series of easier to compute univariate models are estimated. For illustration, let us consider the regression equation for the criterion variable DRM in our analyses ( lists the studied symptoms with abbreviations):
Table 1. The means (SDs) for the 9 studied PTSD symptoms at the first and the last week.
From the univariate models, a 9-by-9 matrix with the fixed effects βkj (k, j = 1,….,9, k = 0 denotes the intercept) is constructed. The matrix represents the dependence of the 9-dimensional state (i.e., DRM, fla, dis, phy, avi, ave, slp, hyp, str) of a typical individual (i.e., for which the random effect bkpj = 0) upon the previous 9-dimensional state. A specific element expresses the extent to which disaggregated variable k at time point t-1 is related to variable j at time t, while controlling for all other variables. The elements of the diagonals are the autoregressive effects (self-loops), while the off-diagonal elements are the cross-regressive effects (connections between different variables).
The matrix contains the fixed effects of the mlVAR model and represents the lag 1-links between the nodes (i.e., the variables). Thus, the matrix can be thought of as the population structure of the temporal network. In correlated estimation, the covariances between parameters within each of the univariate models are estimated. This is feasible for up to about six nodes. For our 9 nodes, orthogonal estimation was applied, in which the parameter covariances within each of the univariate models are fixed to zero.
In the same analysis, the mlVAR also estimates a between-subjects network by investigating the between-person prediction of the person-mean of one variable given the means of other variables. In a second step, the residuals of this first analysis are used to construct a contemporaneous network. The correlations between the residuals represent the relationships between the variables in the same window of measurement that cannot be explained by the temporal effects. Univariate multilevel regression analyses are conducted to predict each residual from all other residuals at the same occasion (measurement window). The resulting partial correlations are used to construct the contemporaneous network. Links without arrowhead between two nodes indicate the partial correlation obtained after controlling for both temporal effects and all other variables in the same window of measurement. In the analyses, a significance level of .05 for the individual effect was used without correction for multiple testing.
Finally, network characteristics were assessed in the form of centrality indices (Opsahl, Agneessens, & Skvoretz, Citation2010). Out-strength centrality indicates the extent to which a node influences other nodes. It was calculated as the sum of edge weights extending out from the node. In-strength centrality indicates the extent to which a node is influenced by other nodes. It was calculated as the sum of edge weights coming into the node. The centrality indices were calculated based on absolute values of the significant pathways. The free statistical software R (R Core Team, Citation2017) was used for the analyses. The mlVAR package (version 0.3.3) was used to estimate the three networks (Epskamp et al., Citation2018) and the qgraph package (Epskamp, Cramer, Waldorp, Schmittmann, & Borsboom, Citation2012) were used to visualize them. The R code used is reported in the supplemental online material.
3. Results
3.1. Descriptive statistics for the weekly symptoms
All the 65 patients of the intent-to-treat sample filled in the process measure for 5 weeks or more and were included in the analyses, resulting in 727 completed questionnaires. A majority (n = 60) provided ratings for at least 10 weeks. The shortened series reflect that three patients dropped out during treatment. Twenty-six patients had their stay extended because of a three-weeks summer vacation and completed measures for 13 to 14 weeks. Within the 727 questionnaires, there were occasional missing completions of items, amounting to 26 in number. The means (SDs) on the symptom variables at the first and the last observation are reported in .
3.2. Networks
The temporal network in shows the significant associations from one week to the next. In general, the three DSM-IV clusters of re-experiencing symptoms, avoidance symptoms, and hyperarousal symptoms collapsed to two clusters of related symptoms: the re-experiencing symptoms and the hyperarousal symptoms together with external avoidance. One symptom – sleep problems – changed affiliation from the hyperarousal cluster to the re-experiencing cluster and was predicted by internal avoidance. One re-experiencing symptom – distressing dreams – showed no relationship to other symptoms. Within the re-experiencing cluster, physiological reactivity predicted flashbacks and distress. That is, when physiological reactivation for a given patient was higher than usual for that patient, subsequent flashbacks and distress were also higher than ususal for him/her. Moreover, more than usual internal avoidance one week was associated with more than usual sleep problems next week. By contrast, more than usual flashbacks one week was connected with less than usual sleep problems the next week. Within the hyperarousal cluster, more than usual hypervigilance predicted more than usual startle response and avoidance of reminders the subsequent week. Seven of the nine symptoms had self-loops, all were negative.
