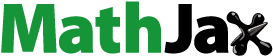
Abstract
The engine rooms of ships accommodate different machinery systems that are critical to ship operations. For these critical machinery systems to continue delivering the high level of service, operators are required to select and apply the best maintenance interventions. Currently, the selection of appropriate maintenance strategies for different engine room’s machinery systems is handled in a traditional way, regardless of in what condition such systems are. The aim of this paper is to propose a semi-qualitative model integrating AHP and PROMETHEE to select appropriate maintenance strategies for different critical machinery found in the engine rooms of ships. The proposed model consists of three maintenance alternatives among which decision-makers must select the best strategy according to four comparison criteria. The model is applied to select the best maintenance strategies for 17 different shipboard machinery systems in the engine room of a barge operating within West African waters. Finally, the results are compared with those obtained from a cost-benefit analysis approach (quantitative) as well as a survey of the experienced marine engineers who operate these machinery in the engine rooms of ships (qualitative). The results of the case study indicate that the proposed semi-qualitative method is a more robust approach for selecting maintenance strategies of shipboard machinery systems.
1. Introduction
The engine rooms accommodate varieties of critical machinery systems which operate uninterruptedly at high pressures and temperatures, making it one of the hostile working environments in ships. Nevertheless, the selection of appropriate maintenance strategies for different engine room machinery in some ships is still handled in the conventional way (i.e. deterministic principles and operational experience). The use of conventional approaches to choose maintenance strategies for critical parts with a high likelihood of failure can lead to catastrophic accident with substantial consequences such as loss of asset, undue delay in transporting cargo from one port to another, customer dissatisfaction as well as exposing ship crew to health and safety risk. For these reasons, it is extremely important to develop a thorough and effective decision-making framework for selecting the best maintenance strategies for different machinery found in the engine rooms of ships.
Selection of the most appropriate maintenance strategies for different engine room machinery will not only prolong the lifetime of ships for sustainable shipping but can also eliminate the consequences associated with the conventional way of maintenance decision-making and planning. However, selection of different maintenance strategies for different engine room machinery is considered as a complex task involving multiple and conflicting evaluation criteria (such as economic, safety, added value and acceptability) (Shafiee Citation2015). Beside involving multiple and conflicting evaluation criteria, it also requires the use of qualitative and quantitative data as well as expert judgment in the case of unavailability of good quality data. Therefore, maintenance strategy selection is considered as a multi-criteria decision-making (MCDM) problem (Nezami and Yildirim Citation2013; Shafiee et al. Citation2019).
Over the past decades, extensive research work using MCDM techniques to select the most suitable maintenance strategy for equipment in different industries have received reasonable attention in the literature. Some recent publications addressing the issue of maintenance strategy selection in different industries are briefly reviewed below.
Focusing on maintenance strategy selection in the textile industry, Shyjith et al. (Citation2008) developed a combined Analytical Hierarchy Process (AHP) and Technique for Order of Preference by Similarity to Ideal Solution (TOPSIS) to select the most suitable maintenance strategy for equipment. Pariazar et al. (Citation2008) proposed a maintenance selection framework based on the AHP approach to determine an optimum maintenance strategy in the textile industry. Ilangkumaran and Kumanan (Citation2009) selected the most appropriate maintenance policy in the textile industry using a hybrid MCDM (AHP and TOPSIS) approach. Ilangkumaran and Kumanan (Citation2012) applied fuzzy AHP and VIKOR to select an optimum maintenance strategy for equipment in the textile industry.
With respect to the oil and gas industry, Bevilacqua and Braglia (Citation2000) employed the AHP approach to select maintenance policy for a refinery in Italy, considering economic factors, applicability, cost and safety as the criteria. Ratnayake and Markeset (Citation2010) proposed a maintenance selection framework based on the AHP technique, considering multiple criteria such as economics, safety, health and environment in selecting maintenance strategy for the Norwegian oil and gas sector. Tan et al. (Citation2011) employed a risk-based methodology and an AHP technique to select the most realistic maintenance strategy for equipment in Fuijian oil refinery ISOMAX unit. The study considered alternatives such as corrective maintenance (CM), time-based maintenance (TBM), shutdown maintenance (SM) and condition-based maintenance (CBM).
In the manufacturing sector, Momeni et al. (Citation2011) applied fuzzy TOPSIS to handle uncertainty in the judgement of decision-makers during maintenance strategy selection for Electerofan Company in the Middle East. Chan and Prakash (Citation2012) selected the most suitable maintenance policy for a manufacturing firm using a fuzzy MCDM technique. The study considered CM, PM, CBM, TPM and Total Quality Maintenance (TQMain) as the alternatives. Sadeghi and Manesh (Citation2012) suggested an integrated Analytical Network Process (ANP) and fuzzy set theory for selecting the best maintenance strategy for transformer equipment in Mobarakeh steel company in Iran. Jayaswal et al. (Citation2013) used a fuzzy TOPSIS method to select the most suitable maintenance strategy from a wide range of alternatives for material handling equipment in India. Kumar et al. (Citation2013) presented a fuzzy simple additive weighting (SAW) approach for the selection of appropriate maintenance strategy for a material handling machine. Nezami and Yildirim (Citation2013) applied fuzzy VIKOR framework to select the most suitable maintenance regime for the manufacturing industry. The framework involved three sustainability indices of social, environmental and economic as the evaluation criteria. Odeyale et al. (Citation2013) applied the AHP technique to select the appropriate maintenance strategy for the manufacturing industry.
