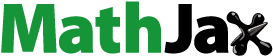
Abstract
A conceptual non-linear, systems-based R&D management model for the road and transport sector was developed to address the associated complexities of transport and road research. It takes account of all the elements of the innovation value chain as well as stakeholders. It stimulates systems-thinking in the R&D management process and allows for a non-linear approach to the process with a number of process pathways, both ‘backward’ (back casting) and ‘forward’ thinking, thus facilitating eventual impact and uptake. It includes an impact assessment ‘sensor’ that influences the process of R&D management. The model was used to augment key performance indicators in an existing transport and road research programme. It was shown that a systems-based approach can improve the impact assessment indicators of an R&D programme in a community access roads and transport research programme.
Introduction
Technological innovation has a significant impact on socioeconomic growth (Porter Citation1990; Pistorius Citation1996; Cainelli, Evangelista, and Savona Citation2010; Bessant et al. Citation2014). In resources-scarce countries it is of particular importance to achieve optimum performance of technological innovation by the effective management of and thus delivery from research and development (R&D) programmes. In poor countries such technological innovation can stimulate job creation and thus improve the quality of life of people.
The classical view of technological innovation centres around consumer product development, but in some fields of knowledge research often leads to ‘softer’ key solutions which could for example include new design methodologies used by government and professionals; often packaged in software. This type of activity is increasing (Spieth, Schneckenberg, and Ricart Citation2014). In road and transport engineering, for example, the core focus of research is to deliver new design methods, alternative construction methodologies, and new materials design methods that are related to civil engineering (Rust Citation2010). Some research in this field focuses on the development of construction equipment and new materials but the former aspects are paramount. These focus areas are of an interdisciplinary and multidisciplinary nature. As such, they form the bridge between the natural sciences (basic research) and the eventual use of the developed technologies. It has been shown that product development as well as process innovation create growth in the construction sector (Martínez-Román, Tamayo, and Gamero Citation2017).
In these disciplines, the development of human resources is essential to successful innovation (South African National R&D Strategy Citation2002). According to Cloete (Citation2014) South Africa has 1.5 full-time equivalent researchers per 1000 persons in the workforce. This is significantly lower than that of countries that have a similar ratio of R&D spending to GDP, such as Portugal (at 4.8) and Italy (at 3.60). The South African Institution for Civil Engineering (SAICE) found that the ratio of population to engineer in South Africa is of the order of 3200:1, twenty times less than those of countries like Australia, the USA, China and India (Wall and Rust Citation2015). In other African countries the ratio is even worse, ranging from 5400:1 in Kenya (Arasa Citation2012) to 49,000:1 in Uganda (Odango Citation2017). This is indicative of the low levels of investment in R&D and impacts severely on Africa's ability in technological innovation in the road and transport field. Although the South African government has recently increased its target for R&D expenditure to 1.5% of GDP by 2019, it is currently only at 0.73% (National Survey of Research & Experimental Development Citation2016). In the road and transport sector in particular, R&D expenditure is as low as 0.3% of industry turnover. These factors emphasize the complexity of managing R&D, particularly in sectors where the focus is mainly on new methodology and systems, the knowledge of which resides within key human resources.
Linear research management models that initiate with idea formulation, followed by research and then development, and subsequently by transfer or implementation of products and/or knowledge are not suitable for research into transport engineering where new knowledge generation and design methods are the primary outputs (Rust Citation2010). In this field the process is much more iterative with many feedback loops. Therefore, a new, improved research management process is required for this field (Rust Citation2015). This article relates the results of qualitative analysis of currently-used R&D management models. This was augmented with a survey among research managers from 42 companies and research organizations to assess the characteristics of research management methods and the extent of their use. From this work, Rust (Citation2015) defined 12 tenets that can be used to develop a research and development management model that would be applicable in road and transport engineering. In this work, emphasis was placed on a holistic, systems approach that provides for non-linearity in the process as well as the elements required in such a system. Aspects such as portfolio balance, the importance of human resources as well as the assessment of the final impact and benefit were discussed. This article discusses the development of a systems-based R&D management model based on these principles.
The R&D management model was then used to evaluate key performance indicators for the UKaid-funded Research for Community Access Partnership (ReCAP) programme that addresses research into rural low volume roads in Africa and Asia. The model was used to assess the balance of the set of indicators and propose an augmented set. ReCAP (Rust, Strydom, and Hine Citation2016) is a programme of applied research funded by the UK Department for International Development (DFID) at the level of £26.2m over a six-year period. It focuses on low volume roads and rural transport services currently covering twelve countries in Africa and five in Asia. Important aspects in the programme are research uptake, integration of capacity building into research projects, regional and international partnerships and future sustainability. The programme has a well-developed management system with defined indicators as part of a logic framework to assess the success of the investment. However, it was agreed that these could be improved and augmented by analyzing the programme and its objectives in a systemic manner.
Background
Definition of R&D and innovation
Innovation is defined by Roberts (Citation1988) as follows:
Innovation is composed of two parts: (1) the generation of an idea or invention, and (2) the conversion of that invention into a business or other useful application. Or in simple terms:
One can also view this as follows:
As such, managing the R&D process is therefore a subset of managing the innovation value chain. In an extensive review of innovation management in the IT sector, Edison, Ali, and Torkar (Citation2013) found more than 40 definitions of innovation. They state that one of the most appropriate definitions for innovation in the IT sector (that often delivers ‘soft’ solutions) is:
Innovation is: production or adoption, assimilation, and exploitation of a value-added novelty in economic and social spheres; renewal and enlargement of products, services, and markets; development of new methods of production; and establishment of new management systems. It is both a process and an outcome.
This definition is broad and encompasses many of the aspects of an R&D and innovation programme in road and transport engineering. Strategic innovation involves the ‘continuous and dynamic strategic creation of new products, services and business models. (Kodama and Shibata Citation2014)
The complexity of managing engineering methodology research
Complexity theory and complex systems modelling have been used in fields such as management science, astronomy, chemistry, evolutionary biology, geology, and meteorology (Rosenhead and Mingers Citation2001), but complex systems are not necessarily complicated. Cilliers (Citation1998) states that we live in a complex world, and that we need to manage such complexity to prosper. Analyzing complex systems and challenges only from fixed scientific points of reference does not solve the challenge of complexity. Processes emanating from this approach will show linearity that cannot address the dynamic nature of the system. Innovation processes inherently displays non-linear dynamics which renders them complex systems and thus complexity theory is useful to explain their nature (Adriani Citation2011; Wagner-Luptacik, Heller-Schuh, and Leitner Citation2006). In linear processes with only forward-thinking activities much reliance is placed on incremental innovation. They also utilize hierarchical managerial practices with an ‘inward departmental orientation’ (Dekkers Citation2007).
