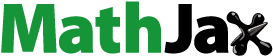
ABSTRACT
Background and objective: Although the treatment of chronic hepatitis C (CHC) has significantly evolved with the introduction of direct-acting antivirals, the treatment uptake rates have been low especially among marginalized groups in the UK, such as people who inject drug (PWID) and men who have sex with men (MSM). Cutting health inequality is a major focus of healthcare agencies. This study aims to identify the optimal allocation of treatment budget for chronic hepatitis CHC among populations and treatments in the UK so that liver-related mortality in patients with CHC is minimized, given the constraint of treatment budget and equity issue.
Methods: A constrained optimization modelling of resource allocation for the treatment of CHC was developed in Excel from the perspective of the UK National Health System over a lifetime horizon. The model was designated with the objective function of minimizing liver-related deaths by varying the decision variables, representing the number of patients receiving each treatment (elbasvir-grazoprevir, ombitasvir-paritaprevir-ritonavir-dasabuvir, sofosbuvir-ledipasvir, and pegylated interferon-ribavirin) in each population (the general population, PWID, and MSM). Two main constraints were formulated including treatment budget and the issue of equity. The model was populated with UK local data applying linear programming and underwent internal and external validation. Scenario analyses were performed to assess the robustness of model results.
Results: Within the constraints of no additional funding over original spending in status quo and the consideration of the issue of equity among populations, the optimal allocation from the constrained optimization modelling (treating 13,122 PWID, 160 MSM, and 904 general patients with ombitasvir-paritaprevir-ritonavir-dasabuvir) was found to treat 2,430 more patients (relative change: 20.7%) and avert 78 liver-related deaths (relative change: 0.3%) compared with the current allocation. The number of patients receiving treatment increased 4,928 (relative change: 60.1%) among PWID and 42 (relative change: 35.8%) among MSM.
Conclusion: The current allocation of treatment budget for CHC is not optimal in the UK. More patients would be treated, and more liver-related deaths would be avoided using a new allocation from a constrained optimization modelling without incurring additional spending and considering the issue of equity.
Introduction
Hepatitis C, as a slowly progressing infectious disease arising from the hepatitis C virus (HCV), is of growing international concern due to the substantial burden of HCV-related morbidity and mortality [Citation1–4]. During the two decades after infection, 27% of those with chronic HCV infection develop liver cirrhosis and 25% develop hepatocellular carcinoma [Citation5,Citation6]. Liver-related deaths due to decompensated cirrhosis and hepatocellular carcinoma, drug-related deaths due to drug overdose and suicide, and HIV-related deaths due to HCV and HIV coinfection are the three major groupings for mortality of chronic hepatitis C (CHC) [Citation7]. Although the transmission of HCV infection worldwide has remained stable based on a declined prevalence, the number of patients with advanced liver diseases including decompensated cirrhosis and hepatocellular carcinoma are growing [Citation8], which has posed a serious challenge for countries’ health spending and threaten the sustainability of funding for viral hepatitis elimination plans of the World Health Organization (WHO) [Citation9].
In the UK (UK), an estimated 160,000 people are chronically infected with HCV [Citation10], and 24,600 patients with a treatment episode in the NHS hepatitis C patients register were recorded in 2018 [Citation11]. People who inject drug (PWID) and men who have sex with men (MSM) are the most at-risk populations, which are disproportionately affected by CHC in the UK [Citation12,Citation13]. PWID even contributed to more than 90% of new infections in 2016 [Citation14]. As marginalized groups, PWID and MSM are generally not in routine clinical care [Citation15] and report low treatment uptake rates ranging from 0.5% to 2.5% [Citation16]. Preventing people from dying prematurely is the primary goal of the CHC care pathway defined by the UK National Health System (NHS) policies [Citation17]. As informed by WHO targets, the All-Party Parliamentary Group (APPG) on Liver Health Inquiry in the UK has heard from patients, leading clinicians, NHS managers, senior pharmaceutical industry representatives, and national public health policy leads. The elimination of hepatitis Cincluded a reduction in liver-related deaths, increased awareness of hepatitis C among PWID and MSM, a minimum of 20,000 new treatment initiations per year, proportional treatment targets to be sets for different populations, and universal access to treatment [Citation18].
Injection-based pegylated interferon-ribavirin (PR) used to be the standard of care for CHC, lasted between 24 and 48 weeks, resulted in significant unpleasant side effects, and reported an overall sustained virus response (SVR) less than 50% [Citation19]. The approach to treatment for CHC continues to evolve with the introduction of tablet-based direct-acting antivirals (DAAs) in Europe in 2015, lasting 8 to 12 weeks, resulting in few side effects and reporting an SVR upwards of 95% for all genotypes [Citation20]. However, the high list prices of DAAs have led many countries and jurisdictions in Europe to restrict reimbursement only for the patient with advanced fibrosis, without drug or alcohol use, and with a specialist prescriber [Citation21]. In the UK, despite the affordable deals on drug costs between NHS and industry and the economic value of increased treatment among PWID and MSM [Citation22–24], a broader treatment funding distributed to PWID and MSM is often lost, and cutting health inequality is a major focus of the NHS plan [Citation18]. Both government agencies and clinicians require evidence-based advice on the practical implementation of utilizing DAAs most efficiently.
Current resource allocation within health technology assessment (HTA) in the UK relies primarily on the cost-effectiveness analysis (CEA) and budget impact analysis (BIA). CEA typically ignores the constraints and barriers to implementation, including budget constraints, capacity constraints, issue of equity, and issue of ethics [Citation25]. They are not flexible in allocating resources among different populations. BIA, however, only integrated the budget constraints in deliberations rather than in quantitative analysis. A constrained optimization modelling approach has been used to solve problems in many fields, such as allocation funding among different investments in financial planning, blending different crude oils to produce gasoline and logistics planning in the military [Citation26]. In healthcare, constrained optimization modelling has been used for the purposes of capacity management, selection of facility location [Citation27–29], development of optimal treatment algorithms [Citation30], design of clinical trials [Citation31], and optimal allocation of resources [Citation32–39], whose value was further introduced by International Society for Pharmacoeconomics and Outcomes Research (ISPOR) task force on Constrained Optimization Methods [Citation40,Citation41]. However, most published constrained optimization studies are not transparent enough in constructing, solving, and reporting constrained optimization modelling and the applications of constrained optimization modelling have been slow [Citation26]. Combining traditional economic evaluations with constrained optimization modelling, this study aims to identify the optimal allocation of budget to fund treatments for CHC among populations and treatments in the UK so that liver-related deaths in patients with CHC are minimized, given the constraint of treatment budget and other implementation barriers.