For the aviation industry, Ahmadi et al. (Citation2009) applied AHP for selection of appropriate maintenance strategy based on two criteria of availability (benefit) and cost of failure. The proposed methodology was illustrated using the ventilation system of a twin-engine aircraft. In another work, Ahmadi et al. (Citation2010) proposed a hybrid MCDM approach combining AHP, TOPSIS, VIKOR and Benefit Cost Ratio (BCR) to select maintenance strategy for an aircraft system.
Cheng and Tsao (Citation2010) employed the ANP technique to select maintenance strategy for a railway rolling stock.
Pourjavad et al. (Citation2013) combined AHP and TOPSIS techniques for maintenance strategy selection in a Mining Industrial Company.
Aghaee and Fazli (Citation2012) employed ANP and Decision-Making Trial and Evaluation Laboratory (DEMATEL) to select the most suitable maintenance strategy in the automobile industry. The study considered CM, TBM, CBM, predictive maintenance (PdM) and TPM as alternative maintenance strategies.
In the pulp and newspaper industry, Al-najjar and Alsyouf (Citation2003) applied a fuzzy SAW decision-making method to select the most efficient maintenance policy for a paper milling machine. Zaim et al. (Citation2012) employed AHP and ANP to select the most appropriate maintenance strategy for a local newspaper industry in Turkey.
For the shipping industry, Nikitas and Ioannis (Citation2013) proposed a framework for selecting the best maintenance strategy for ship equipment based on a hybrid AHP and TOPSIS. Emovon et al. (Citation2018) proposed an integrated Delphi-AHP methodology and an integrated Delphi-AHP-PROMETHEE methodology to select a maintenance strategy for a marine diesel engine.
In other studies, Bertolini and Bevilacqua (Citation2006) proposed a combined AHP and goal programming (GP) solution for maintenance strategy selection problem. Wang et al. (Citation2007) evaluated different maintenance policies for different equipment using fuzzy AHP in order to minimise uncertainty associated with maintenance decision-making. Bashiri et al. (Citation2011) selected optimum maintenance policy using interactive fuzzy linear assignment method. Jajimoggala et al. (Citation2011) evaluated the suitability of four maintenance strategies using a hybrid fuzzy ANP and GP techniques. Fouladgar et al. (Citation2012) proposed a combined AHP and COPRAS (Complex Proportional Assessment) method to determine the most suitable maintenance policy for heavy duty equipment. Kumar and Maiti (Citation2012) modelled a risk-based maintenance (RBM) policy selection for industrial units using fuzzy ANP.
While reviewing literature in the area of maintenance strategy selection, the hybrid MCDM approach has been applied successfully to choose the best maintenance strategy for equipment in different industries. Nonetheless, only one study was found (see Emovon et al. Citation2018) applying hybrid AHP-PROMETHEE method to select a maintenance strategy for a marine diesel engine. However, the uncertainties associated with decision-making were neglected in that work. Moreover, the study failed to evaluate different maintenance strategies for different machinery systems found in the ship’s engine room. To overcome these drawbacks, this paper proposes a hybrid AHP-PROMETHEE maintenance decision-making model to select the most suitable maintenance strategies for different equipment of the engine rooms of ships. The proposed model considers failure-based maintenance (FBM), time-based maintenance (TBM) and condition-based maintenance (CBM) as the maintenance alternatives from which decision-makers are required to select the appropriate strategy according to four evaluation criteria, including economic, safety, added value and acceptability. The Delphi technique is used to screen the evaluation criteria, AHP is then employed to determine the weights of the evaluation criteria and the relative performance of the alternatives with respect to each evaluation criterion, and finally, the PROMETHEE method is used to rank the maintenance strategies. The model is illustrated using a barge operating within the West African (WA) waters. Thereafter, the results of our analysis are compared with those obtained from a survey of experienced marine engineers (qualitative) as well as a cost-benefit analysis approach (quantitative).
The rest of the paper is organised as follows. In Section 2, maintenance strategy decision-making model is proposed for ship engine room machinery. In Section 3, the proposed model is tested on a case study and the results are discussed. Finally, the work is concluded and recommendations for future work are presented in Section 4
2. Proposed methodology
2.1. Hybrid AHP-PROMETHEE model
In this section, a semi-quantitative analysis approach based on an integrated AHP and PROMETHEE model is proposed to select the most appropriate maintenance strategies for different engine room machinery. As shown in , this approach consists of six steps that are described in details below.
