ABSTRACT
Background: Cardiopulmonary resuscitation (CPR) is occurring more frequently at community hospitals but most patients undergoing CPR do not survive to discharge. Tools to predict CPR survival can be improved by the identification of high-yield clinical indicators.Objective: To identify variables associated with survival to discharge following in-hospital cardiac arrest.Methods: Retrospective cohort study of 463,530 hospital admissions from the Nationwide Inpatient Sample (2012–2016). The analysis includes adults (age ≥50) who underwent in-hospital CPR at US community hospitals.Results: Overall survival to discharge was 29.8% (95% CI: 29.5–30.1%). Age was the strongest predictor of survival and had greater prognostic value than the Charlson comorbidity index. Obesity was associated with improved survival (35.9%, 95% CI: 35.1–36.7%), whereas underweight patients had decreased survival (24.0%, 95% CI: 22.2–25.7%). Acute indicators of poor survival included hyperkalemia, hypercalcemia, and sepsis. We generated an ABCD index based upon four high-yield variables (age, body habitus, comorbidity, day of hospital admission). An ABCD score of 2 or less was a sensitive but non-specific predictor of post-CPR survival (96.8% sensitivity, 95% CI: 96.6–97.0), and those with extreme scores differed 3.8-fold with respect to post-CPR survival probability (46.0% versus 12.1%).Conclusion: Age is the strongest predictor of post-CPR survival, but body habitus is also an important indicator that may currently be underutilized. Our results support improved post-CPR survival of obese patients, consistent with an ‘obesity paradox’. The ABCD score provides an efficient means of risk-stratifying patients and can be calculated in less than 1 minute.
1. Introduction
The decision to provide advance consent for CPR in the event of in-hospital cardiac arrest (IHCA) can be challenging for patients. Some patients stand to benefit from CPR if they have few comorbidities and if the underlying cause of IHCA can be identified and resolved. On the other hand, CPR may be futile and only extend suffering when underlying conditions cannot be resolved [Citation1]. CPR may therefore not be appropriate for all patients and providers must support patients in code status discussions at the time of admission and throughout their hospital stay. As an approximate guideline, only one in eight patients who undergo in-hospital CPR will survive to discharge [Citation2]. Ideally, however, code status discussion will be informed by patient-specific factors, and some decision-support algorithms have been developed for this purpose. These include the Pre-arrest Morbidity (PAM) index [Citation3], the Prognosis After Resuscitation (PAR) score [Citation4], and the Good Outcome Following Attempted Resuscitation scoring system (GO-FAR) [Citation5]. Despite the availability of these indices, CPR incidence has risen in recent years with greater hospitalization costs and recipients having an increasingly high comorbidity burden [Citation6].
The clinical utility of a decision-support tool depends upon accuracy for prediction or risk-stratification as well as ease of clinical use. Accuracy crucially depends upon data used to formulate the tool, with larger datasets yielding more robust tools more likely to generalize to other patient populations. The PAM index was innovative at the time of its development, although it is complex (15 predictor variables) and developed from analysis of only 140 patients [Citation3]. The PAR score is less complex (8 predictor variables) and derived from meta-analysis of 14 studies involving an unknown number of patients [Citation4]. Neither index (PAM nor PAR) was effective at predicting post-CPR survival in a validation study [Citation7]. The GO-FAR index is based upon 13 variables and was developed from analysis of 51,240 patients [Citation5]. GO-FAR differs from other indices in that it was developed to predict neurologically intact survival, rather than survival to discharge. Additionally, GO-FAR has been validated by one prospective study [Citation8].