Methods
A constrained optimization modelling of resource allocation for the treatment of CHC was developed in Excel and to work in conjunction with an underlying core Markov model for CHC from the perspective of UK NHS over a lifetime horizon, following the guidance of Constrained Optimization Methods in Health Services Research from the ISPOR task force [Citation40,Citation41].
Problem structuring
According to the targets of elimination hepatitis C set by APPG in the UK, the constrained optimization modelling was designated with the objective function LD(X) of minimizing liver-related deaths by varying the decision variables Xij, representing the number of patients receiving each treatment (j = 1, 2, 3, 4) in each population (i = 1, 2, 3). The optimal patient distribution among treatments and population groups from the constrained optimization model was compared with the current status quo in the UK.
The four treatment strategies including elbasvir-grazoprevir (EBR/GRZ), ombitasvir-paritaprevir-ritonavir-dasabuvir (OBV/PTV/r), sofosbuvir-ledipasvir (SOF/LED) and the previous standard of care (PR) were analysed in the modelling. The three DAAs strategies were selected because they: 1) were recommended by the National Institute for Health and Care Excellence (NICE) for the same indication; and 2) were the predominant strategies utilised in the UK according to the NHS hepatitis C register. The comparative data on efficacy and safety and utilization distribution of four selected strategies were reported in the NICE network-meta-analysis [Citation42] and treatment monitoring report from Public Health England [Citation11], respectively. The general population, and two high-risk populations, including PWID and MSM in the UK were analysed in the modelling [Citation43].
Two main constraints were formulated: treatment budget represented by B and issue of equity represented by E. The treatment budget constraint was the treatment spending in status quo in the UK, calculated as the sum of product of number of treated patients and total lifetime treatment costs in each population [Citation10]. The equity constraint was examined based on the recommendation of proportional treatment for populations developed by APPG, which stated that each patient group could receive only the share of the overall treatment costs proportionate to its size of infected cases in the UK status quo [Citation10,Citation18].
The parameters of constrained optimization modelling included total lifetime costs per patient (TCij and NCi) and liver-related death rates (TDij and NDi) with each treatment or with no treatment in each population. The number of infected patients in each population was represented by Ni.
The liver-related deaths and the number of patients receiving each treatment from the optimal allocation were compared with the status quo of the treatment of CHC in the UK among the general population (1,223 with OBV/PTV/r; 718 with EBR/GRZ; 1,056 with SOF/LED; and 447 with PR), PWID (2,909 with OBV/PTV/r; 1,708 with EBR/GRZ; 2,513 with SOF/LED; and 1,064 with PR), and MSM (42 with OBV/PTV/r; 25 with EBR/GRZ; 36 with SOF/LED; and 15 with PR). In the current status quo, the number of patients receiving each treatment was calculated based on the number of treated patients among each population groups collected from the treatment monitoring report published by the Public Health England [Citation11] and utilization distribution of four selected strategies derived from UK real-world evidence [Citation44]. The liver-related deaths were simulated using the model.
Mathematical formulation
The mathematical problem was defined as follows:
Minimize
Subject to
Budget constraint
Issue of equity
(i = 1, 2 and 3 represents the general population, PWID and MSM, respectively)
(j = 1, 2, 3 and 4 represents EBR/GRZ, OBV/PTV/r, SOF/LED and PR, respectively)
Model development
The development of the constrained optimization modelling for CHC treatment consists of programming the mathematical problem and obtaining the input parameters to estimate objective function and constraints. Linear programming was applied based on one critical condition that a linear incremental change in the independent variable, namely decision variable, and constraint, results in a linear incremental change in the dependent output, namely objective function [Citation38,Citation45]. The programming was developed in Microsoft Excel Solver [Citation32,Citation39,Citation46,Citation47]. In the absence of comparative data on clinical and cost outcomes between PWID/MSM and the general population from long-term follow-up studies to populate the programming, a core Markov model [Citation48–50] widely used with many local adaptations [Citation51–61] for evaluating the chronic progression and treatment of CHC was further adapted to compare clinical and cost outcomes of CHC treatment among different populations in the UK setting. The structure of the core Markov model () was kept as reported in the previous study [Citation62]. Population-specific input including mortality, reinfection, and HRQoL was added in the core model. The local population-specific data was derived from a systematic review of economic evaluation models for the treatment of CHC [Citation62] and extensive reviews of the epidemiologic burden of CHC among the general and high-risk populations [Citation1–4]. The outcomes of the core Markov model including total lifetime treatment costs per patient and liver-related death rates with each strategy and without any treatment in each population were embedded in the constrained optimization programming to determine the optimal allocation.
Patients enter the model with chronic HCV (F0-F3) or compensated cirrhosis (F4) defined by Metavir score and receive pharmacological treatment for CHC. Patients could transit to the next stage of severity (decompensated cirrhosis and hepatocellular carcinoma) and require liver transplants (different health states between the first year and subsequent years). Patients could die at any time according to the UK general mortality and increased mortalities due to advanced liver complications were considered. Recovered patients could get reinfected, leading patients back from the recovered states to chronic HCV states.
The input for the general population (Additional file 1.1) was obtained from Johnson et al. [Citation63] and NICE committee paper [Citation42], including input of baseline characteristics, transition probabilities, clinical efficacy in terms of SVR, health states utilities, and treatment costs consisting of drug costs, treatment monitoring costs, treatment costs of drug-related adverse events and medical treatment costs of different health states.
The input for PWID and MSM was kept identical to that for the general population except for input of mortality, reinfection and utility among PWID, and input of reinfection and utility among MSM (). An increased mortality due to overdose [Citation64], a higher reinfection rate due to high-risk behaviour including needle sharing [Citation65,Citation66], and lower HRQoL due to substance use [Citation67–70] were considered among PWID. A higher reinfection rate due to high-risk sexual activities and network characteristics [Citation15,Citation71,Citation72] and lower HRQoL due to poorer mental health and psychological problems [Citation70,Citation73–75] were considered among MSM.
Table 1. Main difference in Markov inputs for PWID, MSM and the general population
All costs were inflated to the year 2019. Cost and health outcomes were discounted at a 3.5% annual rate based on the UK guidelines [Citation76].
Model validation
Two types of validation were applied to the constrained optimization modelling using linear programming to ensure that appropriate techniques motivated by real-world observations were employed [Citation77]: internal validation and external validation. The conceptualization of the decision problem, model structure, data sources, assumptions, coding, and results were validated by an independent analyst with a pre-defined checklist. The cost and clinical outcomes from the core Markov model were compared with those from the other UK models [Citation48–50,63].
