ABSTRACT
Background: Disasters negatively impact mental health and well-being. Studying how people adapt and recover after adversity is crucial for disaster preparedness and response.
Objective: This study examined how differentially affected communities harness their resources to adapt to the aftermath of a flood. We predicted that stronger individual, interpersonal, and community resources protect against psychosocial resource loss and, through that, are related to fewer symptoms of posttraumatic stress and depression and higher life satisfaction. We also predicted that these effects would be stronger in a flooded community, compared to a threatened, but non-flooded community.
Method: Participants were randomly sampled community members from two neighbouring municipalities. One municipality was severely flooded during the 2014 floods in South East Europe (affected community, na = 223), the other was threatened but not flooded (comparison community, nc = 224). Interviews were conducted one and a half years after the disaster using the Connor-Davidson Resilience Scale 10-item version, the Multidimensional Scale of Perceived Social Support, the Community Resources Scale, the Psychosocial Resource Loss Scale, the PTSD Checklist for DSM-5, the Center for Epidemiological Studies Depression Scale Revised and the Satisfaction with Life Scale.
Results: Stronger individual, interpersonal, and community resources were found to be related to better post-disaster outcomes directly and indirectly through psychosocial resource loss. In the affected community, interpersonal resources and community social capital and engagement were stronger predictors of positive adaptation. In the comparison community, community economic development and trust in community leadership were more important.
Conclusion: This study provides evidence that people affected by disasters can harness their individual, interpersonal, and community resources to recover and adapt. Post-disaster interventions should aim to strengthen family and community ties, thus increasing available social support and community connectedness.
Highlights
• Psychosocial resource loss after disasters can be reduced by harnessing individual, interpersonal and community resources.
• This is then related to better mental health and wellbeing.
• Post-disaster interventions should aim to strengthen social support and community connectedness.
Antecedentes: Las catástrofes tienen un impacto negativo en la salud mental y el bienestar. Estudiar cómo se adaptan y se recuperan las personas tras la adversidad es crucial para la preparación y la respuesta ante los desastres.
Objetivo: Este estudio examinó cómo las comunidades afectadas de forma diferencial aprovechan sus recursos para adaptarse a las secuelas de una inundación. Predecimos que contar con recursos individuales, interpersonales y comunitarios más fuertes protegen contra la pérdida de recursos psicosociales y, a través de ello, se relacionan con menos síntomas de estrés postraumático y depresión y una mayor satisfacción vital. También predijimos que estos efectos serían más fuertes en una comunidad inundada, en comparación con una comunidad amenazada, pero no inundada.
Método: Los participantes fueron miembros de la comunidad seleccionados al azar de dos municipios vecinos. Uno de los municipios sufrió graves inundaciones durante las inundaciones del 2014 en el sureste de Europa (comunidad afectada, na = 223), el otro, estuvo amenazado pero no se inundó (comunidad de comparación, nc = 224). Las entrevistas se realizaron un año y medio después del desastre, utilizando la versión de 10 ítems de la Escala de Resiliencia de Connor-Davidson, la Escala Multidimensional de Apoyo Social Percibido, la Escala de Recursos Comunitarios, la Escala de Pérdida de Recursos Psicosociales, la Lista de Verificación del TEPT para el DSM-5, la Escala de Depresión Revisada del Centro de Estudios Epidemiológicos y la Escala de Satisfacción con la Vida.
Resultados: Los recursos individuales, interpersonales y comunitarios más fuertes se relacionaron con mejores resultados después de la catástrofe, directamente e indirectamente a través de la pérdida de recursos psicosociales. En la comunidad afectada, los recursos interpersonales y el capital social y el compromiso de la comunidad fueron los mayores predictores de una adaptación positiva. En la comunidad de comparación, el desarrollo económico de la comunidad y la confianza en el liderazgo comunitario fueron más importantes.
Conclusión: Este estudio aporta pruebas de que las personas afectadas por catástrofes pueden aprovechar sus recursos individuales, interpersonales y comunitarios para recuperarse y adaptarse. Las intervenciones posteriores a las catástrofes deberían tener como objetivo reforzar los lazos familiares y comunitarios, aumentando así el apoyo social disponible y la conectividad de la comunidad.
背景: 灾难对心理健康和幸福产生负面影响。研究人们在逆境后如何适应和恢复, 对于灾害预防和反应至关重要。
目的: 本研究考查了受到不同影响的社区如何利用其资源来适应洪水的后果。我们预测, 更强大的个人, 人际关系和社区资源可保护人们免受心理社会资源损失, 并因此与更少的创伤后应激和抑郁症状以及更高的生活满意度相关。我们还预测, 与受到洪水威胁但未遭遇洪水的社区相比, 在遭遇洪水的社区中, 这些效应会更强。
方法: 参与者是从两个邻近城市随机抽样的社区成员。一个城市在2014年东南欧洪水期间遭受了严重洪灾 (受影响社区, na = 223), 另一个城市则受到洪水威胁但未遭遇洪水 (对比社区, nc = 224) 。在灾难发生一年半后, 使用10条目版Connor-Davidson韧性量表, 感知社会支持多维量表, 社区资源量表, 社会心理资源损失量表, DSM-5 PTSD检查表, 流行病学研究中心抑郁量表修订版和生活满意度量表进行访谈。
结果: 人们发现, 更强大的个人, 人际关系和社区资源直接或间接地通过心理社会资源损失与更好的灾后结果有关。在受影响的社区中, 人际关系资源, 社区社会资本和参与度是积极适应的更强预测指标。在对比社区中, 社区经济发展和对社区领导的信任更重要。
结论: 本研究提供了受灾难影响的人们可以利用其个人, 人际关系和社区资源来恢复和适应的证据。灾后干预措施应旨在加强家庭与社区的联系, 从而增加可用的社会支持和社区联系。
1. Introduction
As weather-related disasters are becoming more frequent and with more people living in disaster-prone areas, mitigating the impact of disasters is becoming increasingly important. Disasters negatively impact individuals and communities: they increase the incidence of mental health disorders and decrease the quality of life (Norris et al., Citation2002). The mental health consequences of disasters persist in the long-term period for a significant number of survivors. For example, two years after widespread flooding in the UK, the prevalence of anxiety and depression among survivors was higher than 10% and the prevalence of probable PTSD higher than 20% (Jermacane et al., Citation2018). Furthermore, a meta-analysis of 14 studies of PTSD among survivors of flooding showed the incidence of PTSD was 11.5% in the period longer than 6 months post-disaster (Chen & Liu, Citation2015). These detrimental effects can endure even decades after the disaster (Raker et al., Citation2019). Still, a substantial number of survivors experience only transient distress or successfully recover from disaster impact (Bonanno, Brewin, Kaniasty, & La Greca, Citation2010). Therefore, studying resilience as a dynamic process of maintaining and recovering psychological well-being after adversity is one of the key challenges in preparing for future disasters.
Resources are regarded as central to the resilience process because recovery, positive adaptation, wellness, and well-being in high-risk situations result from harnessing available resources. There is ample research showing that individual level resources contribute to the positive adaptation after disasters. Perceived control and sense of mastery, sense of coherence, ability to ‘bounce back’, generalized sense of efficacy and disaster-specific coping self-efficacy, hardiness, self-esteem, and positive affect have all been found to contribute to better mental health outcomes (Ahmad et al., Citation2010; Benight et al., Citation1999; Bonanno et al., Citation2010; Braun-Lewensohn & Sagy, Citation2014; Kaniasty, Citation2006; Ying, Wu, Lin, & Jiang, Citation2014). However, the extensive focus on individual resources has been criticized for a number of reasons. First, previous studies show that no single resource or trait has a dominant influence on post-disaster outcomes; rather, they seem to each explain a relatively small part of the variance (Bonanno, Romero, & Klein, Citation2015). Second, the relative importance of a particular resource can change along with the contextual circumstances as some resources become more or less accessible (Hobfoll, Citation1989, Citation2002). Finally, some also argue that it is not pragmatic to focus on those resources that are less likely to be enhanced by (post-disaster) interventions (Masten, Best, & Garmezy, Citation1990).