2.1.1. Select the engine room’s machinery system
In this step of the model, the machinery to be considered for investigation must be identified. This information can be obtained from the ship’s asset register or by conducting field visit.
2.1.2. Form a decision-making team
A team of decision-makers is tasked to perform the function of selecting the best maintenance alternative. Since maintenance strategy selection involves multidisciplinary analysis to arrive at the appropriate conclusions, the team of decision-makers can include (but not limited to) designers, maintenance practitioners, shipboard operators, ship owners, academics in the area of maintenance, etc. However, the percentage representation of these experts may depend on arrangements of different shipping companies.
2.1.3. Identify maintenance alternatives
The expertise of the decision-making team which comprise professionals with multidisciplinary skills indicates that such team undeniably can propose a set of suitable maintenance strategies. This same task can also achieved through literature review.
2.1.4. Identify evaluation criteria using the Delphi technique
In order to prioritise the maintenance strategies, there is a need to define a set of evaluation criteria. The identification of evaluation criteria is based on the experience of the decision-makers and literature review. The Delphi technique is used to screen the evaluation criteria to ensure that only the most important criteria are considered when selecting the best maintenance alternative. The first step in the Delphi technique is to identify a facilitator and select decision-makers. This is followed by developing a set of questionnaire (either open ended or closed questions) which is sent to the decision-makers for the first round of Delphi survey. The first round survey is analysed and the results of the survey along with a second questionnaire are sent to the decision-makers for second round Delphi survey. The iteration process continues until a consensus is reached by all the decision-makers. The mean value, standard deviation and the content validity ratio are some of the measures used to remove or retain criteria in order to reach a consensus.
2.1.5. Assignment of criteria weight using AHP
In this step, the AHP procedure is utilised to assign weights to decision-makers. The steps for AHP analysis are briefly described below.
Identify the evaluation criteria
considered for selection of the most appropriate maintenance alternative.
Develop AHP questionnaire for each decision-maker to create a pairwise comparison matrix. The numerical values (i.e. weights) obtained from the pairwise comparison from the decision-maker k are used to form a matrix
,
(1)
(1) where
,
,
, and
represents the k-th expert judgement on how criterion i compares to that of criterion j. The values of the pairwise comparison in the matrix are obtained by using the relative importance scale given in .
The weights of criteria
are calculated using the pairwise comparison matrix
. The weights of each criterion is given by:
(2)
(2) where
denotes the weight of the criterion
. The normalised eigenvector
of matrix
can be used to represent the weights of the criteria as:
(3)
(3) Test consistency and determine the aggregate judgement matrix. If all the fuzzy judgement matrices for decision-makers pass the consistency test, then an aggregate fuzzy judgment matrix can be determined. The aggregate judgement matrix for group decision-making is given by:
(4)
(4) The aij in Equation (4) is obtained from aijk in Equation (1) based on arithmetic mean. The consistency ratio of decision-makers can be calculated using the equation:
(5)
(5) where CI denotes the consistency index and RI is the corresponding average random value of CI for
matrix. The RI values for different matrix orders are shown in .
Table 1. Scale for pairwise comparison (Samarakoon and Ratnayake Citation2015).
Table 2. RI values for different matrix orders (Saaty Citation1980).
The decision-maker’s judgment is considered to be consistent and acceptable when CR < 0.1. If CR > 0.1, then the whole decision-making process must be repeated (Saaty Citation1980). CI is given by the equation:
(6)
(6)
where
represents the maximum eigenvalue and is given by:
(7)
(7)
2.1.6. Apply PROMETHEE II
PROMETHEE was developed by Brans and Vincke (Citation1985), providing a useful MCDM method for outranking a set of finite alternatives and then, selecting the best alternative according to a number of conflicting evaluation criteria. The PROMETHEE method uses positive and negative preference flows for different alternatives, in order to produce ranking in relation to decision weights (Kabir et al. Citation2014).
There are different methods of PROMETHEE described in the literature, but Vinodh and Jeya Girubha (Citation2011) reported that PROMETHEE II is widely applied to rank alternatives because it establishes a complete ranking or pre-order of alternatives. In this study, the PRMOTHEE II which is the most popular and commonly used technique among the PROMETHEE family is used. The basic steps for applying PROMETHEE II are presented below.
Determine deviation based on pairwise comparison
(8)
(8)
where
represents the difference between the evaluation of alternatives
and
on each criterion.
Evaluation of preference function
The preference function is defined as the degree of preference of alternative over alternative
with respect to the
criterion:
(9)
(9)
where
denotes preference of alternative
over alternative
with respect to criterion j, as a function of
. The preference is determined by using preference function formulas, for more on preference function formulas see Nikouei et al. (Citation2017).
Evaluation of global preference index
The aggregated preference index for two alternatives and
is given by:
(10)
(10)
where
of
over alternative
is the weighted sum of
for each criterion
,
is the weight of
criterion and
is the set of available alternatives.
