ABSTRACT
Introduction: As challenges to biodiversity mount, land-use policies have been implemented to balance human needs and the integrity of ecological systems. One such program, Payments for Ecosystem Services (PES), incentivizes resource users to protect ecosystem services and has been implemented around the world to reduce soil erosion, create or improve wildlife habitats, and improve water quality and other environmental goals. The PES policy, at its core, is a concept that aims to capture the reciprocal relationships between human systems and ecological function and process. As such, PES epistemologically embodies a coupled human and natural systems approach.
Outcomes: Yet, despite this conceptual alignment, the on-the-ground implementation or evaluation of PES typically does not adopt this coupled approach and PES programs have little integration between socioeconomic, sociocultural, human demographic, and ecological elements. To advance the evolution of PES, we consider what and how socioeconomic and ecological factors have been incorporated into PES program implementation and evaluation. We also present a conceptual model to articulate how PES research can capture the reciprocal relationships among socioeconomics, demography, and ecology and discuss the quantitative modeling approaches that can support this conceptual development, i.e., structural equation and agent-based modeling, and latent trajectory models.
Conclusions: By strengthening the conceptual framework for PES within a coupled human and natural systems approach and identifyinganalytical approaches that can be used to quantify and characterize these complex cross-disciplinary relationships, we aim to support the evolution and advancement of PES, in service of more meaningful and positive outcomes for human well-being and ecological sustainability.
Introduction
Ecosystems and their corresponding services are degrading worldwide at an unprecedented manner (Ferraro and Kiss Citation2002). Ecosystem services, defined as positive benefits, direct or indirect, that wild organisms or ecosystems provide to people, have been identified as necessary for human survival and well-being (MA Citation2005; Harrison et al. Citation2010). In response to widespread environmental degradation, governments, private sector, and other actors have allocated billions of dollars to programs which provide payments to protect ecosystem services, termed payments for ecosystem services (PES) programs. PES programs provide incentives directly to resource users that engage in environmentally desirable actions or to refrain from undesirable actions to bolster ecosystem services, which are typically categorized as provisioning, regulating, supporting, or sociocultural services (Ferraro and Kiss Citation2002; Wunder Citation2007). Most PES programs are geared to improve provisioning (e.g., timber) or regulating (e.g., air or water quality) services. PES programs have been adopted in many countries as a response to many critical global environmental challenges. For example, climate-smart PES programs have been established to reduce carbon emissions from deforestation and forest degradation through carbon market mechanisms (Kanninen et al. Citation2007). In the United States, PES has also been implemented through the Conservation Reserve Program to reduce agriculture-induced soil erosion, create wildlife habitats, improve habitat quality, control crop supply, and transfer income to farmers (Smith Citation1995; Johnson, Misra, and Ervin Citation1997), with farmers receiving conservation payments for converting highly erodible or environmentally sensitive cropland to grass, woodland, or other conservation uses. Several other countries have also implemented ambitious PES programs, such as the Permanent Cover Program in Canada and the conservation payment programs in European Union countries in the 1990s (OECD Citation1997). PES has also been adopted in developing countries, including Mexico, Costa Rica, Nepal, and China (Miteva, Pattanayak, and Ferraro Citation2012). One of the largest national PES programs is China’s Grain-to-Green program (GTGP). GTGP, which aims to reduce soil erosion and increase vegetation cover through tree planting in steep farmland areas where farmers are compensated through cash, wheat or rice (Bennet 2008; Liu et al. Citation2008) was first implemented in 1999 and, in 2008, was extended for another cycle (Liu and Diamond Citation2005).
PES programs aim to reduce human impacts on ecosystems by changing human behavior in consideration of the corresponding socioeconomic, demographic, and cultural conditions (Zbinden and Lee Citation2005; Wunder Citation2008). Given this structure, PES is, at its core, a concept that captures the reciprocal (i.e., bidirectional) relationships between human activities and ecological function and processes. As such, PES epistemologically adopts an approach of coupled human and natural systems (CHANS; Liu et al. Citation2007). Yet, despite the interdisciplinary and coupled nature of the PES concept, the systematic integration of human and ecological processes in PES-related research or program evaluation remains limited (Brouwer, Tesfaye, and Pauw Citation2011; Scullion et al. Citation2011; Miteva, Pattanayak, and Ferraro Citation2012). Some of these gaps in cross-disciplinary integration are likely the result of the genesis of PES. At its inception, PES was largely viewed as an economic construct (Farley and Costanza Citation2010) and early descriptions of PES by Engel, Pagiola, and Wunder (Citation2008) prioritized the economic efficiency and ecosystem services of PES largely within a market model. More recent descriptions of PES (Muradian et al. Citation2010) have broadened to include ecological sustainability, both in a market and nonmarket context.