Public sector R&D, such as the ReCAP programme, is particularly complex due to the number of stakeholders (government, citizens, private sector), arising at various geographical levels (local, national, regional), and interfaces across public domains (Bloch and Bugge Citation2013; Spieth and Lerch Citation2014). In addition, the process of integrating public research institutes into the system of innovation is complex, but these institutes play an important role, especially in developing countries where private sector companies are usually relatively small and therefore cannot fund large R&D projects (Chen and Chen Citation2016). There is a particular lack of adequate theory as well as typologies for how public sector innovation occurs (Arundel and Huber Citation2013). The source of public innovation (mostly process innovations) is mostly from the leadership group i.e. middle and upper middle management (Arundel and Huber Citation2013). Although ‘service innovation’ in the public sector is mostly administration procedures (Djellal, Gallouj, and Miles Citation2013), road engineering, which is also mainly public sector, focuses on technical innovations such as new materials, design methods, prediction models etc., which makes it an interesting case.
Several challenges were experienced with historical public sector research programmes in roads where linear, forward-thinking processes were followed (Rust, McCutcheon, and Coetzee Citation2008). These challenges included inter alia fragmentation of the research programme (also confirmed by Dimitratos and Plakoyiannaki Citation2003), leading to small projects with a corresponding decrease in outputs; and subsequently less mentoring of young researchers; The consequence was low performance of such research programmes. The fragmentation of the South African roads and transport research programme has had a very detrimental effect (Rust, McCutcheon, and Coetzee Citation2008). There is therefore a significant need for an improved research and innovation management approach. A new approach must take into account the complexity of the research and development process in this field.
Open innovation
The sharing of knowledge through Open Innovation (OI) can accelerate internal R&D processes and innovation (Chesbrough Citation2003; Bessant, Moeslein, and Kunne Citation2012). This is particularly true if the research resources are geographically widely distributed. It therefore lends itself to a programme such as ReCAP which utilizes researchers from a number of countries, although OI processes still have a number of knowledge gaps (West and Bogers Citation2014). OI requires inter-organizational networks; organizational structures; evaluation processes and knowledge management systems to be successful (Huizingh Citation2011; Chiaroni, Chiesa, and Frattini Citation2010).
It has been shown that innovation in services must be open to be effective (Storey et al. Citation2015). R&D and Innovation in the roads and transport sector often results in knowledge-based solutions intended for general use in government as well as by the engineering fraternity and, as such, could benefit from Open Innovation. Storey et al. (2015) list service quality and proficient operations and delivery systems as antecedents of service innovation performance. A systems approach also allows for interaction with stakeholders outside the system. The concept of open innovation can be seen as one such process.
A holistic, systems approach
Systems thinking has been used since the 1940s to address the failure of mechanistic thinking to explain certain biological phenomena (Flood and Jackson Citation1991). Organisms should be viewed as entities or systems that have unique ‘emergent’ properties that could not be derived from their parts. They are ‘open’ rather than ‘closed’ to their environment. A system thus consists of linked elements with interactive relationships between them. A boundary can be drawn around a group of richly interactive elements that separates them from their environment. The operation of a system is influenced by its inputs, outputs and the feedback loops that influence its operation. Thus the whole is usually more than the sum of the parts (Flood and Jackson Citation1991).
A holistic approach to R&D management rather than a sequential approach, involving all the stages of the development of a product (i.e. research, development, engineering design, and manufacturing), should be followed (Roussel, Saad, and Erickson Citation1991). This allows for cross functional activity cooperation from various departments in a company leading to a balanced R&D portfolio. The use of concepts such as complex adaptive systems that allows for high levels of cooperation has a direct influence on innovativeness (Akgün et al. Citation2014).
A multidisciplinary approach that integrates different competencies
Innovation in longer-term capital investment projects, such as transport infrastructure, is different in nature from that of the manufacturing sector. Capital projects are usually demand-driven, and consist of project-based activities rather than batch production or mass manufacturing systems that produce goods for the consumer market. (Gann Citation1997). R&D in transport and roads is multidisciplinary and therefore it is important to integrate different technologies and knowledge platforms to achieve optimum productivity (Pretorius and de Wet Citation2000). Firms in engineering and construction compete in dynamic environments where they need to manage technological innovation and uncertainty across organizational boundaries with networks of interdependent suppliers, customers, and regulatory bodies. The knowledge of providing capital infrastructure is distributed throughout these networks, and therefore innovation can only take place in an environment where effective communication can take place (Pretorius and de Wet Citation2000).
In technology road mapping (Phaal, Farrukh, and Probert Citation2001) integration needs to take place between the competencies of technical development and commercial activity (Loyarte et al. Citation2015). ‘Technology blocks’ can be used to build ‘technology families’ that in turn develop a ‘specialisation direction’ (Loyarte et al. Citation2015).
Effectiveness measurement of R&D
The purpose of measuring R&D performance is mainly to enhance R&D investments, as well as to improve internal and external communication thus motivating personnel. Improved communication also leads to enhanced coordination with improved learning and a reduction in project risk and uncertainty. Most importantly, it also assists in the motivation for current and future R&D expenditure (Chiesa et al. Citation2009). The outputs and results of innovation are directly dependent on the quality of the R&D management process across the full innovation value chain. Measurement of R&D programme performance and impact is difficult. Most work conducted to date deal with innovation related to the development of hard products such as that reported in the OSLO manual (OECD Citation2005).
Public sector research, in particular, is in need of an adequate system for measuring public innovation (Bloch and Bugge Citation2013). Public sector research and innovation are characterized like services innovation in that the results are often intangible, production of the solution is simultaneous to its use, and the solution is often customized for the user (Bloch and Bugge Citation2013).
Research performance measurement is challenging and indicators for this purpose vary based on the research organization and the discipline in which it conducts research, as well as the source of the funding. For example, research effectiveness measurement in manufacturing usually relates purely to financial aspects such as return on investment and cost avoidance (Germeraad Citation2003). Activities such as external reviews and thorough analysis of the R&D portfolio are also important (Sohn, Yong Gyu, and Hong Kyu Citation2007).
The measurement of R&D effectiveness can be divided into five categories (Da Costa et al. Citation2014):
Stakeholder value and benefits;
Program objectives and requirements;
Results from product (e.g. quality and performance);
Results from process (R&D management, facilities and equipment etc.), and
People (culture, climate competence).
The usual measures for the manufacturing industry such as certified processes, income generation and growth, are however, not suitable for public sector R&D (Da Costa et al. Citation2014). This is particularly true when the R&D programme is aimed at research into technologies for state owned infrastructure such as roads where stakeholder value and benefit as well as people aspects are important (Bhasin Citation2008; Da Costa et al. Citation2014). When these measures are linked to individual researcher performance, it needs to be done with the understanding of the researchers of the strategic goals of the organization and linkage to the R&D process to prevent a negative impact (Speklé and Verbeeten Citation2014).