Evaluation of outranking flows
The net outranking flow which is used to measure the performance of each alternative is calculated. The positive outranking flows indicate the level of preference of one alternative over the other.
(11)
(11)
The greater values of
show the strong preference of one alternative in comparison to other alternatives. The negative outranking flow for each alternative is defined as:
(12)
(12)
The net outranking flow is the difference between the positive flow
and the negative flow
, which is calculated as:
(13)
(13)
Therefore, if , then alternative
is better than alternative
.
2.2 Cost-benefit analysis (CBA)
The steps for selecting maintenance strategies for different engine room machinery from CBA perspective are described below:
Identify set of machinery , where
represent the total number of machinery in the engine room.
Define the set of maintenance strategies being considered, , where
denote the number of maintenance alternatives under consideration. In this study, three maintenance strategies are considered, namely; FBM, CBM and TBM.
Estimate the costs associated with each maintenance strategy for each machinery where
. To calculate the cost of FBM,
, the cost information such as the cost of replacement, logistics cost and service loss cost due to downtime are considered. Hence, cost of FBM for each machinery found in the engine room over a period of time is given as:
(14)
(14)
where
is the number of technicians/crew required for replacement;
is the time required to transport onshore maintenance crew;
is the expected replacement time,
is the service loss time (downtime);
is the failure rate of the machinery;
is the manpower cost for replacement and
is the cost of machinery downtime.
Similarly, the cost of TBM over time is calculated as:
(15)
(15)
where
is the time for inspection;
is the time required to repair a machinery;
is the cost for inspections tasks on each machinery and
represent the total cost of logistics in relation to TBM. The cost of logistics include all costs associated with spare parts, maintenance tools and mobilisation/administrative activities and is given as:
(16)
(16)
In the above equation, is the cost of spare parts;
is the cost of acquiring tools and
is the mobilisation/administrative cost.
Finally, the cost of CBM, is calculated using the equation
(17)
(17)
where
corresponds to the cost of acquiring condition monitoring (CM) systems and
and
are the costs of installing CM system and performing preventive maintenance (PM).
For a single machinery the benefit of maintenance to the ship owner is modelled as:
(18)
(18)
where
and
represent the benefit of maintenance to ship owner in terms of increased service time, potential failure repair and improved safety, respectively.
At this point, the decision on the appropriate maintenance strategy is made on the basis of benefit–cost (B/C) ratio. The B/C ratio is obtained by assigning monetary values to both benefits and costs associated with each maintenance strategy considered. The B/C ratio on the investment of each maintenance strategy considered is determined as:
(19)
(19)
where
and
corresponds to the benefits and costs of the maintenance strategies being considered.
The appropriate maintenance strategy is the one with . However, if the B/C ratios for all maintenance alternatives is greater than unity, then the alternative with the highest B/C ratio is selected as the most appropriate alternative. It should be noted that this method is heavily dependent on the monetary value of benefits and costs data and in the absence such data uncertainties are likely to be introduced into the maintenance decision-making process.
2.3. Survey of experienced marine engineers
With this approach, marine engineers with several years of experience are asked to map the most appropriate maintenance strategy to different shipboard machinery systems based on their knowledge and expertise.
3. Application and results
In this Section, the proposed model is applied to a case study in order to illustrate the results of the study. A large number of sub-standard ships operate within WA waters and maintenance strategy selection for different machinery in these ships are largely based on the experience of engineers due to lack of good quality data. However, this approach often results in the selection of inappropriate and less cost-effective maintenance strategies for different equipment. Our presented models are applied to select appropriate maintenance strategies for different machinery found in the engine room of ships operating in WA, as shown in . The engine room machinery systems considered in this study are given in .
Table 3. List of engine room machinery considered in the study.
4. Results and discussion
The machinery listed in were considered in the study. The decision-making team consisted of a panel of five experts who were carefully selected during a meeting with stakeholders. They included two chief engineers (DM 1 and DM 2) with several years of experience, representing 40%. DM 3 and DM 4 (40%) were academics in this area of study and the final decision-maker, and DM 5 (20%) was a design engineer from a design consultancy firm.
The maintenance alternatives presented in were identified through literature review and subsequently agreed upon by all the DMs based on their knowledge and experience.
Table 4. Maintenance strategy alternatives considered in the study.
A total of 15 evaluation criteria were initially identified through literature review and face to face interview with the panel of experts. In order to select the essential criteria for analysis, 5 DMs screened the 15 criteria using the Delphi technique. The Delphi process was terminated after evaluating the results of the second round questionnaire since there was no significant difference between the outcome of the first round and second round iteration process. The consensus measurements for all five DMs were evaluated after the second round of the Delphi survey. Evaluation criteria with mean values less than 50% were discarded. The final evaluation criteria considered for the study are as follows:
C1: Economic criteria. Applying each maintenance strategy to engine room machinery has different cost implications. In this study, the economic criteria is considered in terms of capital investment, cost of annual O&M, cost of training personnel and cost of equipment damage. The measurement of factors, C11, C12 and C13 is in monetary unit and a smaller value is preferred to a higher value while the C14is measured using the ordinal scale (very low, low, moderate, high and very high). A minimum impact is preferred to a maximum impact.