It is commonly recognized that multiple ecological systems play a part in the resilience process, including close relationships, community systems, and built and natural environment (Maercker & Hecker, Citation2016; Ungar & Theron, Citation2020). A rich body of literature in community (for an overview see e.g. Harvey, Citation2007) and developmental psychology (for an overview see e.g. Masten & Narayan, Citation2012) highlights the importance of person-environment interactions in complex and changing social contexts that shape resilience. Disaster researchers have recently begun to integrate the ecological framework in the study of resilience-promoting resources. One resource that has received particular attention is social support, which has consistently been shown to be related to better mental health and overall functioning after disasters (Bonanno et al., Citation2010; Norris & Kaniasty, Citation1996; Norris, Stevens, Pfefferbaum, Wyche, & Pfefferbaum, Citation2008). At the community level, the most commonly studied resource has been social capital, the construct that encompasses feelings of trust and belonging to a community, attachment to the community, and engagement and participation of community members (Bonanno et al., Citation2015). Social capital and community efficacy were found to be related to posttraumatic stress (PTS) in the aftermath of an earthquake and a tsunami (Hikichi, Aida, Tsuboya, Kondo, & Kawachi, Citation2016), a hurricane (Ursano et al., Citation2014) and a flood (Wind & Komproe, Citation2012). Furthermore, studies conducted in the context of prolonged political violence showed that the availability of community resources, defined as a composite of leadership, collective efficacy, preparedness and social capital, were related to stress reactions (Braun-Lewensohn & Sagy, Citation2014).
At the same time, there are still gaps in our understanding of resources that promote disaster resilience. In a recent review, Bonanno et al. (Citation2015) substantiate that the study of resilience-promoting factors after disasters with regards to communities lags behind that of individual-level factors, despite rich theoretical conceptualizations. Studies that do look into community resources typically research how these resources relate to individual psychological adaptation, rather than community adaptation assessed across community units (Bonanno et al., Citation2015). This limits current understanding of disaster resilience for two reasons. First, researchers agree that community adaptation cannot be identified in studies of individuals within only one population as the overall average at the level of the community hides the variability across community units (Norris et al., Citation2008). Second, this approach fails to identify potential differences between resilience and recovery processes in different communities. Identifying protective factors that interact with the level of risk is one of the central objectives of resilience research as they help uncover the potential mechanisms that underlie resilience (Luthar, Cicchetti & Becker, Citation2000). It is also an important strategy for disaster preparedness and response as it can point out resources that are particularly important in the aftermath of disasters. A couple of notable studies highlight this point. For example, Benight (Citation2004) found that collective efficacy was a stronger predictor of distress in a community affected by a flood than in a similar, non-affected community, reaffirming the importance of social resources in coping with disasters. Moreover, West, Price, Gros, and Ruggiero (Citation2013) found that the relationship between community support and mental health after a hurricane differs across types of community: whereas community support buffers against psychological distress in nonurban areas, that effect was not observed in an urban sample. These findings provide strong evidence that community recovery is tied to the wider social context. However, more studies that look into key resources across ecological levels are needed (Bonanno et al., Citation2015).
Furthermore, given the importance of resources for post-disaster adaptation, it is critical to study the processes behind building up and maintaining resources (Southwick, Bonanno, Masten, Panter-Brick, & Yehuda, Citation2014). According to the Conservation of Resources theory (COR), when confronted with an event threatening to deplete one’s resources (e.g. job loss), people try to offset such losses by drawing on available resources in their environment (e.g. savings, social networks) (Hobfoll, Citation1989, Citation2002). Because of this role of resources in the coping process, a threat of resource loss, their actual loss, or a lack of resource gain after investment of resources lead to psychological distress. The Conservation of Resources theory has been extensively applied to disaster research, with numerous studies showing that resource loss has consistently been found to be one of the strongest predictors of symptoms of psychological distress after disasters (Benight et al., Citation1999), over and above disaster exposure, previous exposure to stress and pre-disaster mental health (Hobfoll, Tracy, & Galea, Citation2006; Zwiebach, Rhodes, & Roemer, Citation2010).
Even though all coping efforts include the use and, therefore, expenditure of resources, where resources are abundant the final resource sum should remain unchanged or even increase (Hobfoll, Citation2002). In other words, to achieve good post-disaster adaptation, resources need to be diverse in a sense that multiple resources exist in a substitutable manner (Norris et al., Citation2008). However, few studies up to date have looked into how resource loss can be prevented and how resource redundancy can be used to mitigate it. Some studies found evidence that having strong social support does indeed reduce later resource loss, which can in turn positively contribute to post-disaster outcomes (Littleton, Axsom, & Grills-Taquechel, Citation2009; Norris & Kaniasty, Citation1996). Moreover, an illustrative multi-level study conducted one year after a flood showed that in communities with higher social capital individuals employed fewer individual psychosocial resources to cope with the effects of the disaster (Wind & Komproe, Citation2012).Yet, these studies examined only one aspect of the social context and did not look into other potential community level resources, such as economic resources, preparedness or leadership. Furthermore, they focused mainly on mental health outcomes, rather than overall wellness, that also includes positive adaptation such as life satisfaction.
The present study aims to build on existing research and address previously identified gaps. We analysed how people in communities that were differentially exposed to a flood harness different resources to mitigate psychosocial resource loss and therefore experience better post-disaster outcomes. To address existing gaps, we studied resources at different levels of ecological context and multiple outcomes that go beyond mental health, we analysed the differences in the contributions of resources across differentially exposed communities, and how harnessing available resources is related to reducing resource loss. We made two predictions about the relationship between resources, psychosocial resource loss and indicators of adaptation. First, we predicted that having stronger resources at the individual, interpersonal, and community level will be related to less psychosocial resource loss after a disaster, and through that to lower symptoms of posttraumatic stress (PTS) and depression and higher life satisfaction. Second, we predicted that this relationship will be stronger in a flooded community than in a threatened, but non-flooded comparable community.
2. Method
2.1. Event
We conducted the study after the 2014 floods in South East Europe. In Croatia, more than 300,000 people were endangered along the critical 67 kilometres of the River Sava embankment. Despite lavish efforts to reinforce the embankment by emergency responders and community members, it eventually breached on 17 May, causing severe flooding in several municipalities. Two persons died and more than 13,000 were evacuated. Some of these people had to be rescued from their flooded homes due to the suddenness of the water surge and refusal of early(-ier) evacuation.
2.2. Participants and procedure
Participants were community members from two neighbouring municipalities in Croatia (N = 447). Half of the participants (n = 223) were residents of the most severely flooded municipality (referred hereinafter as the ‘affected community’); the other half (n = 224) lived in a nearby municipality, about 30 km away, that was not flooded, but was threatened by the flood and narrowly escaped the disaster (‘comparison community’). The sample size was large enough to detect small to medium differences in correlation coefficients (Cohen’s q = 0.27) with a probability of 80% (Faul, Erdfelder, Buchner, & Lang, Citation2009).
The comparison community was carefully selected based on its proximity and similarity on a number of parameters to the affected community according to 2011 census data. The two communities were similar in terms of size, population age and gender composition, educational attainment, and economic indicators. A known a priori difference was the larger percentage of ethnic minorities members in the affected community than in the neighbouring communities that were almost exclusively populated by ethnic Croats. We acknowledged this difference in the subsequent analysis, however, having a deep insight of the socio-cultural-political context of the region, we did not expect it would impact the results. Moreover, even though ethnicity is sometimes found to be related to post-disaster outcomes, it is often confounded with other factors, such as socioeconomic status (Bonanno et al., Citation2010). Where socioeconomic status is controlled for, the effects of ethnic differences are no longer found (Bonanno, Galea, Bucciarelli, & Vlahov, Citation2007). As the two studied communities are among the most disadvantaged ones in Croatia based on a number of economic criteria, we expected that this context will similarly impact both communities and overpower the possible differences related to ethnic status. Finally, the minority in question is regarded as well and fully integrated in the Croatian society, enjoying full and equal civic status and benefits as any other citizen, as is often emphasized by the leaders of this ethnic group (e.g. Šoštarić, Citation2014), and found in studies focusing on social distance (Vujević Hećimović, Brajović, & Ilin, Citation2010).