Figure 1. The temporal network of PTSD symptoms. Arrows represent temporal prediction. Thickness of an edge represents the strength of connection. Green edges represent positive connections and red edges represent negative connections.
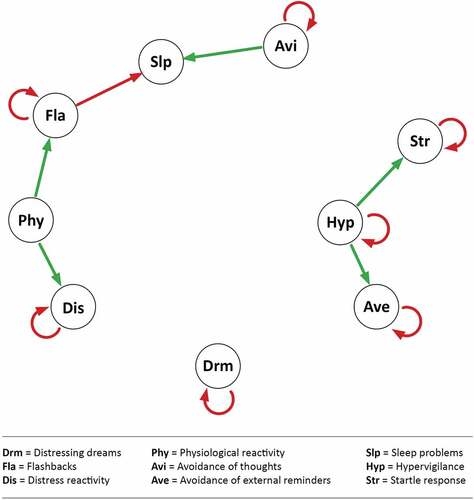
With regard to centrality, physiological reactivation and hypervigilance had high out-strength (1.4 and 1.7, respectively), whereas sleep problems had high in-strength (1.9).
The contemporaneous network depicted in showed two clusters of connected symptoms. The DSM-IV re-experiencing and avoidance symptoms formed one cluster and the DSM-IV hyperarousal symptoms the other. The two clusters were linked through a connection between physiological reactivity and sleep problems. Consistent with the temporal network, strong connections between hypervigilance and startle response and between distress and physiological reactivity appeared. Beyond the temporal network, contemporaneous connections between internal and external avoidance and between flashbacks and dreams appeared.
Figure 2. The contemporaneous network of PTSD symptoms. Thickness of an edge represents strength of connection. Green edges represent positive connections and red edges represent negative connections.
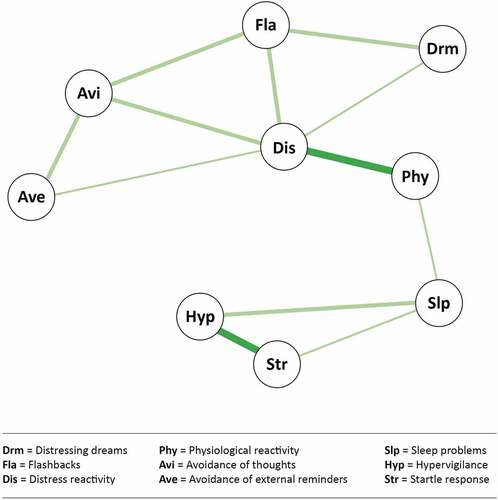
In the between-person network (), the re-experiencing symptoms (together with sleep problems) and the hyperarousal symptoms formed two clusters, linked by avoidance symptoms. People who, on average, had higher startle response also, on average, had higher hypervigilance. Strong between-person relationships also occurred between internal and external avoidance, between bad dreams and sleep problems, and between distress and physiological reactivity.
4. Discussion
The main purpose of this study was to examine the dynamic relationships among symptoms in PTSD patients receiving standard or a modified version of prolonged exposure therapy. Nine PTSD symptoms were studied, the eight common to DSM-IV and ICD-11 plus sleep problems. Within the temporal network, physiological reactivity to intrusions in a given week was positively related to distress reactivity and to flashbacks the subsequent week. That is, when physiological reactivity for a given patient was higher than expected for that patient a week, next week distress reactivity and flashbacks were also higher than expected. Hypervigilance a week was positively related to startle response and external avoidance the subsequent week. In addition, sleep problems were positively predicted by previous week internal avoidance and negatively predicted by previous week flashbacks. Seven of 9 symptoms had self-loops, all were negative. These self-loops most likely represent a methodological artefact, known as Nickell’s Bias, which often occur when the time series are relatively short (Nickell, Citation1981). Alternatively, they may reflect the clinical observation that PTSD symptoms tend to oscillate between re-experiencing and avoidance (Horowitz, Citation1986).