Another challenge to an integrated or CHANS approach in PES programs is that the underlying framework assumes a positive relationship between ecosystem quality and human economic or sociocultural well-being. This conceptual foundation has received considerable attention in the broader ecosystem services literature (Balvanera et al. Citation2014; Schröter et al. Citation2014; Harrison et al. Citation2014), with the criticism that many studies that relate human well-being to ecological changes are typically correlational, lacking direct empirical evidence (Mertz et al. Citation2007; McNally, Uchida, and Gold Citation2011; de Groot et al. Citation2010). Ecosystems can be assessed by many metrics, including metrics that measure ecosystem function, process, or composition. To date, most empirical ecosystem services research has focused on the links between changes in ecosystem composition, i.e., species richness, and ecosystem services (Balvanera et al. Citation2016). Even within this limited scope, the relationship between ecosystem services and ecosystems composition is complex (Cardinale et al. Citation2012; Harrison et al. 2014; Balvanera et al. Citation2016; Daw et al. Citation2016). In some systems, at shorter time scales, metrics of human well-being have increased as environmental conditions have degraded (Raudsepp-Hearne, Peterson, and Bennett Citation2010). Ecosystem disservice describes an inverse relationship in which an improved ecological process or function harms local people (Woodroffe, Thirgood, and Rabinowitz Citation2005). The term ecosystem service elasticity (Daw et al. Citation2016) has been used to describe the complex relationship between human well-being and different ecosystem metrics, complexity affirmed by recent research (Schröter et al. Citation2014; Harrison et al. 2014; Balvanera et al. Citation2014).
Nonetheless, in light of the pressing challenges to global ecosystems, the need for sustainability policies and strategies that support both human well-being and ecological viability is paramount. While PES policies have been identified as a solution to balancing human and ecological priorities, long-term, sustainable improvement of ecosystem features and of social and economic benefits from PES programs has not been clearly demonstrated in all but a few cases (McMaster and Davis Citation2001; Sierra and Russman Citation2006; Asquith, Vargas, and Wunder Citation2008). In this paper, we consider what and how socioeconomic and ecological system elements have been incorporated into PES program evaluation to consider the extent to which current PES research captures the reciprocal relationships between the sociocultural or economic and ecological dimensions. Through this review, we identify areas where and how stronger connections or bridges can be made between largely disparate disciplinary approaches to PES research.
Socioeconomics of PES
What socioeconomic factors are considered in PES?
PES schemes aim to affect change in human behavior and patterns of resource use by providing economic incentives to encourage compliance with resource regulations that reduce human impacts on ecosystems. A wide range of factors are often considered in PES program development and implementation including income sources and levels (Cooper and Osborn Citation1998; Chen et al. Citation2009b), land productivity (Zbinden and Lee Citation2005), alternative livelihoods (Cooper and Osborn Citation1998), distance from household to land enrolled in the program, land plot slope, age of contract holders, household labor supply, and social norms (Chen et al. Citation2009a, Citation2009b). The existence of a PES program, itself, may affect socioeconomic factors. For instance, China’s Natural Forest Conservation Program (NFCP) has substantially reduced fuelwood consumption of PES participants (Chen et al. Citation2014). In addition to the direct impact of PES on the contracted land, PES may also indirectly affect land-use decisions in larger areas due to increased regulations, changed social norms, and alternative opportunities to produce spillover effects or lead to increased degradation elsewhere to produce leakage effects when harmful human actions are moved to places not under contracts (Andam et al. Citation2008).
How have social and economic factors responded to PES programs?
