ABSTRACT
Background: The empirical literature of network analysis studies of posttraumatic stress symptoms (PTSS) has grown rapidly over the last years.
Objective: We aimed to assess the characteristics of these studies, and if possible, the most and least central symptoms and the strongest edges in the networks of PTSS.
Method: The present systematic review, conducted in PsycInfo, Medline, and Web of Science, synthesizes findings from 20 cross-sectional PTSS network studies that were accepted for publication between January 2010 and November 2018 (PROSPERO ID: CRD42018112825).
Results: Results indicated that the network studies investigated a broad range of samples and that most studies used similar analytic approaches including stability analysis. Only strength centrality was generally adequately stable. Amnesia was consistently reported to have lowest strength, while there was substantial heterogeneity regarding which nodes had highest strength centrality. The strongest edge weights were typically within each DSM-IV/DSM-5 PTSD symptom cluster.
Conclusions: Hypothesis-driven studies are needed to determine whether the heterogeneity in networks resulted from differences in samples or whether they are the product of underlying methodological reasons.
Antecedentes: La literatura empírica los estudios de análisis en redes de síntomas de estrés postraumático (SEPT) ha crecido rápidamente en los últimos años.
Objetivos: Nuestro objetivo fue el evaluar las características de estos estudios y, de ser posible, evaluar cuáles eran aquellos síntomas más cardinales y cuáles no, y cuáles eran los enlaces más fuertes en las redes de los SEPT.
Métodos: La presente revisión sistemática, realizada en PsycInfo, Medline, y Web of Science, sintetiza los hallazgos de 20 estudios transversales en redes sobre SEPT que se basaron sobre información transversal, y que fueron aceptados para publicación entre enero de 2010 y noviembre de 2018 (PROSPERO ID: CRD42018112825).
Resultados: Los resultados indicaron que los estudios en redes investigaron un amplio rango de muestras, y que la mayoría de estudios emplearon enfoques analíticos similares, incluyendo el análisis de estabilidad. Solo la centralidad de la fuerza fue generalmente adecuadamente estable. Se informó consistentemente que la amnesia tenía la fuerza más baja, mientras que había una heterogeneidad sustancial con respecto a qué nodos tenían la centralidad de la fuerza más alta. Los pesos de los enlaces de red más fuertes se encontraban, por lo general, dentro de cada racimo de síntomas para trastorno de estrés postraumático contemplados en el DSM IV/DSM 5.
Conclusiones: Se necesitan estudios derivados de hipótesis para determinar si la heterogeneidad de las redes resultó de las diferencias en las muestras, o si resultaron del producto de cuestiones metodológicas subyacentes.
背景:在过去的几年中, 有关创伤后应激症状 (PTSS) 的网络分析研究的实证文献迅速增多。目的:我们旨在评估这些研究的特征, 如果可能也会评估PTSS网络中最中心和最非中心的症状以及最强边。方法:本系统综述在PsycInfo, Medline和Web of Science中进行, 总结了20项横断面PTSS网络研究的发现, 这些研究基于横断面数据并于2010年1月至2018年11月之间被接收发表 (PROSPERO ID:CRD42018112825)。结果:结果表明, 网络研究调查了各种各样的样本, 大多数研究使用了包括稳定性分析在内的类似分析方法。通常只有强度中心足够稳定。失忆症一直被报告为强度最低,而关于哪些节点的强度中心性存在很大的异质性。最强的边权重通常在每个DSM-IV/DSM-5 PTSD症状簇内。结论:需要进行假设驱动的研究, 以确定网络中的异质性是由样本差异引起还是潜在方法学原因的产物。
KEYWORDS:
PALABRAS CLAVE:
Over the last years, the network approach to psychopathology (Borsboom, Citation2017; Borsboom & Cramer, Citation2013; Fried & Cramer, Citation2017; McNally, Citation2016) has become increasingly popular. The network approach argues that mental disorders are characterized by causal interactions between observable psychopathological phenomena, for example symptoms. The emergence, maintenance and cessation of a mental disorder can be described by the dynamic interplay of symptoms. A prototypical example for a causal interaction between symptoms of posttraumatic stress disorder (PTSD) is that in the aftermath of a traumatic event one could experience intrusive memories of the trauma that may give rise to physiological arousal, which may, in turn, promote sleeping problems. This has been set in contrast to the ‘traditional’ model of psychiatric disorders, which conceptualizes mental disorders as non-observable common causes of their observable symptoms (Borsboom, Cramer, & Kalis, Citation2019). In such a model, one assumes absence of direct relations between symptoms conditional on their common cause. This seems implausible in the case of mental disorders, where there are clear evidence of symptom interactions.
The first two studies to introduce the network approach to the field of traumatic stress were published in 2015 (De Schryver, Vindevogel, Rasmussen, & Cramer, Citation2015; McNally et al., Citation2015). Since then, a considerable number of studies using network analytic methods on traumatic stress have been published. Several studies analysed the network structure of symptoms of posttraumatic stress (e.g. Benfer et al., Citation2018), others have explored relationships with covariates (e.g. Armour, Fried, Deserno, Tsai, & Pietrzak, Citation2017), or connections to other mental disorders (e.g. Afzali et al., Citation2017). Although most studies compared their individual contributions with existing literature, no systematic review of network analytic studies in traumatic stress research has been undertaken so far. Synthetizing findings from the existing empirical network studies may help us to specify hypotheses for a network theoretical account of PTSD. Such hypotheses would have to take existing theories about the development of PTSD, which have been proposed over the last decades, into account. These theories seek to pinpoint important aspects of the symptomatology and explain how symptoms (or clusters of symptoms) connect with each other. The conditioning theories of PTSD (e.g. Foa, Huppert, & Cahill, Citation2006; Keane, Marshall, & Taft, Citation2006), argue that psychological and physiological cue reactivity lay at the core of PTSD. Cognitive models of PTSD emphasize the role of memories or intrusions of trauma (Brewin, Gregory, Lipton, & Burgess, Citation2010; Rubin, Berntsen, & Bohni, Citation2008), and negative (threat-relevant) cognitions (Ehlers & Clark, Citation2000). All these theories emphasize different aspects of the PTSD symptomatology and are, therefore, not exclusive but complementary to each other.