We used a stratified random sample in which a proportionate number of households were randomly selected in each street based on a registry of household numbers. Participants were eligible for the study if they had been between 25 and 65 years old, had lived in the community at least 5 years prior to the flood, and had been present in the community in the period leading to the flood. If several participants in one household were eligible for the study, one participant was randomly selected. Up to three attempts were made to contact the participant. In case the participant was not eligible or refused to participate, a new household was randomly selected. The response rate was 71% in the affected community and 57.8% in the comparison community. The main reasons for failing to participate in the affected community were lack of time or not wanting to be reminded of the floods; in the comparison community they were lack of time and not seeing the benefits of the study. In the affected community, 6.0% of the community members participated in the study; in the comparison community, 5.7% of the community members participated.
The study was conducted in November 2015, after the majority of the affected community residents returned to the municipality. It was supported and announced by several community leaders, including local self-government, school principals, and religious leaders. The individual face-to-face interview was conducted in the homes of the participants and privacy during the interview was ensured. The interview lasted about one hour. Interviewers underwent extensive training prior to the beginning of the study. The local language was used and the instruments were either validated prior to this study or developed specifically for the local context and pre-piloted. When prior validation did not exist, experts fluent in both English and the local language translated and back translated the instrument (Resource Loss Scale, PTSD Checklist for DSM-V). The study was approved by the Ethical Board of the Department of Psychology, Faculty of Humanities and Social Sciences, University of Zagreb. Signed informed consent was obtained from all participants and an individual code was assigned to every participant to assure confidentiality. If a participant reported symptoms of distress, s/he was provided with information on stress and coping and referred where to seek help.
2.3. Measures
2.3.1. Individual resources
Individual resources were assessed as the ability to tolerate and bounce back from change, problems, illness, pressure, failure, and painful feelings (e.g. Can deal with whatever comes) with the Connor-Davidson Resilience Scale 10-item version (Campbell-Sills & Stein, Citation2007). Responses ranged from 0 (not true at all) to 4 (true nearly all the time). Scores were calculated as an average response across items with higher scores indicating stronger individual resources (αtotal = .88, αaffected = .89, αcomparison = .88).
2.3.2. Interpersonal resources
Interpersonal resources were assessed by the 12-item Multidimensional Scale of Perceived Social Support (Zimet, Dahlem, Zimet, & Farley, Citation1988) as a perception of support from family (e.g. My family really tries to help me), friends (e.g. I can talk about my problems with my friends) and significant other (e.g. There is a special person who is around when I am in need). Responses ranged from 1 (strongly disagree) to 7 (strongly agree). Scores were calculated as an average response across items with higher scores indicating stronger interpersonal resources (αt = .91, αa = .91, αc = .92).
2.3.3. Community resources
Community resources were defined as individual-level perceptions of community social capital and engagement (6 items, e.g. There is trust between members of community; Community members work together to solve problems), economic development (4 items, e.g. There are diverse ways to secure livelihood), preparedness (4 items, e.g. People know what to do in case of a disaster) and leadership (6 items, e.g. Community members trust the local authorities). We measured community resources using the Community Resources Scale (Bakic, Citation2017) that was developed in the context of the 2014 flooding. Responses ranged from 0 (not at all) to 4 (to a full extent). Scores were calculated as an average response across items with higher scores indicating stronger community resources (αt = .92, αa = .89, αc = .92).
2.3.4. Psychosocial resource loss
Psychosocial resource loss measure was measured using a list of key resources proposed by Hobfoll et al. (Citation2006) that reflect the five essential elements of mass trauma intervention – promoting feelings of safety, calming, a sense of self- and community efficacy, connectedness, and hope (Hobfoll et al., Citation2007). Participants rated the amount of loss of 11 resources since the flood: time for adequate sleep, feeling valuable to others, free time, feeling of accomplishing one’s goals, time with loved ones, sense of optimism, sense of humour, feeling of control over one’s life, feeling that life is peaceful, motivation to get things done, and feeling that life has a purpose. Responses ranged from 0 (not at all) to 4 (to a large extent). Scores were calculated as an average response across items with higher scores indicating greater resource loss (αt = .9, αa = .89, αc = .84).
2.3.5. Posttraumatic stress symptoms
Posttraumatic stress (PTS) symptoms in the past month were assessed with the 20-item PTSD Checklist for DSM-V (Weathers et al., Citation2013). Responses ranged from 0 (not at all) to 4 (extremely). Scores were calculated as an average response across items with higher scores indicating a more severe level of PTS symptoms (αt = .93, αa = .93, αc = .92). When calculating rates of probable PTSD, a cut-off score of 33 was used with a score calculated as a sum of all responses (Bovin et al., Citation2016).
2.3.6. Depression symptoms
Depression symptoms in the past two weeks were assessed with the 20-item Center for Epidemiologic Studies Depression Scale Revised (Eaton, Smith, Ybarra, Muntaner, & Tien, Citation2004). Responses ranged from 0 (not at all or less than 1 day last week) to 4 (nearly every day for two weeks). Scores were calculated as an average response across items with higher scores indicating higher depression symptoms (αt = .94, αa = .94, αc = .93). When calculating rates of probable depression, a cut-off score of 16 was used with a score calculated as a sum of all responses (Eaton et al., Citation2004).
2.3.7. Life satisfaction
Life satisfaction was measured with the 5-item Satisfaction with Life Scale (Diener, Emmons, Larsen, & Griffin, Citation1985) as a global assessment of quality of life (e.g. I am satisfied with my life). Responses ranged from 1 (strongly disagree) to 7 (strongly agree). Scores were calculated as an average response across items with higher scores indicating higher life satisfaction (αt = .88, αa = .88, αc = .88).
2.3.8. Socioemografic variables and exposure
Sociodemografic variables included age, gender, war veteran status, ethnic background, employment, education, and marital status. Exposure to traumatic events prior to the flood was assessed with Trauma History Screen (Carlson et al., Citation2011) where participants reported experiencing or not 14 potential traumatic events. Exposure to 2014 floods was measured by a single item referring to feeling life threat (no/yes). The participants were also asked if they had attended psychological counselling of therapy prior to the flooding.
2.4. Data analysis
First, we analysed descriptive statistics in the affected and the comparison communities. Then, we examined the bivariate relationships between the study variables (Pearson r). Finally, we tested the proposed process of resilience with structural equation modelling in lavaan (Rosseel, Citation2012). Missing data was treated using full information maximum likelihood that was shown to result in less bias than ad hoc missing data techniques (Enders, Citation2001).
To test the proposed model, we first specified all study variables as latent constructs measured by parcels (Little, Rhemtulla, Gibson, & Schoemann, Citation2013). As the use of parcels can mask model misspecifications, especially at the measurement model, we followed the recommendations on constructing parcels for the unidimensional and multidimensional constructs (for a review of critical issues in the use of parcels see e.g. Marsh, Lüdtke, Nagengast, Morin, & Von Davier, Citation2013). For the constructs that met the assumption of unidimensionality, we constructed parcels based on item loadings and residual covariance, assigning items with correlated unique terms to a single parcel (Marsh et al., Citation2013). We parcelled the multidimensional constructs (as measured by the Multidimensional Scale of Perceived Social Support, the Community Resources Scale, and the PTSD Checklist for DSM-V) homogenously, meaning that all items corresponding to one factor were included in one parcel Three parcels were calculated for each latent construct, except for the Community Resource Scale that has 4 factors.
We then tested the relationship of different levels of resources, resource loss, and positive adaptation on the whole sample (N = 447). The model included direct and indirect paths from the individual, interpersonal, and community resources on one side, and indicators of positive adaptation – PTS, depression symptoms, and life satisfaction – on the other side. Indirect paths were estimated through psychosocial resource loss. Then, we used multigroup structural equation modelling to test whether exposure to a flood moderated the indirect relationship of resources and positive adaptation through resource loss. To do so, we fitted the model in both the affected and the comparison communities and compared the size of indirect coefficients. Finally, we analysed the relationship of different factors of the Community Resource Scale to resource loss and positive adaptation in both affected and comparison communities. Additionally, we tested a number of alternative models to clarify the order of the variables in the model and to test the potential influence of confounding variables to the relationships of interest. Where the addition of control variables did not change the results, the more parsimonious model without control variables was kept.