Balanced Score Card approach
A Balanced Score Card (BSC) is a performance measurement system for implementing strategy and basically consists of a set of performance measures (broader than mere financial measures) that are cascaded down from the corporate level to the business unit level and finally the employee level (Bremser and Barsky Citation2004; Kaplan and Norton Citation2001). Using the indicators of a BSC at researcher level should be exercised with care though due to the uncertainty associated with research and potential negative effect on researcher motivation (Pogrebnyakov, Kristensen, and Gammelgaard Citation2017). The benefits of using this approach include the following – of which most are relevant to ReCAP (Bremser and Barsky Citation2004):
The BSC utilizes causal sets of performance measures to monitor results. Variance analysis of metrics provides insight into deviation from objectives.
The primary purpose of the BSC is to highlight strategy and its impact on operating decisions. Utilizing the BSC over multiple periods provides the basis for feedback and planning.
The BSC provides a common framework and reference point for employees and/or stakeholders across levels and functions as well as top-down alignment.
The requirement to use causal linkages throughout the BSC forces employees to analyze where performance deviates from targets.
BSC objectives provide decision support including the evaluation of alternatives.
The BSC requires frequent monitoring and routine feedback of operating measures to employees across organization levels thus providing motivation for employee actions.
Technology is very important, but its impact is not easily measured by traditional financial metrics (Bremser and Barsky Citation2004; Coombs and Bierly Citation2006). The Balanced Score Card approach can be very useful in linking financial and non-financial measures to strategy and associated key performance measures and allows the integration of metrics that combine several types of quantitative and qualitative measures.
Stakeholders and technology transfer
Technology transfer is vital to ensure uptake and eventual impact. One of the technology transfer models discussed in literature is the well-known S-curve which relates the performance of a technology to its rate of diffusion in the industry and market (Betz Citation1996; Nieto, Lopez, and Cruz Citation1998). A keen interest in the stakeholder group in innovation projects is vital for successful technology transfer. This implies a requirement for a sound knowledge base in the stakeholder group. Incentives for technology transfer are equally important (Barbolla and Corredera Citation2006; Miller et al. Citation2016). Open innovation can be stimulated by sound networks with customers, as well as with innovation project collaborators and competitors (Bessant and Phillips Citation2013). This requires highly skilled research and innovation managers (Cetindamar, Phaal, and Probert Citation2016).
Rapid responses to customer feedback play a significant role in successful technology transfer especially during the early stage or ‘fuzzy end’ of the innovation value chain and in this stage open innovation can reduce significantly the R&D risk (Consoli and Elche Citation2014; Robbins and O’Gorman Citation2015; Thanasopon, Papadopoulos, and Vidgen Citation2016).
Summary
The literature review has indicated a number of important aspects that should be considered in determining the critical characteristics of an effective R&D management system. These include:
Public sector research into areas such as road engineering is complex due to the number of stakeholders and variables involved, and therefore simple linear management processes are not suitable;
In a research programme such as ReCAP, the research resources are dispersed amongst a number of participating organizations and therefore the principles of open innovation pertaining to information sharing, shared learning and knowledge management are important for the success of the programme;
Impact or benefit from public sector research is difficult to attribute to the inputs therefore it is prudent to follow a Balanced Score Card approach with indicators that are monitored over time with the associated trend analysis;
The human resource or knowledge pool plays an important role in this type of research, and
Technology transfer to stakeholders is vital for the programme to achieve impact.
R&D and innovation management models
As mentioned, many R&D and innovation management models in the current literature are very linear in nature. A case in point is the first generation ‘Department Stage Model’ discussed by Saren (Citation1984) as shown in .
This early model (described as a first stage model by Rothwell and Zegveld Citation1985) is extremely linear with no feedback about the performance of each of the stages and leads to a number of problems, particularly that of transferring the knowledge and know-how from one department to the other.
A second stage ‘Market Pull Model’ is described by Twiss (Citation1980) as depicted in . Although this model places more emphasis on understanding of the market as part of innovation, it is still a linear approach with limited feedback.
An example of a third stage model is the Coupling Model (Twiss Citation1980) as depicted in . This model starts to incorporate some feedback loops but still has a notion of linearity from Idea to Market.
Although Twiss's Activity Stage Model is an example of an integrated model that links the knowledge of market needs to the R&D process, as depicted in , it still has a very linear trend. Some systems thinking aspects such as interaction with the external environment are included.
However, none of the above models are non-linear, or systems based. In addition, it was found that, in 73 case studies in typical text books, they deal mostly with the development of hard products for the consumer market (72%) with 9 dealing with software development and 10 with services (Rust Citation2015). These models are also often dependent on the company structure and the process of passing inventions from the R&D department to marketing and sales can be a challenge. None addressed the development of new methodologies for the road and transport sector and the uptake and embedment of the new methodologies into practice.
Objectives and research methodology
As indicated above, a significant portion of research in the road and transport sectors is focused on engineering methodology intended for use by engineering professionals, rather than on developing hard products for consumer markets. The main purpose of the study was to develop a systems-based model for R&D management in this sector, taking its particular characteristics into account.
Research questions
For this research study, the following research questions were posed:
What principles should be used to develop an improved R&D management process in the road engineering sector?
What are the potential benefits of using a systems-based approach?
Can a systems-based research management model be applied to improve the key performance indicators for a road research programme?
Research approach
The approach followed for this study was reported in detail by Rust (Citation2015). It entailed mainly qualitative research with some quantitative analysis of survey results.
The data and information for this study were collected from (Rust Citation2015):
workshops on R&D management and selected interviews with road and transport sector professionals;
the analysis of nine past R&D and innovation programmes and projects through case studies, and
a survey to determine the use of R&D and innovation management processes among research managers.
The survey included the following aspects (Rust Citation2015):
‘the linking of the R&D programme and projects to an overall strategic plan;
the differentiation in management processes between longer-term basic R&D projects and shorter-term development projects;
the use of portfolio management tools to balance the research project portfolio;
the use of formal technology transfer projects to ensure the implementation of research results;
the formal assessment of the impact (effectiveness) of R&D programmes and projects;
the use of integrated, systems-based approaches in R&D management, including feedback loops to ensure team learning;
the use of a formal investment decision process to allocate funding to projects;
the use of formal planning of human resource development; and
the use of formal project management processes.’
The extent to which the following tools were used was also evaluated (Rust Citation2015):
‘scenario planning;
technology foresight studies;
stakeholder needs analysis;
technology road mapping;
technology trees; and
causal maps.’
The analysis of the survey results allowed for the determination of the ‘extent of use’ of these aspects of the process, and their perceived significance to the survey respondents.
A five-point Lickert scale was used and the statistical analysis of the results was conducted using SPSS v 16.