Reviewing the literature on network studies systematically has unique challenges. Currently, no methods for conducting a meta-analysis of networks are available. It is therefore not surprising that the few reviews on network literature are mainly narrative (e.g. Contreras, Nieto, Valiente, Espinosa, & Vazquez, Citation2019; Fried & Cramer, Citation2017; Smith et al., Citation2018). A notable exception is a paper by Forbes, Wright, Markon, and Krueger (Citation2017b), who summarized and compared network-specific metrics across different studies. To be able to compare network-specific metrics (e.g. centrality of a given node) across studies, the individual studies need to use similar statistical methods (e.g. Gaussian Graphical Models; Epskamp, Waldorp, Mõttus, & Borsboom, Citation2018), and investigate the same network model (e.g. a network consisting of the same symptoms).
Hence, this preregistered systematic review had two goals. As a primary outcome, we aimed to assess the characteristics of the current network studies of posttraumatic stress symptoms in four categories: (a) sample characteristics, (b) included measures, (c) used statistical methods and (d) endorsement of open science practices. Given that the identified studies used methods which allow for comparison of their individual results, we also aimed to assess secondary outcomes. These were: (1) the three most and least central symptoms in each network; and (2) the strongest associations within each network. This review will provide an overview of network studies of posttraumatic stress symptoms (PTSS), as well as assist in identifying gaps in the literature, point to future directions and help to translate the findings from network studies to research on traumatic stress in general.
1. Methods
1.1. Protocol and preregistration
The methods of the literature search, inclusion criteria, search terms, and methods of analysis were specified, documented in a protocol and registered in advance following the PRISMA guidelines (Moher, Liberati, Tetzlaff, & Altman, Citation2009; PROSPERO ID: CRD42018112825, http://www.crd.york.ac.uk/PROSPERO/display_record.php?ID=CRD42018112825).
1.2. Inclusion and exclusion criteria
The following inclusion criteria were applied: (1) original empirical studies, (2) written in English; (3) peer-reviewed; (4) measured PTSS; (5) conducted network analysis of PTSS; (6) estimated a network based on cross-sectional data; (7) accepted for publication during January 2010 and November 2018.
1.3. Information sources and search
Articles were identified through a literature search in OVID (PsycInfo, Medline, Medline epub ahead of print) and Web of Science, with the search being restricted to studies published between January 2010 and November 2018. The following search terms were used: (network analysis OR network approach OR network model OR network structure OR network modelling) AND (posttraumatic stress disorder OR post-traumatic disorder OR PTSD OR posttraumatic stress symptoms OR PTS or PTS symptom or PTSD symptoms). In addition, we included articles selected by hand through cross-referencing and accepted articles that we knew of. The search date was 8 November 2018.
1.4. Study selection and data collection process
The unblinded study selection process was undertaken independently by two reviewers. Potential studies were identified with the use of the search strategy outlined above. After removing duplicates and manuscripts not available in English, potential studies were screened by title and abstract for eligibility. If the eligibility was still unclear, full-text review was undertaken. Finally, disagreements between reviewers were resolved by consensus. The data were extracted by two reviewers independently. The extracted data were then compared by the two reviewers and disagreements resolved. Finally, the data were transformed into the presented tables and figures, which were also cross-checked by both reviewers.
1.5. Data items
For the primary outcome, namely the characterization of the current network studies of posttraumatic stress symptoms, several variables grouped in four categories were assessed: (a) study participants (sample type, trauma type, time since trauma, percentage of females, and PTSD severity), (b) measurement (measure used to assess PTSS), (c) statistics (sample size, sample size per node, type of regularization, stability analysis and the CS-Coefficients, significance testing of strength and edge weights), and (d) reproducibility (preregistration, open data, included script, open access). Open access was defined as gold (published in an online open-access journal) or green (published in any journal, but the authors have self-archived a copy in a freely accessible archive or website) open access between October 2018 and February 2019. The secondary outcomes included centrality and edge weights. Higher centrality of a symptom indicates a stronger association with other symptoms in the network. Three commonly assess indices of centrality are strength, closeness, and betweenness. The strength of a node indicates the mean magnitude of the partial correlations of each edge linked to the node (for more details, see Opsahl, Agneessens, & Skvoretz, Citation2010). The edge weights reflect the association between two nodes (symptoms) after controlling for all other nodes (symptoms) in the network. We assessed centrality by extracting the three nodes with highest and lowest centrality and, if available, counted nodes which significantly differed in strength from at least two-thirds of the other nodes. For edge weights, for each study with the information available (thus, had conducted significance testing and provided the results), we listed the edges with edge weights which differed significantly from the weight of at least two-thirds of all the other edges.
1.6. Risk of bias
At the time of writing, no specific instruments to assess the quality or bias of network studies of psychopathological symptoms exist. Furthermore, due to the rapid development of statistical tools over the last years, no technical standard for conducting network analysis with PTSS has been established so far. Accordingly, we chose not to evaluate the quality of the studies as such, but rather to describe them in terms of the pre-specified characteristics above. Moreover, extracting these characteristics systematically should also help with identifying potential sources of bias. Thus, assessing the risk of bias across all or for individual studies was not applicable for this review.