Evaluation of the effects of PES on human livelihoods is an ongoing, active field of research. PES effects are often nonuniform, i.e., different for different PES participants and beneficiaries (Daw et al. Citation2011). PES socioeconomic or cultural effects are also context-dependent, influenced by factors such as social relationships, institutional arrangements, property rights, capabilities, and various capitals (Pagiola, Arcenas, and Platais Citation2005; Zilberman, Lipper, and McCarthy Citation2008). However, empirically-based quantitative evaluations of the socioeconomic benefits of PES programs remain rare in the literature.
Studies on the impacts of PES on human livelihoods have mainly focused on poverty alleviation (Pattanayak, Wunder, and Ferraro Citation2010), which is partially because environmentally significant land often coincides in areas of poverty (Wunder Citation2008). Although poor landholders may not have extensive human, financial, or technical capitals, poor landholders are often able to participate in PES because they have eligible land and property rights for compliance to PES contracts (Pagiola, Arcenas, and Platais Citation2005). Because poor landholders often have lower opportunity costs, they tend to receive higher economic benefits in PES programs with a flat payment scheme. However, in PES programs where the payment rate is determined in competitive auctions, poor landholders often bid less, resulting in less economic gains than others. Further, the impacts of PES on the poor can also be quite different due to heterogeneities in the size of land holdings (which determines the transactional costs of PES) and wealth (which is correlated to the capitals and techniques) among poor landholders (Zilberman, Lipper, and McCarthy Citation2008). In general, the efforts on monitoring PES impacts are largely focused on supporting compliance rather than sanctioning noncompliance as sanctions can be politically and logistically costly especially for government-coordinated PES programs (Pattanayak, Wunder, and Ferraro Citation2010).
PES has been found to have mixed impacts on the economy of local stakeholders. On one hand, many logging-related jobs may be terminated due to the implementation of PES programs (e.g., NFCP). On the other hand, PES may create new employment opportunities in artificial tree plantation (e.g., Costa Rica’s Environmental Services Payment program), park guards, tourism development, and monitoring for compliance to PES contracts, leading to more stable and diversified incomes (Grieg-Gran, Porras, and Wunder Citation2005). In a few Latin American cases, studies have found that PES has increased small landholders’ land-tenure security as property rights are often required for PES participation and increased human and social capitals from improved internal organization for PES implementation (Grieg-Gran, Porras, and Wunder Citation2005). PES may also have impacts on land and labor market when the land is contracted to PES programs and labor is released from agricultural sectors (Wunder Citation2008). Conservation payments from China’s GTGP have facilitated the shifting of rural labor from agricultural sector to off-farm employment in urban regions (Uchida, Rozelle, and Xu Citation2009). Further, food security has been a concern for PES programs that remove land from agriculture; however, this concern is also context dependent. A study on China’s GTGP suggested that food security has not been significantly compromised by the PES program because most of the lands that have been enrolled in the program are marginal land with low yield of crops (Xu et al. Citation2006).
Ecology of PES
What ecological factors are considered in PES?
The PES framework assumes that the relationship between ecosystem services and ecological responses is a positive one – e.g., improved forest cover or increased species richness would lead to improved services. While the broader field of ecosystem services research considers an extremely wide range of ecosystem services (e.g., Figure 3 in Harrison et al. 2014), within the PES realm, the most commonly measured ecosystem service in PES programs is land use and land cover (LULC). LULC measures floristic changes, primarily in vegetation structure rather than composition, most often of forests, and can often be measured using remotely sensed satellite imagery. Satellite remote sensing provides a tractable means to analyze change in forest cover over broad scales and several modeling approaches to estimating the fraction of forest cover based on Landsat multispectral imagery have been tested and implemented (e.g., Asner et al. Citation2005; Rogan et al. Citation2008; Hansen et al. Citation2013). These approaches primarily fall within the categories of image classification (cover interval classes), spectral mixture analysis, and continuous value machine-learning routines. Far fewer PES programs and program evaluations have included faunal as well as more detailed floral responses to PES programs (but see Liu et al. Citation2008; Tuanmu et al. Citation2016). Few studies consider cover quality, structural complexity, and vegetation or other associated faunal characteristics (Tuanmu et al. Citation2016).
How have ecological factors responded to PES programs?