Survey results
Target group
Detail of the survey results was given by Rust (Citation2015). Of the 126 organizations targeted for the survey, 42 responded but in three cases both the researchers in a programme and their managers responded thus providing 45 responses. These responses were from South African as well as international organizations across a variety of research fields, however, most of the responses came from the transport, infrastructure, road engineering, and construction fields.
Respondent analysis
The profile of the respondents is given in . It can be noted that 54% of the responses came from within South Africa and the rest from international research organizations. Both R&D managers and general managers responded. There was a balance between educational institutions (40%) and government research laboratories (31%) with only 3% from the private sector. It can also be noted that most of the respondents (63%) operate in the infrastructure field.
Figure 5: Profile of survey respondents (Rust Citation2015).
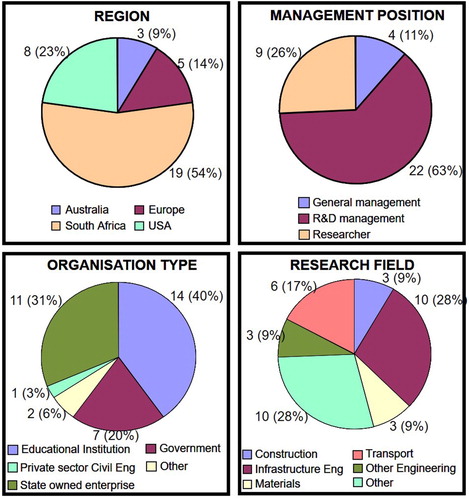
Very few private sector companies conduct R&D into generic ‘soft’ solutions for roads and transport (see above), although they do develop new materials and equipment. These projects are, however, confidential which resulted in a low response from the private sector.
Survey data analysis
A complete statistical analysis of the data has been reported previously (Rust Citation2015). This work found that the following characteristics are used most often in R&D management processes:
‘linkage of R&D programme and projects to a strategic plan;
management of longer-term basic R&D projects vs shorter-term development projects;
the use of a formal investment decision process to allocate funding to projects;
the use of formal planning of human resources development; and
the use of formal project management processes.’
In the gap analysis between importance and extent of use, it was found that there is a significant need for improved portfolio management; improved management of technology transfer projects; impact assessment frameworks and systems; and the use of a systems-based model for research management, indicating the need for a conceptual model and management tools for an improved process.
The detail of the statistical analysis (Rust Citation2015) will not be repeated here. Suffice to indicate that a between-groups analysis of variance (ANOVA) and principal component analyses were conducted. From this analysis four important characteristics of a new research model were identified. These were:
The use of a systems approach;
The importance of the human resource pool;
The importance of strategic planning, including investment decision and portfolio management processes; and
Technology transfer processes.
Principles for a systems-based R&D management model
The analysis of the qualitative data and survey data (detailed analysis reported previously, Rust Citation2015) led to the formulation of a set of tenets or principles for the development of a systems-based R&D management model. These were:
‘A holistic approach should be integral to the R&D management process, taking into consideration the broader environment within which the system operates, the needs of stakeholders and communities and should address the full innovation chain;
The process should be based on a systems approach, which is non-linear, takes cognisance of the interaction between the elements of the system with feedback loops and the fact that small changes can cause large effects elsewhere in the system;
Integration of elements or levels of the process should be used to enhance the value and quality of the outcome from the research process;
The manpower pool should be central to the process;
Strategic planning must be a core element of the process;
The research and development programme must be balanced addressing both short-term and long-term challenges as well as a balance between technology push and market pull;
The concepts of core competencies and platforms should be integral to the model;
The process must stimulate creativity and invention;
The process should counter the effects of fragmentation;
The process should assess the effectiveness of the R&D programme;
Stakeholder interaction and technology transfer are essential, and
The process should allow effective internal and external communication, including the motivation for stable long-term funding.’
These principles were used as input into the development of a systems-based model for R&D management in the road and transport sector. A number of supporting tools were also developed but these will be discussed in a subsequent article.
Systems-based R&D management model
One of the main findings of this study is that R&D should take place in a framework based on a systems approach and taking into account the bigger picture (holistic approach) within the full innovation value chain. The model should be viewed as a system with elements that are linked, interact with each other through feedback loops and interact with their environment to ensure an effective, interactive process.
The model developed in this study is viewed as a system as defined by Flood and Jackson (Citation1991), consisting of main elements which interact with each other and are interdependent. Flood and Jackson state that a system comprises elements which are linked to one another through a series of relationships. If a group of elements are richly interactive a boundary can be drawn around them separating them from their environment. A system also has inputs and outputs with feedback loops that influence the operation of the system and therefore the whole is usually more than the sum of the parts.
Beer (Citation1959) showed a schematic of a dynamic system as being a circular diagram as depicted in . The notion of a circular representation of a management model is valuable in view of the fact that circular causality holds true in a complex system (i.e. the system can impact on itself). Beer (ibid) discussed cybernetic systems that also include a ‘sensor’ that monitors the state of the system and allows for self-regulation and adjustment.
Figure 6: A dynamic system as depicted by Beer (Citation1959).
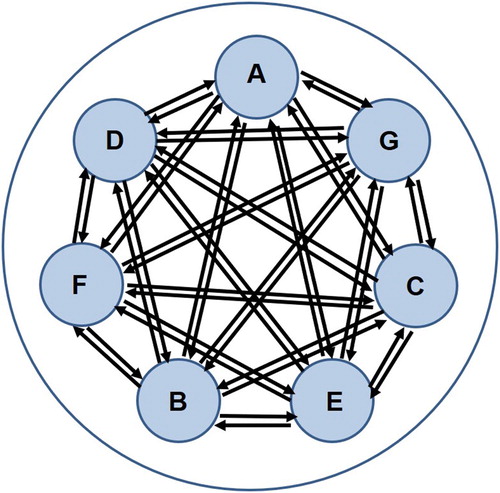
An R&D management model that addresses the full innovation value chain, should address the following elements of R&D such as described by the logic model (West and Bogers Citation2014):
inputs/resources;
activities;
outputs;
outcomes (uptake), and
eventual impact.
Elements of the systems-based management model
Strategy
For R&D programmes in the roads and transport sector, strategic planning that includes technology strategy, is as important as for private sector companies developing consumer products. It is vital that an understanding of the strategic challenges facing the sector as well as international technology trends be understood to inform the selected technology development pathways and roadmaps. Tools such as technology needs determination processes, technology scanning, technology road mapping and foresight studies are often used for this purpose.
Research and development
It is important to understand that research is often not as well directed as development projects. As such, research projects should be managed differently than development projects. Research projects have less defined direction (but directed by strategic objectives) and timelines than development projects in order to allow for the fostering of the creativity required to solve complex problems.
Outputs
This refers to the usual list of publications, patents, technology demonstrators, algorithms, methods, systems etc. that arises from the R&D process.