C11: Capital investment. This factor corresponds to the capital expenditure involved in implementing the maintenance strategy. It consists of the cost of purchasing new equipment, cost of installation and cost of purchasing computers as well as advance software for condition monitoring, if required.
C12: Cost of annual operation and maintenance (O&M). This factor refers to the annual cost of O&M activities. This includes engineering/technician service cost and cost of materials for ensuring that different shipboard machinery systems continue to perform their intended function throughout the period of operation.
C13: Cost of training. This factor is evaluated in terms of the cost required to train personnel, in order to acquire the needed skills and knowledge in implementing maintenance strategies.
C14: Cost of equipment damage. This criterion refers to the maintenance strategy that can reduce or eliminate the possibility of equipment damage.
C2: Safety criteria. In the engine room of ships, health, safety and environmental issues remain a top priority. It is therefore, one of the key factors that cannot be ignored when selecting maintenance strategies for different shipboard machinery systems. The factors considered for this criterion include the safety impact on personnel, ship and the environment. Each of the factors described below is measured using an ordinal scale (very low, low, moderate, high and very high). A maximum impact is preferred to a minimum impact.
C21: Safety of personnel. The failure of engine room machinery may lead to serious injuries or death to personnel who work in the engine room. In this situation, the most effective maintenance strategy must be implemented regardless of the cost implication.
C22: Safety of ship. Failure of critical shipboard machinery systems in the engine room can put the whole ship in danger. It is advisable that greater attention is paid to high risk machinery, therefore the best maintenance strategy must be selected to ensure that the risk associated with failure of high risk machinery is reduced to tolerable levels.
C23: Safety of the environment. Failure of different shipboard machinery systems found in the engine room can lead to serious environmental consequences such as pollution. Selecting the most suitable maintenance strategy can potentially reduce the environmental risks associated with the failure of shipboard machinery systems.
C3: Added value. The added value criterion refers to the benefits to be derived from implementing each maintenance strategy. The relevant factors considered are improved operational efficiency and maintenance. Each of the factors described below is measured using an ordinal scale (very low, low, moderate, high and very high). A maximum impact is preferred to a minimum impact.
C31: Improved operational efficiency. Failure of engine room machinery can lead to reduction in ships performance. Selection of the appropriate maintenance strategy can reduce the number of failures to engine room machinery, thereby improving the operational efficiency of the entire ship.
C32: Improved maintenance. The type of failure and its significance often determine how fast a failed machinery can be restored to normal operating condition by engineers. Therefore, selecting an appropriate maintenance strategy can potentially reduce the number of failures to machinery. This can also reduce maintenance costs and lead-times, corresponding to an improvement in the availability of shipboard machinery systems.
C4: Acceptability. This criterion is divided into acceptance by ship owners and regulators and classification societies. Each of the factors described below is measured using an ordinal scale (very low, low, moderate, high and very high). A maximum approval is preferred to a minimum approval.
C41: Acceptance by ship owners. Ship owners are interested in maintenance strategies which are likely to yield more return on investment and incur low cost for implementation. Therefore, the appropriate maintenance strategy requires the support and approval of ship owners.
C42: Acceptance by regulators. The successful implementation of a maintenance strategy in the marine transportation sector sometimes requires a broad acceptance and approval by regulatory agencies and classification societies.
The local weights of the main criteria and the sub-criteria were evaluated using the scale in and Equations (1)–(4). To obtain the local weights, the DMs were asked to make pairwise comparisons for the main and sub-criteria. The global weights for sub-criteria were then calculated by aggregating the local weights of main criteria and the local weights of sub-criteria. The simple average or geometric mean of individual DM can be used to obtain the results, however, in this study, a consensus was reached by the DMs during evaluation. For example, the single judgement matrix shown in represents DMs’ opinions of the main criteria with respect to the main engine. also indicates the pairwise comparison matrix of sub-criteria with respect to economic criteria for the main engine.
Table 5. Pairwise comparison matrix of main criteria for the main engine.
Table 6. Pairwise comparison matrix of sub-criteria with respect to economic criteria for the main engine.
The consistency ratio of the pairwise comparison matrix in is 7% using RI of 0.89. Since the CR < 10%, then the information for establishing the matrix is deemed consistent and satisfactory. The local weights of the main criteria and the local and global weights of sub-criteria for the main engine are given in .
Table 7. Local and global weights of main criteria for the main engine.
After aggregating the values, the important weights of the main criteria were obtained as W = [0.6058, 0.1346, 0.1442, 0.1154]. As shown in , the DMs considered C1 as the most important determinant criterion when selecting appropriate maintenance strategies for shipboard machinery systems.