Network analysis includes an estimation of the centrality of variables derived from the pattern of network connections. In the temporal network, hypervigilance and physiological reactivation had the highest out-strength, indicating that they were the most predictive of other symptoms. Sleep problems had the highest in-strength, indicating that this symptom was the one being most predicted by other symptoms.
Inconsistent with our findings, Greene et al. (Citation2018) found an exaggerated startle response to have the highest out-strength in a temporal network of PTSD symptoms. Greene et al., however, studied civilians who were currently exposed to frightening stimuli and it is reasonable that startle response has a more prominent role among them than among our PTSD patients, who no longer were exposed to their traumas. Furthermore, the lagged associations over a half day in Greene et al.s’ study should not necessarily be expected to be the same as our associations over a week. Consistent with our findings, Greene et al. found sleep problems to have the highest in-strength. By contrast, Short et al. (Citation2017) found that sleep efficiency (sleep onset latency and wake after sleep onset) predicted PTSD symptoms and not the other way around. The discrepant findings may relate to sample differences. Most notably, the majority of subjects in Short et al. received outpatient psychopharmacological treatment, whereas our inpatients received exposure therapies with a focus on PTSD symptoms and were free of medication.
The contemporaneous network had many of the links showed in the temporal network. In addition, it showed positive links between internal and external avoidance and between re-experiencing and dreams. Thus, these variables may be causally connected in one direction or other, but on a time level smaller than one week.
Although some studies have addressed the temporal dynamics of PTSD by studying lagged within-person relationships (e.g., Short et al., Citation2017), they have focused latent variables. The network approach used here instead targets the observable symptoms themselves. If corroborated in future research, the present findings may lead to specifications – on the level of observables – of dynamic theories (e.g., therapy theories) of PTSD. They suggest that the physiological response to reminders impact flashbacks and emotional distress. As the physiological reactions (e.g., sweating, heart pounding) often are anxiety symptoms, the findings corroborate the central role of anxiety in PTSD. This has been a debated issue, for instance, reflected in the movement of PTSD from the anxiety disorders in DSM-IV to a new diagnostic group – trauma-related disorders – in DSM-5. Moreover, the findings suggest that avoidance of reminders of the traumatic event(s) is based on general hypervigilance and not on fear of re-experiencing symptoms. However, this finding should be interpreted cautiously, as external avoidance was connected to distress reactions to reminders (a re-experiencing symptom) in the contemporaneous network. The focus on observable symptoms instead of disorders leads to more specific and therefore actionable treatment recommendations. In particular, symptoms with high out-strength may be targets for potentially effective interventions, although this has not been tested for any disorder yet (Wichers, Wigman, Bringmann, & de Jonge, Citation2017). The findings suggest that physiological reactivation and hypervigilance should be primary targets of PTSD treatment. If these findings are corroborated, a natural further step in research would be to examine mechanisms of change, for instance, how physiological reactivation influences later distress and flashbacks. As mentioned, this reactivation is mainly about anxiety symptoms, and a possible mechanism may be that exacerbation of these symptoms may strengthen catastrophic thoughts such as ‘anxiety will never end’, ‘I will lose control’, or ‘I may die from a heart attack’ (Foa et al., Citation2007). In turn, these thoughts may strengthen distress reactivity as well as flashbacks. Hypervigilance involves scanning the environment for aspects that are reminiscent of the trauma. This threat monitoring increases the likelihood that cues perceived as signs of danger will be noticed, thus strengthening the belief that they are currently in danger. In turn, this experience of current threat strengthens the tendency to avoid the situation. The reduced threshold for threat cues associated with hypervigilance may also influence startle response. Clarifying such mechanisms may lead to improved interventions. For instance, if catastrophic thoughts mediate the relationship between physiological reactivation and flashbacks, this relationship may be blocked by changing the thoughts through tailored behavioural experiments (Ehlers & Wild, Citation2015).