Outcomes/uptake
This refers to the uptake or use of the outputs by customers and other stakeholders to achieve their own goals. For example, if a new road design system is developed, then the uptake and outcome will be the use of the system by consulting engineers to design roads that are more cost effective, last longer or can carry higher loads. The process of technology transfer to ensure that the receivers of the technology are adequately equipped to use the technology is paramount.
Impact assessment
This is the process of assessing the impact that the uptake and embedment of the new system will have eventually. An example is improved functional performance of roads that leads to lower freight costs or fewer traffic accidents. This process acts as the ‘sensor’ that Beer describes in his cybernetic, complex systems concept. It feeds back information about the effectiveness of the R&D process so that adjustments can be made.
People or knowledge pool
People and the associated knowledge pool are the core of the model and refer to the technical and managerial staff that drive the R&D process both inside and outside the R&D organization. In the case of the ReCAP programme this would include all participants with technical knowledge, not only the ReCAP programme staff. As such, it includes all researchers as well as technical staff in the road authorities receiving the new knowledge. Understanding this element of the system is vital, particularly in an R&D process that aims to generate soft solutions and methodologies such as those required in the road and transport sector. The knowledge, skill and knowhow embedded in the people are critical to the success of the process. An effective R&D process also builds the human capital through aspects such as further qualifications and experience gained, both in R&D as well as in implementation of the outputs of R&D.
Non-linear systems approach
It is important to note that the process flow in the model is non-linear and that many pathways can be followed through the model during the R&D process depending on the nature of the problem addressed. The process flow can also be bidirectional and iterative. For example, if, in the strategy/needs determination phase a problem is identified, the following pathways (depending on the nature of the problem), can be followed:
For a simple problem, the human capital pool can be consulted, and if reasonable knowledge exists it can be repackaged into a specific solution and uptake can be initiated: problem, human capital pool, solution, uptake;
If a significant amount of rework needs to take place the pathway would be: problem, human capital pool, development, solution, uptake;
For more complex problems a research phase may be necessary: problem, human capital pool, research, human capital pool, development, solution, uptake.
In addition, the notion of planning from the future backwards is important (also referred to as back casting). This implies that, during the planning process, the first step should be to envisage the required impact, then the potential uptake (including mechanisms for uptake) and only then the R&D programme and its outputs should be identified. This implies that the envisaged uptake will influence the nature of the solution and therefore the nature of the R&D to be conducted. This is an essential step (similar to market pull) and does not come naturally for researchers that often focus on ‘pet topics’ (technology push). This particularly applies in the ReCAP programme where Terms of Reference development needs to start with the desired impact.
One of the most important elements of this systems approach is the impact evaluation element which acts as a ‘sensor’ in the system and feeds back (to the system) information on its health. The system then corrects based on the input received by influencing the strategy and planning process or the R&D process. It is of vital, importance to plan and design the impact evaluation process prior to the initiation of the programme, so that the correct information and data can be collected during the full R&D process.
All complex systems operate within an environment that influences the system. shows some of the aspects in the environment to be considered.
Some of the critical aspects in are:
Strategy phase: stakeholder and user participation to ensure a holistic definition of problems and needs; the definition of the objectives of the programme in relation to general objectives of the country (e.g. job creation, asset preservation etc.)
R&D phase: The definition of an integrated R&D programme that addresses the strategic objectives, identified needs and that will lead to uptake and problem solving. This should take cognisance of existing skills and strengths in all the participating R&D organizations. An open innovation approach is ideal in this instance to ensure sharing of for example, new design methodologies to accelerate the R&D process. A uniform team approach should be followed to ensure that maximum benefit is derived from the investment. The focus should be on building capabilities and platforms that will address the identified needs, however, some room for creativity should be allowed in the research phase (as opposed to the development phase with stricter briefs and deadlines);
Outputs and outcomes phase: A most critical aspect is to define or develop the delivery mechanisms or impact pathways required to achieve the optimum impact. This implies for example an analysis of stakeholder organizations as well as legislation and policy that can facilitate uptake (or hinder uptake for that matter);
During the uptake phase partnering with industry and government departments is vital to ensure the successful piloting of new solutions or knowledge as well as the mainstreaming of such solutions.
The impact evaluation phase should be grounded in the body of knowledge on M&E (monitoring and evaluation) practice and policy; as well as taking cognisance of aspects such as indicator definition and trend analysis. Of particular importance is to find ways of assessing the impact of soft solutions such as design methodologies as well as to address social impact such as job creation, and lives saved due to a reduction in traffic accidents.
Supporting tools
The model discussed above is a strategic level management model that should be supported by decision tools such as:
Technology foresight studies can be used to define the future technology landscapes that will influence the R&D programme;
Technology road mapping is a powerful tool to develop a time-based road map for developing solutions and technologies that are linked to the current as well as envisaged future needs and challenges in the industry;
Technology trees can be used to ensure that capabilities are integrated into useful platforms from which solutions can be delivered and that there is balance in the portfolio of R&D projects;
Technology diffusion theory and models can be used to ensure effective technology transfer into the industry;
Investment decision processes utilizing aspects such as the level of need in a specific area and the ‘return on investment’ can be used to prioritize projects;
An impact assessment system can be used to assess the uptake and impact of the solutions in order to, firstly inform future strategy and, secondly as part of the investment decision and motivation for future funding.
It is vital that a comprehensive R&D strategy with specific strategic focus areas be developed from which the required projects that fit together in a holistic portfolio can be identified. This is as opposed to a list of projects emanating from a list of needs identified by individuals. At the same time the strategic ‘top down’ approach can be strengthened by ‘bottom up’ input on challenges and problems experienced in practice. However, these inputs must be considered within the strategic portfolio of focus areas.
Application of the model in the development of the ReCAP benefit assessment system
Using the systems-based model to define augmented key performance indicators for ReCAP
A study funded by the ReCAP programme was conducted to assess the best way to address impact assessment of the programme that is broader than just Return on Investment calculations. This study (Rust, Strydom, and Hine Citation2016) aimed to utilize existing information on benefit assessment of research and through interactive discussions with managers and officials of the ReCAP programme determine how to update their existing set of performance measures to take cognizance of, particularly, the social and environmental benefits of the research programme. The analysis of existing knowledge on the subject, as well as the systems model described above were used as a basis to define gaps in their current system and to define new performance measures. The final set of performance measures are superimposed on the systems model in .
Figure 9: Full set of research performance measures superimposed on the systems model (bulleted indicators were existing; numbered indicators are new).