1.7. Deviations from the protocol
The following changes to the protocol were made: First, we added an additional inclusion criterion, namely that the study investigated PTSS network based on cross-sectional data. This change was made, because only one (Greene, Gelkopf, Epskamp, & Fried, Citation2018) of the 21 primarily identified studies used a longitudinal design, whereas all other assessed cross-sectional data. Second, two of the variables we planned to evaluate were not assessed because we found them highly subjective (‘interpretation based on statistics’, and ‘complete description of the methods used’). Third, we did not present data on ‘item variability’ (whether the centrality measures were correlated with the item variance), because few studies reported the required information. Fourth, we added the percentage of females in the samples of the individual studies as an item of the ‘study participants’ group of the primary outcome.
2. Results
2.1. Study selection
The study selection process is shown in . Initially, 242 articles were identified by database search and three additional articles were identified through other sources. After removal of duplicates, 198 studies were screened and 158 records were excluded. The full text of 40 studies was assessed, of which 20 studies were subsequently excluded (11 did not present a network of PTSS alone, four did not present a network of PTSS, four were not empirical studies, and one presented networks which were not based on cross-sectional data, see Table S1 for details about the excluded studies). In total 20 studies were selected for the systematic review (Armour et al., Citation2017; Bartels et al., Citation2019; Benfer et al., Citation2018; Birkeland, Blix, Solberg, & Heir, Citation2017; Birkeland & Heir, Citation2017; Bryant et al., Citation2017; Cao et al., Citation2019; Epskamp, Borsboom, & Fried, Citation2018; Fried & Cramer, Citation2017; Fried et al., Citation2018; McNally, Heeren, & Robinaugh, Citation2017; McNally et al., Citation2015; Mitchell et al., Citation2017; Moshier et al., Citation2018; Phillips, Wilson, Sun, Workgroup, & Morey, Citation2018; Ross, Murphy, & Armour, Citation2018; Russell, Neill, Carrion, & Weems, Citation2017; Spiller et al., Citation2017; Sullivan, Smith, Lewis, & Jones, Citation2018; von Stockert, Fried, Armour, & Pietrzak, Citation2018).
2.2. Characteristics of the cross-sectional studies of PTSS
The results of our primary outcome, namely assessing the characteristics of network studies of the PTSS are presented in .
Table 1. Characteristics of cross-sectional network studies of PTSS
2.2.1. Design
Although all 20 of the included studies used cross-sectional data to estimate networks, they differed in their design. Most studies estimated one PTSS network structure and the corresponding centrality indices in one sample (n = 10), but others estimated several networks in the same study (two: n = 7, three: n = 1, and four: n = 2). The studies presenting more than one network had several reasons for their procedure. For example, they compared samples with different characteristics (e.g. different trauma types; Benfer et al., Citation2018) or assessed the same samples at two different time points (Bryant et al., Citation2017; von Stockert et al., Citation2018). This resulted in a total of 35 individual networks. Of these networks, two were based on the same dataset (Epskamp et al., Citation2018; Fried & Cramer, Citation2017), two studies had partly overlapping datasets (Birkeland et al., Citation2017; Birkeland & Heir, Citation2017), one study’s subgroups overlapped (Phillips et al., Citation2018), and one study assessed the same sample with two different questionnaires (Moshier et al., Citation2018). The different design choices limited the comparability of the included networks and have implications for the interpretation of the secondary outcomes.
2.2.2. Study participants
Of the 35 networks, 14 were estimated in a community sample, nine in a clinical sample, six in a veteran sample, and six in a combined veteran and clinical sample. With regard to all assessed variables, we found large heterogeneity (e.g. the percentage of females ranged from zero to 100, likewise the percentage of PTSD diagnosis).
2.2.3. Measures
The most used questionnaire was the ‘The PTSD Checklist’ (PCL; Weathers, Litz, Herman, Huska, & Keane, Citation1993), which was used in different versions by a total of 11 studies (n = 15 networks). There were a slightly higher number of studies based on the DSM-IV conceptualization of PTSD (n = 12 studies, n = 22 networks), compared with those based on a DSM-5 criteria (n = 8 studies, n = 13 networks).
2.2.4. Statistics
In general, the included studies used comparable statistics to estimate their networks. All used gLASSO to estimate the networks and more than half of the studies (n = 12 studies, n = 23 networks) specified to have used polychoric correlations. The ratio of observations per node varied between 7.6 and 463.9. The CS-Coefficients for betweenness and closeness were all below 0.50, indicating unstable centrality indices (Costantini et al., Citation2019; Epskamp et al., Citation2018). CS-Coefficients for strength varied between 0.07 and 0.75. Significance testing for strength centrality and edge weights were conducted in the majority of the studies, respectively, networks (n = 15 studies, n = 28 networks). Three studies additionally estimated direct acyclic graphs (Bartels et al., Citation2019; McNally et al., Citation2017; Phillips et al., Citation2018).
2.2.5. Reproducibility
Three quarters (n = 15) of the included articles were freely accessible at the time of writing. Eleven studies included their analytic script (R codes). For six studies the full dataset was available, another six published the covariance matrices. However, no study was preregistered.