To mitigate the effects of non-normal multivariate distribution, we calculated parameter estimates using maximum likelihood estimation with robust standard errors and scaled test statistics (Zhong & Yuan, Citation2011). We analysed the fit of the models using Hu and Bentler (Citation1999) criteria for the Root Mean Square Error of Approximation (RMSEA), the Bentler Comparative Fit Index (CFI) and the Standardized Root Mean Square Residual (SRMR). Indirect regression coefficients and their differences between the affected and comparison communities were found significant if their 95% confidence interval (CI) calculated from the empirical sampling distribution based on 1000 samples did not contain 0. This approach has been found to have the best balance of Type I error and statistical power when testing the mediation hypothesis (e.g. MacKinnon, Lockwood, Hoffman, West, & Sheets, Citation2002).
3. Results
3.1. Descriptive statistics and correlation analysis
As expected, a significantly higher number of participants in the affected community identified themselves as belonging to an ethnic minority (affected community: na = 78; comparison community: nc = 2; X2 (1, N = 447) = 87.98, p < .001). Therefore, shows the sample characteristics for the affected community, broken down by minority status, and the comparison community. There were only a few identified differences between the majority and minority nationals living in the affected community. Compared to the majority nationals and the comparison community, minority nationals less often declared themselves as war veterans, were more often unemployed after the flood, and more often reported feeling life threat during the flood. Interestingly, comparison community members more often reported feeling life threat during the flood compared to majority nationals in the affected community. They were also more likely to have attained higher education and had marginally more traumatic experiences prior to the flooding compared to the overall sample in the affected community.
Table 1. Summary of sample characteristics in the affected and comparison community and results of difference testing between the affected (majority and minority nationals) and comparison community
However, we observed notable differences in study variables between the two communities: members from the affected community reported having fewer community resources, more psychosocial resource loss, and higher symptoms of PTS and depression. No differences were found between majority and minority nationals in the affected community. The rates of probable PTSD in the affected community were 32.4% (95% CI: 24.8% – 40.7%) for the majority nationals and 33.3% (95% CI: 23.1% – 43.6%) for the minority nationals, compared to 21.9% (95% CI: 16.5% – 27.7%) in the comparison community. For probable depression, the rates were 35.9% (95% CI: 28.3% −44.4%) for the majority nationals and 34.6% (95% CI: 24.4% – 44.9%) for the minority nationals, compared to 23.7% (95% CI: 18.3% – 29.5%) in the comparison community. As there were no significant differences in the study variables between the majority and minority nationals in the affected community, subsequent analysis were conducted on all members of the affected community together.
Correlations between the variables are presented in . The relationships between the study variables were all significant, small to medium in size, and in the expected direction. There were some significant relationships between sample characteristics that were found to be different in the affected and the comparison community and the study variables. War veteran status was significantly related to less interpersonal resources; higher education was related to fewer symptoms of PTS; the number of traumatic events experienced before the flooding and feeling life threat during the flooding were related to more psychosocial resource loss, higher symptoms of PTS and depression and lower life satisfaction. These correlations were small. Unemployment was not significantly related to any of the study variables.
Table 2. Summary of intercorrelations for scores on the study variables and intercorrelations between control and study variables (N = 447)
3.2. Structural model: the indirect effect of resource loss
Results of model testing among all participants are shown in . The model fitted the data well (χ2 (188) = 302.8, p < .001; CFI = .98; RMSEA (90% CI) = .04 (.03 – .05); SRMR = .04) and all parcels loaded on the respective factors significantly (p < .001) and strongly (.59 – .94). The model showed significant direct relationship between resources and positive adaptation. Stronger individual resources were related to lower symptoms of PTS and depression and higher life satisfaction. Stronger interpersonal resources were related to lower symptoms of depression and higher life satisfaction. Psychosocial resource loss was related to higher symptoms of both PTS and depression symptoms and lower life satisfaction. Furthermore, stronger individual and community resources were related to less psychosocial resource loss since the flood. Overall, the model accounted for 18% of the variance in resource loss, 42% of the variance in PTS symptoms, 33% of the variance in depression symptoms, and 51% of the variance in life satisfaction. There were no significant direct associations between interpersonal resources and psychosocial resource loss (β = −0.06, p = 0.414) and PTS symptoms (β = −0.08, p = 0.187), nor between community resources and PTS symptoms (β = 0.07, p = 0.128), depression symptoms (β = 0.02, p = 0.656), and life satisfaction (β = 0.06, p = 0.172).
Figure 1. Relationship between individual, interpersonal and community resources, resource loss and positive adaptation in a community sample (N = 447)
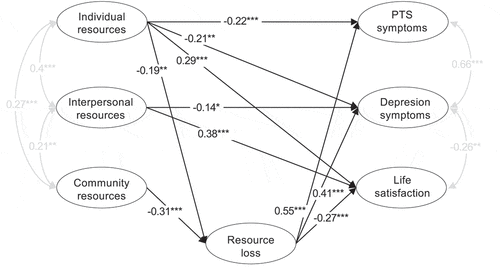
We found significant indirect relationships between resources and positive adaptation through psychosocial resource loss. Having stronger individual resources was related to less psychosocial resource loss and thus to lower PTS (b = −0.12, 95% CI: −0.2, −0.03) and depression symptoms (b = −0.08, 95% CI: −0.15, −0.03), and to higher life satisfaction (b = 0.1, 95% CI: 0.04, 0.19). The same was found for community resources: stronger resources were also related to less psychosocial resource loss and through that to lower PTS (b = −0.2, 95% CI: −0.29, −0.14) and depression symptoms (b = −0.14, 95% CI: −0.22, −0.09), as well as to higher life satisfaction (b = 0.17, 95% CI: 0.1, 0.26). Indirect relationships between interpersonal resources and positive adaptation were not significant (for PTS symptoms: b = −0.03, 95% CI: −0.12, 0.04; for depression symptoms: b = −0.02, 95% CI: −0.08, 0.03; for life satisfaction b = 0.03, 95% CI: −0.03, 0.09).
3.2.1. Alternative model
To further test the adequacy of the proposed model, we tested an alternative model where we reversed the role of assumed predictors and outcomes; that is, we tested whether mental health and life satisfaction were related to psychosocial resource loss, and through that to the levels of resources. As the two models have exactly the same fit to the data, no statistical decision of the model adequacy could have been made. However, analysis of the size and significance of the indirect effects (Table S1 in the Supplementary Material) has shown that the estimates of indirect effects were mostly not significant, and for those that were, the effects were smaller than in the proposed model. Therefore, the alternative model had little practical significance in explaining the data.
3.3. Structural model: the moderating effect of exposure
To analyse the moderating effect of exposure to a flood, the model was tested in the affected and the comparison community. First, we established that the measurement model was the same in the two communities, since the partial intercept invariance model resulted in a good fit (χ2 (404) = 544.5, p < .001; CFI = .97; RMSEA (90% CI) = .04 (.03 – .05); SRMR = .05), that was not different from the loading invariance model (Δχ2 (13) = 20.5, p = .084). Then, we compared this model, where all regression coefficients were freely estimated, to a constrained model, where all regression coefficients were constrained to be equal in the two communities. As the constrained model resulted in a worse fit (Δχ2 (15) = 29.8, p = .013), we proceeded to calculate the differences in the size of indirect path coefficients and their bootstrap confidence intervals.
The estimates of indirect path coefficients in the affected and comparison communities are presented in . In the affected community, individual and interpersonal resources were more strongly related to positive adaptation through lower psychosocial resource loss. For individual resources, the difference was significant for PTS symptoms, while for interpersonal resources it was significant for all indicators of positive adaptation. In the comparison community, indirect paths from community resources to positive adaptation were all significant; however, compared to the affected community, only the path to life satisfaction was significantly stronger. The entire model accounted for 19% of the variance in resource loss, 53% of the variance in PTS symptoms, 38% of the variance in depression symptoms, and 56% of the variance in life satisfaction in the affected community. In the comparison community, it explained 13% of the variance in resource loss, 21% of the variance in PTS symptoms, 18% of the variance in depression symptoms, and 46% of the variance in life satisfaction.