Interestingly, the central components of PE (Foa et al., Citation2007) aim to affect the symptoms found central in this study. Imagery exposure (and listening to tapes of this activity) is purported to reduce physiological reactivation and in-vivo exposure to reduce hypervigilance. A natural further step would then be to study how imagery and in-vivo exposure exert their effects on reactivation and hypervigilance, respectively, and alternatively, how these therapy components affect the connections between reactivation and hypervigilance and the affected symptoms.
A reminder of what scores are analysed in the three networks may help interpreting and comparing the findings. The temporal network is based on the patients’ time-dependent state scores, that is, the time-specific deviations from their linear change trajectories. It is a directed network representing the average within-person lagged relationships between the symptoms, controlled for each other and unaffected by the between-person differences in within-person means. The contemporaneous network represents the within-person relationships of state scores at the same measurement occasion, controlled for the temporal effects. This network is undirected but its connections may reflect causal relationships transpiring within the week. Finally, the between-person network is based on the patients ‘trait’ scores, that is, their personal means of symptoms. It represents the partial correlations between these means when the state scores are removed. Thus, the three networks are based on different and independent data and they should not be assumed to be isomorphic.
Actually, the three networks are fairly similar in reproducing two of the three DSM-IV clusters, re-experiencing and hyperarousal. The temporal network was less connected than the other two. Sleep problems showed the expected affiliation to the hyperarousal cluster in the contemporaneous network, but connected to the re-experiencing cluster in the temporal and between-person network. Internal and external avoidance shifted affiliation across clusters and were related to each other only in the contemporaneous and the between-person network.
Comparing our between-person findings to the ones of available cross-sectional network studies, the strong connections between dreams and sleep problems, between internal and external avoidance, and between hypervigilance and startle response were all found in a large multi-sample cross-sectional network study (Fried et al., Citation2018). The strong connection between physiological reactivity and distress in our sample could not be compared to the Fried et al. study because these authors combined the two symptoms.
4.1. Strengths and limitations
The temporal network represents the within-person relationships over the course of therapy, which are of particular relevance for psychotherapy theories. This is because therapy theories are dynamic in the sense that they concern how change in a process variable relates to a subsequent change in an outcome variable during therapy. Such knowledge directly informs therapists what process variables and what connections between such variables that need to be affected to achieve patient improvement.
The studied sample had high clinical representativeness as research eligibility was similar to treatment eligibility and only 3 (4.2%) of 71 treatments eligible patients declined research participation. Moreover, the drop-out rate was low, 3 (4.6%) of 65 ITT patients.
The studied patients had experienced a variety of traumas – often of a prolonged and/or repeated type – and all patients considered eligible for treatment were also considered eligible for the study. The patients had a high degree of co-morbidity, long duration of PTSD, and over half of the patients had experienced repeated and/or prolonged traumas. Such a general and clinically representative sample is suited for an initial investigation of the temporal networks of PTSD patients in treatment.
The present study has several limitations. The most important one was the limited number of observations of about 10 per person, while at least 20 observations per person is recommended (Ramseyer, Kupper, Caspar, Znoj, & Tschacher, Citation2014). In addition, the sample size was limited. Thus, the stability and accuracy of the network should have been investigated. However, to the authors’ knowledge there are no implemented packages in R that estimate stability and accuracy in longitudinal data (Epskamp, Borsboom, & Fried, Citation2018). In order to test if the results found with the mlVAR method could be replicated, the results should have been compared with a second validation dataset. No such dataset was available at the time of the writing, thus future replications of the results are needed. Moreover, only a limited number of the measured DSM-IV PTSD symptoms could be subjected to network analysis and we chose those deemed most important in the development of the ICD-11. Negative alterations in cognition and mood, which are included in DSM-5, are likely to play a causal role in PTSD networks (Zalta et al., Citation2014), but are not included in DSM-IV. In temporal networks, variables control for each other, and inclusion of cognitions and mood might have altered the whole network. Symptom ratings were done on Monday mornings and based on the experiences of the three last days. Thus, the patients rated a less active period of their inpatient treatment and this may have affected the results. We studied process on a weekly time scale, and larger or especially lesser scales could be associated with different and more accurate results. Our findings probably reflect accumulated causal effects across several symptom episodes, although some of the effects transpiring within the weekly interval may have been captured in the contemporaneous network.