2.3. Strength centrality
For 32 of the 35 networks included, node strength (or expected influence in one study; Phillips et al., Citation2018) centrality analyses were available (exceptions: Birkeland et al., Citation2017; McNally et al., Citation2015; Sullivan et al., Citation2018). presents the three items with the strongest and weakest centrality within each network. In 27 networks, ‘amnesia’ was reported to be among the three items with lowest centrality. In the networks based on DSM-IV items, ‘recurrent thoughts of trauma’ (B1) was reported to have strong centrality in nine of 19 networks. ‘Persistent negative emotional state’ (D4) was found to be among the top three items in eight of 13 networks based DSM-5 symptoms. Still, heterogeneity was high overall. Significance testing for centrality was available for 20 networks (for 10 of 19 DSM-IV and for 10 of 13 DSM-5 networks, see Figure S1). The node strength of ‘amnesia’ was found to differ significantly from two-thirds of all other node’s strength in 10 networks (five DSM-IV and five DSM-5 networks). In six networks (three DSM-IV and three DSM-5 networks) no node surpassed this threshold. Only in six of 20 networks, more than one node’s strength differed from two-thirds of the others.
Figure 2. Top three and bottom three nodes according to node strength. DSM-IV (left), and DSM-5 (right)
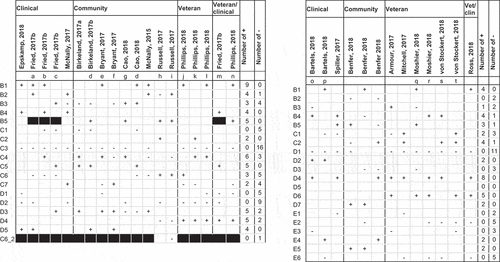
2.4. Individual edges
Results of significance testing of differences between individual edges were available for 22 networks (n = 14 DSM-IV and n = 8 DSM-5 networks). A summary of these results is presented in (results from individual studies can be found in the Supplemental Materials). Data on edges from all networks presenting significance testing were included (including all four networks presented by Phillips and colleagues, although they include data from overlapping sub-samples). An edge was included in the summary figure if it was significantly different from two-thirds of the other edge weights in the same network. The results indicate that the edge between ‘exaggerated startle response’ and ‘hypervigilance’ was especially prominent in both DSM-IV and DSM-5 networks. In general, most of the edges fulfiling the outlined criteria above were edges between symptoms within the same DSM cluster (84.2% of all edges in the DSM-IV networks summary, and 88.1% of all edges in the DSM-5 networks summary). A notable exception was the edge between the items ‘nightmares’ (B2) and ‘sleeping difficulties’ (D1) in DSM-IV based networks. Furthermore, 65.8% of the included edges in the DSM-IV based networks, and 72.9% in the DSM-5 based networks were edges between symptoms following each other in the questionnaire’s item order.
Figure 3. Number of networks finding edge-weights to be stronger than at least two-thirds of the total computed edge weights in network, DSM-IV (left), and DSM-5 (right)
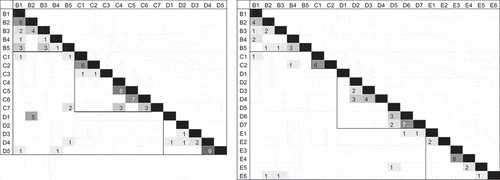
3. Discussion
The main findings of this first preregistered systematic review of network studies of PTSS were threefold. First, there was a considerable heterogeneity in the sample characteristics, but in general, comparable statistical approaches were used to estimate and assess the networks. Second, there was generally large heterogeneity in strength centrality, but ‘amnesia’ (memory impairment) was consistently found to be among the least central nodes in the networks. Third, the strongest edges were almost exclusively between symptoms of the same symptom cluster as defined by the version of the DSM to which the relevant measure corresponded.
3.1. Characteristics of network studies of PTSS
The included studies showed large heterogeneity regarding the type of sample (clinical, community, and veteran), the types of trauma exposed to (e.g. lifetime or sexual abuse in childhood) and with different intervals since the traumatic event. Community samples were all measured at a specific time point after a specific event, such as a natural disaster or mass violent event, whereas the clinical and veteran samples often had less information of specific trauma or timing of measurement after the traumatic experiences. The percentage of participants with probable PTSD varied substantially, likewise the gender balance. The sample characteristics seem to represent the diversity of samples in research on traumatic stress in general.
The statistics used were relatively similar across studies. Most used polychoric correlations with gLASSO regularization, which identifies sparse networks. It could be that the networks reflect the algorithm used. If other fitting algorithms were used, different and denser networks might have been identified which could have yielded additional theoretically relevant interconnections. With the exception of the earlier studies, most performed stability analysis and provided CS coefficients, following recommendations presented in tutorials by the developers of the network methodology (e.g. Epskamp et al., Citation2018; Epskamp, Cramer, Waldorp, Schmittmann, & Borsboom, Citation2012; Epskamp & Fried, Citation2017). Given that no studies found their betweenness and closeness centrality to be above the recommended cut off for interpretation (0.5), we provide empirical evidence against the use of these two indices to the recent debate about the usefulness of centrality (Bringmann, Elmer, Epskamp, & Krause, Citation2019; Hallquist, Wright, & Molenaar, Citation2019). The CS-coefficients indicated sufficient stability for strength in most studies.
At least some open science practices were endorsed by the majority of the studies. The percentage of open accessible papers (75%) is impressively higher than the average in traumatic stress research in general (in 2017, 17% of all studies were published ‘gold’ open access (Olff, Citation2018)). The fact that the developers of the network methodology themselves have been very committed to open science is likely to have influenced the open science practices among those who applied this methodology on their own data. This has probably also contributed to the popularity of this studies and facilitated that application of network analysis within the field of traumatic stress.