The Nationwide Inpatient Sample (NIS) is the largest available nationally representative all-payer database of inpatient hospital admissions in the USA. Analyses of NIS data have identified associations between increased post-CPR mortality and chronic kidney disease [Citation9], acute kidney injury [Citation10], end-stage liver disease [Citation11], hospitals located in the Northeast USA [Citation12], and multiple CPR attempts [Citation13]. Unexpected or paradoxical associations have also been identified. For example, an NIS study of women 13–49 years of age (2002–2011) showed that post-CPR mortality was lower among pregnant compared to nonpregnant women [Citation14]. Another NIS study (2003–2011) found that smokers who underwent CPR had a higher rate of survival to discharge than non-smokers (28.2% vs. 24.1%) [Citation15]. Associations between survival and obesity have been conflicting. One NIS analysis (2001–2008) showed that survival to discharge was lower in morbidly obese patients, particularly if IHCA occurred after 7 days of hospitalization [Citation16]. However, another NIS study (2003–2011) showed that obese patients had improved survival to hospital discharge (31.4%) compared to non-obese patients (24.1%) [Citation17].
This study analyzed recent NIS sample cohorts (2012–2016) to identify factors associated with survival to hospital discharge. Our goal was to understand the relative importance of factors associated with survival, with the aim of identifying high-yield indicators that warrant consideration as predictors in decision-support tools (e.g., PAM, PAR and GO-FAR) [Citation3–5]. We attempt to clarify the association between obesity and survival, which has been inconsistent in previous NIS studies [Citation16,Citation17]. Finally, we formulate an efficient ABCD score based upon only four high-yield predictors, which can be used to risk-stratify patients with respect to post-CPR survival probability.
2. Methods
The analysis was performed using a sample of 35,777,371 hospital admissions over a 5-year period (2012: 7,296,968; 2013: 7,119,562; 2014: 7,071,762, 2015: 7,153,989; 2016: 7,135,090). Each admission was representative of approximately five nationwide admissions. Among the 35,777,371 admissions, we identified 113,499 CPR events (2012: 21,702; 2013: 22,667; 2014: 23,684; 2015: 23,471; 2016: 21,975). Of these, we included patients age 50 or greater, and we excluded 3 admissions for which no diagnosis codes were available. This yielded 92,706 CPR admissions, which were representative of 463,530 (± 8.66) such admissions nationwide. This paper reports nationwide estimates unless otherwise stated. For 2012, 2013, 2014, and the first three quarters of 2015, CPR events were identified using ICD-9 procedure codes 9960 (cardiopulmonary resuscitation) and 9963 (closed-chest cardiac massage). For 2016 and the last quarter of 2015, CPR events were identified based upon the ICD-10 procedure code 5A12012 (performance of cardiac output, single, manual). Obese patient admissions were identified using ICD-9/10 diagnosis codes containing the word ‘obesity’ and/or codes specifying a body mass index of 30 or greater. Underweight patient admissions were identified using ICD-9/10 diagnosis codes corresponding to ‘underweight’ and/or ‘body mass index less than 19’. The day on which CPR was performed was calculated using the recorded procedure and admission dates, such that day 0 is defined as the day of admission, and day 1 indicates that at least one midnight has passed since admission.
The R package ‘comorbidity’ was used to calculate the Charlson comorbidity index (function: comorbidity) [Citation18,Citation19]. A hierarchy of comorbidities was applied (option: assign0 = TRUE), such that a given comorbidity with differing levels of severity was not counted multiple times. The weighted Charlson score was used for all analyses [Citation18]. The R package ‘survey’ was used to calculate percent survival and confidence internals (functions: svymean and confint). This allowed percent survival to be estimated with adjustment for NIS stratum (NIS_STRATUM) and admission weights (DISCWT). Depending upon the subgroup analyzed, a small number of NIS strata were associated with only one CPR admission. In such cases, the stratum contribution to the total variance was set equal to the average of all other strata (i.e., survey package option: survey.lonely.psu = ‘adjust’). Associations between variables and survival to hospital discharge were evaluated using generalized linear models with inverse-probability weighting and design-based standard errors (R package: survey; function: svyglm).
We develop an index to predict survival to discharge and calculated measures of test accuracy. Balanced accuracy estimates and associated confidence intervals were calculated using the R ‘caret’ package (function: confusionMatrix). Sensitivity, specificity, positive predictive value, and negative predictive value and associated confidence intervals were calculated using the R ‘bdpv’ package (function: BDtest). Exact confidence intervals were calculated for sensitivity and specificity, whereas asymptotic standard logit intervals were calculated for positive/negative predictive values [Citation20]. The ROC curve AUC statistic and confidence interval were calculated using the R ‘pROC’ package (function: roc) [Citation21].