PES programs typically evaluate and report program performance based on the level of program participation, or actions or behaviors of program participants, e.g., cessation of logging, acres left fallow, or number of trees planted. When ecological results or outcomes of PES programs are included, LULC is reported (Miteva, Pattanayak, and Ferraro Citation2012; Kroeger Citation2013). For example, in a review of primary literature on nine PES programs in Costa Rica and Mexico (see Table 3 in Miteva, Pattanayak, and Ferraro Citation2012), the authors find that LULC is the only ecological parameter or outcome monitored for the PES programs. Other PES meta-analyses have drawn similar conclusions. Brouwer, Tesfaye, and Pauw (Citation2011) found that quantitative, empirical information on environmental performance of existing PES schemes was lacking and that quantitative data on environmental objectives were reported by less than 50% of the PES programs reviewed. Scullion et al. (Citation2011) concluded that evidence of environmental benefits from PES programs remains limited. Even the relationship with LULC is nonuniform. For example, an increase in forest area and LULC was not found to lead to an increase in ecological performance (Hall et al. Citation2012), in part because an increase in forest cover can lead to lower species richness, particularly if a monoculture is being planted. The need for more ecologically relevant metrics has been flagged as a key ingredient to PES program development (Yin et al. Citation2013). Several authors have suggested other ecological variables or indicators of interest to monitor, including spatial configuration or connectivity of the landscape, structural complexity, faunal responses, changes to physical soil properties or erosion (Liu et al. Citation2008; Liu and Yang Citation2013; Tuanmu et al. Citation2016). This can include documenting changes in abundance, distribution, or occupancy of umbrella or indicator species (Liu and Yang Citation2013), capturing changes in floral or faunal species richness (Tuanmu et al. Citation2016) as well as the structural complexity and connectivity of the landscape (Zheng et al. Citation2013).
PES as a CHANS construct – continuing the evolution of the PES framework
The PES literature continues to track PES program participation and the impacts on human socioeconomics and demography to evaluate the ability of PES programs to lead to permanent and positive changes in environmental and human conditions, i.e., assessing the sustainability of PES programs. However, these efforts have not led to an in-depth understanding of reciprocal linkages (i.e., bidirectional) between PES and the associated human–natural systems over space and time. Nor has this research answered a key question: how do changes in the social and economic variable(s) of interest lead to changes in ecological responses and vice versa? To answer this question, the PES community will need to consider feedback loops and complex, dynamic reciprocal relationships that change over space and time and often give rise to emergent properties at macro-spatial or temporal scales, an approach that is captured by the CHANS construct.
The CHANS framework
The CHANS framework is largely equivalent to the similarly titled social–ecological systems (Ostrom Citation2009), human–environment systems (Turner et al. Citation2003), or social–environmental systems (Eakin and Luers Citation2006). Despite their many different contexts and settings, CHANS have been found to share common complex phenomena. For instance, a comparative analysis of CHANS systems (Liu et al. Citation2007) revealed analogous complex features (e.g., feedback, nonlinearity and thresholds, heterogeneity, time lags) in six CHANS systems across the globe. Study of other CHANS systems in the Amazon (Malanson, Zeng, and Walsh Citation2006a, Citation2006b), southern Yucatán (Manson Citation2005), Wolong Nature Reserve of China (An et al. Citation2005, Citation2006), and Northern Ecuador (Walsh et al. Citation2008) has identified similar features. CHANS research also adopts multi-scalar and cross-disciplinary approaches (e.g., Bian Citation1997; Phillips Citation1999; Walsh et al. Citation1999; Manson Citation2008).
The CHANS framework has a large part of its intellectual origin in complexity science. Originating, in part, from general systems theory (von Bertalanffy Citation1968; Warren, Franklin, and Streeter Citation1998), the study of complex systems focuses on heterogeneous subsystems, autonomous entities, nonlinear relationships, and multiple interactions such as feedback, learning, and adaptation (Arthur Citation1999; Axelrod and Cohen Citation1999; Manson Citation2001; Crawford et al. Citation2005; Levin et al. Citation2013). Complexity can be manifested or measured in many forms, including path-dependence, self-organization, difficulty of prediction, and emergence of qualities not analytically tractable from system components and their attributes alone (Manson Citation2001; Bankes Citation2002; An et al. Citation2014; National Research Council Citation2014).