Scenario analysis
Two scenario analyses were performed to assess the robustness of model results, by: 1) changing the objective function from minimization of liver-related deaths to maximization of quality-adjusted life-years (QALYs) gained and adopting only budget constraint. Then the results of optimal allocation were compared with those of ICER-based priority applied by NICE; 2) investigating the minimum additional funding required to treat at least 20,000 people every year to reach the HCV elimination target in the UK, as suggested by APPG.
Results
Parameters derived from the core Markov model
The total lifetime treatment costs per patient with CHC and liver-related death rates with each strategy and without any treatment, derived from the core Markov model, are presented in . The detailed cost outcomes and other clinical outcomes are shown in Additional file 1.2.
Table 2. Parameters derived from the core Markov model
The total lifetime treatment costs per patient with each strategy among three populations ranged from a lower value set of £44,991 (OBV/PTV/r), £48,272 (EBR/GRZ), £50,321 (SOF/LED), and £66,613 (PR) among the general population, to a median value set of £45,483 (OBV/PTV/r), £48,660 (EBR/GRZ), £50,815 (SOF/LED), and £63,852 (PR) among PWID, then to a higher value set of £50,396 (OBV/PTV/r), £53,587 (EBR/GRZ), £55,736 (SOF/LED), and £68,718 (PR) among MSM. The total lifetime treatment costs per patient without any treatment among three populations were incurred by the medical treatment costs of different health states of CHC and ranged from £27,850 among PWID to £32,769 among MSM and the general population.
The liver-related death rates with each strategy among three populations ranged from a lower value set of 15.96% (OBV/PTV/r), 15.89% (EBR/GRZ), 15.88% (SOF/LED), and 18.37% (PR) among PWID, to a median value set of 18.38% (OBV/PTV/r), 18.31% (EBR/GRZ), 18.28% (SOF/LED), and 21.37% (PR)among the general population, then to a higher value set of 19.27% (OBV/PTV/r), 19.22% (EBR/GRZ), 19.20% (SOF/LED), and 21.92% (PR) among MSM. The liver-related death rates without any treatment among three populations ranged from 19.16% among PWID to 22.98% among MSM and the general population.
Optimal allocation of treatment resources in the base case
The optimal allocation of resources for the treatment of CHC using constrained optimization modelling is presented in , which summarized the decision variables and objective function in the optimal allocation and the comparison with the current status quo in the UK.
Table 3. Optimal allocation of treatment resources for CHC in the base case
Within the constraints of no additional funding over original spending in status quo and the consideration of the issue of equity among populations, the optimal allocation from the constrained optimization modelling (treating 13,122 PWID, 160 MSM, and 904 the general patients with OBV/PTV/r) was found to treat 2,430 more patients (relative change: 20.7%) and avert 78 liver-related deaths (relative change: 0.3%) compared with the current allocation. The number of patients receiving treatment increased 4,928 (relative change: 60.1%) among PWID and 42 (relative change: 35.8%) among MSM.
Model validation
The conceptualization of the decision problem was based on the clinical practice of CHC treatment and validated by a health economics expert (S.A.). The specification and value of parameters in constrained optimization modelling were selected based on the UK real-world evidence [Citation8,Citation10,Citation18,Citation66,Citation78–81] identified by extensive reviews [Citation1–4] and derived from the core Markov model in the UK setting populated with UK local data identified by the systematic review [Citation62]. The numbers of liver-related deaths estimated by this model are consistent with those currently observed in the UK [Citation82].
Scenario analysis
In the first scenario of maximizing QALY within the constraint of no additional funding and without the consideration of the issue of equity, the allocation results from the constrained optimization model were the same as the standard allocation from the ICER-based priority (). Compared with no treatment, OBV/PTV/r treatment among the general patients was found to lead to the lowest ICER value among the three populations. Optimal allocation (treating 20,066 the general patients with OBV/PTV/r) was found to treat 5,880 more patients (relative change: 41.4%), increase 17,303 more QALYs gained (relative change: 1.3%) compared with the base case optimal allocation. The change of objective function from the minimization of liver-related deaths to the maximization of QALYs gained did not impact the optimal allocation in this case, but the change of constraint did.
Table 4. Optimal allocation in the scenario of ICER-based analysis
In the second scenario, increasing the funding by 2.1% with the consideration of the issue of equity was found to raise the overall number of patients receiving treatment to 20,000 to reach the HCV elimination target, as suggested by APPG recommendation ().
Table 5. Optimal allocation to reach APPG target
Discussion
This study showed that even without additional funding over original spending and within the constraint of equity in resource allocation among populations, the optimal allocation from the constrained optimization modelling could treat more patients and avert more liver-related deaths compared with the status quo of treating CHC patients in the UK.
Implementation of constrained optimization modelling
The value of constrained optimization modelling in resource allocation has been introduced by several studies. Juusola et al. [Citation39] developed a linear resource allocation model alongside the traditional static CEA to determine the optimal mix of HIV treatment and prevention so that QALYs gained and HIV infections averted were maximized, given a fixed budget. In accordance with our analyses of liver-related deaths and QALYs gained, the choice of objective function did not change the optimal resource allocation. However, the approximating diminishing with linear objective function in Jessie et al. analyses could lead to a less accurate estimate. Lasry et al. [Citation38] developed a nonlinear allocation model alongside the epidemic model to apport HIV prevention resources among interventions and populations so that HIV incidence was minimized, given a fixed budget. However, the structure of the epidemic model of HIV did not include the stratification of geography, age, and race. It did not report the type of behavior change intervention, which weaken its impact on dictating healthcare resource allocation. Demarteau et al. [Citation32] developed a linear allocation model alongside the Markov model to identify the mix of cervical cancer prevention strategies so that the cancer cases were minimized, given a fixed budget. In line with our results of ICER-based scenario analysis, Demarteau et al. reported that when only considering budget constraints, the resource allocation of a constrained optimization model obtained the same results as that of a CEA with ICER-based priority. However, the static Markov model could not be accounted for the dynamic effect of vaccination on infection, such as herd protection, and the constrained optimization modelling could not address equity problems. Earnshaw et al. [Citation33] developed a linear allocation model alongside the Markov model to select sets of interventions so that the QALYs gained of patients with type II diabetes were maximized, given budget and equity constraints. However, only drug costs were included in the model.