3. Results
3.1. Survival to discharge following in-hospital CPR is influenced by year, race, socioeconomic status, and admission day
The overall probability of survival to discharge was 29.8% (95% CI: 29.5%-30.1%) among 463,530 admissions during which CPR was performed ()). There was slight improvement in survival between 2012 (28.6%) and 2016 (30.1%) ()). However, percent survival confidence intervals overlapped from year-to-year, except 2012 survival was significantly lower (28.6%) compared to 2014, 2015 and 2016. Survival was higher among whites (30.8%) compared to blacks (27.6%) or other ethnic groups (28.2%), and survival was higher among patients from wealthier zip codes ()). The hospital day on which CPR occurred had a strong effect on survival probability. Patients with CPR events on the day of admission (day 0) had significantly greater survival (35.9%) than those with CPR on day 1 or after (26.9%). Male and female survival probabilities did not differ significantly ()).
Figure 1. Variables associated with survival to discharge in older adults following in-hospital CPR. (A) Percent survival by subgroup. Plots show point estimates and 95% confidence intervals (left margin: group, sample size; right margin: point estimates with 95% confidence intervals). (B) Percent survival versus age. Point estimates are shown with 95% confidence intervals (inset: line plot with least-squares slope). (C) Percent survival in subgroups by age and Charlson Index. (D) Cross-validation prediction accuracy (Charlson Index vs. Age). In each simulation trial, 20,000 randomly sampled admissions were used for model training (50% survivors, 50% non-survivors), and 20,000 randomly sampled admissions were used for model testing (50% survivors, 50% non-survivors). Univariate logistic regression models were estimated in each trial (Charlson Index or age as a predictor). The testing accuracy distribution among all simulations is shown (null expectation: 50% accuracy)
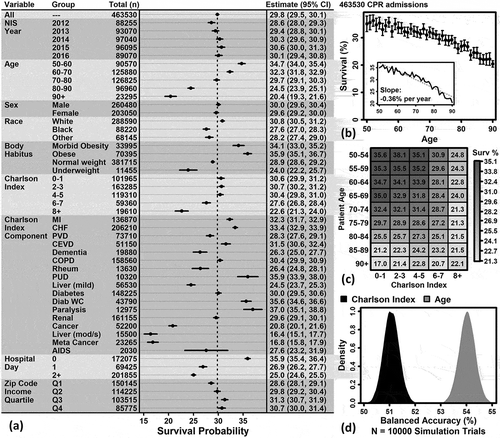
3.2. Age is the strongest predictor of survival to discharge following in-hospital CPR
Percent survival was much greater among those aged 50–60 (34.7%) compared to those 90 or greater (20.4%). Percent survival declined approximately 0.36% per year between the ages of 50 and 90 ()). Charlson index was predictive of survival, although survival was similar for patients with low scores (0–5) and declined only among patients with a score of 6 or greater ()). Only some Charlson Index components were predictive of poor outcomes. Survival was substantially lower among patients with cancer (20.8%), patients with metastatic cancer (16.8%), and patients with moderate-to-severe liver disease (16.4%). Other Charlson Index components were associated with only modestly decreased or even improved survival ()). Predictive ability of the Charlson Index declined with age. Among patients age 90 or older, those with an index score 8 or higher had higher survival (22.1%) than those with an index score of 0 or 1 (17.0%). A similar paradoxical trend was seen in the 85–89 age group ()). In cross-validation simulation trials, age was a stronger predictor of survival to discharge than the Charlson Index (balanced accuracy of 54.0% versus 51.1%, )).
