ABSTRACT
Background: Non-medical switching (NMS) is defined as switching to a clinically similar but chemically distinct medication for reasons apart from lack of effectiveness, tolerability or adherence.
Objective: To update a prior systematic review evaluating the impact of NMS on outcomes.
Data sources: An updated search through 10/1/2018 in Medline and Web of Science was performed.
Study selection: We included studies evaluating ≥25 patients and measuring the impact of NMS of drugs on ≥1 endpoint.
Data extraction: The direction of association between NMS and endpoints was classified as negative, positive or neutral.
Data synthesis: Thirty-eight studies contributed 154 endpoints. The direction of association was negative (n = 48; 31.2%) or neutral (n = 91; 59.1%) more often than it was positive (n = 15; 9.7%). Stratified by endpoint type, NMS was associated with a negative impact on clinical, economic, health-care utilization and medication-taking behavior in 26.9%,41.7%,30.3% and 75.0% of cases; with a positive effect seen in 3.0% (resource utilization) to 14.0% (clinical) of endpoints. Of the 92 endpoints from studies performed by the entity dictating the NMS, 88.0%were neutral or positive; whereas, only 40.3%of endpoints from studies conducted separately from the interested entity were neutral or positive.
Conclusions: NMS was commonly associated with negative or neutral endpoints and was seldom associated with positive ones.
Introduction
Non-medical switching is typically defined as a change in a patient’s medication to a clinically similar but chemically distinct (i.e., not a bioequivalent generic) medication for reasons apart from lack of clinical effectiveness, tolerability or adherence [Citation1,Citation2]. An underlying cause of non-medical switching involves formulary changes aimed at decreasing the acquisition cost of prescription medications. While such practices will likely result in decreased costs of procuring the target medication, non-medical switching has been associated with unintended consequences [Citation3]. A prior systematic review of 29 studies (published prior to November 2015) evaluating ambulatory patients suggested non-medical switching was frequently associated with a negative impact on clinical and economic endpoints, overall resource utilization (not just target medication acquisition costs) and patient adherence or persistence to their prescribed medication regimens [Citation3].
Since the publication of this prior systematic review, a great deal of attention has been paid to the consequences of non-medical switching by medical and patient advocate organizations and state legislatures [Citation4–Citation7] and new studies/data have been generated and published [Citation8–Citation18]. Both the Cochrane Handbook for Systematic Reviews of Interventions [Citation19] and the methods guide for the Agency for Healthcare Research and Quality (AHRQ) Evidence-based Practice Center program [Citation20] remark on the importance of updating of a systematic review as new evidence becomes available. Therefore, we performed an update of the prior systematic review with the aim of further evaluating current knowledge of the impact of non-medical switching on clinical and economic endpoints, resource utilization and medication-taking behaviors.
Materials and methods
Preparation of this report was in accordance with the Preferred Reporting Items for Systematic Reviews and Meta-Analyses (PRISMA) statement [Citation21]. The goal of this guideline is to facilitate the transparent reporting of systematic reviews and meta-analyses to assess the benefits and harms of a health-care intervention.
Search strategy
We performed an updated systematic literature search from November 2015 (end date of prior systematic literature search) through 1 October 2018 in Medline and Web of Science (WoS) using the same search strategy as the prior review (). WoS was selected as a second literature source to maximize capture of abstracts from major medical and managed care meetings. The search was limited to human studies in the English language and excluded case studies, editorials and review articles. Bibliographical database searches were augmented by manual searches of the reference sections of relevant studies (‘backward citation tracking’) and a review of the first 500 results of a Google Scholar search.
Table 1. MEDLINE search strategy.
Study selection
Two investigators screened citations and assessed eligible studies for inclusion in duplicate with disagreement reconciled through discussion and consensus. Studies were included in this review if they were real-world studies performed in the USA (US), included ≥25 patients and evaluated the impact of non-medical switching of prescription drugs on at least one endpoint of interest. For the purposes of this review, non-medical switching was defined as switching to a chemically distinct but clinically similar medication for reasons other than lack of clinical efficacy or response, adverse effects or poor adherence. Outcomes of interest for this review included clinical (i.e., any measure of patient health including patient satisfaction), economic (i.e., medical or treatment costs, excluding index drug costs), resource utilization (i.e., office or emergency room visits or hospitalizations) and medication-taking behavior (i.e., adherence, persistence or discontinuation). Studies evaluating the impact of simple brand to generic substitution, changes in dosage form or route of administration or evaluating temporary (i.e., in-hospital) non-medical switches were excluded.