3.2. Node strength of symptoms
We want to emphasize three key findings of our analysis of strength centrality. First, ‘amnesia’ (memory impairment) had low strength in most of the included studies. This is in line with a systematic review of factor-analytic studies on PTSS, which reported weak loading of ‘amnesia’ onto the corresponding clusters (Armour, Műllerová, & Elhai, Citation2016). These findings indicate that amnesia is loosely related to the other symptoms in the DSM-IV and DSM-5 definition of PTSD. Therefore, it seems that for most trauma-exposed individuals, amnesia is not a core symptom of PTSD.
Second, there were two additional symptoms that were repeatedly among the top three symptoms according to centrality: ‘recurrent thoughts of trauma’ in DSM-IV networks and ‘persistent negative emotional state’ in DSM-5 networks. Having recurrent thoughts of trauma is a prototypical symptom of PTSD. The above average association with other PTSS is therefore not surprising, and in line with cognitive theories of PTSD that emphasize the role of memories or intrusions of trauma (Brewin et al., Citation2010; Rubin et al., Citation2008). The symptom ‘persistent negative emotional state’ was added with the fifth revision of the DSM criteria of PTSD. This is a non-specific symptom which shares conceptual overlap with symptoms of several other mental disorders, such as major depressive disorder. This might point to common mechanisms underlying this comorbidity.
Third, besides the named exceptions, there was much heterogeneity in the individual items’ node strength across studies. There may be several reasons for this. Given that there are many different combinations of symptoms that qualify as PTSD (Galatzer-Levy & Bryant, Citation2013), different trauma types may produce particular profiles of PTSS (Kelley, Weathers, McDevitt‐Murphy, Eakin, & Flood, Citation2009; Stein, Wilmot, & Solomon, Citation2016). In accordance, the latent structure of PTSS has been found to vary across different trauma types (Shevlin & Elklit, Citation2012). Further factors known to impact the emergence or maintenance of PTSD such as gender, rate and type of comorbidities, genetics, and resilience might moderate symptom profiles and symptom networks in a similar way. Some of the included studies were specifically aimed to investigate the influence of such factors. For example, one study compared networks of PTSS across several levels of combat exposure and found a higher centrality of intrusive thoughts in the high-exposure group compared to the low-exposure group (Phillips et al., Citation2018). Another study examined the impact of gender on network structure and found that the females’ network had higher global connectivity, and centrality were higher for detachment and intrusions compared with the males’ network (Cao et al., Citation2019).
The large heterogeneity found in centrality estimations might also be due to methodological factors. With regard to the estimation procedure, the properties of the estimation technique depend on several factors, which varied across studies (e.g. the sample size; Epskamp et al., Citation2018). Furthermore, the estimation procedure applied by all included studies uses regularization, which poses a further challenge to generalizability of the individual networks. Still, Fried and colleagues (Fried et al., Citation2018) concluded that the PTSS networks did replicate quite well across several similar datasets, when conducting joint estimation of networks of PTSS. However, the replicability of symptom networks has also been questioned (Forbes et al., Citation2017b), and there is an ongoing debate about the definition of replicability of networks per se (Borsboom et al., Citation2017; Forbes, Wright, Markon, & Krueger, Citation2017a; Jones, Williams, & McNally, Citationn.d.; Steinley, Hoffman, Brusco, & Sher, Citation2017). Furthermore, validity of centrality (Bringmann et al., Citation2019) and its usefulness in networks of psychopathological symptoms have also been doubted recently (Dablander & Hinne, Citation2019). Last, high severity of PTSD in a sample is known to bias the network structure by introducing spurious correlations (Berkson’s Bias; De Ron, Fried, & Epskamp, Citationn.d.). This further limits the direct comparison of networks estimated in populations affected with different PTSD severity. Therefore, the heterogeneity of these findings might at least in part reflect these issues.
3.3. Connections between symptoms
All edges with an edge that significantly differed from two-thirds of the included edges of a given network were found to be positive. The large majority of the identified edges were within the clusters specified by the DSM. Additionally, we discovered that the majority of the identified edges were between items that follow each other in the questionnaire. This is in line with literature indicating that prior items can influence interpretation and responses on later items, also called ‘question ordering effect’ (Krosnick, Citation2018; Tourangeau & Rasinski, Citation1988). Hence, our finding is not limited to network analysis, but impacts other statistical methods building on individually assessed items as well (e.g. factor analytic studies). This bias could be overcome by shuffling the order of the items in the questionnaire in randomized sequences for every participant.
With regard to individual edges, the most often identified edges were between ‘hyperarousal’ and ‘startle’, the two avoidance items and ‘diminished interests in activities’ and ‘restricted range of affect’. Only one of the more often found edges was between symptoms of different clusters, namely between ‘sleep difficulties’ and ‘nightmares’ in the DSM-IV networks. While some of these edges likely represent generic associations between independent symptoms (e.g. between ‘restricted affect’ and ‘diminished interest in activities’), others might be the result of an underlying latent variable (e.g. general avoidance underlying the two items assessing specific forms of avoidance). We therefore recommend that more attention should be directed to the design of the network model (i.e. which symptoms or elements to include in a network).