3.3. Obesity is associated with improved survival to discharge following in-hospital CPR
Survival to discharge was higher among obese patients compared to non-obese patients (35.9%, 95% CI: 35.1%-36.7%). Survival was slightly reduced in patients with morbid obesity but still higher compared to non-obese patients (34.1%, 95% CI: 33.0%-35.2%). On the other hand, underweight patients had significantly reduced survival compared to all other patients (24.0%, 95% CI: 22.2%-25.7%). Obese patient survival was significantly higher among all age groups except in patients 90 or greater (P < 0.05, general linear model; )). Likewise, obese patient survival was significantly higher in all examined subgroups that differed according to Charlson Index ()) and each of five chronic conditions (MI, CHF, COPD, diabetes, and renal disease; )).
Figure 2. Influence of obesity and morbid obesity on the probability of survival to discharge following in-hospital CPR. (A – C) Percent survival in non-obese and obese patients of varying age, Charlson Index, and chronic condition (D, E) Percent discharge to home among CPR survivors in non-obese and obese patients of varying age and Charlson Index. (F, G) Percent survival in obese or morbidly obese patients with CPR performed at varying times post-admission (H, I) Percent survival in obese and morbidly obese patients with or without diagnosis codes for ventricular tachycardia (V-tach) or ventricular fibrillation (V-fib). In all figures (A – I), an asterisk (*) denotes a significant y-axis percentage difference in the comparison between obese and non-obese patients or between morbidly obese and non-morbidly obese patients (*P < 0.05, general linear model). All such comparisons were made within the subgroups indicated on the horizontal axis
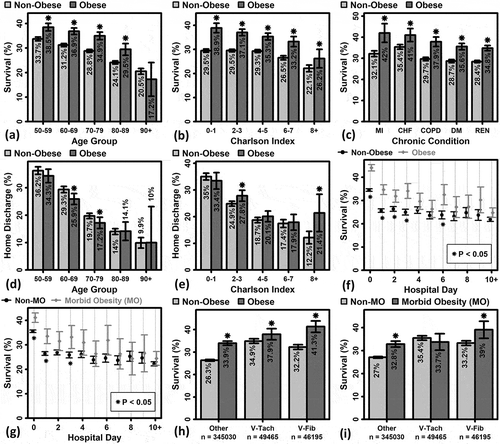
A significantly lower percentage of obese patients aged 60–79 who survived CPR were discharged to home compared to non-obese patients ()). However, among CPR survivors with high comorbidity (Charlson Index ≥8), obese patients were more likely to be discharged home compared to non-obese patients ()). There was no evidence that obese or morbidly obese patients had lower CPR survival when CPR was performed late during a hospital admission ()). Likewise, there was no significant evidence that obese or morbidly obese patients with shockable rhythms were less likely to survive to discharge following CPR ()).
3.4. Acute conditions associated with poor survival include hyperkalemia, hypercalcemia and sepsis
CPR survivors were compared to non-survivors to identify diagnosis codes with differing frequency in each group. ICD-9 and ICD-10 codes for hypokalemia, acute posthemorrhagic anemia, ventricular tachycardia, complete AV block, and ventricular fibrillation were more frequent among survivors ()). On the other hand, do not resuscitate status, palliative care encounter, severe sepsis, acidosis, defibrination syndrome, coma, kidney failure, dehydration, anoxic brain damage, and hyperkalemia were more frequent among non-survivors ()). Survival probabilities for patients with hyperkalemia and hypercalcemia were poor (24.1% and 24.4%, respectively; )). This did not appear due to underlying renal disease, since patients with hyperkalemia or hypercalcemia but without chronic kidney disease still had decreased survival ()). Shockable rhythms were associated with high survival rates (e.g., ventricular tachycardia/flutter; )), and survival rates were excellent for patients with magnesium disorder (44.2%) and long QT (63.4%). Survival was poor, however, in those with sepsis (22.4%) and acidosis (24.6%) ()).