4.2. Implications
As elaborated above, the present study invites an increased focus on within-person relationships on the level of observables instead of latent constructs in psychotherapy research. Future studies should examine the replicability of our temporal PTSD network and also include causal variables beyond symptoms (e.g., catastrophic interpretations of symptoms, threat monitoring) to investigate mechanisms of change (Jones, Heeren, & McNally, Citation2017). A smaller timescale than weekly should be investigated.
The present within-person results make a preliminary basis for the recommendation to monitor and facilitate change in physiological reactivation and hypervigilance in the treatment of PTSD.
Supplemental Material
Download MS Word (15 KB)Disclosure statement
No potential conflict of interest was reported by the authors.
Supplementary material
Supplemental data for this article can be accessed here.
References
- American Psychiatric Association. (1994). Diagnostic and statistical manual of mental disorders (4th ed.). Washington, DC: American Psychiatric Association..
- Borsboom, D. (2017). A network theory of mental disorders. World Psychiatry: Official Journal of the World Psychiatric Association (WPA), 16, 5–11. doi:10.1002/wps.20375
- Bradley, B., Greene, J., Russ, E., Dutra, L., & Westen, D. (2005). A multidimensional metaanalysis of psychotherapy for PTSD. American Journal of Psychiatry, 162, 214–227.
- Bringmann, L. F., Vissers, N., Wichers, M., Geschwind, N., Kuppens, P., Peeters, F., & Tuerlinckx, F. (2013). A network approach to psychopathology: New insights into clinical longitudinal data. PloS One, 8, e60188. doi:10.1371/journal.pone.0060188
- Edwards, J. R., & Bagozzi, R. P. (2000). On the nature and direction of relationships between constructs and measures. Psychological Methods, 5, 155–174. doi:10.1037//1082-989X.5.2.155
- Ehlers, A., & Wild, J. (2015). Cognitive therapy for PTSD: Updating memories and meanings of trauma. In U. Schnyder & M. Cloitre (Eds.), Evidence based treatments for trauma-related psychological disorders: A practical guide for clinicians (pp. 161–187). Switzerland: Springer International Publishing.
- Epskamp, S., Borsboom, D., & Fried, E. I. (2018). Estimating psychological networks and their accuracy: A tutorial paper. Behavior Research Methods, 50, 195–212. doi:10.3758/s13428-017-0862-1
- Epskamp, S., Cramer, A. O. J., Waldorp, L. J., Schmittmann, V. D., & Borsboom, D. (2012). Qgraph: Network visualizations of relationships in psychometric data. Journal of Statistical Software, 48, 1–18.
- Epskamp, S., Waldorp, L. J., Mõttus, R., & Borsboom, D. (2018). Discovering psychological dynamics: The gaussian graphical model in cross-sectional and time-series data. arXiv:1609.04156v6[stat.ME] (Open Access).
- First, M. B., Reed, G. M., Hyman, S. E., & Saxena, S. (2015). The development of the ICD-11 clinical descriptions and diagnostic guidelines for mental and behavioural disorders. World Psychiatry: Official Journal of the World Psychiatric Association (WPA), 14, 82–90. doi:10.1002/wps.20189
- Foa, E. B., Hembree, E., & Rothbaum, B. O. (2007). Prolonged exposure therapy for PTSD: Emotional processing of traumatic experiences. New York, NY: Oxford University Press.
- Foa, E. B., Riggs, D. S., Dancu, C. V., & Rothbaum, B. O. (1993). Reliability and validity of a brief instrument for assessing post-traumatic stress disorder. Journal of Traumatic Stress, 6, 459–473.