Table 3. Unstandardized regression coefficients, standard errors and confidence intervals for indirect paths between individual, interpersonal and community resources and PTS and depression symptoms and life satisfaction through psychosocial resource loss in the affected and the comparison community (na = 223, nc = 224)
3.3.1. Alternative models
To test whether or not the differences in sociodemographic variables between the affected and comparison communities impacted the results, we have analysed three alternative models. First, we have fitted the model in the affected community only, comparing the majority and minority nationals. The results of the model testing (Table S2 in the Supplementary Material) show that 1) the partial intercept invariance model fitted well in the two subgroups, showing that the measured constructs did not differ between the two groups, and that 2) constraining the structural regression coefficients had no impact on the model fit, meaning that all structural regression coefficients were the same in the two subgroups of the affected community. Then, we fitted the model again in the affected and comparison community, but this time using only the results from majority nationals. The measurement model did not differ between the two groups; however, there were significant differences in regression paths between the two communities (Table S3 in the Supplementary Material). Comparing the estimates of indirect path coefficients from this model (Table S4 in the Supplementary Material) to the coefficients from the full sample () shows that the magnitudes of the effects and their differences were unchanged. Finally, we fitted the model in the affected and comparison communities controlling for sociodemographic variables that were found related to the study variables, namely, war veteran status, education, number of traumatic events before the flood and feeling life threat. The model fitted the data well (χ2 (562) = 780.1, p < .001; CFI = .96; RMSEA (90% CI) = .04 (.04 – .05); SRMR = .06). Again, the estimates of indirect path coefficients (Table S5 in the Supplementary Material) did not differ from the model without control variables ().
3.4. Supplementary analysis: a closer look at community resources
To further clarify the role of community resources, we analysed the relationship of the four subscales of the Community Resources Scale with psychosocial resource loss and indicators of positive adaptation. The model fitted the data well (χ2 (184) = 282.2, p < .001; CFI = .98; RMSEA (90% CI) = .05 (.04 – .06); SRMR = .04) and the results of testing the indirect paths are presented in Table S6 in the Supplementary Material. In the affected community, the social capital and community engagement subscale was more strongly indirectly related to symptoms of PTS and depression, and marginally more strongly related to life satisfaction. In the comparison community, the economic development subscale was significantly indirectly related to all indicators of positive adaptation; in the affected community, there were no significant relationships. However, the difference in paths did not reach statistical significance. Finally, in the comparison community, the leadership subscale was more strongly indirectly related to all indicators of positive adaptation. Preparedness was not significantly related to the outcomes in either community.
4. Discussion
The results of this study indicate that, one and a half years after the disaster, the affected community is still recovering. The rates of probable PTSD and depression were significantly higher in the affected community than in the comparison community. With over 32% of the community members at risk of PTSD and almost 36% at risk of depression, our results indicate worse mental health outcomes than in similar studies of the consequences of flooding (Chen & Liu, Citation2015; Jermacane et al., Citation2018). As the rates of probable mental health disorders were high in the comparison community as well (over 20%), the mental health consequences were likely exacerbated by prior exposure to traumatic events, as this whole area of the country has been severely affected during the Croatian Homeland War (1991–1995). Traumatic pre-exposure is a well-known factor of greater mental health risks in repeated expose to adversity (Lowe, Bonumwezi, Valdespino-Hayden, & Galea, Citation2019). At the same time, life satisfaction did not differ between the communities, highlighting the notion that positive adaptation is more than mental health and can co-occur with mental health symptoms (Southwick et al., Citation2014). Individual and interpersonal resources did not differ between the two communities, indicating relative stability or recovery at the level of the community. However, the affected community experienced more psychosocial resource loss and estimated less availability of community resources than the comparison community. These findings are in line with the previous research showing that disasters increase psychosocial resource losses and that the level of exposure is related to the depletion of community resources (Benight, Citation2004; Braun-Lewensohn & Mosseri Rubin, Citation2014).
Furthermore, our findings reaffirm the important role resources play in positive adaptation in the aftermath of disasters. We found that resources from different levels of ecological systems are both directly and indirectly related to mental health and life satisfaction, thus supporting the multisystemic perspective in the study of resilience (Ungar & Theron, Citation2020). Individual resources, defined as the ability to tolerate and bounce back from problems, as well as interpersonal resources, defined as the support from family, friends, and the significant other, both had direct salutogenic effect on mental health and life satisfaction, as previously found in other studies (Ahmad et al., Citation2010; Bonanno et al., Citation2010; Kaniasty & Norris, Citation2009; Ying et al., Citation2014). Resources from different levels of ecological systems also had an indirect effect on mental health and life satisfaction, through lower psychosocial resource loss. There is numerous evidence that resource loss has a detrimental effect on positive adaptation after disasters (Benight et al., Citation1999; Hobfoll, Canetti-Nisim, & Johnson, Citation2006; Zwiebach et al., Citation2010), but less is known on how to mitigate psychosocial resource loss This study supports the often discussed notion that any single resource at different systemic levels can promote a ‘cascade of changes’ (Ungar & Theron, Citation2020, p. 3) or that people tend to build and maintain ‘resource caravans’, the associations of linked resources, that protect against resource loss (Hobfoll, Citation2014, p. 22). Furthermore, this study shows that community level resources can contribute to reducing individual level resource loss and thus supplement resources on lower levels of ecological systems. This finding is in line with Wind and Komproe (Citation2012) conclusion that disaster-affected individuals in communities with high social capital rely less on individual resources to protect their mental health. It should also be regarded as an important strategy for interventions, as community level interventions can reach a larger number of people at the same time.
Moreover, we found that depending on the exposure level, different resources are harnessed in order to protect against psychosocial resource loss and adapt in the post-disaster environment. This, in addition to the fact that our models were significantly better in explaining the variance of the outcomes in the affected community, provides new evidence that the processes that occur in the context of high risk are qualitatively different to those that occur in the absence of high risk (Luthar, Cicchetti, & Becker, Citation2000). In the affected community individual resources more strongly related to PTS symptoms through psychosocial resource loss. This finding is in line with previous studies that have shown that individual resources have a more important role in reducing symptoms of PTS at higher levels of risk (Besser, Zeigler-Hill, Weinberg, Pincus, & Neria, Citation2014; Green, Calhoun, Dennis, & Beckham, Citation2010). No such effect was found for depression symptoms and life satisfaction, contrary to some previous studies (e.g. Kaniasty, Citation2006). It is possible that, due to the inclusion of interpersonal and community resources, the relative importance of intrapersonal coping strategies in this study was diminished. Furthermore, interpersonal resources were found to be more strongly indirectly related to all indicators of positive adaptation in the affected community, thus supporting the important role of social support in buffering the effects of high risk (Arnberg, Hultman, Michel, & Lundin, Citation2012; McGuire et al., Citation2018). In fact, in the comparison community, interpersonal resources were not significantly related to positive adaptation. This could be attributed to the type of resource loss that was experienced: in the comparison community resource loss was likely related to more individually experienced stressors; therefore, the coping efforts might have not relied as much on interpersonal support. It could also reflect a qualitatively different adaptation process in differentially exposed communities, as social support can be seen as a potential catalyst of the resilience process in the context of high risk (Abramson et al., Citation2015).
Finally, and contrary to our expectations, community resources as a whole were more strongly indirectly related to positive adaptation in the comparison community. However, an additional analysis showed that the pattern of relationships was not the same for all aspects of community resources. Community social capital and engagement was more important in the affected community, whereas trust in leadership and economic development was more important in the comparison community. Taken together with the important role of interpersonal resources, this study points out that the recovery of communities affected by a disaster is deeply embedded in the social environment and that improving and strengthening this dimension in the post-disaster period is essential for individual and community mental health and wellness (Ungar & Theron, Citation2020). Nevertheless, more research is needed to determine the appropriate timing of these supports. A longitudinal study after hurricane Katrina showed that the level of children’s PTS was associated with increased social support from parents and peers only about a year and a half after the disaster (Lai, Osborne, Piscitello, Self-Brown, & Kelley, Citation2018). It is therefore possible that the ‘naturally occurring’ social support systems come into play only in the mid- to long-term period after disaster if not facilitated by interventions. Further research that examines longitudinal patterns of relationships between interpersonal and community resources and psychological outcomes should clarify this point.