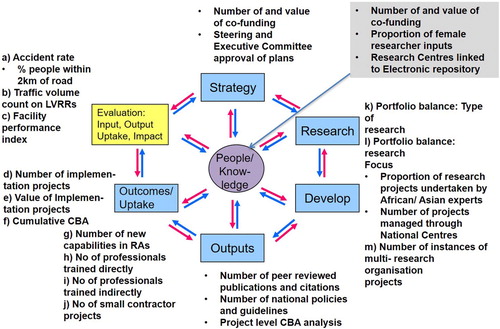
As can be seen, the performance measures also correlate well with the well-known logic model (Reed and Jordan Citation2007; WK Kellog Citation2004). The logic model depicts the research process as input, process, output, outcome (uptake) and impact. The basic work for ReCAP will be used in the future to develop an online, live system for recording and analyzing progress of the programme in line with the performance indicators shown above.
Discussion of augmented performance indicators
The new indicators added to the ReCAP benefit assessment process in order to achieve a better-balanced score card are discussed below.
a) Accident rate per km per 100,000 people
It is intended that this indicator could show the effect on traffic safety of an intervention. However, it would require some narrative to go with the measurement to indicate the specific contribution of the programme to traffic safety which can be influenced by a combination of a number of factors. Although this indicator is probably best addressed at the network level, before and after studies can also be conducted at the road section level to investigate the effectiveness of a specific intervention (such as the application of a hazardous sites management system for rural roads).
b) Traffic volume count on low volume rural roads
If benchmarked against a before status, this indicator could be useful to determine the effectiveness of a road(s) as a facility(ies). Improvements on the facility should mean that the road will attract more traffic.
c) Facility performance index (how the solution improves the performance of the facility)
The idea is that a facility performance index be developed to describe the overall performance of a facility such as a link in the road network. This should ideally be a composite index that combines structural performance (e.g. roughness, deformation) and functional performance (such as skid resistance of the surfacing). However, some R&D would be required to develop such an index. Monitoring of this index over time will however give a good picture of the improvement of the overall performance of the road network where ReCAP research solutions have been applied.
d) Number of implementation projects where ReCAP research has been applied
This is a simple count of the number of implementation projects to indicate the breadth of implementation from the programme.
e) Total monetary value of implementation projects where ReCAP research has been applied
This indicator is recorded at the project level and then aggregated at the other levels to indicate the size of the uptake taking place. Monitored over time, this indicator can provide information on the cumulative effectiveness of the programme or the individual themes.
f) Cumulative benefit-cost ratio for projects assessed
Benefit-cost ratios can be calculated at project level to give an indication of the direct financial benefit of interventions. This should however, also be tracked over time to assess the cumulative monetary benefit of the programme or theme.
g) Number of new or enhanced capabilities created in road authorities
The road authorities are important users of new solutions created, particularly when it pertains to the endorsement of new design methods and the use of decision support systems. In this regard effectiveness at road authority level will have a significant impact on the performance of the whole road and transport system. Often new solutions can only be used effectively within road authorities if the right capability and capacity is created to allow for its effective use. A capability is defined as the ability of the authority to carry out a specific task. This may include well-trained personnel as well as tools such as decision support systems and data bases.
h) Number of professionals directly exposed to ReCAP research findings through training and workshop interventions
As indicated in , human capital is essential to and central to the success of any R&D programme. This includes human resources in government and industry that have to use the solutions and knowledge developed. In addition to the indicators already in the ReCAP logframe, it is therefore suggested that the number of professionals exposed to training in new ReCAP programme solutions be monitored.
i) Number of professionals exposed to ReCAP through indirect training
This indicator describes the situation where trainers have been trained and then, in turn, train professionals. It also incorporates the situation where ReCAP outputs have been included in the curricula of universities and training institutions and then used to train students.
j) Number of small contractor and consultant capacity development projects/company processes improved from ReCAP outputs
Small local/national contractor and consultant development is one way of meaningful job creation in the transport sector. This is a count of the number of such instances.
k) Portfolio balance: Type of research (basic, applied, experimental development, technology transfer)
The R&D portfolio of projects should be based on the strategy of the programme. One should therefore monitor the balance of the portfolio of projects to ensure that it addresses the strategic objectives. For ReCAP, it will be important to monitor the balance between research, solution development and technology transfer/implementation. To this end, the well-known classification of projects according to the Fascati manual (OECD Citation1994) can be used (basic research, applied research and experimental development) to which can be added implementation projects as a fourth category.
l) Portfolio balance: Research focus (materials, design, construction, management systems, environmental impact, transport services)
Another balance factor that should be considered is the focus of the research based on elements of the road infrastructure or transport services value chain. To this end categories such as materials, design, construction, maintenance, management systems etc. can be considered. The ratio between these efforts can be adjusted as the strategic focus of the programme shifts. It may also vary from country to country or region to region.
m) Number of instances of multi-country, multi-research organization or multi-agency funded projects
This measure indicates the level of co-operation and partnering internally within the programme and is vital in an open innovation environment where information should flow freely between participants. It also indicates whether research organizations use each other's strengths (core capabilities) effectively.
Contribution to state of the art and implications for practice
This paper contributes to the knowledge pool of research management by offering a set of principles for a holistic, systems approach to research management as opposed to a linear, forward thinking only approach. A new systems-based model is offered and it is demonstrated how the model can be used to improve research management and in particular, the assessment of the impact of research.
From this study, a number of aspects were identified that should be considered by R&D managers. A culture of systems thinking should be instilled in researchers so that they can understand and indeed think about the many pathways to eventual impact. Researchers should also be encouraged to do back casting from an envisioned future impact when planning their projects so that they can visualize the eventual impact, the uptake mechanism, and nature of the users which will influence the nature of the output and therefore the research approach and process. Research managers should understand the role of research effectiveness monitoring and impact assessment. In this regard they should develop an appropriate system and initiate the collection of relevant input data across the full innovation value chain from the onset. Retrospective studies are often used to determine research effectiveness but to find the appropriate data sometimes years after the research had been conducted can be challenging. External champions in the receiving organization to which technology is transferred can play a vital role in understanding and analyzing the effectiveness and impact of the research. A balanced score card approach can be a useful instrument to develop a portfolio of key performance measures that will help to assess the effectiveness of the research investment.
Future work will focus on the development of decision management tools such as a needs determination process, and technology trees to support the strategic management model.
Disclosure statement
No potential conflict of interest was reported by the authors.
Additional information
Funding
References
- Adriani, P. 2011. “Complexity and Innovation.” In The Sage Handbook of Complexity and Management, edited by P. Allen, S. Maguire, and B. McKelvey, 454–470. Los Angeles: SAGE.
- Akgün, A. E., H. Keskin, J. C. Byrne, and O. O. Ilhan. 2014. “Complex Adaptive System Mechanisms, Adaptive Management Practices, and Firm Product Innovativeness.” R&D Management 44 (1): 18–41.
- Arasa, Gilbert M. 2012. Presentation by Eng Gilbert M Arasa, Registrar of the Engineers Board of Kenya, April 22.