Data abstraction
Two investigators, through use of a standardized tool, independently abstracted all data with disagreements resolved by discussion. The following data were sought from each study: first author’s last name, year of publication; medication(s) switched; primary disease state; data source; duration of follow-up; data required for validity assessment (including whether the study was performed by the entity responsible for/dictating the non-medical switch; Appendix 1); funding source; whether disease stability prior to the switch was adequately demonstrated; endpoint type (clinical, economic, health-care utilization, medication-taking behavior); and direction and statistical significance of association between non-medical switching and each reported endpoint(s).
Validity assessment
The internal validity of the included studies was assessed using an adapted version of the Newcastle–Ottawa Scale (NOS) (Appendix 1) [Citation22]. A total of eight items within four domains (selection of study group, comparability of study group, ascertainment of exposure and ‘other’) were evaluated. Under the domain ‘other’, we assessed whether studies were performed separately from the entity responsible for dictating the non-medical switch. If a study satisfactorily met a criterion, a star (*) was assigned for that item; otherwise a minus sign (-) was noted. Each study was assessed by two investigators independently, with disagreements resolved through discussion and consensus.
Data synthesis
The direction of the association between non-medical switching and each individual endpoint identified within included studies was classified as negative, positive or neutral. An association was deemed ‘negative’ if the non-medical switch reflected a worsening of the endpoint and was statistically significant. For example, endpoints reflecting decreased adherence, worsened clinical outcomes, increased health-care utilization or increased costs were deemed negative. ‘Positive’ associations were those in which the endpoint improved in a statistically significant manner. For example, endpoints reflecting increased adherence, improved clinical outcomes, decreased health-care utilization or decreased costs were deemed positive. If no statistically significant relationship was observed between the switch and the endpoint, the direction of association was deemed ‘neutral’. In all cases, the p-value used to conclude statistical significance was based on each study’s defined p-value. Statistical significance was not reported for three usable endpoints from 2 studies; thus, we independently analyzed the data to estimate a p-value [Citation12,Citation23]. When studies reported overlapping endpoints (e.g., any hospitalization versus disease-specific hospitalization), the most disease-specific endpoint was included. If a datapoint was reported by a study in both a continuous and dichotomous way (e.g., hemoglobin A1c value versus attainment of goal hemoglobin A1c [Citation11]) the continuous endpoint was preferentially used as it provides a higher level of information. We did not evaluate economic endpoints that included the change in index (for non-medical switching) medication costs in the overall analysis, but did report these costs separately.
The overall frequency in which non-medical switching was associated with a negative, neutral or positive impact on all endpoints was reported as percentages. As with the prior systematic review, each included study was classified into one of the two ‘non-medical switching’ categories based on whether disease stability prior to the switch was demonstrated. Category (A) consisted of studies in which patients were demonstrated to have stable or well-controlled disease; whereas, Category (B) studies did not attempt to document this. The definition of stable or well-controlled disease varied across included studies but generally required patients to meet objective, disease-specific endpoints (e.g., laboratory values or vital signs) or not requiring supplemental resource utilization (e.g., emergency room visits or hospitalizations) within a defined period prior to the non-medical switch. The frequencies of each type of endpoint were stratified by the direction of association, non-medical switch category (A or B) and endpoint type. Additional stratified analyses were performed to determine if there was a time trend in the number and results of non-medical switching studies (2015–2018, 2010–2014, 2005–2009, 2000–2004), if the results (frequency in which endpoints were negative, neutral or positive) varied by duration of study follow-up (<6 months versus ≥6 months) or between studies performed by the entity responsible for/dictating the non-medical switch (to assess the potential for reporting biases). For all stratified analyses, the direction of the association between non-medical switching and each individual endpoint was again classified negative, positive or neutral via the definitions utilized in the summary of the overall results.