Figure 3. Diagnostic codes enriched among survivors and non-survivors. (A, C) ICD9 and ICD10 diagnostic codes overrepresented among patients that survived to discharge following in-hospital CPR. (B, D) ICD9 and ICD10 diagnostic codes overrepresented among patients that did not survive to discharge following in-hospital CPR. (E) Percent survival by subgroup. Plots show point estimates and 95% confidence intervals (left margin: group, sample size; right margin: point estimates with 95% confidence intervals). (F) Percent survival by potassium level and CKD status (*P < 0.05, non-CKD vs. CKD, general linear model). (G) Percent survival by calcium level and CKD status (*P < 0.05, non-CKD vs. CKD, general linear model)
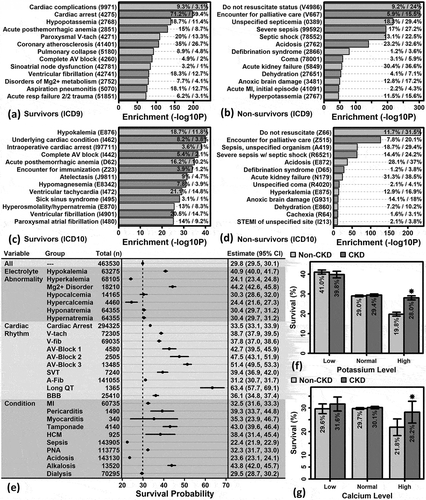
3.5. A simple ABCD score to risk-stratify patients with respect to the probability of post-CPR survival
We formulated a simple ABCD index score to predict CPR survival, with values ranging from −2 (higher predicted survival) to 4 (lower predicted survival) ()). The four variables incorporated into the score were each significantly associated with survival to discharge when combined as covariates within the same general linear model (). A majority of patients (57%) had an ABCD score of 1 or greater ()), and those with a score of −2 had a survival rate 3.8 times higher than those with a score of 4 (46.0% versus 12.1%) ()). An ABCD score ≤2 was 96.8% sensitive for predicting survival to discharge ()). Alternatively, an ABCD score ≤ −1 was 82.0% specific for predicting survival ()). Accuracy and positive and negative predictive values likewise varied based upon the ABCD score threshold chosen ()). The receiver operating characteristic (ROC) curve AUC statistic was modest (0.581) but significantly greater than the null expectation of 0.50 (95% CI: 0.577, 0.585; )).
Table 1. Survey-weighted general linear model with ABCD variables. Survival was used as a quasi-binomial response variable (1 if a patient survived to discharge; 0 otherwise). The model was estimated using 463,530 hospital admissions (3 admissions excluded due to a lack of within-strata replication). Age was incorporated as an ordinal variable, whereas obesity, underweight, comorbidity and CPR day were incorporated as categorical predictors (coded as 1 or 0; see footnotes)
Figure 4. A simple ABCD score to risk-stratify patients with respect to the probability of survival following in-hospital CPR. (A) ABCD score definition. (B) Histogram showing number of CPR admissions relative to ABCD score. (C) Percent survival to discharge versus ABCD score. 95% confidence intervals are shown. (D – H) Accuracy, sensitivity, specificity, positive predictive value (PPV), and negative predictive value (NPV) at different ABCD score thresholds. 95% confidence intervals are shown (not visible for all estimates). (I) ROC curve. The area under the curve (AUC) statistic and confidence interval is shown (top margin)
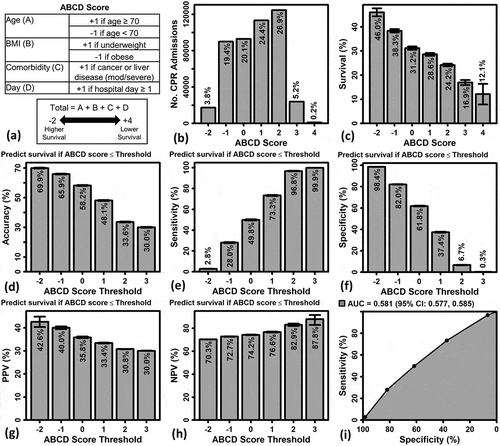
4. Discussion
CPR is frequently performed on patients unlikely to benefit but who might have been recognized as poor candidates based upon objective indicators [Citation1]. Tools have been developed to predict post-CPR survival [Citation3–5], but these may be refined using ‘big data’ approaches to highlight variables with the strongest prognostic value [Citation22,Citation23]. This study utilized the Nationwide Inpatient Sample (NIS) (2012–2016) to identify factors associated with the survival to discharge in 463,530 hospital admissions during which CPR was performed (adults 50 or older). Our results identify high-yield clinical indicators to inform goals-of-care discussions (e.g., Age, Body habitus, Comorbidity (cancer, liver disease), Day of hospital admission). We have incorporated this information into a compact ABCD index that is easily remembered and serves to focus attention on indicators most predictive of CPR outcome.