- Fried, E., Eidhof, M. B., Palic, S., Constantini, G., Dijk, H. M. H., Bockting, C. L. H., … Karstoft, K.-I. (2018). Replicability and generalizability of posttraumatic stress disorder networks: A cross-cultural multisite study of PTSD symptoms in four trauma patient samples. Clinical Psychological Science, 6, 335–351. doi:10.1177/2167702617745092
- Greene, T., Gelkopf, M., Epskamp, S., & Fried, E. (2018). Dynamic networks of PTSD symptoms during conflict. Psychological Medicine, 48, 2409–2417. doi:10.1017/S0033291718000351
- Horowitz, M. J. (1986). Stress-response syndromes: A review of posttraumatic and adjustment disorders. Hospital & Community Psychiatry, 37, 241–249.
- Jones, P. J., Heeren, A., & McNally, R. J. (2017, August 2). Commentary: A network theory of mental disorders. Frontiers in Psychology, 8,1305. (Open Access).
- Kessler, R. C., Berglund, P., Delmer, O., Jin, R., Merikangas, K. R., & Walters, E. E. (2005). Lifetime prevalence and age-of-onset distributions of DSM-IV disorders in National Comorbidity Survey Replication. Archives of General Psychiatry, 62, 593–602.
- Kwiatkowski, D., Phillips, P. C. B., Schmidt, P., & Shin, Y. (1992). Testing the null hypothesis of stationarity against the alternative of a unit root. How sure are we that economic time series have a unit root? Journal of Econometrics, 54, 159–178.
- Langkaas, T. F., Hoffart, A., Øktedalen, T., Ulvenes, P. G., Hembree, E. A., & Smucker, M. (2017). Exposure and non-fear emotions: A randomized controlled study of exposure-based and rescripting-based imagery in PTSD treatment. Behaviour Research and Therapy, 97, 33–42. doi:10.1016/j.brat.2017.06.007
- McNally, R. J. (2016). Can network analysis transform psychopathology? Behaviour Research and Therapy, 86, 95–104. doi:10.1016/j.brat.2016.06.006
- NICE. (2005). Post-traumatic Stress Disorder (PTSD): The management of PTSD in adults and children in primary and secondary care. NICE clinical guideline 26. [NICE guideline]. Available from: http://guidance.nice.org.uk/CG26.
- Nickell, S. (1981). Biases in dynamic models with fixed effects. Econometrica, 49, 1417–1426. doi:10.2307/1911408
- Opsahl, T., Agneessens, F., & Skvoretz, F. (2010). Node centrality in weighted networks: Generating degree and shortest paths. Social Networks, 32, 245–251. doi:10.1016/j.socnet.2010.03.006
- Ramseyer, F., Kupper, Z., Caspar, F., Znoj, H., & Tschacher, W. (2014). Time-Series Panel Analysis (TSPA): Multivariate modeling of temporal associations in psychotherapy process. Journal of Consulting and Clinical Psychology, 828–838. doi:10.1037/a0037168
- R Core Team. (2017). R: A language and environment for statistical computing. Vienna, Austria: R Foundation for Statistical Computing. Available from: https://www.R-project.org/
- Short, N. A., Allan, N. P., & Schmidt, N. B. (2017). Sleep disturbance as a predictor of affective functioning and symptom severity among individuals with PTSD: An ecological momentary assessment study. Behaviour Research and Therapy, 97, 146–153. doi:10.1016/j.brat.2017.07.014
- Smucker, M. R. (2005). Imagery rescripting and reprocessing therapy (IRRT): A treatment manual for adult survivors of childhood sexual abuse experiencing PTSD. Milwaukee: International Trauma Institute.
- Wichers, M., Wigman, J. T. W., Bringmann, L. F., & de Jonge, P. (2017). Mental disorders as networks: Some cautionary reflections on a promising approach. Social Psychiatry and Psychiatric Epidemiology, 52, 143–145. doi:10.1007/s00127-016-1319-z
- Zalta, A. K., Gillihan, S. J., Fisher, A. J., Mintz, J., McLean, C. P., Yehuda, R., & Foa, E. B. (2014). Change in negative cognitions associated with PTSD predicts symptom reduction in prolonged exposure. Journal of Consulting and Clinical Psychology, 82, 171–175. doi:10.1037/a0034735