Results
Study characteristics
The initial search strategy yielded 3,177 citations and an additional 14 citations were manually identified (). After screening and full-text assessment, 38 studies (11 studies added based on the updated search) with a total of 260,256 patients (median: 591; range: 31–102,076) and a median follow-up of 6 months (range: 0.5 to 18 months) were included in our review () [Citation8–Citation18,Citation23–Citation49]. A total of 26 studies (68.4%) were published between 2000 and 2014, while 12 (31.6%) were published between 2015 and 2018. A majority of studies (n = 23; 60.5%) assessed the impact of non-medical switching in cardio-metabolic disease including hypertension, hyperlipidemia or diabetes, followed by immune-mediated conditions (n = 5; 13.2%), acid suppression (n = 3; 7.9%), psychiatric disorders (n = 2; 5.3%) and other miscellaneous other conditions (n = 5; 13.2%). The largest proportion of studies acquired data through chart review (n = 16; 42.1%), followed by claims (n = 9; 23.7%), claims with integrated electronic health records (n = 7; 18.4%) and other methods (n = 6; 15.8%). Twenty (52.6%) studies were externally funded. According to non-medical switch categories A (patients with stable/well-controlled disease) and B (patients without documented stable/well-controlled disease), there were 11 (28.9%) and 27 (71.1%) studies, respectively. A total of 25 (65.8%) studies were performed by the entity responsible for/dictating the non-medical switch.
Table 2. Characteristics of included studies.
Validity assessment results
Upon validity assessment using the NOS, only 4 of the 38 studies were given a minus sign in >1 evaluated item, suggesting a lower risk of bias in most included studies (Appendix 2). One study in category B relied on patient self-report for the ascertainment of the non-medical switch and was not awarded a star for this item [Citation12]. Three studies (one in category A and two in category B) were unmatched and were not given a star for comparability of study population. Three studies (all in category B) did not meet criteria for low risk of bias within one of the endpoint domains because they utilized either a subjective outcome and/or the duration or completeness or follow-up were inadequate. Finally, 25 studies were not awarded a star because they were performed by the entity dictating the non-medical switch.
Endpoint level results
The 38 included studies reported 154 eligible endpoints (64 added based on updated search). The most frequent outcome type was clinical (n = 93; 60.4%), followed by resource utilization (n = 33; 21.4%), economic (n = 24; 15.6%) and medication-taking behavior (n = 4; 2.6%) (). Nearly 40% (n = 65) of outcomes were from studies published between 2015 and 2018. Forty-nine (31.8%) of the endpoints came from category A studies (i.e., those requiring patients to have stable/well-controlled disease). Two-thirds of endpoints were from studies that followed patients for ≥6 months (n = 97; 63.0%) and more than half were from a study performed by the entity dictating the non-medical switch (n = 92; 59.7%). Qualitatively, the direction of association was negative (n = 48; 31.2%) or neutral (n = 91; 59.1%) more often than it was positive (n = 15; 9.7%).
Table 3. Overall sub-classification of outcomes.
When stratified by outcome type, non-medical switching was associated with a negative impact on clinical, economic, health-care utilization and medication-taking behavior outcomes in 26.9%, 41.7%, 30.3% and 75.0% of cases, respectively; and a positive effect was seen in only 3.0% (resource utilization) to 14.0% (clinical) of outcomes (). Only seven studies reported index drug costs and the effects were negative, neutral and positive in two, one and four studies, respectively.
Figure 2. Direction of Outcomes in All Studies and Stratified by Non-medical Switch Categories*.
*Panel I = all studies; II = category A studies; III = category B studies
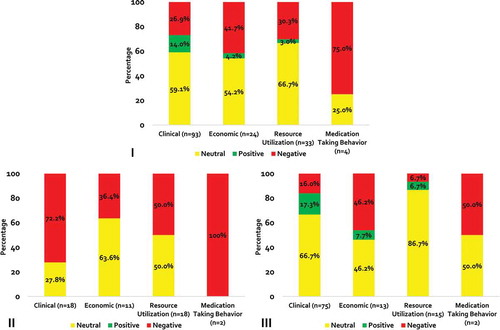
When analysis was restricted to category A studies (patients with stable/well-controlled disease), 28 (57.1%) and 21 (42.9%) had a negative and neutral direction of association (). Non-medical switching was not positively associated with any outcome in category A studies. Most clinical outcomes had a negative direction of association (n = 13; 72.2%). All medication-taking behavior outcomes (n = 2; 100%), half (n = 9) of all resource utilization outcomes and 36.4% (n = 4) of economic outcomes were negative. Out of a total of 105 outcomes in category B (patients without documented stable/well-controlled disease), the direction of association was negative for 20 (19.0%) outcomes, neutral for 70 (66.7%) outcomes, and positive for 15 (14.3%) outcomes (). The majority of clinical and resource utilization outcomes were neutral (n = 50; 66.7% and n = 13; 86.7%, respectively). Half of mediation taking behavior outcomes (n = 1) and 46.2% (n = 6) of economic outcomes were negative.