Constrained optimization modelling has been also applied in hepatitis C. Busschots et al. [Citation47] developed a constrained optimization modelling in Excel to demonstrate the number of HCV patient need to treat per year to reach the WHO targets for HCV elimination in Belgium, given the constraints of the number of known HCV patients, annual HCV incidence and the number of years until the year 2030 is reached. However, no specific treatment or prevention interventions were incorporated in the model. Chhatwal et al. [Citation83] developed a Markov microsimulation model to inform the optimal timing of HCV treatment for patients on the liver transplant waiting list. However, only two scenarios were compared, treatment on the waiting list versus treatment after the liver transplant, which made the term ‘optimal’ loosely used and made the analysis closer to a traditional CEA.
Despite the potential value of constrained optimization modeling, the implementation in healthcare has been slow and not been based on evidence that demonstrates the optimal solution in a mathematical sense.
Added value of this optimization modelling
Constrained optimization modeling is an emerging tool to expand the comparison of different allocations of resources across population groups. Rather than the comparison of one health technology versus another health technology in a traditional CEA and BIA, the optimization approach expands across various technologies and across population groups. Equitable patient access was addressed in this constrained optimization modelling to integrate the health equity between the general population and marginalised high-risk populations. The impact of reinfection on treatment outcomes and lower HSUVs among marginalised high-risk populations were addressed, which were not always considered in the limited CEAs among PWID [Citation84,Citation85] and MSM [Citation24].
Constrained optimization modeling is a flexible tool to solve different decision problems based on a simple yet accurate framework. It can be either embedded with traditional models or populated with data directly from literature. Although there are four treatments among three different population groups in our analysis, the structure of the decision problem is still straightforward. The simplicity of constrained optimization modelling design in Excel allows it to be adapted easily and used for different types of decision problems, such as determining the mix of interventions and populations and investigating the additional funding need in our analyses. Furthermore, constrained optimization modelling could be applied in determining the timing to initiate treatment [Citation86] and the price of health technologies [Citation45], which could not readily be included in a traditional CEA or BIA. In our analysis, the objective of finding optimal distribution of treatment budget to minimize the liver-related deaths in CHC patients requires decisions different from those typically made in a CEA or BIA. What’s more, our analysis addressed public health problems with minor reformulations: 1) what is the effect of considering equity issue between population groups; and 2) how much increased funding is needed to reach a specific target, which cannot be answered in a CEA or BIA.
Constrained optimization modelling is a practically implementable tool to solve the context-specific problem. It allows the decision-makers to set a variety of constraints reflecting local conditions, such as limited budget, limited coverage rates, limited capacity, and measure of equity, for deciding a particular problem. Data from the national treatment monitoring database in the UK were derived to populate the modelling. The early data in the register suggest that PWID and MSM are starting to be identified via local and outreach services. As more data become available, linking these data to targeting allocation of resources for treating patients would improve equity of access to treatment and inform the progress of eliminating hepatitis C in the UK. Common resource allocation methodologies including cost-effectiveness ranking and net-benefit analysis [Citation87] cannot provide quantitative information for policymakers about how to allocate funds among the interventions and population groups and how many extra funds are still needed to reach specific public health target and cannot include equity or other implementation constraints. Our analyses of the base case and different scenarios can help policymakers allocate limited funds and decide the amount of additional funding and reveal the acceptable levels of costs and health outcomes to trade-off for equity consideration.
Limitations of this optimization analysis
Only the general population and high-risk populations including PWID and MSM were analysed in constrained optimization modelling. The other at-risk populations in the UK [Citation12] included prisoners, immigrants, commercial sex workers, homeless people, and hemodialysis patients. The prisoners and immigrants were another contribution subgroup to hepatitis C. According to the statistics from the Ministry of Justice in 2018 [Citation88], the total number of prisoners in the UK was 83,123. The estimated range of HCV seroprevalence in prisoners was from 1.3%-19.2% [Citation43], and 32% of prisoners reported drug use history [Citation89]. The relatively high HCV seroprevalence among prisoners is caused by a high population of PWID in prison and unsafe injecting and tattooing taking place within prison. There is also a high seroprevalence of HCV within some South Asian (ranging from 0.0% to 9.6%) and Eastern European (ranging from 3.1%-9.3%) immigrants due to a high risk of infection from unsafe medical and dental care or unsterile shaving and barber’s equipment [Citation43]. The accurate estimates on the overlap between PWID and prisoners or immigrants were not clear due to the lack of data. However, the population subgroups in the analysis covered more than 90% of CHC patient in the UK, including the general population (n = 66,000), PWID (n = 119,850) and MSM (n = 1,800) [Citation90].
Besides treatment initiatives to lead to a decline in liver-related deaths and in overall prevalence, the care pathway for hepatitis C spans the entire range of interventions from preventing, testing, and linking to care. Harm reduction including needle and syringe provision (NSP) and opioid substitution treatment (OST) was recognised as a key strategy for PWID to prevent transmission. Similarly, protected sexual behaviour campaigns and education about transmission risks are crucial for MSM. The indirect effect of ‘treatment as prevention’ was not considered in this analysis. The effect of prevention interventions, however, have been investigated in other dynamic transmission models among PWID [Citation91–97] and MSM [Citation98,Citation99].
Given limited comparative data between the general population and high-risk populations including PWID and MSM, a set of assumptions was made in the core Markov model. The non-AIDS mortality in MSM was used as the proxy of HCV-mono-infected MSM, which was not significantly different compared with that in the general norm (hazard ratio:0.61, p = 0.440) according to an observational cohort study conducted in Spain [Citation100]. A clinical trial conducted in the UK reported that the increased disease progression of CHC was associated with male gender, old age, and alcohol assumption greater than 40 units per week [Citation101]. To our knowledge, however, no studies confirmed the accelerated liver disease progression among PWID and MSM. Therefore, a conservative assumption of identical transition probability was used in the model. A poorer HRQoL among PWID [Citation67,Citation68,Citation70] and MSM [Citation70,Citation73–75] compared to the population norm was reported in many qualitative humanistic burden studies. However, no utility elicitation studies reported the comparative differences from hepatitis C patients. An adjustment of 0.8 for health states utility values (HSUVs) of PWID used by several CEAs [Citation69,Citation102] was applied and a conservative assumption of adjustment of 0.9 for HSUVs of MSM was made in the scenario analysis of ICER-based priority. However, the main objective function in the base case was to minimize liver-related deaths and the choice of the objective function in our analysis did not change the resource allocation.