The most appropriate maintenance alternative is selected for each machinery with respect to the criteria and scales outlined in the study using the Visual PROMETHEE software (http://www.promethee-gaia.net/software.html). In this study, the linear criterion is selected for the first three quantitative criteria as the preference function while the rest of the nine qualitative criteria use the usual criterion as the preference function. This is because evidence in the literature indicates that the usual preference function is appropriate for the qualitative type of evaluation criteria while the linear preference function is suitable for the quantitative type of evaluation criteria (Turcksin et al. Citation2011). The ranking of maintenance strategies for the main engine is given in Table .
Table 8. Positive, negative and net outranking flows for maintenance alternatives for the main engine.
Now, based on the available data, the CBA procedure was applied to rank the maintenance strategies. The maintenance strategies were also ranked based on the expertise of a chief engineer and two second engineers with several years of experience working in the engine rooms of ships operating within WA waters.
The results obtained using these approaches were compared to the results of the proposed model. The comparison results of the final rankings of different maintenance strategies for the different engine room machinery are given in .
Table 9. Comparison results of the final rankings of maintenance strategies.
From the information in , the proposed semi-quantitative (i.e. AHP-PROMETHEE) model produced mixed results but it was found out that CBM and TBM were the two preferred maintenance strategies for the majority of machinery found in the engine room of ships operating in WA, while FBM is less preferred. This is because these two maintenance strategies are capable of preventing high risk failures. Similarly, mixed results were also obtained using the qualitative approach (i.e. experience and knowledge of marine engineers) with TBM and CBM dominating.
From the CBA perspective, the TBM alternative was selected for all the machinery considered. This is because the benefits from TBM included the money saved from avoiding functional failures of the machinery as well as the cost of FBM. Another benefit from the TBM is the saving from logistics and mobilisation since this maintenance strategy is often carried out by ship crew, especially in ships operating within WA waters.
Although CBM dominated as the most preferred maintenance strategy for more machinery using the proposed model, it was next to TBM from the CBA perspective. This difference may be explained by the high capital and installation costs of the CM system as well as the longer downtime involved in the installation of CM systems. The FBM strategy produced a lower B/C ratio, making it the least cost-effective maintenance strategy.
While both the semi-qualitative model and the qualitative approach produced similar results, the proposed AHP-PROMETHEE model is a more robust method for selecting appropriate maintenance strategies for different engine room machinery. This is because the proposed semi-qualitative AHP-PROMETHEE model is considered as one of the powerful and simplest ranking techniques for dealing with complex decision-making problems due to many alternatives and multiple criteria compared to other MCDM methods (Silva et al. Citation2010).
Sensitivity analysis of the proposed model was carried out by modifying the weights of the evaluation criteria by using the walking weights feature provided in the Visual PROMETHEE software. The modification of criteria weights was achieved through feedback from decision-makers. The results of the sensitivity analysis by changing weights of the evaluation criteria in relation to the main engine are shown in . The results indicate that when the weights of criteria were modified, there was a change in the final ranking of the maintenance strategies in relation to the main engine. This means that the result of the proposed model can be influenced by the criteria weights assigned by decision-makers.
5. Conclusions
In this work, a semi-qualitative model combining AHP and PROMETHEE methods was developed to support maintenance strategy selection for critical shipboard machinery systems in the engine room of ships. The proposed model consisted of three maintenance alternatives (including failure-based maintenance (FBM), time-based maintenance (TBM) and condition-based maintenance (CBM)) among which decision-makers selected the best strategy according to four comparison criteria: economic, safety, added value and acceptability. AHP was used to determine the weights of decision-makers while PROMTHEE was employed to rank the maintenance strategies.
The proposed approach was applied to select appropriate maintenance strategies for 17 different shipboard machinery systems found in the engine room of ships operating within West African (WA) waters. Thereafter, the results of the proposed method were compared to the results of quantitative and qualitative approaches such as CBA and operational experience of marine engineers.
From the results, cost was identified as the most important evaluation criteria considered during maintenance strategy selection for engine room machinery systems in ships operating in WA. The combined AHP and PROMETHEE model produced mixed results, with CBM and TBM being the two most preferred maintenance strategies for engine room machinery. Although, the combined AHP and PROMTHEE model and expert knowledge produced similar results. Thus, the proposed AHP-PROMETHEE approach can be considered as a robust method for selecting appropriate maintenance alternatives for shipboard machinery systems.
The strength of the combined AHP and PROMETHEE method is that, it considers multiple and conflicting evaluation criteria and uses both qualitative and quantitative data as well as expert judgments during maintenance strategy selection. It is therefore prudent for ship owners in WA to upgrade maintenance records of their engine room machinery using the results of the proposed model.
For future work, the proposed model will be applied to select maintenance strategies for industrial equipment in other industry sectors such as renewable energy. Also, other hybrid methods such as AHP-TOPSIS (see Dinmohammadi and Shafiee Citation2017) and AHP-VIKOR methods can be applied to determine other solutions.