Age and comorbidity burden are frequently associated but should be considered separately when working with older patients [Citation22]. Age is not a component of the PAM index [Citation3] and is included but weakly weighted within the PAR index [Citation4]. Our analysis, however, showed that age was the strongest overall predictor of survival to discharge following in-hospital CPR (). Age was also a stronger predictor than the Charlson comorbidity index score ()). Survival rates varied continuously in all age groups examined, whereas those with Charlson index scores ≤5 did not differ with respect to survival rate ()). Moreover, higher Charlson index scores were not associated with decreased survival in patients 85 and older ()). The Charlson index score may have been less robustly associated with survival because several score components were not associated with poor outcomes (e.g., peptic ulcer disease, diabetes with complications, and paralysis; )). Indeed, only some forms of comorbidity were predictive of poor survival, most notably cancer and moderate-to-severe liver disease ()). We did not observe decreased CPR survival among CKD or dialysis patients ()). A composite score of comorbidity may therefore fail to risk-stratify patients effectively since not all comorbidities negatively impact CPR survival.
In our study, obese patients had an approximately 6% higher rate of post-CPR survival compared to non-obese patients, an effect size tantamount to 15 years of aging ()). Underweight patients were also less likely to survive, which had not previously been discerned from analysis of NIS data [Citation17]. Body habitus is not a predictor in the PAM, PAR or GO-FAR indices [Citation3–5], suggesting that this information may currently be underutilized. Our results conflict with one prior NIS study, which excluded obese patients but focused more narrowly on morbidly obese patients (representing 1.1% of CPR cases) [Citation16]. However, our results agree well with an analysis of earlier NIS cohorts (2003–2011) that considered 836,289 admissions with CPR performed on adults 18 years and older, of which 67,216 (8.0%) instances involved an obese patient [Citation17]. Other NIS analyses have also shown that improved post-CPR survival is associated with conditions linked to increased body mass index, such as diabetes [Citation24] and pregnancy [Citation14]. Theoretically, large body habitus could reduce the ability of CPR to effectively promote circulation, while further increasing electrical impedance to hinder treatment of shockable rhythms. Neither of these concerns, however, appear to be borne out by empirical evidence [Citation25]. Our results therefore support an ‘obesity paradox’ regarding post-CPR survival, wherein obese patients are more likely to survive to hospital discharge [Citation17].
Those involved in the care of critically ill patients should be aware of post-CPR survival indicators to effectively lead goals-of-care discussions. The ABCD score emphasizes only those indicators emerging as the most influential in our analysis ()). It is easily remembered, can be calculated in less than 1 minute, and may therefore at least be heuristically useful for risk-stratification ()). The ABCD score includes stable predictors, in contrast to the GO-FAR score, which includes some dynamic variables that may not be stable during hospital admission (e.g., hypotension/hypoperfusion, respiratory insufficiency). The ABCD score is either sensitive or specific, depending upon the threshold chosen, although is not both sensitive and specific (). To avoid the error of not resuscitating a patient who might survive to discharge following CPR, our results suggest that patients with a score of 2 or less would be good CPR candidates (96.8% sensitivity). This threshold would have identified 5.4% of CPR interventions as likely futile during the 2012–2016 study period (about 25,000 CPR interventions nationally). Alternatively, the ABCD score can be mapped to a specific post-CPR survival probability (12.1–46.0%), which may be informative for patients who may have different expectations regarding the odds of post-CPR survival [Citation26].