That leadership and community economic development were more important resources in a non-flooded community is contradictory to the theoretical predictions (Norris et al., Citation2008). But some studies indicated that higher community economic development was related to better outcomes only among participants who were not exposed to disasters (Lowe, Sampson, Gruebner, & Galea, Citation2015). In the present study it is likely that disaster mitigation efforts and subsequent governmental relief targeting the affected community has superseded the leadership role of municipal authorities and diminished the effects of economic loss. Although often regarded as an important resource (Braun-Lewensohn & Sagy, Citation2014; Krishna, Ronan, & Alisic, Citation2018), disaster preparedness was not related to positive adaptation in either community. It is possible that the suddenness of the event as well as previous war-related experience with displacement shaped a relatively disengaged relationship. This would be in line with some studies that indicate that cumulative exposure to disasters can reduce disaster preparedness (Liddell, Saltzman, Ferreira, & Lesen, Citation2020). Further research is needed to clarify these findings, particularly conducted in communities differentially exposed to risks.
5. Study limitations
This study has several limitations. First, as all measurements have been conducted in a single time point, the true causal relationships could not be determined. Rather, the order of the variables entered into the model was based on logical sequence and predictions based on Hobfoll’s COR theory (Citation1989). Because of the cross-sectional nature of the data, it is also not possible to determine whether or not there are other factors that can affect the findings. Even though we attempted to overcome these limitations by testing alternative models with different order of the variables or with control variables, we acknowledge that factors such as pre-disaster and current mental health, time of the measurement, as well as observed and unobserved differences between the two communities might have impacted the results. However, previous studies have found that resource-to-outcome relationship holds true even after accounting for pre-disaster mental health (e.g. Zwiebach et al., Citation2010). It has also been found that psychosocial resource loss is a stronger predictor of distress over longer time periods than vice versa (Heath, Hall, Russ, Canetti, & Hobfoll, Citation2012), thus supporting our proposed order of variables in the models.
Second, community resources in this study were measured at an individual level and can be more accurately described as individual-level perceptions of community resources or the availability of those resources to the individual. The limitation of this approach is the possibility that individuals cannot accurately estimate community resources, and therefore the results reflect a portion of the variance attributed to participant’s individual characteristics. Nevertheless, our findings are in line with previously conducted multi-level studies that have assessed community resources at an aggregate level (e.g. Ursano et al., Citation2014; Wind & Komproe, Citation2012). Therefore, it is likely that individual perceptions are embedded in the actual resources available in the community.
Although the fit of the analytical model to the data was good and the percentage of explained variance in indicators of positive adaptation was high, the study was less successful in explaining the variance of psychological resource loss. This is an important consideration for future studies, given the importance of resource loss for post-disaster recovery. It is evident that there are a multitude of resources that play a part in fostering resilience; future studies should aim to identify those resources with the strongest potential to protect from psychosocial resource loss and therefore lead to better post-disaster outcomes. Furthermore, even though the sample size was adequate for addressing the research questions and included approximately 6% of all community members from each community, in absolute terms it was modest in size.
Finally, we have not said anything about the generalizability of this study yet. Even though it is not a limitation in the same sense as those previously mentioned, it is possible that some characteristics of the studied communities have shaped the results of this study, particularly as it concerns the relative (un)importance of economic development and trust in leadership in the affected community. This study was conducted in two rural communities that have both been economically disadvantaged and where unemployment was rampant. Additionally, a high percentage of participants have previously experienced a number of potentially traumatic events related to war experiences. The closing of workplaces and further increased unemployment after the disaster, combined with high material losses and a government-led reconstruction programme, might have reduced previous socioeconomic differences in the affected community (that have already been small) and diminished the leadership role of the municipal authorities. Furthermore, this study was conducted in the mid-term period after the flooding, when a higher emphasis on social ties and resources may dominate, which may be different at other recovery phases.
6. Conclusions and implications
Despite its limitations, this study adds to the knowledge of the resilience process after exposure to disasters. We explored how communities adapt to a disaster by harnessing resources and mitigating resource loss. We included resources from different levels of the ecological systems and moved forward from operationalizing positive adaptation only as the absence of psychopathology. The samples were of sufficient size and randomly sampled, therefore strengthening the generalizability of the findings in the similar contexts. We were also able to compare findings between two communities similar in terms of population composition and life experiences but differentially exposed to flooding. The findings indicate that strong individual, interpersonal, and community resources protect against psychosocial resource loss and through that reduce symptoms of PTS and depression and improve life satisfaction. Individual resources, and especially interpersonal resources and community social capital and engagement, were found to be particularly important in the flooded community. These findings support the notion that processes occurring in the presence of high risk are different from those occurring in the presence of low risk (Luthar et al., Citation2000), hence contributing to the development of resilience models in disaster research and planning interventions at different stages of disaster response. Future studies might benefit from examining these relationships in different social contexts and, particularly, longitudinally, at different times post-disaster.
Our findings have implications for disaster preparedness efforts and post-disaster interventions. Building resources in communities is likely to positively influence community members’ health and well-being even in the absence of high community-level exposure to risk. Individual resources, community economic development, and trust in community leadership play an important role in improving community mental health and life satisfaction. However, after exposure to disasters, and following the stepwise model of psychosocial support (Inter-Agency Standing Committee, Citation2007), interventions should aim to strengthen family and community ties. As disasters have a lasting impact on community life, these interventions should continue in the long-term period (Reifels et al., Citation2014). Finding ways to promote social support and community connectedness could be the key to fostering disaster resilience.
Supplemental Material
Download ()Data availability statement
The data that support the findings of this study are available on request from the corresponding author, H. B. The data are not publicly available due to their containing information that could compromise the privacy of research participants.
Disclosure statement
No potential conflict of interest was reported by the authors.
Supplementary material
Supplemental data for this article can be accessed here.
Additional information
Funding
References
- Abramson, D. M., Grattan, L. M., Mayer, B., Colten, C. E., Arosemena, F. A., Bedimo-Rung, A., & Lichtveld, M. (2015). The resilience activation framework: A conceptual model of how access to social resources promotes adaptation and rapid recovery in post-disaster settings. The Journal of Behavioral Health Services & Research, 42(1), 42–15. doi:10.1007/s11414-014-9410-2.
- Ahmad, S., Feder, A., Lee, E. J., Wang, Y., Southwick, S. M., Schlackman, E., … Charney, D. S. (2010). Earthquake impact in a remote South Asian population: Psychosocial factors and posttraumatic symptoms. Journal of Traumatic Stress, 23(3), 408–412. doi:10.1002/jts.20535.
- Arnberg, F. K., Hultman, C. M., Michel, P.-O., & Lundin, T. (2012). Social support moderates posttraumatic stress and general distress after disaster. Journal of Traumatic Stress, 25(6), 721–727. doi:10.1002/jts.21758.
- Bakic, H. (2017, May 11). Community resilience: Measurement and implications [Conference presentation]. ERFCON 2017 Preconference Growing Resilience: Researching Beyond Academia, Zagreb, Croatia.
- Benight, C. C. (2004). Collective efficacy following a series of natural disasters. Anxiety, Stress and Coping, 17(4), 401–420. doi:10.1080/10615800512331328768.
- Benight, C. C., Ironson, G., Klebe, K., Carver, C. S., Wynings, C., Burnett, K., … Schneiderman, N. (1999). Conservation of resources and coping self-efficacy predicting distress following a natural disaster: A causal model analysis where the environment meets the mind. Anxiety, Stress, & Coping, 12(2), 107–126. doi:10.1080/10615809908248325.
- Besser, A., Zeigler-Hill, V., Weinberg, M., Pincus, A. L., & Neria, Y. (2014). Intrapersonal resilience moderates the association between exposure-severity and PTSD symptoms among civilians exposed to the 2014 Israel–Gaza conflict. Self and Identity, 14(1), 1–15. doi:10.1080/15298868.2014.966143.
- Bonanno, G. A., Brewin, C. R., Kaniasty, K., & La Greca, A. M. L. (2010). Weighing the costs of disaster: Consequences, risks, and resilience in individuals, families, and communities. Psychological Science in the Public Interest, 11(1), 1–49. doi:10.1177/1529100610387086.