Disclosure statement
No potential conflict of interest was reported by the authors.
References
- Aghaee M, Fazli S. 2012. An improved MCDM method for maintenance approach selection: a case study of auto industry. Manage Sci Lett. 2:137–146. doi: https://doi.org/10.5267/j.msl.2011.09.012
- Ahmadi A, Gupta S, Karim R, Kumar U. 2010. Selection of maintenance strategy for aircraft systems using multi-criteria decision making methodologies. Int Reliab Qual Saf Eng. 17(3):223–243. doi: https://doi.org/10.1142/S0218539310003779
- Ahmadi A, Khouy IA, Kumar U, Schunnesson H. 2009. Selection of maintenance strategy using analytical hierarchy process. Commun Depend Qual Manage. 12(1):121–132.
- Al-najjar, B., Alsyouf, I. 2003. Selecting the most efficient maintenance approach using fuzzy multiple criteria decision making. Int J Prod Econ. 84, 85–100. doi: https://doi.org/10.1016/S0925-5273(02)00380-8
- Bashiri M, Badri H, Hejazi TH. 2011. Selecting optimum maintenance strategy by fuzzy interactive linear assignment method. Appl Math Model. 35(1):152–164. doi: https://doi.org/10.1016/j.apm.2010.05.014
- Bertolini M, Bevilacqua M. 2006. A combined goal programming – AHP approach to maintenance selection problem. Reliab Eng Syst Saf. 91(7):839–848. doi: https://doi.org/10.1016/j.ress.2005.08.006
- Bevilacqua M, Braglia M. 2000. The analytic hierarchy process applied to maintenance strategy selection. Reliab Eng Syst Saf. 70(1):71–83. doi: https://doi.org/10.1016/S0951-8320(00)00047-8
- Brans J-P, Vincke P. 1985. Note – a preference ranking organisation method: (The PROMETHEE method for multiple criteria decision-making). Manage Sci. 31:647–656. doi: https://doi.org/10.1287/mnsc.31.6.647
- Chan FTS, Prakash A. 2012. Maintenance policy selection in manufacturing firms using the fuzzy MCDM approach. Int J Prod Res. 50(23):7044–7056. doi: https://doi.org/10.1080/00207543.2011.653451
- Cheng YH, Tsao HL. 2010. Rolling stock maintenance strategy selection, spares parts’ estimation, and replacements’ interval calculation. Int J Prod Econ. 128(1):404–412. doi: https://doi.org/10.1016/j.ijpe.2010.07.038
- Dinmohammadi A, Shafiee M. 2017. Determination of the most suitable technology transfer strategy for wind turbines using an integrated AHP-TOPSIS decision model. Energies. 10(5):17. doi: https://doi.org/10.3390/en10050642
- Emovon I, Norman RA, Murphy AJ. 2018. Hybrid MCDM based methodology for selecting the optimum maintenance strategy for ship machinery systems. J Intell Manuf. 29(3):519–531. doi: https://doi.org/10.1007/s10845-015-1133-6
- Fouladgar MM, Yazdani-Chamzini A, Lashgari A, Zavadskas EK, Turskis Z. 2012. Maintenance strategy selection using AHP and COPRAS under fuzzy environment. Int J Strategic Prop Manage. 16(1):85–104. doi: https://doi.org/10.3846/1648715X.2012.666657
- Ilangkumaran M, Kumanan S. 2009. Selection of maintenance policy for textile industry using hybrid multi-criteria decision making approach. J Manuf Technol Manage. 20(7):1009–1022. doi: https://doi.org/10.1108/17410380910984258
- Ilangkumaran M, Kumanan S. 2012. Application of hybrid VIKOR model in selection of maintenance strategy. Int J Inform Syst Supply Chain Manage. 5(2):59–81. doi: https://doi.org/10.4018/jisscm.2012040104
- Jajimoggala S, Rao VVSK, Satyanarayana B. 2011. Maintenance strategy evaluation using ANP and goal programming. Int J Strategic Dec Sci. 2(2):56–77. doi: https://doi.org/10.4018/jsds.2011040104
- Jayaswal P, Sagar MK, Kushwah K. 2013. Maintenance strategy selection by fuzzy TOPSIS method of material handling equipment. Int J Emerging Trends Eng Devel. 2(3):126–135.
- Kabir, G., Sadiq, R., & Tesfamariam, S. 2014. A review of multi-criteria decision-making methods for infrastructure management. Struct Infrastructure Eng. 10(9):1176–1210. doi: https://doi.org/10.1080/15732479.2013.795978
- Kumar G, Maiti J. 2012. Modeling risk based maintenance using fuzzy analytic network process. Expert Syst Appl. 39(11):9946–9954. doi: https://doi.org/10.1016/j.eswa.2012.01.004
- Kumar M, Jayaswal P, Kushwah K. 2013. Exploring fuzzy SAW method for maintenance strategy selection problem of material handling equipment. Int J Curr Eng Technol. 3(2):600–605.