- Arundel, Anthony, and Dorothea Huber. 2013. “From too Little to too Much Innovation? Issues in Measuring Innovation in the Public Sector.” Structural Change and Economic Dynamics 27: 146–159. doi: 10.1016/j.strueco.2013.06.009
- Barbolla, A. M. B., and J. R. C. Corredera. 2006. “Factors for Success in University-Industry Research Contracts.” Proceedings of the Second European Conference on Management of Technology: Doctoral papers, Birmingham, UK, 1–8.
- Beer, S. 1959. “What Has Cybernetics to Do with Operational Research?” Journal of the Operational Research Society 10: 1–21. doi: 10.1057/jors.1959.1
- Bessant, J., K. Moeslein, and C. Kunne. 2012. Opening Up Healthcare Innovation: Innovation Solutions for a 21st Century Healthcare System. AIM Advanced Institute of Management Research, London, for a 21st century healthcare system. AIM Advanced Institute of Management Research, London.
- Bessant, J., and W. Phillips. 2013. “Innovation Management and Dynamic Capability.” In The Sage Handbook of Strategic Supply Management: Relationships, Chains, Networks and Sectors, edited by C. Harland, G. Nassimbeni, and E. Schneller. Sage.
- Bessant, J., B. Ramalingam, H. Rush, N. Marshall, K. Hoffman, and B. Gray. 2014. Innovation Management, Innovation Ecosystems and Humanitarian Innovation. Project report published by UK Department of International Development.
- Betz, F. 1996. “Forecasting and Planning Technology.” In Handbook of Technology Management, edited by G. H. Gaynor, 12.2. New York: McGraw-Hill.
- Bhasin, S. 2008. “Lean and Performance Measurement.” Journal of Manufacturing Technology Management 19 (5): 670–684. doi: 10.1108/17410380810877311
- Bloch, C., and M. M. Bugge. 2013. “Public Sector Innovation—From Theory to Measurement.” Structural Change and Economic Dynamics 27: 133–145. doi: 10.1016/j.strueco.2013.06.008
- Bremser, W. G., and N. P. Barsky. 2004. “Utilizing the Balanced Scorecard for R&D Performance Measurement.” R&D Management 34 (3): 229–238.
- Cainelli, G., R. Evangelista, and M. Savona. 2010. “The Impact of Innovation on Economic Performance in Services.” The Service Industries Journal 224: 52–59.
- Cetindamar, Dilek, Robert Phaal, and David R. Probert. 2016. “Technology Management as a Profession and the Challenges Ahead.” Journal of Engineering and Technology Management 41: 1–13. doi: 10.1016/j.jengtecman.2016.05.001
- Chen, Jian-Hung, and Yijen Chen. 2016. “The Evolution of Public Industry R&D Institute – The Case of ITRI.” R&D Management 46 (1): 49–61.
- Chesbrough, H. 2003. Open Innovation: The New Imperative for Creating and Profiting from Technology. Boston, MA: Harvard Business School Press.
- Chiaroni, D., V. Chiesa, and F. Frattini. 2010. “Unravelling the Process from Closed to Open Innovation: Evidence from Mature, Asset-Intensive Industries.” R&D Management 40 (3): 222–245.
- Chiesa, V., F. Frattini, V. Lazzarotti, and R. Manzini. 2009. “Performance Measurement of Research and Development Activities.” European Journal of Innovation Management 12 (1): 25–61. doi: 10.1108/14601060910928166
- Cilliers, P. 1998. Complexity and Postmodernism. London: Routledge Publishers.
- Cloete, Nico. 2014. “The South African Higher Education System: Performance and Policy.” Studies in Higher Education 39 (8): 1355–1368. doi: 10.1080/03075079.2014.949533
- Consoli, Davide, and Dioni Elche. 2014. “An Analysis of the Knowledge Base of Scientific Research and Development Business Services.” R&D Management 44 (4): 341–354.
- Coombs, J. E., and P. E. Bierly. 2006. “Measuring Technological Capability and Performance.” R&D Management 36 (4): 421–438.
- Da Costa, Janaina Mascarenhas Hornos, Josef Oehmen, Eric Rebentisch, and Deborah Nightingale. 2014. “Toward a Better Comprehension of Lean Metrics for Research and Product Development Management.” R&D Management 44 (4): 370–383.
- Dekkers, R. 2007. “Did the Paradigm Shift in Innovation Management Occur?” Proceedings of the 16th International Association of Management of Technology Conference (IAMOT), 1749–1768.
- Dimitratos, P., and E. Plakoyiannaki. 2003. “Theoretical Foundations of an International Entrepreneurial Culture.” Journal of International Entrepreneurship 1: 187–215. doi: 10.1023/A:1023804318244
- Djellal, Faridah, Faïz Gallouj, and Ian Miles. 2013. “Two Decades of Research on Innovation in Services: Which Place for Public Services?” Structural Change and Economic Dynamics 27: 98–117. doi: 10.1016/j.strueco.2013.06.005
- Edison, Henry, Nauman bin Ali, and Richard Torkar. 2013. “Towards Innovation Measurement in the Software Industry.” Journal of Systems and Software 86: 1390–1407. doi: 10.1016/j.jss.2013.01.013
- Flood, R. L., and M. C. Jackson. 1991. Creative Problem Solving. London: John Wiley and Sons.
- Gann, D. 1997. “Technology Policy: An International Comparison of Innovation in Major Capital Projects.” Paper presented at the 1st International Conference on Technology Policy and Innovation, Macau.
- Germeraad, P. 2003. “Measuring R&D in 2003.” Research-Technology Management 46 (6): 47–56. doi: 10.1080/08956308.2003.11671597
- Huizingh, E. K. R. E. 2011. “Open Innovation: State of the Art and Future Perspectives.” Technovation 31: 2–9. doi: 10.1016/j.technovation.2010.10.002
- Kaplan, R. S., and D. P. Norton. 2001. The Strategy Focused Organization. Boston, MA: Harvard Business School Press.
- Kodama, Mitsuru, and Tomoatsu Shibata. 2014. “Strategy Transformation Through Strategic Innovation Capability – A Case Study of Fanuc.” R&D Management 44 (1): 75–103.
- Loyarte, Edurne, Jorge Posada, Seán Gaines, Shabs Rajasekharan, Igor G. Olaizola, Oihana Otaegui, María Teresa Linaza, et al. 2015. “Technology Roadmapping (TRM) and Strategic Alignment for an Applied Research Centre: A Case Study with Methodological Contributions.” R&D Management 45 (5): 474–486.