- Bonanno, G. A., Galea, S., Bucciarelli, A., & Vlahov, D. (2007). What predicts psychological resilience after disaster? The role of demographics, resources, and life stress. Journal of Consulting and Clinical Psychology, 75(5), 671–682. doi:10.1037/0022-006X.75.5.671.
- Bonanno, G. A., Romero, S. A., & Klein, S. I. (2015). The temporal elements of psychological resilience: An integrative framework for the study of individuals, families, and communities. Psychological Inquiry, 26(2), 139–169. doi:10.1080/1047840X.2015.992677.
- Bovin, M. J., Marx, B. P., Weathers, F. W., Gallagher, M. W., Rodriguez, P., Schnurr, P. P., & Keane, T. M. (2016). Psychometric properties of the PTSD checklist for diagnostic and statistical manual of mental disorders-fifth edition (PCL-5) in veterans. Psychological Assessment, 28(11), 1379–1391. doi:10.1037/pas0000254.
- Braun-Lewensohn, O., & Mosseri Rubin, M. (2014). Personal and communal resilience in communities exposed to missile attacks: Does intensity of exposure matter? Journal of Positive Psychology, 9(2), 175–182. doi:10.1080/17439760.2013.873946.
- Braun-Lewensohn, O., & Sagy, S. (2014). Community resilience and sense of coherence as protective factors in explaining stress reactions: Comparing cities and rural communities during missiles attacks. Community Mental Health Journal, 50(2), 229–234. doi:10.1007/s10597-013-9623-5.
- Campbell-Sills, L., & Stein, M. B. (2007). Psychometric analysis and refinement of the Connor–Davidson resilience scale (CD-RISC): Validation of a 10-item measure of resilience. Journal of Traumatic Stress, 20(6), 1019–1028. doi:10.1002/jts.20271.
- Carlson, E. B., Smith, S. R., Palmieri, P. A., Dalenberg, C., Ruzek, J. I., Kimerling, R., … Spain, D. A. (2011). Development and validation of a brief self-report measure of trauma exposure: The trauma history screen. Psychological Assessment, 23(2), 463–477. doi:10.1037/a0022294.
- Chen, L., & Liu, A. (2015). The incidence of posttraumatic stress disorder after floods: A meta-analysis. Disaster Medicine and Public Health Preparedness, 9(3), 329–333. doi:10.1017/dmp.2015.17.
- Diener, E., Emmons, R. A., Larsen, R. J., & Griffin, S. (1985). The satisfaction with life scale. Journal of Personality Assessment, 49(1), 71–75. doi:10.1207/s15327752jpa4901_13.
- Eaton, W. W., Smith, C., Ybarra, M., Muntaner, C., & Tien, A. (2004). Center for epidemiologic studies depression scale: Review and revision (CESD and CESD-R). In M. E. Maruish (Ed.), The use of psychological testing for treatment planning and outcomes assessment (3rd ed., pp. 363–377). Mahwah, NJ: Lawrence Erlbaum.
- Enders, C. K. (2001). The impact of nonnormality on full information maximum-likelihood estimation for structural equation models with missing data. Psychological Methods, 6(4), 352–370. doi:10.1037/1082-989X.6.4.352.
- Faul, F., Erdfelder, E., Buchner, A., & Lang, A.-G. (2009). Statistical power analyses using G*Power 3.1: Tests for correlation and regression analyses. Behavior Research Methods, 41(4), 1149–1160. doi:10.3758/brm.41.4.1149.
- Green, K. T., Calhoun, P. S., Dennis, M. F., & Beckham, J. C. (2010). Exploration of the resilience construct in posttraumatic stress disorder severity and functional correlates in military combat veterans who have served since september 11, 2001. Journal of Clinical Psychiatry, 71(7), 823–830. doi:10.4088/JCP.09m05780blu.
- Harvey, M. R. (2007). Towards an ecological understanding of resilience in trauma survivors. Journal of Aggression, Maltreatment & Trauma, 14(1–2), 9–32. doi:10.1300/J146v14n01_02.
- Heath, N. M., Hall, B. J., Russ, E. U., Canetti, D., & Hobfoll, S. E. (2012). Reciprocal relationships between resource loss and psychological distress following exposure to political violence: An empirical investigation of COR theory’s loss spirals. Anxiety, Stress and Coping, 25(6), 679–695. doi:10.1080/10615806.2011.628988.
- Hikichi, H., Aida, J., Tsuboya, T., Kondo, K., & Kawachi, I. (2016). Can community social cohesion prevent posttraumatic stress disorder in the aftermath of a disaster? A natural experiment from the 2011 Tohoku earthquake and tsunami. American Journal of Epidemiology, 183(10), 902–910. doi:10.1093/aje/kwv335.
- Hobfoll, S. (2014). Resource caravans and resource caravan passageways: A new paradigm for trauma responding. Intervention, 12(1), 21–32. doi:10.1097/wtf.0000000000000067.
- Hobfoll, S. E. (1989). Conservation of resources: A new attempt at conceptualizing stress. American Psychologist, 44(3), 513–524. doi:10.1037/0003-066X.44.3.513.
- Hobfoll, S. E. (2002). Social and psychological resources and adaptation. Review of General Psychology, 6(4), 307–324. doi:10.1037/1089-2680.6.4.307.
- Hobfoll, S. E., Canetti-Nisim, D., & Johnson, R. J. (2006). Exposure to terrorism, stress-related mental health symptoms, and defensive coping among Jews and Arabs in Israel. Journal of Consulting and Clinical Psychology, 74(2), 207–218. doi:10.1037/0022-006X.74.2.207.
- Hobfoll, S. E., Tracy, M., & Galea, S. (2006). The impact of resource loss and traumatic growth on probable PTSD and depression following terrorist attacks. Journal of Traumatic Stress, 19(6), 867–878. doi:10.1002/jts.20166.
- Hobfoll, S. E., Watson, P., Bell, C. C., Bryant, R. A., Brymer, M. J., Friedman, M. J., … Ursano, R. J. (2007). Five essential elements of immediate and mid–term mass trauma intervention: Empirical evidence. Psychiatry: Interpersonal and Biological Processes, 70(4), 283–315. doi:10.1521/psyc.2007.70.4.283.
- Hu, L., & Bentler, P. M. (1999). Cutoff criteria for fit indexes in covariance structure analysis: Conventional criteria versus new alternatives. Structural Equation Modeling: A Multidisciplinary Journal, 6(1), 1–55. doi:10.1080/10705519909540118.
- Inter-Agency Standing Committee. (2007). IASC guideliness on mental health and psychosocial support in emergency settings. Retrieved from https://interagencystandingcommittee.org/iasc-task-force-mental-health-and-psychosocial-support-emergency-settings/iasc-guidelines-mental
- Jermacane, D., Waite, T. D., Beck, C. R., Bone, A., Amlôt, R., Reacher, M., … Oliver, I. (2018). The english national cohort study of flooding and health: The change in the prevalence of psychological morbidity at year two. BMC Public Health, 18(1), 1–8. doi:10.1186/s12889-018-5236-9.
- Kaniasty, K. (2006). Sense of mastery as a moderator of longerterm effects of disaster impact on psychological distress. In J. Strelau & T. Klonowicz (Eds.), People under extreme stress (pp. 131–147). Hauppauge, NY: Nova Science Publishers. doi:10.1017/CBO9780511730030.011.
- Kaniasty, K., & Norris, F. H. (2009). Distinctions that matter: Received social support, perceived social support, and social embeddedness after disasters. In Eds., Y. Neria, S. Galea, & F. H. Norris Mental health and disasters (pp. 175–200). Cambridge: Cambridge University Press. doi:10.1002/jcop.20012
- Krishna, R. N., Ronan, K. R., & Alisic, E. (2018). Children in the 2015 South Indian floods: Community members’ views. European Journal of Psychotraumatology, 9(sup2), Article 1486122. doi:10.1080/20008198.2018.1486122.
- Lai, B. S., Osborne, M. C., Piscitello, J., Self-Brown, S., & Kelley, M. L. (2018). The relationship between social support and posttraumatic stress symptoms among youth exposed to a natural disaster. European Journal of Psychotraumatology, 9(sup2), Article 1450042. doi:10.1080/20008198.2018.1450042.