A strength of our study was the analysis of a large number of hospital admissions (463,530), which is a larger sample size than has been used to formulate some predictive indices such as PAM and GO-FAR [Citation3–5]. However, our analysis also has several limitations. First, the overall percentage of patients surviving to discharge following CPR was 29.8% in our analysis, which is consistent with analyses of earlier NIS cohorts [Citation9–17], although studies utilizing other data sources have frequently calculated survival rates below 20% [Citation27–29]. The absolute post-CPR survival rates calculated in our analysis may therefore be optimistic, although this would not necessarily bias associations we identified between variables and post-CPR survival. Second, our results are based upon ICD-9/10 diagnostic and procedure codes, which may contain errors or coding biases. Third, ICD-9/10 codes included in NIS do not provide measures of functional status, which may be an important predictor of outcomes in older patients [Citation22]. Fourth, we have identified variables associated with post-CPR survival, but we cannot fully exclude the possibility of confounding variables driving such associations.
The decision to proceed with aggressive care following IHCA can be life-saving but may also cause unnecessary pain and increase costs associated with end-of-life treatment [Citation30]. In a recent systemic review, a documented discussion with a healthcare provider was the only intervention shown to reduce aggressive end-of-life care in cancer patients, emphasizing the important role of such interactions [Citation31]. This study identified high-yield factors associated with post-CPR survival based upon a large nationally representative database. Our findings can help improve awareness of key clinical indicators to facilitate productive and evidence-based goals-of-care conversations.
Acknowledgments
This work benefited from numerous interactions between the authors and their Internal Medicine colleagues at The Jewish Hospital (Cincinnati, OH) and Fairfield Medical Center (Lancaster, OH). This was an unfunded study.
Disclosure statement
The authors have no conflicts of interest to declare.
References
- Levinson M, Mills A, Barrett J, et al. Why didn’t you write a not-for-cardiopulmonary resuscitation order?’ Unexpected death or failure of process? Australian Health Rev. 2018;42(53–8).
- Ebell MH, Becker LA, Barry HC, et al. Survival after in-hospital cardiopulmonary resuscitation. A meta-analysis. J General Internal Med. 1998;13:805–816.
- Cohn EB, Lefevre F, Yarnold PR, et al. Predicting survival from in-hospital CPR: meta-analysis and validation of a prediction model. J Gen Intern Med. 1993;8:347–353.
- Ebell MH. Prearrest predictors of survival following in-hospital cardiopulmonary resuscitation: a meta-analysis. J Fam Pract. 1992;34:551–558.
- Ebell MH, Jang W, Shen Y, et al. Development and validation of the good outcome following attempted resuscitation (GO-FAR) score to predict neurologically intact survival after in-hospital cardiopulmonary resuscitation. JAMA Intern Med. 2013;173:1872–1878.
- Kazaure HS, Roman SA, Sosa JA. Epidemiology and outcomes of in-hospital cardiopulmonary resuscitation in the USA, 2000–2009. Resuscitation. 2013;84:1255–1260.
- Ohlsson MA, Kennedy LM, Juhlin T, et al. Evaluation of pre-arrest morbidity score and prognosis after resuscitation score and other clinical variables associated with in-hospital cardiac arrest in southern Sweden. Resuscitation. 2014;85:1370–1374.
- Thai TN, Ebell MH. Prospective validation of the good outcome following attempted resuscitation (GO-FAR) score for in-hospital cardiac arrest prognosis. Resuscitation. 2019;140:2–8.
- Saeed F, Adil MM, Kaleem UM, et al. Outcomes of In-hospital cardiopulmonary resuscitation in patients with CKD. Clin J Am Soc Nephrol. 2016;11:1744–1751.
- Saeed F, Adil MM, Kaleem UM, et al. National outcomes of in-hospital cardiopulmonary resuscitation in patients with acute kidney injury: years 2005-2011. Clinical Nephrology. 2018;90:325–333.
- Ufere NN, Brahmania M, Sey M, et al. Outcomes of in-hospital cardiopulmonary resuscitation for patients with end-stage liver disease. Liver Int. 2019;39:1256–1262.