A greater proportion of endpoints from studies published between 2015 and 2018 were negative when compared to outcomes from studies published before 2015 (35.4% versus 28.1%; ). Endpoints from studies with a duration of follow-up ≥6 months were also more frequently negative (35.1% versus 23.2% in studies with <6 months of follow up; ). Nearly 90% of endpoints from studies performed by the entity dictating the non-medical switch were neutral or positive; whereas, only 40.3% of endpoints from studies not conducted by the entity mandating the switch were neutral or positive ().
Discussion
In this updated systematic review, non-medical switching continued to be frequently associated with negative outcomes. Less than 10% of endpoints had a positive direction of association. Among the subset of studies including patients with stable/well-controlled disease, non-medical switching was most frequently associated with negative clinical endpoints, and non-medical switching was not positively associated with any endpoint in this subset.
Most endpoints in the present systematic review were from studies performed by the same entity dictating/requiring the non-medical switch. Of these outcomes, ~90% were neutral or positive, while the majority of endpoints in studies conducted separately from the entity dictating the non-medical switch were negative. This suggests the possibility of reporting biases, as studies showing an intervention are not beneficial are less likely to be published. Future studies evaluating non-medical switching should be performed by researchers unrelated to the entities mandating the switch to remove such potential biases.
In addition to the data from studies included in this systematic review, surveys suggest both clinicians and patients view non-medical switching in a negative fashion [Citation12,Citation50–Citation52]. In a survey of 569 clinicians, 77% stated that, in their opinion, it was common for the new medication to be less effective after a non-medical switch [Citation50]. Nearly one-half of the clinicians also expressed concern regarding an increased risk of side effects with the new medication; with 37% of clinicians reporting the need to start a new medication to alleviate side effects they believed to be caused by the non-medical switch. Finally, persistence to the new medication was also a concern, with ~44% of respondents reporting at least one patient needing to switch back to their original medication. A survey of 143 patients performed by a special commission established by the Massachusetts Legislature reported on patients’ impressions of non-medical switching; with 70% of respondents reporting decreased effectiveness, 86% reporting worse side effects and 48% reporting having to try multiple medications before finding an alternative that worked after a non-medical switch [Citation51]. Nearly all (94%) of respondents noted they were in favor of legislation that would prohibit insurers from financially pressuring them to switch medications for non-medical reasons.
Non-medical switching has been addressed in guidance documents and position statements by medical, legislative and patient advocate organizations [Citation1,Citation2,Citation4–Citation7,Citation51,Citation53–Citation55]. An American Medical Association (AMA) policy on standards for drug formularies and therapeutic interchange strongly discourages non-medical switching for ambulatory patients with chronic diseases [Citation1]. The AMA has also endorsed a set of prior authorization and management reform principles Citation[4]; which includes a principle aimed at lessening the negative impact that unanticipated formulary changes could have on patients’ access to medication. This principle states that when a medication is removed from formulary after the beneficiary enrollment period is over, it should be covered for the duration of the benefit year; which at a minimum provides a buffer period for patients experiencing a non-medical switch. Reports initiated by state legislatures have also addressed non-medical switching [Citation5,Citation51]. A 2018 report to the Massachusetts Legislature by a commission formed to study medication switching concluded that for patients on stable medication regimens, unplanned changes can result in harm (e.g., physical, psychological and financial distress) [Citation51]. Patient advocate organizations have released position statements on non-medical switching [Citation6,Citation7,Citation53–Citation55]. One such example, the National Diabetes Volunteer Leadership Council, emphasized their disapproval of the practice of non-medical switching and has called for patients with well-controlled diabetes to be allowed to continue the medications that allow them to achieve adequate glycemic control [Citation53].