- Liddell, J. L., Saltzman, L. Y., Ferreira, R. J., & Lesen, A. E. (2020). Cumulative disaster exposure, gender and the protective action decision model. Progress in Disaster Science, 5, Article 100042. doi:10.1016/j.pdisas.2019.100042.
- Little, T. D., Rhemtulla, M., Gibson, K., & Schoemann, A. M. (2013). Why the items versus parcels controversy needn’t be one. Psychological Methods, 18(3), 285–300. doi:10.1037/a0033266.
- Littleton, H. L., Axsom, D., & Grills-Taquechel, A. E. (2009). Adjustment following the mass shooting at Virginia Tech: The roles of resource loss and gain. Psychological Trauma: Theory, Research, Practice, and Policy, 1(3), 206–219. doi:10.1037/a0017468.
- Lowe, S. R., Bonumwezi, J. L., Valdespino-Hayden, Z., & Galea, S. (2019). Posttraumatic stress and depression in the aftermath of environmental disasters: A review of quantitative studies published in 2018. Current Environmental Health Reports, 6(4), 344–360. doi:10.1007/s40572-019-00245-5.
- Lowe, S. R., Sampson, L., Gruebner, O., & Galea, S. (2015). Psychological resilience after Hurricane Sandy: The influence of individual- and community-level factors on mental health after a large-scale natural disaster. PLoS ONE, 10(5), Article e0125761. doi:10.1371/journal.pone.0125761.
- Luthar, S. S., Cicchetti, D., & Becker, B. (2000). The construct of resilience: A critical evaluation and guidelines for future work. Child Development, 71(3), 543–562. doi:10.1111/1467-8624.00164.
- MacKinnon, D. P., Lockwood, C. M., Hoffman, J. M., West, S. G., & Sheets, V. (2002). A comparison of methods to test mediation and other intervening variable effects. Psychological Methods, 7(1), 83–104. doi:10.1037/1082-989X.7.1.83.
- Maercker, A., & Hecker, T. (2016). Broadening perspectives on trauma and recovery: A socio-interpersonal view of PTSD. European Journal of Psychotraumatology, 7(1), Article 29303. doi:10.3402/ejpt.v7.29303.
- Marsh, H. W., Lüdtke, O., Nagengast, B., Morin, A. J. S., & Von Davier, M. (2013). Why item parcels are (almost) never appropriate: Two wrongs do not make a right - Camouflaging misspecification with item parcels in CFA models. Psychological Methods, 18(3), 257–284. doi:10.1037/a0032773.
- Masten, A. S., Best, K. M., & Garmezy, N. (1990). Resilience and development: Contributions from the study of children who overcome adversity. Development and Psychopathology, 2(4), 425–444. doi:10.1017/S0954579400005812.
- Masten, A. S., & Narayan, A. J. (2012). Child development in the context of disaster, war, and terrorism: Pathways of risk and resilience. Annual Review of Psychology, 63(1), 227–257. doi:10.1146/annurev-psych-120710-100356.
- McGuire, A. P., Gauthier, J. M., Anderson, L. M., Hollingsworth, D. W., Tracy, M., Galea, S., & Coffey, S. F. (2018). Social support moderates effects of natural disaster exposure on depression and posttraumatic stress disorder symptoms: Effects for displaced and nondisplaced residents. Journal of Traumatic Stress, 31(2), 223–233. doi:10.1002/jts.22270.
- Norris, F. H., Friedman, M. J., Watson, P. J., Byrne, C. M., Diaz, E., & Kaniasty, K. (2002). 60,000 disaster victims speak: Part I. An empirical review of the empirical literature, 1981-2001. Psychiatry, 65(3), 207–239. doi:10.1521/psyc.65.3.207.20173.
- Norris, F. H., & Kaniasty, K. (1996). Received and perceived social support in times of stress: A test of the social support deterioration deterrence model. Journal of Personality and Social Psychology, 71(3), 498–511. doi:10.1037/0022-3514.71.3.498.
- Norris, F. H., Stevens, S. P., Pfefferbaum, B., Wyche, K. F., & Pfefferbaum, R. L. (2008). Community resilience as a metaphor, theory, set of capacities, and strategy for disaster readiness. American Journal of Community Psychology, 41(1–2), 127–150. doi:10.1007/s10464-007-9156-6.
- Raker, E. J., Lowe, S. R., Arcaya, M. C., Johnson, S. T., Rhodes, J., & Waters, M. C. (2019). Twelve years later: The long-term mental health consequences of Hurricane Katrina. Social Science and Medicine, 242, 112610. doi:10.1016/j.socscimed.2019.112610.
- Reifels, L., Bassilios, B., Spittal, M., King, K., Fletcher, J., & Pirkis, J. (2014). Patterns and predictors of primary mental health service use following bushfire and flood disasters. European Journal of Psychotraumatology, 5(1), Article 26527. doi:10.3402/ejpt.v5.26527.
- Rosseel, Y. (2012). lavaan: An R package for structural equation modeling. Journal of Statistical Software, 48(2). doi:10.18637/jss.v048.i02
- Šoštarić, T. (2014, October 14). Islam u Hrvatskoj - model za cijelu Europu. Aljazeera Balkans. Retrieved from http://balkans.aljazeera.net/vijesti/islam-u-hrvatskoj-model-za-cijelu-evropu
- Southwick, S. M., Bonanno, G. A., Masten, A. S., Panter-Brick, C., & Yehuda, R. (2014). Resilience definitions, theory, and challenges: Interdisciplinary perspectives. European Journal of Psychotraumatology, 5(1), Article 25338. doi:10.3402/ejpt.v5.25338.
- Ungar, M., & Theron, L. (2020). Resilience and mental health: How multisystemic processes contribute to positive outcomes. Lancet Psychiatry, 7(5), 441–448. doi:10.1016/S2215-0366(19)30434-1.
- Ursano, R. J., McKibben, J. B. A., Reissman, D. B., Liu, X., Wang, L., Sampson, R. J., & Fullerton, C. S. (2014). Posttraumatic stress disorder and community collective efficacy following the 2004 Florida Hurricanes. PLoS ONE, 9(2), Article e88467. doi:10.1371/journal.pone.0088467.
- Vujević Hećimović, G., Brajović, S., & Ilin, K. (2010). Socijalna distanca građana Hrvatske prema narodima s područja bivše Jugoslavije s obzirom na vrijeme i neka socio-demografska obilježja. Suvremena Psihologija, 13(1), 137–154. https://hrcak.srce.hr/83031
- Weathers, F. W., Litz, B. T., Keane, T. M., Palmieri, P. A., Marx, B. P., & Schnurr, P. P. (2013). The PTSD checklist for DSM-5 (PCL-5). Scale available from the National Center for PTSD. Retrieved from www.ptsd.va.gov.
- West, J. S., Price, M., Gros, K. S., & Ruggiero, K. J. (2013). Community support as a moderator of postdisaster mental health symptoms in urban and nonurban communities. Disaster Medicine and Public Health Preparedness, 7(5), 443–451. doi:10.1017/dmp.2013.74.
- Wind, T. R., & Komproe, I. H. (2012). The mechanisms that associate community social capital with post-disaster mental health: A multilevel model. Social Science & Medicine, 75(9), 1715–1720. doi:10.1016/j.socscimed.2012.06.032.
- Ying, L., Wu, X., Lin, C., & Jiang, L. (2014). Traumatic severity and trait resilience as predictors of posttraumatic stress disorder and depressive symptoms among adolescent survivors of the Wenchuan earthquake. PLoS ONE, 9(2), Article e89401. doi:10.1371/journal.pone.0089401.
- Zhong, X., & Yuan, K.-H. (2011). Bias and efficiency in structural equation modeling: Maximum likelihood versus robust methods. Multivariate Behavioral Research, 46(2), 229–265. doi:10.1080/00273171.2011.558736.
- Zimet, G. D., Dahlem, N. W., Zimet, S. G., & Farley, G. K. (1988). The multidimensional scale of perceived social support. Journal of Personality Assessment, 52(1), 30–41. doi:10.1207/s15327752jpa5201_2.
- Zwiebach, L., Rhodes, J., & Roemer, L. (2010). Resource loss, resource gain, and mental health among survivors of Hurricane Katrina. Journal of Traumatic Stress, 23(6), 751–758. doi:10.1002/jts.20579.