3.4. Strengths and limitations
This systematic review was preregistrated. This promotes transparency, helps reduce potential for bias, and reduce the probability of unintended duplication of reviews. However, there are also some limitations. First, no standards for conducting reviews on network analytic studies were available and had to be developed for this review. Moreover, we were not able to assess the quality of the studies or the risk of bias. We tried to make these limitations as transparent as possible. Furthermore, we hope that this article contributes to the development of standards for reviews of network studies. Second, we did not assess all individual edges in each included study, because it was not feasible with our resources. We hope that future technical developments will allow comparing individual edges across multiple studies more easily (e.g. Williams, Rast, Pericchi, & Mulder, Citationn.d.). Moreover, we call for the inclusion of a list with all non-zero edges in the supplement materials for in all future network studies. This would allow more precise analyses of the presence or absence of specific edges across several studies. Third, we deviated from the prespecified protocol in several ways. However, we describe our deviations explicitly in the methods section of this article. Fourth, the conclusions that can be drawn from this systematic review are limited by limitations in the current literature on network analysis of PTSD. For example, whereas the uniformity of the analyses in the publications to date made it possible to compare results across studies, it also prohibited examination of the effects of using different fitting algorithms. The vast majority of the studies we found were cross-sectional, which do not allow for conclusions about cause and effects between co-occurring symptoms.
3.5. Conclusions and implications
Network analysis in traumatic stress research only begun this decade and there has been rapid rate of publications (see also the upcoming special issue on network analyses within the field of traumatic stress in Journal of Traumatic Stress), and a speedy and continued development of technical possibilities. With this review, we not only provide a summary of the first generation of network studies of PTSS, but we also propose future directions for the field. First, we call for the systematic investigation of the above-mentioned reasons potentially underpinning the heterogeneity in strength centrality across studies. We urge the field to improve the measurement of the individual PTSS (e.g. with regard to the order of the items in the questionnaire). Second, although most network analytic studies cite the theoretical framework conceptualizing mental disorders as (dynamic) networks, no adaptation to PTSD has been published so far. Developing a network theoretical account of PTSD has the potential to provide a more comprehensive understanding of how the symptoms influence each other, which can give insight into how PTSD develops and is maintained. Relatedly, theoretical accounts and empirical examination of covariation between PTSS and non-PTSS presenting problems will contribute to the future understanding of the mechanisms of PTSS. Third, following the central line of argument of the network approach, intensive longitudinal investigations of the interplay of PTSS within individuals are needed. So far, only a few studies conducting these analyses have been published (Greene et al., Citation2018; Hoffart, Langkaas, Øktedalen, & Johnson, Citation2019) and future work in this area is urgently needed. With these challenges ahead and with new methods at the dawn (e.g. Bayesian methods; Williams et al., Citationn.d.), we think that the time is ripe for the next generation of network studies.
Supplemental Material
Download MS Word (16.7 KB)Supplemental Material
Download MS Word (30.7 KB)Disclosure statement
No potential conflict of interest was reported by the authors.
Data availability statement
The data that support the findings of this study are openly available as supplemental materials in http://osf.io/95es2.
Supplementary Material
Supplemental data for this article can be accessed here.
References
- Afzali, M. H., Sunderland, M., Teesson, M., Carragher, N., Mills, K., & Slade, T. (2017). A network approach to the comorbidity between posttraumatic stress disorder and major depressive disorder: The role of overlapping symptoms. Journal of Affective Disorders, 208, 490–14.
- Armour, C., Fried, E. I., Deserno, M. K., Tsai, J., & Pietrzak, R. H. (2017). A network analysis of DSM-5 posttraumatic stress disorder symptoms and correlates in US military veterans. Journal of Anxiety Disorders, 45, 49–59.
- Armour, C., Műllerová, J., & Elhai, J. D. (2016). A systematic literature review of PTSD’s latent structure in the diagnostic and statistical manual of mental disorders: DSM-IV to DSM-5. Clinical Psychology Review, 44, 60–74.
- Bartels, L., Berliner, L., Holt, T., Jensen, T. K., Jungbluth, N., Plener, P., … Sachser, C. (2019). The importance of the DSM-5 symptoms of cognitions and mood in traumatized children and adolescents: Two network approaches. Journal of Child Psychology and Psychiatry, 60(6), 545–554.
- Benfer, N., Bardeen, J. R., Cero, I., Kramer, L. B., Whiteman, S. E., Rogers, T. A., … Weathers, F. W. (2018). Network models of posttraumatic stress symptoms across trauma types. Journal of Anxiety Disorders, 58, 70–77.
- Birkeland, M. S., Blix, I., Solberg, Ø., & Heir, T. (2017). Gender differences in posttraumatic stress symptoms after a terrorist attack: A network approach. Frontiers in Psychology, 8, 2091.
- Birkeland, M. S., & Heir, T. (2017). Making connections: Exploring the centrality of posttraumatic stress symptoms and covariates after a terrorist attack. European Journal of Psychotraumatology, 8(sup3), 1333387.
- Borsboom, D. (2017). A network theory of mental disorders. World Psychiatry, 16(1), 5–13.
- Borsboom, D., Cramer, A., & Kalis, A. (2019). Brain disorders? Not really… Why network structures block reductionism in psychopathology research. Behavioral and Brain Sciences, 42, E2.
- Borsboom, D., & Cramer, A. O. (2013). Network analysis: An integrative approach to the structure of psychopathology. Annual Review of Clinical Psychology, 9, 91–121.
- Borsboom, D., Fried, E. I., Epskamp, S., Waldorp, L. J., van Borkulo, C. D., van der Maas, H. L., & Cramer, A. O. (2017). False alarm? A comprehensive reanalysis of “evidence that psychopathology symptom networks have limited replicability” by Forbes, Wright, Markon, and Krueger (2017). Journal of Abnormal Psychology, 126(7), 989–999.
- Brewin, C. R., Gregory, J. D., Lipton, M., & Burgess, N. (2010). Intrusive images in psychological disorders: Characteristics, neural mechanisms, and treatment implications. Psychological Review, 117(1), 210–232.