Though this paper reports on an update of a prior systemic review, the present findings should not be undervalued. Both Cochrane Collaboration and AHRQ’s Evidence-based Practice Center Program recommend that authors evaluate the need to update a systematic review based on several factors, including stakeholder impact (e.g., importance to guideline developers or policy makers) as well as the amount and impact of new information [Citation19,Citation20,Citation56,Citation57]. Of note, more than 40% of the endpoint data included in our updated systematic review were from studies published between 2015 and 2018; meaning the updated review added a substantial amount of new data to the previously conducted review [Citation3]. Moreover, the rate of publication of studies evaluating non-medical switching appears to be increasing; as have the number of guidance documents and position statements on the topic [Citation4–Citation7,Citation51,Citation53–Citation55]. This suggests the topic is considerably important to multiple stakeholder and decision-making groups. It is also worth noting that endpoints evaluated from studies published between 2015 and 2018 were more frequently negative as compared to earlier studies (i.e., 35% versus 28%). Therefore, it seems prudent to continue to closely monitor the literature describing the consequences of non-medical switching.
Our systematic review has several limitations worthy of discussion. Due to methodological characteristics of included studies, it cannot be ruled out that some ‘neutral’ findings would have been found to be significant if study’s internal validity were improved. For example, the median follow-up duration (i.e., 6 months) for studies in the review might not be long enough to capture the most important endpoints, especially for the many cardio-metabolic conditions. While decreased control of surrogate endpoints (e.g., hemoglobin A1c, blood pressure, cholesterol levels) might be assessable within 6 months of a non-medical switch, the impact of switching on final health outcomes (e.g., microvascular complications of diabetes, myocardial infarction, stroke) would likely take >6 months to manifest [Citation58]. This hypothesis is further supported by the fact that endpoints from studies with follow-up ≥6 months in our systematic review were more frequently negative. In addition, many studies may not have been adequately powered to show statistical differences for all endpoints assessed, and thus, would be classified as ‘neutral’. For these reasons, additional and more rigorous research into the impact of non-medical switching is warranted. Next, endpoints with a large magnitude of effect received the same weight as those with a smaller magnitude of effect, and endpoints were not valued upon their corresponding clinical significance. Results were not combined statistically in a meta-analysis due to differences in the types (e.g., clinical, economic, resource utilization or medication-taking behavior) and definitions of endpoints; which would be expected to result in substantial heterogeneity if studies were meta-analyzed. We were also not able to assess endpoints in specific disease state categories, as studies assessed a heterogeneous set of conditions resulting in small study/endpoint numbers. Lastly, ~70% of included studies did not provide strong clinical data to demonstrate patients truly had stable/well-controlled disease. This limitation may bias our results towards more neutral or positive outcomes.
Conclusion
Non-medical switching in ambulatory patients was commonly associated with either negative or neutral outcomes and was seldom associated with positive ones. Among the subset of studies conducted by groups that performed/dictated the non-medical switch, outcomes were mostly neutral and positive. For this subset of studies, the possibility of reporting bias cannot be excluded. Non-medical switching was associated with mainly negative effects on clinical outcomes in patients with stable/well-controlled disease.
Disclosure statement
No potential conflict of interest was reported by the authors.
Additional information
Funding
References
- H-125.991 Drug formularies and therapeutic interchange. Chicago, IL: American Medical Association; 2010. [cited 2018 Nov 1]. Available from: https://policysearch.ama-assn.org/policyfinder/detail/Drug%20Formularies%20and%20Therapeutic%20Interchange%20H-125.991?uri=%2FAMADoc%2FHOD.xml-0-227.xml
- Gray T, Bertch K, Galt K, et al. ACCP position statement: guidelines for therapeutic interchange – 2004. Pharmacotherapy. 2005;25:1666–15.
- Nguyen E, Weeda ER, Sobieraj DM, et al. Impact of non-medical switching on clinical and economic outcomes, resource utilization and medication-taking behavior: a systematic literature review. Curr Med Res Opin. 2016;32:1–10.
- Prior Authorization and Utilization Management Reform Principles. American Medical Association. 2017 [cited 2018 Nov 10]. Available from: https://www.ama-assn.org/sites/default/files/media-browser/principles-with-signatory-page-for-slsc.pdf
- Non-Medical Switching of Medications in Select States. Connecticut general assembly. Office of legislative research. 2017 [cited 2018 Nov 10]. Available from: https://www.cga.ct.gov/2017/rpt/pdf/2017-R-0201.pdf
- How utilization management protocols can block access to life-saving treatments. The doctor-patient rights project. 2018 [cited 2018 Nov 11]. Available from: http://dontswitchme.org/wp-content/uploads/2018/01/DPRP-UM-Report-1.pdf
- Promoting access to affordable prescription drugs: policy analysis and consumer recommendations for state policymakers, consumer advocates, and health care stakeholders. 2016 [cited 2018 Nov 11]. Available from: https://consumersunion.org/wp-content/uploads/2016/08/Promoting-Access-to-Affordable-Prescription-Drugs_Aug-2016.pdf
- Gibofsky A, Skup M, Mittal M, et al. Effects of non-medical switching on outcomes among patients prescribed tumor necrosis factor inhibitors. Curr Med Res Opin. 2017;33:1945–1953.