Application of the CHANS framework in PES research
The CHANS framework recognizes the coupled nature of human and natural systems with consideration of many complexity features (Liu et al. Citation2007; An Citation2012; An et al. Citation2014). The CHANS framework reinforces a complex systems approach that has the capacity to synthesize and integrate data and models from various disciplines, scale up findings across spatial and temporal scales through approaches including interpolation or extrapolation, and simulate emergent systems dynamics that are difficult to obtain through empirical studies alone. Conceptually, PES embodies the CHANS construct by the very nature of its design. However, in practice, more meaningful integrations of socioeconomic and ecological factors are needed to answer critical questions that are integral to PES evaluation, e.g., how does human demography change as a function of ecological responses and how do changes in human activity affect ecological structure, function, and process? To date, relatively little attention has been paid to the complex interrelationships among PES components: human livelihood decisions, socioeconomic status and changing demographics, and the associated ecological responses.
To what can we attribute the lack of integration among the socioeconomic and ecological elements within PES research and literature? We posit that the lack of integration is due, largely, to the fact that coupled human–natural systems have mostly been studied separately, and many of the existing analytical methods are disciplinary and less suited to cross-disciplinary explorations (An Citation2012). Despite numerous and repeated calls for meaningful integration of human and natural elements in coupled complex systems (Ostrom Citation2007), the PES literature, as with other related areas of study, remains largely canalized between natural and social sciences. The absence of ecological factors or processes within the PES research has been found to stem, in part, from the lack of interdisciplinary dialogue (Vogt et al. Citation2015). A history of division between natural and social sciences, along with postulated unidirectional exploration of connections between natural and human systems, has hindered understanding of complexity in CHANS (Liu et al. Citation2007; An Citation2012). Another notable challenge to engaging in or catalyzing transdisciplinary PES research is the need for a robust and multifaceted data set that captures sociocultural, demographic, economic, and ecological data. Although beyond the scope of this paper, this topic is explored in recent literature (Leavey Citation2016) and warrants attention.
To support the continued evolution of the CHANS concept in PES and continued or improved efficacy of PES programs, a more direct integration of socioeconomic and ecological responses is imperative. In this context, we propose a new CHANS-based framework for PES research ().
Figure 1. An illustration of a CHANS framework for PES research. Solid arrows represent recognized impacts, while dotted arrows represent unstudied or understudied relationships. The legend provides a description of the reciprocal relationships among PES programs, human systems, and ecosystems. The diagonal time line captures the temporal dimension which is essential in PES research as all elements in this CHANS will change and evolve over time. Lines shown here can include nonlinear, synergistic, or lagged effects that may occur on different temporal and spatial scales.
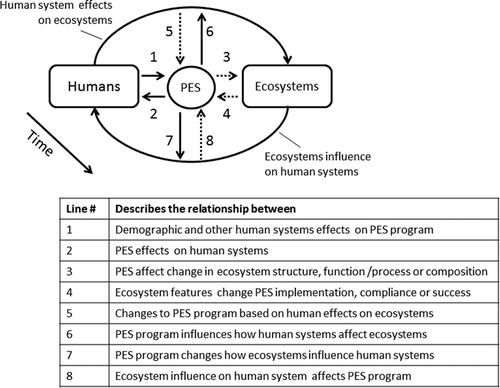
This illustration captures the human and ecological subsystems (rectangular boxes) and the reciprocal linkages that connect each subsystem, denoted by the two curved arrows that link from humans to ecosystems (top) and ecosystems to humans (bottom). Considerable literature has documented how humans, i.e., through various resource extraction or land-use actions, have affected corresponding ecosystem features and processes, e.g., land cover, forest composition, soil erosion, and biodiversity (e.g., Matson et al. Citation1997; Vitousek et al. Citation1997; Tscharntke et al. Citation2005; Johnson, Edwards, and Erhardt Citation2007; Kadafa Citation2012; Nakayama and Shankman Citation2013). Another body of literature, although less populated, has documented the opposite relationship of how changes in ecosystem features have influenced socioeconomic parameters (e.g., Schuster and Highland Citation2007; Antwi-Agyei et al. Citation2012; Dikgang and Muchapondwa Citation2012).