- Bringmann, L. F., Elmer, T., Epskamp, S., & Krause, R., Schoch, D., Wichers, M., ... & Snippe, E. (2019). What do centrality measures measure in psychological networks? Journal of Abnormal Psychology, 128(8), 892. doi:10.1037/abn0000446
- Bryant, R. A., Creamer, M., O’Donnell, M., Forbes, D., McFarlane, A. C., Silove, D., & Hadzi-Pavlovic, D. (2017). Acute and chronic posttraumatic stress symptoms in the emergence of posttraumatic stress disorder: A network analysis. JAMA Psychiatry, 74(2), 135–142.
- Cao, X., Wang, L., Cao, C., Fang, R., Chen, C., Hall, B. J., & Elhai, J. D. (2019). Sex differences in global and local connectivity of adolescent posttraumatic stress disorder symptoms. Journal of Child Psychology and Psychiatry, 60(2), 216–224.
- Contreras, A., Nieto, I., Valiente, C., Espinosa, R., & Vazquez, C. (2019). The study of psychopathology from the network analysis perspective: A systematic review. Psychotherapy and Psychosomatics, 88(2), 71–83.
- Costantini, G., Richetin, J., Preti, E., Casini, E., Epskamp, S., & Perugini, M. (2019). Stability and variability of personality networks. A tutorial on recent developments in network psychometrics. Personality and Individual Differences, 136, 68–78.
- Dablander, F., & Hinne, M. (2019). Node centrality measures are a poor substitute for causal inference. Scientific Reports, 9(1), 6846.
- De Ron, J., Fried, E. I., & Epskamp, S. (n.d.). Psychological networks in clinical populations: A tutorial on the consequences of Berkson’s Bias [ preprint]. Psychological Medicine, doi:10.31234/osf.io/5t8zw
- De Schryver, M., Vindevogel, S., Rasmussen, A. E., & Cramer, A. O. (2015). Unpacking constructs: A network approach for studying war exposure, daily stressors and post-traumatic stress disorder. Frontiers in Psychology, 6, 1896.
- Ehlers, A., & Clark, D. M. (2000). A cognitive model of posttraumatic stress disorder. Behaviour Research and Therapy, 38(4), 319–345.
- Epskamp, S., Borsboom, D., & Fried, E. (2018). Estimating psychological networks and their accuracy: A tutorial paper. Behavior Research Methods, 50(1), 195–212.
- Epskamp, S., Cramer, A. O., Waldorp, L. J., Schmittmann, V. D., & Borsboom, D. (2012). qgraph: Network visualizations of relationships in psychometric data. Journal of Statistical Software, 48(4), 1–18.
- Epskamp, S., & Fried, E. (2017). A tutorial on regularized partial correlation networks. Psychological Methods, 23(4), 617–634.
- Epskamp, S., Waldorp, L. J., Mõttus, R., & Borsboom, D. (2018). The Gaussian Graphical Model in cross-sectional and time-series data. Multivariate Behavioral Research, 53(4), 453–480.
- Foa, E. B., Huppert, J. D., & Cahill, S. P. (2006). Emotional processing theory: An update. In B. Rothbaum (Ed.), Pathological anxiety: Emotional processing in etiology and treatment (pp. 3–24). New York, NY: The Guilford Press.
- Forbes, M. K., Wright, A. G., Markon, K. E., & Krueger, R. F. (2017a). Further evidence that psychopathology networks have limited replicability and utility: Response to Borsboom et al. (2017) and Steinley et al. (2017). Journal of Abnormal Psychology, 126(7), 1011–1016.
- Forbes, M. K., Wright, A. G. C., Markon, K. E., & Krueger, R. F. (2017b). Evidence that psychopathology symptom networks have limited replicability. Journal of Abnormal Psychology, 126(7), 969–988.
- Fried, E. I., & Cramer, A. O. (2017). Moving forward: Challenges and directions for psychopathological network theory and methodology. Perspectives on Psychological Science, 12(6), 999–1020.
- Fried, E. I., Eidhof, M. B., Palic, S., Costantini, G., Huisman-van Dijk, H. M., Bockting, C. H., … Karstoft, K. (2018). Replicability and generalizability of PTSD networks: A cross-cultural multisite study of PTSD symptoms in four trauma patient samples. Clinical Psychological Science, 6(3), 335–351.
- Galatzer-Levy, I. R., & Bryant, R. A. (2013). 636,120 ways to have posttraumatic stress disorder. Perspectives on Psychological Science, 8(6), 651–662.
- Greene, T., Gelkopf, M., Epskamp, S., & Fried, E. (2018). Dynamic networks of PTSD symptoms during conflict. Psychological Medicine, 48(14), 2409–2417.
- Hallquist, M., Wright, A., & Molenaar, P. C. (2019). Problems with centrality measures in psychopathology symptom networks: Why network psychometrics cannot escape psychometric theory. Multivariate Behavioral Research, 1-25. doi:10.1080/00273171.2019.1640103
- Hoffart, A., Langkaas, T. F., Øktedalen, T., & Johnson, S. U. (2019). The temporal dynamics of symptoms during exposure therapies of PTSD: A network approach. European Journal of Psychotraumatology, 10(1), 1618134.
- Jones, P. J., Williams, D. R., & McNally, R. J. (n.d.). Sampling variability is not nonreplication: A Bayesian reanalysis of Forbes, Wright, Markon, & Krueger [ preprint].
- Keane, T. M., Marshall, A. D., & Taft, C. T. (2006). Posttraumatic stress disorder: Etiology, epidemiology, and treatment outcome. Annual Review of Clinical Psychology, 2, 161–197.