- Rosenblatt L, Buikema AR, Seare J, et al. Economic outcomes of first-line regimen switching among stable patients with HIV. J Manag Care Spec Pharm. 2017;23:725–734.
- Wolf D, Skup M, Yang H, et al. Clinical outcomes associated with switching or discontinuation from Anti-TNF inhibitors for nonmedical reasons. Clin Ther. 2017;39:849–862.
- Blonde L, Burudpakdee C, Divino V, et al. The impact of non-medical switch on type 2 diabetes patients treated with Canagliflozin in the commercially insured US population. Curr Med Res Opin. 2018;34:1–21.
- Flores NM, Patel CA, Bookhart BK, et al. Consequences of non-medical switch among patients with type 2 diabetes. Curr Med Res Opin. 2018;34:1475–1481.
- Naville-Cook C, Rhea L, Triboletti M, et al. Analyzing the clinical outcomes of a rapid mass conversion from rosuvastatin to atorvastatin in a VA medical center outpatient setting. J Pharm Technol. 2017;33:189–194.
- Boktor M, Motlis A, Aravantagi A, et al. Substitution with Alternative Anti-TNFα Therapy (SAVANT)-outcomes of a Crohn’s disease cohort undergoing substitution therapy with certolizumab. Inflamm Bowel Dis. 2016;22:1353–1361.
- Yip E, Karimi S, Pien LT. Evaluation of a therapeutic interchange from fluticasone/salmeterol to mometasone/formoterol in patients with chronic obstructive pulmonary disease. J Manag Care Spec Pharm. 2016;22:316–323.
- Andreoli MT, Pinnolis M, Kieser T, et al. Feasibility and efficacy of a mass switch from ranibizumab (Lucentis) to bevacizumab (Avastin) for treatment of neovascular age-related macular degeneration. Digit J Ophthalmol. 2015;21:1–17.
- Asias BD, Stock EM, Small NL, et al. Clinical and financial outcomes of switching insulin glargine to insulin detemir in a veteran population with type 2 diabetes. J Diabetes Metab Disord. 2015;14:53.
- Low GA, Weilburg JB. Rockett H et al. Therapeutic substitution from atorvastatin to simvastatin: impact on LDL control. Am J Pharm Benefits. 2015;7:e37–e43.
- Higgins JPT, Thomas J, Chandler J, Cumpston M, Li T, Page MJ, Welch VA (editors). Cochrane Handbook for Systematic Reviews of Interventions. 2nd Edition. Chichester, UK: John Wiley & Sons, 2019.
- Tsertsvadze A, Maglione M, Chou R, et al. Updating comparative effectiveness reviews: current efforts in AHRQ’s effective health care program. J Clin Epidemiol. 2011;64:1208–1215.
- Liberati A, Altman DG, Tetzlaff J, et al. The PRISMA statement for reporting systematic reviews and meta-analyses of studies that evaluate healthcare interventions: explanation and elaboration. BMJ. 2009;339:b2700.
- Wells GA, Shea B, O’Connell D, et al. The Newcastle-Ottawa Scale (NOS) for assessing the quality of nonrandomised studies in meta-analyses. Ottawa, ON: Ottawa Hospital Research Institute; 2014. [cited 2018 Oct 31]. Available from: http://www.ohri.ca/programs/clinical_epidemiology/oxford.asp
- Cheetham TC, Chan J, Benson V, et al. Successful conversion of patients with hypercholesterolemia from a brand name to a generic cholesterol-lowering drug. Am J Manag Care. 2005;11:546–552.
- Liu Y, Skup M, Lin J, et al. Impact of non-medical switching on healthcare costs: a claims database analysis. Value Health. 2015;18:A252.
- Saseen JJ, Ghushcyan V, Kaila S, et al. Maintaining goal blood pressures after switching from olmesartan to other angiotensin receptor blockers. J Clin Hypertens. 2013;15:888–892.