This analysis only partially addressed the equity issue, which considered that all populations could be reached with identical treatment uptake rates. In this case, the constrained optimization modelling tended to maximize the treatment uptake rates among high-risk populations, which were not always included in routine clinical practice. It also considered each population as homogeneous. However, with added information, the modelling could be adapted to take into account regional disparities, such as differences in epidemiology, contact rate, healthcare capacity, and costs in large countries with diverse reginal health settings.
The constraint of capability, such as healthcare practitioners and laboratory equipment, was not considered in the modelling. However, aligned with WHO targets, the delivery of treatment of CHC in the UK has been expanded in the community facilitates without referral to specialist care [Citation18]. With the ease of dispending treatment in the community, all general practitioners could be accredited to deliver treatment with less spending. Old PR treatment requires sophisticated laboratory capacity to diagnose the presence of chronic infection, the viral genotype, and assess the degree of liver fibrosis. Combinations of DAAs treatments, however, are effective against all genotypes and much safer than PR, thus preventing the need for genotyping and reducing the complexity of monitoring for adverse events.
Recommendations for future studies
Given the study results, the generation of new clinical studies for the treatment efficacy of DAAs among PWID and MSM with CHC are suggested. The gold standard of a clinical study is the double-blinded randomized controlled trial RCT. Typical RCTs of DAAs for CHC included the general patients with serum positive for HCV and excluded patients with other causes for the liver disease other than CHC and substance abuse [Citation103]. The stigma surrounding hepatitis C within MSM communities was reported as significantly higher than stigma surrounding HIV [Citation18]. Including PWID and MSM in the clinical trial and following up with them to complete the full treatment protocol could be difficult but meaningful to get information about viral kinetics and treatment outcome by performing key comparisons between PWID/MSM and the general patients. In this case, HSUVs for CHC of PWID and MSM could be collected alongside the trial to generate patient preference-related evidence which is not only commonly used in a typical CEA, but also could be used in constrained optimization modelling.
The indirect effect of ‘treatment as prevention’ is suggested to be expanded. The future model could simulate the movement of PWID through different injecting durations, prevention intervention states, and injecting risk [Citation92]. Stratifications by injecting duration, and ex-injecting drug users, could capture the higher rates of injecting cessation during the first few years of injecting and the varying death rates according to the different injecting duration categories. Stratifications by different prevention intervention states, such as no intervention, on OST only, on NSP only, and both OST and NSP, could capture the different compliance to intervention, which may have an impact on treatment discontinuation. Stratifications by high and low injecting risk, with high-risk being defined as being homeless and/or a crack cocaine injector that has been shown to increase the risk of HCV infection acquisition among PWID, which results in more benefit of ‘treatment as prevention’. The future model could simulate the movement of MSM through different interaction behaviour with sexual partnerships [Citation104], HIV pre-exposure prophylaxis states, and HIV infection status [Citation105]. Stratifications by sexual partnerships could include ‘regular partnerships’ (relationships lasting more than one time step), ‘casual partnerships’ (individuals who meet up with for casual sex on more than one occasion), and ‘random partnerships’ (individuals who meet up with for only a single interaction) to capture different sexual risk and the potential compliance to treatment.
The inequities in the treatment of CHC root in the multiple social and structural factors including HCV stigma, housing, access to social and welfare supports, access to employment and income, and CHC treatment delivery system [Citation106]. Treatment alone is not enough to eradicate HCV and the inequities in CHC testing and treatment also need to be addressed to achieve hepatitis C elimination targets. The evidence for case-finding and linkage to care was summarized by Public Health England to support decision-making on prioritisation of resources [Citation107]. The integrated resource allocation covering the whole care pathway of CHC is suggested from awareness-raise, prevention, testing, diagnosis, treatment, to monitoring.
The detailed process of the model validation for the constrained optimization modelling is suggested in further studies of constrained optimization modelling. Demarteau et al. [Citation32] and Juusola et al. [Citation39] reported the validation of optimization analyses by only investigating the impact of changing the value of key parameters on model results. The future optimization model should be valid for all possible combinations of decision variables as suggested by the ISPOR guidelines [Citation40].
Conclusion
The current allocation of treatment budget for CHC is not optimal in the UK. More patients would be treated and more liver-related deaths would be avoided using a new allocation from a constrained optimization modelling without incurring additional spending and considering the issue of equity.
Author contribution
All authors contributed to study conception and design. Ru Han contributed to the model construction, acquisition of data, analysis and interpretation of data and first draft of the manuscript. Samuel Aballea and Shuyao Liang contributed to the model validation. Clément François and contributed to critical revision of the manuscript for important intellectual content. Clément François and Mondher Toumi contributed to supervision of the study. All authors read and approved the final manuscript.
Disclosure statement
The authors declare that the research was conducted in the absence of any commercial or financial relationships that could be construed as a potential conflict of interest.
Data availability statement
The data relating to the model parameters are all included in the article and/or its supplementary materials. The model used in the study can be made available to researchers upon request.
Additional information
Funding
References
- Han R, Zhou J. Disease burden of hepatitis c infection in Asia: an overview of systematic review. Value Health. 2018;21:S63.
- Han R, Zhou J. Comparison of clinical burden of Hcv infection between Asia and Europe: an overview of systematic review. Value Health. 2018;21:S63.
- Han R, Zhou J. Humanistic and economic burden of HCV infection: an overview of systematic review. Value in Health. 2018;21:S65–S66.
- Han R, Zhou J, François C, et al. Prevalence of hepatitis C infection among the general population and high-risk groups in the EU/EEA: a systematic review update. BMC Infect Dis. 2019;19(1):655.
- Thein HH, Yi Q, Dore GJ, et al. Estimation of stage-specific fibrosis progression rates in chronic hepatitis C virus infection: a meta-analysis and meta-regression. Hepatology. 2008;48(2):418–14.
- Butt AA, Yan P, Lo Re V 3rd, et al.Liver fibrosis progression in hepatitis C virus infection after seroconversion. JAMA Intern Med. 2015;175(2):178–185.
- Grebely J, Dore GJ. What is killing people with hepatitis C virus infection? Semin Liver Dis. 2011;31(4):331–339.
- European Union HCV Collaborators. Hepatitis C virus prevalence and level of intervention required to achieve the WHO targets for elimination in the European union by 2030: a modelling study. Lancet Gastroenterol Hepatol. 2017;2(5):325–336.
- Hatzakis A, Lazarus JV, Cholongitas E, et al. Securing sustainable funding for viral hepatitis elimination plans. Liver Int. 2020;40(2):260–270.
- Harris HE, Harris R CA, Mandal S. Hepatitis C in England, 2019 report: working to eliminate hepatitis C as a major public health threat. London: Public Health England; 2019.