- Kelley, L. P., Weathers, F. W., McDevitt‐Murphy, M. E., Eakin, D. E., & Flood, A. M. (2009). A comparison of PTSD symptom patterns in three types of civilian trauma. Journal of Traumatic Stress, 22(3), 227–235.
- Krosnick, J. A. (2018). Questionnaire design. In D. L. Vannette & J. A. Krosnick (Eds.), The Palgrave handbook of survey research (pp. 439–455). Cham, Switzerland: Springer.
- McNally, R. J. (2016). Can network analysis transform psychopathology? Behaviour Research and Therapy, 86, 95–104.
- McNally, R. J., Heeren, A., & Robinaugh, D. J. (2017). A Bayesian network analysis of posttraumatic stress disorder symptoms in adults reporting childhood sexual abuse. European Journal of Psychotraumatology, 8(sup3), 1341276.
- McNally, R. J., Robinaugh, D. J., Wu, G. W., Wang, L., Deserno, M. K., & Borsboom, D. (2015). Mental disorders as causal systems: A network approach to posttraumatic stress disorder. Clinical Psychological Science, 3(6), 836–849.
- Mitchell, K. S., Wolf, E. J., Bovin, M. J., Lee, L. O., Green, J. D., Rosen, R. C., … Marx, B. P. (2017). Network models of DSM–5 posttraumatic stress disorder: Implications for ICD–11. Journal of Abnormal Psychology, 126(3), 355–366.
- Moher, D., Liberati, A., Tetzlaff, J., & Altman, D. G. (2009). Preferred reporting items for systematic reviews and meta-analyses: The PRISMA statement. Annals of Internal Medicine, 151(4), 264–269.
- Moshier, S. J., Bovin, M. J., Gay, N. G., Wisco, B. E., Mitchell, K. S., Lee, D. J., … Keane, T. M. (2018). Examination of posttraumatic stress disorder symptom networks using clinician-rated and patient-rated data. Journal of Abnormal Psychology, 127(6), 541–547.
- Olff, M. (2018). Psychotraumatology on the move. European Journal of Psychotraumatology, 9(1), 1439650.
- Opsahl, T., Agneessens, F., & Skvoretz, J. (2010). Node centrality in weighted networks: Generalizing degree and shortest paths. Social Networks, 32(3), 245–251.
- Phillips, R. D., Wilson, S. M., Sun, D., Workgroup, V. M.-A. M., & Morey, R. (2018). Posttraumatic stress disorder symptom network analysis in US military veterans: Examining the impact of combat exposure. Frontiers in Psychiatry, 9, 608.
- Ross, J., Murphy, D., & Armour, C. (2018). A network analysis of DSM-5 posttraumatic stress disorder and functional impairment in UK treatment-seeking veterans. Journal of Anxiety Disorders, 57, 7–15.
- Rubin, D. C., Berntsen, D., & Bohni, M. K. (2008). A memory-based model of posttraumatic stress disorder: Evaluating basic assumptions underlying the PTSD diagnosis. Psychological Review, 115(4), 985–1011.
- Russell, J. D., Neill, E. L., Carrion, V. G., & Weems, C. F. (2017). The network structure of posttraumatic stress symptoms in children and adolescents exposed to disasters. Journal of the American Academy of Child and Adolescent Psychiatry, 56(8), 669–677.
- Shevlin, M., & Elklit, A. (2012). The latent structure of posttraumatic stress disorder: Different models or different populations? Journal of Abnormal Psychology, 121(3), 610–615.
- Smith, K. E., Crosby, R. D., Wonderlich, S. A., Forbush, K. T., Mason, T. B., & Moessner, M. (2018). Network analysis: An innovative framework for understanding eating disorder psychopathology. International Journal of Eating Disorders, 51(3), 214–222.
- Spiller, T. R., Schick, M., Schnyder, U., Bryant, R. A., Nickerson, A., & Morina, N. (2017). Symptoms of posttraumatic stress disorder in a clinical sample of refugees: A network analysis. European Journal of Psychotraumatology, 8(sup3), 1318032.
- Stein, J. Y., Wilmot, D. V., & Solomon, Z. (2016). Does one size fit all? Nosological, clinical, and scientific implications of variations in PTSD Criterion A. Journal of Anxiety Disorders, 43, 106–117.
- Steinley, D., Hoffman, M., Brusco, M. J., & Sher, K. J. (2017). A method for making inferences in network analysis: Comment on Forbes, Wright, Markon, and Krueger (2017). Journal of Abnormal Psychology, 126(7), 1000–1010.
- Sullivan, C. P., Smith, A. J., Lewis, M., & Jones, R. T. (2018). Network analysis of PTSD symptoms following mass violence. Psychological Trauma: Theory, Research, Practice, and Policy, 10(1), 58–66.
- Tourangeau, R., & Rasinski, K. A. (1988). Cognitive processes underlying context effects in attitude measurement. Psychological Bulletin, 103(3), 299–314.
- von Stockert, S. H., Fried, E. I., Armour, C., & Pietrzak, R. H. (2018). Evaluating the stability of DSM-5 PTSD symptom network structure in a national sample of US military veterans. Journal of Affective Disorders, 229, 63–68.
- Weathers, F. W., Litz, B. T., Herman, D. S., Huska, J. A., & Keane, T. M. (1993). The PTSD checklist (PCL): Reliability, validity, and diagnostic utility. Paper presented at the Annual meeting of the international society for traumatic stress studies, San Antonio, TX.
- Williams, D. R., Rast, P., Pericchi, L., & Mulder, J. (n.d.). Comparing Gaussian Graphical Models with the posterior predictive distribution and Bayesian model selection [ Preprint]. doi:10.31234/osf.io/yt386