- Fugit RV, Resch ND. Conversion of patients from simvastatin to lovastatin in an outpatient pharmacy clinic. Am J Health-Syst Pharm. 2000;57:1703–1708.
- Gates R, Cookson T, Ito M, et al. Therapeutic conversion from fosinopril to benazepril at a Veterans Affairs medical center. Am J Health-Syst Pharm. 2006;63:1066–1068.
- Billups SJ, Plushner SL, Olson KL, et al. Clinical and economic outcomes of conversion of simvastatin to lovastatin in a group-model health maintenance organization. J Manag Care Pharm. 2005;11:681.
- Manzo BA, Matalka MS, Ravnan SL. Evaluation of a therapeutic conversion from amlodipine to felodipine. Pharmacotherapy. 2003;23:1508–1512.
- Graham MR, Allcock NM. Irbesartan substitution for valsartan or losartan in treating hypertension. Ann Pharmacother. 2002;36:1840–1844.
- Taylor AJ, Grace K, Swiecki J, et al. Lipid-lowering efficacy, safety and costs of a large-scale therapeutic statin formulary conversion program. Pharmacotherapy. 2001;21:1130–1139.
- Good CB, Fultz SL, Trilli L, et al. Therapeutic substitution of cimetidine for nizatidine was not associated with an increase in healthcare utilization. Am J Manag Care. 2000;6:1141–1146.
- Oatis MGM, Stowers AD. Conversion from amlodipine to felodipine ER: did the change fulfill expectations? Formulary. 2000;35:435–442.
- Kamal KM, Zacker C, Civitarese L. Switching between different ARBs in patients with controlled BP. Am J Pharm Benefits. 2012;4:e81–7.
- Signorovitch J, Bao Y, Samuelson T, et al. Switching from adalimumab to other disease-modifying antirheumatic drugs without apparent medical reasons in rheumatoid arthritis: impact on health care service use. Ann Rheum Dis. 2012;71(suppl3):717.
- West JC, Rae DS, Mojtabai R, et al. Clinically unintended medication switches and inability to prescribe preferred medications under Medicare Part D. J Psychopharmacol. 2012;26:784–793.
- Wu EQ, Yu AP, Lauzon V, et al. Economic impact of therapeutic substitution of a brand selective serotonin reuptake inhibitor with an alternative generic selective serotonin reuptake inhibitor in patients with major depressive disorder. Ann Pharmacother. 2011;45:441–451.
- Signorovitch J, Zhang J, Wu EQ, et al. Economic impact of switching from valsartan to other angiotensin receptor blockers in patients with hypertension. Curr Med Res Opin. 2010;26:849–860.
- Bryant GA, McDanel DL, Horner KE, et al. Evaluation of dosing and clinical outcomes in patients undergoing conversion of insulin glargine to insulin detemir. Pharmcotherapy. 2013;33:56–62.
- Alemayehu B, Ke X, Youssef NN, et al. Esomeprazole formulary exclusion: impact on total health care services use and costs. Postgrad Med. 2012;124:149–163.
- Miller AE, Hansen LB, Saseen JJ. Switching statin therapy using a pharmacist-managed therapeutic conversion program versus usual care conversion among indigent patients. Pharmacotherapy. 2008;28:553–561.
- Meissner B, Dickson M, Shinogle J, et al. Drug and medical cost effects of a drug formulary change with therapeutic interchange for statin drugs in a multistate managed Medicaid organization. J Manag Care Pharm. 2006;12:331.
- Tran F, Boggie DT, Delattre ML, et al. Therapeutic interchange involving replacement of rofecoxib or celecoxib with valdecoxib. Am J Health-Syst Pharm. 2004;61:1391–1394.
- Andrade SE, Gurwitz JH, Cernieux J, et al. Evaluation of a formulary switch from conjugated to esterified estrogens in a managed care setting. Med Care. 2000;38:970–975.
- Clay DR, Bourg MP, Lawrence DB. Outcomes of an amlodipine-to-felodipine therapeutic interchange program. Am J Health-Syst Pharm. 2000;57:1604–1607.
- Mamdani MM, Reisig CJ, Stevenson JG. Cost analysis of therapeutic interchange of calcium channel blockers for the treatment of hypertension: unexpected results from a conversion program. J Manag Care Pharm. 2000;6:390–394.