- Kolte D, Khera S, Aronow WS, et al. Regional variation in the incidence and outcomes of in-hospital cardiac arrest in the USA. Circulation. 2015;131:1415–1425.
- Kazaure HS, Roman SA, Sosa JA. A population-level analysis of 5620 recipients of multiple in-hospital cardiopulmonary resuscitation attempts. J Hosp Med. 2014;9:29–34.
- Mogos MF, Salemi JL, Spooner KK, et al. Differences in mortality between pregnant and nonpregnant women after cardiopulmonary resuscitation. Obstet Gynecol. 2016;128:880–888.
- Gupta T, Kolte D, Khera S, et al. Relation of smoking status to outcomes after cardiopulmonary resuscitation for in-hospital cardiac arrest. Am J Cardiol. 2014;114:169–174.
- Shahreyar M, Dang G, Waqas Bashir M, et al. Outcomes of in-hospital cardiopulmonary resuscitation in morbidly obese patients. JACC Clin Electrophysiol. 2017;3:174–183.
- Gupta T, Kolte D, Mohananey D, et al. Relation of obesity to survival after in-hospital cardiac arrest. Am J Cardiol. 2016;118:662–667.
- Charlson ME, Pompei P, Ales KL, et al. A new method of classifying prognostic comorbidity in longitudinal studies: development and validation. J Chron Dis. 1987;40:373–383.
- Quan H, Sundararajan V, Halfon P, et al. Coding algorithms for defining comorbidities in ICD-9-CM and ICD-10 administrative data. Med Care. 2005;43:1130–1139.
- Mercaldo ND, Lau KF, Zhou XH. Confidence intervals for predictive values with an emphasis to case-control studies. Stat Med. 2007;26:2170–2183.
- Robin X, Turck N, Hainard A, et al. pROC: an open-source package for R and S+ to analyze and compare ROC curves. BMC Bioinformatics. 2011;12:77.
- Swindell WR, Ensrud KE, Cawthon PM, et al. Indicators of “healthy aging” in older women (65-69 years of age). A data-mining approach based on prediction of long-term survival. BMC Geriatr. 2010;10:55.
- Swindell WR, Cummings SR, Sanders JL, et al. Data mining identifies digit symbol substitution test score and serum cystatin C as dominant predictors of mortality in older men and women. Rejuvenation Res. 2012;15:405–413.
- Echouffo-Tcheugui JB, Kolte D, Khera S, et al. Comparison of survival after in-hospital cardiac arrest in patients with versus without diabetes mellitus. Am J Cardiol. 2018;121:671–677.
- White RD, Blackwell TH, Russell JK, et al. Body weight does not affect defibrillation, resuscitation, or survival in patients with out-of-hospital cardiac arrest treated with a nonescalating biphasic waveform defibrillator. Crit Care Med. 2004;32:S387–92.
- Portanova J, Irvine K, Yi JY, et al. It isn’t like this on TV: revisiting CPR survival rates depicted on popular TV shows. Resuscitation. 2015;96:148–150.
- van Gijn MS, Frijns D, van de Glind EM, et al. The chance of survival and the functional outcome after in-hospital cardiopulmonary resuscitation in older people: a systematic review. Age Ageing. 2014;43:456–463.
- Ehlenbach WJ, Barnato AE, Curtis JR, et al. Epidemiologic study of in-hospital cardiopulmonary resuscitation in the elderly. N Engl J Med. 2009;361:22–31.
- Hayashi T, Matsushima M, Bito S, et al. Predictors associated with survival among elderly in-patients who receive cardiopulmonary resuscitation in Japan: an observational cohort study. J Gen Intern Med. 2019;34:206–210.
- Duncan I, Ahmed T, Dove H, et al. Medicare cost at end of life. Am J Hosp Palliat Care. 2019;36:705–710.
- Abedini NC, Hechtman RK, Singh AD, et al. Interventions to reduce aggressive care at end of life among patients with cancer: a systematic review. Lancet Oncol. 2019;20:e627–e36.