- Momeni M, Fathi M, Zarchi M, Azizollahi S. 2011. A fuzzy TOPSIS-based approach to maintenance strategy selection: a case study. Middle-East J Sci Res. 8(3):699–706.
- Nezami FG, Yildirim MB. 2013. A sustainability approach for selecting maintenance strategy. Int J Sustain Eng. 6(4):332–343. doi: https://doi.org/10.1080/19397038.2013.765928
- Nikitas N, Ioannis D. 2013. Application of analytic hierarchy process & TOPSIS methodology on ships’ maintenance strategies. J Polish Safety Reliab Assoc. 4(1):21–28.
- Nikouei MA, Oroujzadeh M, Mehdipour-Ataei S. 2017. The PROMETHEE multiple criteria decision making analysis for selecting the best membrane prepared from sulfonated poly(ether ketone)s and poly(ether sulfone)s for proton exchange membrane fuel cell. Energy. 119:77–85. doi: https://doi.org/10.1016/j.energy.2016.12.052
- Odeyale SO, Alamu OJ, Odeyale EO. 2013. The analytical hierarchy process concept for maintenance strategy selection in manufacturing industries. Pac J Sci Technol. 14(1):223–233.
- Pariazar M, Shahrabi J, Zaeri MS, Parhizi S. 2008. A combined approach for maintenance strategy selection. J Appl Sci. 23(8):4321–4329. doi: https://doi.org/10.3923/jas.2008.4321.4329
- Pourjavad E, Shirouyehzad H, Shahin A. 2013. Selecting maintenance strategy in mining industry by analytic network process and TOPSIS. Int J Indus Syst Eng. 15(2):171–192.
- Ratnayake RMC, Markeset T. 2010. Technical integrity management: measuring HSE awareness using AHP in selecting a maintenance strategy. J Qual Maintenance Eng. 16(1):44–63. doi: https://doi.org/10.1108/13552511011030327
- Saaty TL. 1980. The analytical hierarchy process. New York, NY: McGraw-Hill.
- Sadeghi A, Manesh RA. 2012. The application of fuzzy group analytic network process to selection of best maintenance strategy – a case study in Mobarakeh steel company, Iran. Procedia – Social Behav Sci. 62:1378–1383. doi: https://doi.org/10.1016/j.sbspro.2012.09.236
- Samarakoon SMK, Ratnayake RMC. 2015. Strengthening, modification and repair techniques’ prioritization for structural integrity control of ageing offshore structures. Reliab Eng Syst Saf. 135:15–26. doi: https://doi.org/10.1016/j.ress.2014.10.023
- Shafiee M. 2015. Maintenance strategy selection problem: an MCDM overview. J Qual Maintenance Eng. 21(4):378–402. doi: https://doi.org/10.1108/JQME-09-2013-0063
- Shafiee M, Labib A, Maiti J, Starr A. 2019. Maintenance strategy selection for multi-component systems using a combined analytic network process and cost-risk criticality model. doi:10.1177/1748006X17712071.
- Shyjith K, Ilangkumaran M, Kumanan S. 2008. Multi-criteria decision-making approach to evaluate optimum maintenance strategy in textile industry. J Qual Maintenance Eng. 14(4):357–386. doi: https://doi.org/10.1108/13552510810909975
- Silva VBS, Morais DC, Almeida AT. 2010. A multicriteria group decision model to support watershed committees in Brazil. Water Resour Manage. 24(14):4075–4091. doi: https://doi.org/10.1007/s11269-010-9648-2
- Tan Z, Li J, Wu Z, Zheng J, He W. 2011. An evaluation of maintenance strategy using risk based inspection. Saf Sci. 49(6):852–860. doi: https://doi.org/10.1016/j.ssci.2011.01.015
- Turcksin L, Bernardini A, Macharis C. 2011. A combined AHP-PROMETHEE approach for selecting the most appropriate policy scenario to stimulate a clean vehicle fleet. Proc – Social Behav Sci. 20:954–965. doi: https://doi.org/10.1016/j.sbspro.2011.08.104
- Vinodh S, Jeya Girubha R. 2011. Promethee based sustainable concept selection. Appl Math Model. 36(11):5301–5308. doi: https://doi.org/10.1016/j.apm.2011.12.030
- VPSolutions. 2013. Visual PROMETHEE 1.4 Manual. http://www.promethee-gaia.net/assets/vpmanual.pdf (n.d.). Visual PROMETHEE, 192 p.
- Wang L, Chu J, Wu J. 2007. Selection of optimum maintenance strategies based on a fuzzy analytic hierarchy process. Int J Prod Econ. 107(1):151–163. doi: https://doi.org/10.1016/j.ijpe.2006.08.005
- Zaim S, Turkyılmaz A, Acar MF, Al-Turki U, Demirel OF. 2012. Maintenance strategy selection using AHP and ANP algorithms: a case study. J Qual Maintenance Eng. 18(1):16–29. doi: https://doi.org/10.1108/13552511211226166