- Nelson WW, Vermeulen LC, Geurkink EA, et al. Clinical and humanistic outcomes in patients with gastroesophageal reflux disease converted from omeprazole to lansoprazole. Arch Intern Med. 2000;160:2491–2496.
- Parra D, Beckey NP, Korman L. Retrospective evaluation of conversion of amlodipine to alternative calcium channel blockers. Pharmacotherapy. 2000;20:1072–1078.
- Walters J, Noel H, Folstad J, et al. Prospective evaluation of the therapeutic interchange of felodipine ER for amlodipine in patients with hypertension. Hosp Pharm. 2000;35:48–51.
- Cote BR, Petersen EA. Impact of therapeutic switching in long-term care. Am J Manag Care. 2008;14:SP23–8.
- Special commission to study switching medications report to the massachusetts legislature. 2018 [cited 2018 Nov 10]. Available from: https://www.mass.gov/files/documents/2018/06/06/final-report-special-commission-report-on-medication-switching.pdf
- Global Healthy Living Foundation. Patient perspectives on medication switching for non-medical reasons: a survey of stabilized patients by the Global Healthy Living Foundation. Upper Nyack, NY: Global Healthy Living Foundation; 2015 [cited 2018 Nov 4]. Available from: http://www.50statenetwork.org/wp-content/uploads/2015/04/GHLF-Switching-Stable-Patients-Survey_Summary.pdf
- Ellingson L. Position on non-medical switching for diabetes patients. J Diabetes Metab Disord Control. 2017;4:138‒139.
- Access to Care Position Statement. Lupus and Allied Disease Associations, Inc cited 2018 Nov 10 Available from: http://www.nolupus.org/LADAFinalAccessPosition.pdf
- Issue Brief: Non-medical switching and step therapy. Hemophilia foundation of America. 2016 [cited 2018 Nov 10]. Available from: http://www.hemophiliafed.org/uploads/PGR_IssueBrief_NonMedicalSwitching_2016-10-25.pdf
- Cochrane. Editorial and publishing policy resource, 2016 [cited 2018 Nov 1]. Available from: http://community.cochrane.org/editorial-and-publishing-policy-resource
- Garner P, Hopewell S, Chandler J, et al. When and how to update systematic reviews: consensus and checklist. BMJ. 2016;354:i3507.
- Bikdeli B, Punnanithinont N, Akram Y, et al. Two decades of cardiovascular trials with primary surrogate endpoints: 1990–2011. J Am Heart Assoc. 2017;6:1–9.
Appendices
Appendix 1.Modified Newcasttle-Ottawa Quality Assessment Scale for Evaluation of the Impact of Non-Medical Switching
Note: A study can be awarded a maximum of one star (*) for each numbered item within each category for a maximum of eight stars (*)
Selection
1) Representativeness of the ‘non-medical switch’ cohort
a) truly representative of the average patient with [disease state] in the community *
b) somewhat representative of the average patient with [disease state] in the community *
c) selected group of users e.g., nurses, volunteers
d) no description of the derivation of the cohort
2) Selection of the comparator/non-switch cohort (i.e., patients who continued therapy or patients prior to the switch)
a) drawn from the same community as the non-medical switch cohort *
b) drawn from a different source
c) no description of the derivation of the non-switch cohort
3) Ascertainment of non-medical switch
a) secure record (e.g., patient chart or electronic health records) *
b) structured interview *
c) assumed due to patient selection criteria
c) written self-report
d) no description
Comparability
1) Comparability of cohorts on the basis of the design or analysis
a) study matched non-medical switch and non-switch patients or performs multivariable regression analyses *
Outcome
1) Assessment of outcome
a) independent blind assessment *
b) record linkage *
c) self-report
d) no description
2) Was follow-up long enough for outcomes to occur
a) yes *
3) Adequacy of follow up of cohorts
a) complete follow up – all subjects accounted for *
b) subjects lost to follow up unlikely to introduce bias – small number lost (10%) OR description provided of those lost *
c) follow up rate <90% and no description of those lost
d) no statement
Other
1) Entity conducting study
a) Study conducted by entity not involved in the non-medical switch (i.e., the entity did not dictate the switch)*
Appendix 2.Modified Newcastle-Ottawa Quality Assessment Scale Results by Non-medical Switch Category
Studies were given a star (*) or minus sign (-) in each domain if they were determined to have high or low quality, respectively.
NMS = non-medical switch