- Harris HE, Costella A, Mandal S, et al. Hepatitis C treatment monitoring in England: Content, completeness and preliminary findings from the Hepatitis C patient registry and treatment outcome system. November 2018. Public Health England, London.
- NICE. Hepatitis B and C testing: people at risk of infection public health guideline 2012 Mar 2013; Available from: https://www.nice.org.uk/guidance/ph43.
- WHO. guidelines on hepatitis B and C testing. Geneva: World Health Organization; 2017. License: CC BY-NC-SA 3.0 IGO.
- Public Health England, H.P.S.. public health wales, and public health agency Northern Ireland, shooting up: infections among people who inject drugs in the UK 2016.. London: Public Health England; 2017.
- Nijmeijer BM, Koopsen J, Schinkel J, et al. Sexually transmitted hepatitis C virus infections: current trends, and recent advances in understanding the spread in men who have sex with men. J Int AIDS Soc. 2019;22(6):e25348.
- Martin NK, Foster GR, Vilar J, et al. HCV treatment rates and sustained viral response among people who inject drugs in seven UK sites: real world results and modelling of treatment impact. J Viral Hepat. 2015;22(4):399–408.
- NHS England. Operational delivery networks for hepatitis C care in adults. 2017. https://www.england.nhs.uk/wp-content/uploads/2018/08/Operational-delivery-networks-for-hepatitis-C-care-adult.pdf
- P.P.G.o.L.H.I. A. Report. All-party parliamentary group on liver health inquiry report, eliminating hepatitis C in England. All-Party Parliamentary Group on Liver Health. 2018.
- National institute for health and clinical excellence. Peginterferon alfa and ribavirin for the treatment of mild chronic hepatitis C [NICE technology appraisal guidance106]. Revised Nov 2007. Available from: http://www.nice.org.uk/nicemedia/live/11590/33534/33534.pdf Accessed 2019 Jul 22
- Han RZJ. PIN19 - a cost comparison of treating chronic hepatitis c patients with sofosbuvir-based regimens. Value Health. 2018;21:S224.
- Marshall AD, Cunningham EB, Nielsen S, et al. Restrictions for reimbursement of interferon-free direct-acting antiviral drugs for HCV infection in Europe. Lancet Gastroenterol Hepatol. 2018;3(2):125–133.
- Martin NK, Vickerman P, Foster GR, et al. Can antiviral therapy for hepatitis C reduce the prevalence of HCV among injecting drug user populations? A modeling analysis of its prevention utility. J Hepatol. 2011;54(6):1137–1144.
- Martin NK, Vickerman P, Grebely J, et al. Hepatitis C virus treatment for prevention among people who inject drugs: modeling treatment scale-up in the age of direct-acting antivirals. Hepatology. 2013;58(5):1598–1609.
- Martin NK, Thornton A, Hickman M, et al. Can hepatitis C virus (HCV) direct-acting antiviral treatment as prevention reverse the HCV epidemic among men who have sex with men in the UK? epidemiological and modeling insights. Clin Infect Dis. 2016;62(9):1072–1080.
- Thokala P, Dixon S, Jahn B. Resource modelling: the missing piece of the HTA jigsaw? Pharmacoeconomics. 2015;33(3):193–203.
- Earnshaw S. Are We Ready to Use Constrained Optimization in Health Outcomes Research? Value Health. 2018 Sep;21(9):1029-1030.
- Ndiaye M, Alfares H. Modeling health care facility location for moving population groups. Comput Oper Res. 2008;35(7):2154–2161.
- Araz C, Selim H, Ozkarahan I. A fuzzy multi-objective covering-based vehicle location model for emergency services. Comput Oper Res. 2007;34(3):705–726.
- Verter V, Lapierre SD. Location of preventive health care facilities. Ann Oper Res. 2002;110(1–4):123–132.
- Ehrgott, M., Güler, Ç., Hamacher, H.W. Mathematical optimization in intensity modulated radiation therapy. Ann Oper Res. 2010;175(1):309–365.
- Bertsimas D, Farias VF, Trichakis N. Fairness, efficiency, and flexibility in organ allocation for kidney transplantation. Operations Research. 2013;61(1):73–87.
- Demarteau N, Breuer T, Standaert B. Selecting a mix of prevention strategies against cervical cancer for maximum efficiency with an optimization program. Pharmacoeconomics. 2012;30(4):337–353.
- Earnshaw SR, Richter A, Sorensen SW, et al. Optimal allocation of resources across four interventions for type 2 diabetes. Med Decis Making. 2002;22(5):S80–91. Suppl.
- Alistar SS, Long EF, Brandeau ML, et al. HIV epidemic control-a model for optimal allocation of prevention and treatment resources. Health Care Manag Sci. 2014;17(2):162–181.
- Brandeau ML, Zaric GS, Richter A. Resource allocation for control of infectious diseases in multiple independent populations: beyond cost-effectiveness analysis. J Health Econ. 2003;22(4):575–598.
- Lasry A, Sansom SL, Hicks KA, et al. Allocating HIV prevention funds in the USA: recommendations from an optimization model. PLoS One. 2012;7(6):e37545.
- Lasry A, Richter A, Lutscher F. Recommendations for increasing the use of HIV/AIDS resource allocation models. BMC Public Health. 2009;9(1): S8.
- Lasry A, Sansom SL, Hicks KA, et al. A model for allocating CDC’s HIV prevention resources in the USA. Health Care Manag Sci. 2011;14(1):115–124.
- Juusola JL, Brandeau ML. HIV treatment and prevention: a simple model to determine optimal investment. Med Decis Making. 2016;36(3):391–409.
- Crown W, Buyukkaramikli N, Thokala P, et al. Constrained optimization methods in health services research-an introduction: report 1 of the ISPOR optimization methods emerging good practices task force. Value Health. 2017;20(3):310–319.
- Crown W, Buyukkaramikli N, Sir MY, et al. Application of constrained optimization methods in health services research: report 2 of the ISPOR optimization methods emerging good practices task force. Value Health. 2018;21(9):1019–1028.
- NICE National Institute for Health and Care Excellence. National institute for health and care excellence single technology appraisal Elbasvir-grazoprevir for treating chronic hepatitis C [ID842]. 2016.
- European centre for disease prevention and control. hepatitis B and C epidemiology in selected population groups in the EU/EEA. ECDC: Stockholm: 2018. https://www.ecdc.europa.eu/en/publications-data/hepatitis-b-and-c-epidemiology-selected-population-groups-eueea
- Macken L, Gelson W, Priest M, et al. Efficacy of direct-acting antivirals: UK real-world data from a well-characterised predominantly cirrhotic HCV cohort. J Med Virol. 2019;91(11):1979–1988.