- Martínez-Román, Juan, Juan A. Tamayo, and Javier Gamero. 2017. “Innovativeness and Its Influence on Growth and Market Extension in Construction Firms in the Andalusian Region.” Journal of Engineering and Technology Management 43: 19–33. doi: 10.1016/j.jengtecman.2016.11.002
- Miller, Kristel, Rodney McAdam, Sandra Moffett, Allen Alexander, and Pushyarag Puthusserry. 2016. “Knowledge Transfer in University Quadruple Helix Ecosystems: An Absorptive Capacity Perspective.” R&D Management 46 (2): 383–399.
- National Survey of Research & Experimental Development. 2016. Human Science Research Council, Pretoria, South Africa.
- Nieto, M., F. Lopez, and F. Cruz. 1998. “Performance Analysis of Technology Using the S Curve Model: The Case of Digital Signal Processing (DSP) Technologies.” Technovation 18 (6/7): 439–457. doi: 10.1016/S0166-4972(98)00021-2
- Odango, Michael M. 2017. “Why Engineers Are Still in Short Supply in Uganda.” Article in Newsvision, March 28.
- OECD. 1994. The Measurement of Scientific and Technical Activities, Proposed Standard Practice for Surveys of Research and Experimental Development (“Frascati Manual”). Paris: Organization for Economic Co-operation and Development.
- OECD. 2005. OSLO Manual: Guidelines for Interpreting and Collecting Innovation Data. 3rd ed. Paris: Organization for Economic Co-operation and Development.
- Phaal, R., C. J. P. Farrukh, and D. R. Probert. 2001. “Characterisation of Technology Roadmaps: Purpose and Format.” Proceedings of Portland International Conference on Management of Engineering and Technology (PICMET) (1): 367–374.
- Pistorius, C. 1996. Technology as the Engine of Growth. Research for a Competitive Industry, Scientech ‘96, Annual magazine of the Foundation for Research Development, p.2.
- Pogrebnyakov, Nicolai, Jonas D. Kristensen, and Jens Gammelgaard. 2017. “If You Come, Will They Build It? The Impact of the Design and use of a Performance Management System on Researcher Motivation.” Journal of Engineering and Technology Management 43: 67–82. doi: 10.1016/j.jengtecman.2017.01.004
- Porter, M. E. 1990. “The Competitive Advantage of Nations.” Harvard Business Review, March – April, 2 (3): 73–93.
- Pretorius, M. W., and G. de Wet. 2000. “A Model for the Assessment of New Technology for the Manufacturing Enterprise.” Technovation 20: 3–10. doi: 10.1016/S0166-4972(99)00092-9
- Reed, J. H., and G. Jordan. 2007. “Using Systems Theory and Logic Models to Define Integrated Outcomes and Performance Measures in Multi-Program Settings.” Research Evaluation 16 (3): 169–181. doi: 10.3152/095820207X243909
- Robbins, Peter, and Colm O’Gorman. 2015. “Innovating the Innovation Process: An Organisational Experiment in Global Pharma Pursuing Radical Innovation.” R&D Management 45 (1): 76–93.
- Roberts, E. B. 1988. “What We've Learned: Managing Invention and Innovation.” Research-Technology Management 31 (1): 11–29. doi: 10.1080/08956308.1988.11670497
- Rosenhead, J., and J. Mingers. 2001. Rational Analysis for a Problematic World Revisited: Problem Structuring Methods for Complexity, Uncertainty and Conflict. 2nd ed. Hoboken: Wiley.
- Rothwell, R., and W. Zegveld. 1985. Reindustrialisation and Technology. Harlow, UK: Longman.
- Roussel, P. A., K. N. Saad, and T. J. Erickson. 1991. Third Generation R&D. Boston, MA: Harvard Business School Press.
- Rust, F. C. 2010. “Critical Requirements for a Systems-Based R&D Management Process.” CSIR Biennial Conference: ‘Science real and relevant’, Pretoria.
- Rust, F. C. 2015. “Requirements for a Systems-Based Research and Development Management Process in Transport Infrastructure Engineering.” The South African Journal of Industrial Engineering 26 (1): 86–101. doi: 10.7166/26-1-904
- Rust, F. C., R. T. McCutcheon, and L. Coetzee. 2008. “Transport Research: Quo Vadis?” Proceedings of the South African Annual Transport Conference, Pretoria.
- Rust, F. C., J. Strydom, and J. Hine. 2016. ReCAP Benefit Assessment System (ReCAP-BAS): Scoping document. Project report to The Research for Community Access Partnership, London.
- Saren, M. A. 1984. “A Classification and Review of Models of the Intra-Firm Innovation Process.” R&D Management 14 (1): 11–24.
- Sohn, S. Y., J. Yong Gyu, and H. Hong Kyu. 2007. “Structural Equation Model for the Evaluation of National Funding on R&D Project of SMEs in Consideration with MBNQA Criteria.” Evaluation and Program Planning 30 (1): 10–20. doi: 10.1016/j.evalprogplan.2006.10.002
- South Africa‘s National Research and Development Strategy. 2002. South African Department of Science and Technology, Pretoria, South Africa.
- Speklé, Roland F, and Frank H. M. Verbeeten. 2014. “The use of Performance Measurement Systems in the Publicsector: Effects on Performance.” Management Accounting Research 25: 131–146. doi: 10.1016/j.mar.2013.07.004
- Spieth, Patrick, and Martin Lerch. 2014. “Augmenting Innovation Project Portfolio Management Performance: The Mediating Effect of Management Perception and Satisfaction.” R&D Management 44 (5): 498–515.
- Spieth, Patrick, Dirk Schneckenberg, and Joan E. Ricart. 2014. “Business Model Innovation – State of the art and Future Challenges for the Field.” R&D Management 44 (3): 237–247.
- Storey, C., P. Cankurtaran, P. Papastathopoulou, and E. J. Hultink. 2015. “Success Factors for Service Innovation: A Meta-Analysis.” Journal for Product Innovation Management 33 (5): 511–648.
- Thanasopon, Bundit, Thanos Papadopoulos, and Richard Vidgen. 2016. “The Role of Openness in the Fuzzy Front-End of Service Innovation.” Technovation 47: 32–46. doi: 10.1016/j.technovation.2015.11.007
- Twiss, B. 1980. Managing Technological Innovation. 2nd ed. London, UK: Longman.
- Wagner-Luptacik, P., B. Heller-Schuh, and K. H. Leitner. 2006. “The Firm as Innovation System: Applying Complexity Principles to Drive New Product Development.” Proceedings of the 15th International Association of Management of Technology Conference (IAMOT), China.
- Wall, K., and F. C. Rust. 2015. “A Rating Tool to Assess the Condition of South African Infrastructure.” The 5th Conference on Smart and Sustainable Built Environments. Pretoria, South Africa.
- West, J., and M. Bogers. 2014. “Leveraging External Sources of Innovation: A Review of Research on Open Innovation.” Journal of Product Innovation Management 31 (4): 814–831. doi: 10.1111/jpim.12125
- WK Kellogg Foundation. 2004. Logic Model Development Guide.