- Lauria DT, Maskery B, Poulos C, et al. An optimization model for reducing typhoid cases in developing countries without increasing public spending. Vaccine. 2009;27(10):1609–1621.
- Becker NG, Starczak DN. Optimal vaccination strategies for a community of households. Math Biosci. 1997;139(2):117–132.
- Busschots D, Toghanian S, Bielen R, et al. Eliminating viral hepatitis C in Belgium: the micro-elimination approach. BMC Infect Dis. 2020;20(1):181.
- Shepherd J, Waugh N, Hewitson P. Combination therapy (interferon alfa and ribavirin) in the treatment of chronic hepatitis C: a rapid and systematic review. Health Technol Assess. 2000;4(33):1–67.
- Shepherd J, Brodin H, Cave C, et al. Pegylated interferon alpha-2a and −2b in combination with ribavirin in the treatment of chronic hepatitis C: a systematic review and economic evaluation. Health Technol Assess. 2004;8(39):iii–iv, 1–125.
- Shepherd J, Jones J, Hartwell D, et al. Interferon alpha (pegylated and non-pegylated) and ribavirin for the treatment of mild chronic hepatitis C: a systematic review and economic evaluation. Health Technol Assess. 2007;11(11):1–205. iii.
- Annemans L, Warie H, Nechelput M, et al. A health economic model to assess the long term effects and cost-effectiveness of PEG IFN alpha-2a in hepatitis C virus infected patients. Acta Gastroenterol Belg. 2004;67(1):1–8.
- García-Contreras F, Nevárez-Sida A, Constantino-Casas P, et al. Cost-effectiveness of chronic hepatitis C treatment with thymosin alpha-1. Arch Med Res. 2006;37(5):663–673.
- Hornberger J, Farci P, Prati D, et al. The economics of treating chronic hepatitis C patients with peginterferon alpha-2a (40 kDa) plus ribavirin presenting with persistently normal aminotransferase. J Viral Hepat. 2006;13(6):377–386.
- Lidgren M, Hollander A, Weiland O, et al. Productivity improvements in hepatitis C treatment: impact on efficacy, cost, cost-effectiveness and quality of life. Scand J Gastroenterol. 2007;42(7):867–877.
- Lin WA, Tarn YH, Tang SL. Cost-utility analysis of different peg-interferon alpha-2b plus ribavirin treatment strategies as initial therapy for naive Chinese patients with chronic hepatitis C. Aliment Pharmacol Ther. 2006;24(10):1483–1493.
- Nakamura J, Kobayashi K, Toyabe S, et al. The cost-effectiveness of the new protocol reflecting rapid virologic response to peginterferon alpha-2b and ribavirin for chronic hepatitis C. Eur J Gastroenterol Hepatol. 2007;19(9):733–739.
- Salomon JA, Weinstein MC, Hammitt JK, et al. Cost-effectiveness of treatment for chronic hepatitis C infection in an evolving patient population. Jama. 2003;290(2):228–237.
- Sullivan SD, Jensen DM, Bernstein DE, et al. Cost-effectiveness of combination peginterferon alpha-2a and ribavirin compared with interferon alpha-2b and ribavirin in patients with chronic hepatitis C. Am J Gastroenterol. 2004;99(8):1490–1496.
- Sullivan SD, Craxi A, Alberti A, et al. Cost effectiveness of peginterferon alpha-2a plus ribavirin versus interferon alpha-2b plus ribavirin as initial therapy for treatment-naive chronic hepatitis C. Pharmacoeconomics. 2004;22(4):257–265.
- Wong J, Nevens F. Cost-effectiveness of peginterferon alfa-2b plus ribavirin compared to interferon alfa-2b plus ribavirin as initial treatment of chronic hepatitis C in Belgium. Acta Gastroenterol Belg. 2002;65(2):110–111.
- McEwan P, Kim R, Yuan Y. Assessing the cost utility of response-guided therapy in patients with chronic hepatitis C genotype 1 in the UK using the MONARCH model. Appl Health Econ Health Policy. 2013;11(1):53–63.
- Han R, François C, Toumi M. Systematic review of health state utility values used in european pharmacoeconomic evaluations for chronic hepatitis C: impact on cost-effectiveness results. Applied Health Economics and Health Policy; 2020;19(1):29-44.
- Johnson SJ, et al. Economic evaluation of ombitasvir/paritaprevir/ritonavir and dasabuvir for the treatment of chronic genotype 1 hepatitis c virus infection. J Med Econ. 2016;19(10):983–994.
- Cornish R, et al. Risk of death during and after opiate substitution treatment in primary care: prospective observational study in UK general practice research database. Bmj. 2010;341:c5475.
- Aspinall EJ, et al. Treatment of hepatitis C virus infection among people who are actively injecting drugs: a systematic review and meta-analysis. Clin Infect Dis. 2013;57(2):S80–9. Suppl.
- Latham NH, et al. Staying hepatitis C negative: a systematic review and meta-analysis of cure and reinfection in people who inject drugs. Liver Int. 2019;39(12):2244–2260.
- Fischer JA, et al. Quality of life of people who inject drugs: characteristics and comparisons with other population samples. Qual Life Res. 2013;22(8):2113–2121.
- Dalgard O, et al. Health-related quality of life in active injecting drug users with and without chronic hepatitis C virus infection. Hepatology. 2004;39(1):74–80.
- Barnett PG, Zaric GS, Brandeau ML. The cost-effectiveness of buprenorphine maintenance therapy for opiate addiction in the USA. Addiction. 2001;96(9):1267–1278.
- Helbling B, et al. Host- rather than virus-related factors reduce health-related quality of life in hepatitis C virus infection. Gut. 2008;57(11):1597–1603.
- Richardson D, Fisher M, Sabin CA. Sexual transmission of hepatitis C in MSM may not be confined to those with HIV infection. J Infect Dis. 2008;197(8): 1213–1214. author reply 1214-5.
- Falade-Nwulia O, et al. Understanding and addressing hepatitis C reinfection in the oral direct-acting antiviral era. J Viral Hepat. 2018;25(3):220–227.
- Liu J, et al. The influence of social support on quality of life of men who have sex with men in China: a preliminary study. PLoS One. 2015;10(5):e0127644.
- Safren SA, Blashill AJ, O’Cleirigh CM. Promoting the sexual health of MSM in the context of comorbid mental health problems. AIDS Behav. 2011;15(1): S30–4. Suppl.
- Zhang P, et al. Effect of chronic disease self-management program on the quality of life of HIV-infected men who have sex with men: an empirical study in Shanghai, China. Int J Health Plann Manage. 2019;34(3):1055–1064.
- The National Institute for Health and Care Excellence. Excellence TNIFHAC. Guide to the methods of technology appraisal. 2013. https://www.nice.org.uk/process/pmg9/chapter/foreword
- McCarl BA, Apland J. Validation of linear programming models. 2016;18(2):155–164. Journal of Agricultural and Applied Economics, 1986;18(2):155-164.
- NHS England announces ‘huge investment’ for new hepatitis C treatments. Pharm J. June 20 2015;294(7867).
- Jin F, Matthews GV, Grulich AE. Sexual transmission of hepatitis C virus among gay and bisexual men: a systematic review. Sex Health. 2017;14(1):28–41.
- Immunisation HBSD. Public health england operational delivery network (ODN) area profiles: data and provisional modelling of the HCV epidemic. Public Health England; 2018.
- Gompels M, et al. The use of funnel plots with regression as a tool to visually compare HIV treatment outcomes between centres adjusting for patient characteristics and size: a UK Collaborative HIV Cohort study. HIV Med. 2018;19(6):386–394.
- Aspinall EJ, et al. Trends in mortality after diagnosis of hepatitis C virus infection: an international comparison and implications for monitoring the population impact of treatment. J Hepatol. 2015;62(2):269–277.
- Chhatwal J, et al. Optimal timing of hepatitis C treatment for patients on the liver transplant waiting list. Hepatology. 2017;65(3):777–788.
- Martin NK, et al. Prioritization of HCV treatment in the direct-acting antiviral era: an economic evaluation. J Hepatol. 2016;65(1):17–25.
- Martin NK, et al. Cost-effectiveness of hepatitis C virus antiviral treatment for injection drug user populations. Hepatology. 2012;55(1):49–57.
- Denton BT, et al. Optimizing the start time of statin therapy for patients with diabetes. Med Decis Mak. 2009;29(3):351–367.
- WL W. Operations research: applications and algorithms. Belmont, CA: Wadsworth; 1994.
- Ministry of justice. Statistics on prison population. 2018. https://www.gov.uk/government/collections/prison-population-statistics
- Taylor A, et al. Low incidence of hepatitis C virus among prisoners in Scotland. Addiction. 2013;108(7):1296–1304.
- De Angelis D, et al. An evidence synthesis approach to estimating hepatitis C prevalence in England and Wales. Stat Methods Med Res. 2009;18(4):361–379.
- Kwon JA, et al. Estimating the cost-effectiveness of needle-syringe programs in Australia. Aids. 2012;26(17):2201–2210.
- Platt L, et al. Public Health Research, in Assessing the impact and cost-effectiveness of needle and syringe provision and opioid substitution therapy on hepatitis C transmission among people who inject drugs in the UK: an analysis of pooled data sets and economic modelling. Southampton (UK): NIHR Journals Library; 2017.
- Pollack HA. Cost-effectiveness of harm reduction in preventing hepatitis C among injection drug users. Med Decis Making. 2001;21(5):357–367.
- Cousien A, et al. Effectiveness and cost-effectiveness of interventions targeting harm reduction and chronic hepatitis C cascade of care in people who inject drugs: the case of France. J Viral Hepat. 2018;25(10):1197–1207.
- Cousien A, et al. Hepatitis C treatment as prevention of viral transmission and liver-related morbidity in persons who inject drugs. Hepatology. 2016;63(4):1090–1101.
- Jacobs P, Calder P, Taylor M, et al. Cost effectiveness of streetworks’ needle exchange program of Edmonton. Can J Public Health. 1999;90(3):168–171.
- Jozaghi E, Reid AA, Andresen MA. A cost-benefit/cost-effectiveness analysis of proposed supervised injection facilities in Montreal, Canada. Subst Abuse Treat Prev Policy. 2013;8:25.
- Chen YH, Guigayoma J, McFarland W, et al. Increases in pre-exposure prophylaxis use and decreases in condom use: behavioral patterns among hiv-negative san francisco men who have sex with men, 2004-2017. AIDS Behav; 2018:23(7):1841-1845.
- Schmidt HA, McIver R, Houghton R, et al. Nurse-led pre-exposure prophylaxis: a non-traditional model to provide HIV prevention in a resource-constrained, pragmatic clinical trial. Sex Health; 2018;15(6):595-597.
- Coelho L, Grinsztejn B, Castilho JL, et al. Mortality in HIV-infected women, heterosexual men, and men who have sex with men in Rio de Janeiro, Brazil: an observational cohort study. The Lancet HIV. 2016;3(10):e490–e498.
- Asher AK, Portillo CJ, Cooper BA, et al. Clinicians‘ views of hepatitis C virus treatment candidacy with direct-acting antiviral regimens for people who inject drugs. Subst Use Misuse. 2016;51(9):1218–1223.
- Peter Vickerman AM, Williams J. Assessing the cost-effectiveness of interventions linked to needle and syringe programmes for injecting drug users. An economic modelling report. 2008.
- Wright M, Grieve R, Roberts J, et al. Health benefits of antiviral therapy for mild chronic hepatitis C: randomised controlled trial and economic evaluation. Health Technol Assess. 2006;10(21):1–113.
- Scott N, Stoové M, Wilson DP, et al. Eliminating hepatitis C virus as a public health threat among HIV-positive men who have sex with men: a multi-modelling approach to understand differences in sexual risk behaviour. J Int AIDS Soc. 2018;21(1):e25059.
- Macgregor L, Desai M, Martin NK, et al. Scaling up screening and treatment for elimination of hepatitis C among men who have sex with men in the era of HIV pre-exposure prophylaxis. EClinicalMedicine. 2020;19:100217.
- WHO. Barriers and facilitators to hepatitis C treatment for people who inject drugs. 2012. London School of Hygiene and Tropical Medicine, University of London, UK
- Public Health England., Hepatitis C: interventions for case-finding and linkage to care. 2019.
- NICE National Institute for Health and Care Excellence. Elbasvir–grazoprevir for treating chronic hepatitis C Technology appraisal guidance [TA413]. 2016.
- Berenguer J, Gil-Martin Á, Jarrin I, et al. All-oral direct-acting antiviral therapy against hepatitis C virus (HCV) in human immunodeficiency virus/HCV-coinfected subjects in real-world practice: madrid coinfection registry findings. Hepatology. 2018;68(1):32–47.