When PES programs are implemented, i.e., oval in the center of , the programs first directly affect and are affected by the human subsystem as represented by lines 1 and 2. In this model, the human subsystem refers to local people or communities that reside in the CHANS system, as opposed to policy makers or government agencies. Numerous studies have documented demographic factors that impact PES participation (Chen et al. 2009a, 2009b), and how local people’s well-being or demographics may change due to PES (Grieg-Gran, Porras, and Wunder Citation2005; Pattanayak, Wunder, and Ferraro Citation2010). PES programs also directly affect and are affected by, ecosystems. These relationships are represented by dotted lines 3 and 4 as they are less studied or poorly understood. The line that links PES to ecosystems (line 3) is not meant to imply that a PES program would directly alter ecosystem structure or function without going through humans. What we emphasize here is the often longer term, nonlinear, or lagged ecological processes or interactions (e.g., succession, prey–predator relationships, and biological competition) induced or triggered by the PES program that are related to human actions. Likewise, the relationship that links ecosystems to PES (line 4) has also been rarely studied, i.e., how changes in species richness or ecological structural or compositional complexity have influenced PES program development, implementation, or effectiveness.
PES programs likely change, and are changed, by the way that humans affect ecosystems (lines 5 and 6), although these relationships are also not well studied and understood. Ongoing research at two protected areas in China is addressing this knowledge gap. For instance, indigenous people in Wolong Nature Reserve and Fanjingshan National Nature Reserve traditionally extract fuelwood and medicinal plants directly from the reserves (An et al. Citation2005; Wandersee et al. Citation2012). With the implementation of the aforementioned PES programs, NFCP, and GTGP, local people have changed resource use patterns, illustrating how PES may affect the way humans make use of or rely on local ecosystems, represented by line 6. In Wolong, local people reduced the amount of fuelwood extracted from local forests after NFCP initiation (Chen et al. Citation2014; Tuanmu et al. Citation2016). Household surveys in 2014 and 2015 in Fanjingshan suggest that local villagers made fewer forest visits for mushroom and medicinal plant collection post-PES initiation (An et al. unpublished data). Some households which received direct income from PES invested the money on public infrastructure (e.g., road construction). These changes in resource use and income may, in turn, affect ecosystems (the top curved arrow) which can have substantial influence on PES program success (line 5).
Likewise, we expect that PES programs would change, and be changed by, the bottom curved arrow which represents how ecosystems changes affect humans. For example, more widespread availability of primary forest post-PES implementation at Fanjingshan might account for the reported decline in medicinal herb extraction, found in the understory layer of primary canopy forests. Since GTGP implementation, local people tend to spend income and associated time from PES participation on other more lucrative, less financially tenuous activities such as running local businesses (e.g., restaurant, hotel). This example shows that the PES programs at Fanjingshan may trigger local people to shift from more resource-dependent to a more market-dependent lifestyle.
Methodological applications of the CHANS framework for PES
Developing an integrated CHANS framework for PES is more than a change in a conceptual paradigm. Adopting a CHANS framework, along with its complex systems approach, will allow PES programs to continue to develop conceptually and in practice. The current gaps in the CHANS framework for PES also highlight the need for more advanced analytical approaches. As depicts, PES includes various looped or coupled processes, where factors affect or are affected by one another and each could be a driver and a consequence simultaneously or at different stages. Traditional regression-based approaches, where dependent and independent variables are easily identifiable, may not adequately address a complex system of this nature. One modeling approach that has been used to address complex system analyses is called structural equation modeling (SEM). SEM is widely used in the social sciences to study complex interdependent relationships between different latent variables, often represented as various paths, such as the ones as shown in . SEM uniquely estimates coefficients of various causal relationships (paths) by choosing the most fitted model whose estimated covariance structure best match the empirical covariance matrices (An et al. Citation2003; Bollen and Curran Citation2006; Weeks et al. Citation2004; Santibáñez-Andrade et al. Citation2015).
When developing conservation strategies for the forests of the Magdalena river basin in Mexico City, Santibáñez-Andrade et al. (Citation2015) applied SEM to evaluate complex relationships among ecosystem composition, structure, and function (measures of the current quality and quantity of natural resources, stressors from human activities, such as presence of rubbish, grazing, visitors, and fire, and environmental measures, e.g., relative humidity, environmental temperature, soil temperature, altitude, and slope). With data from 75 plots of 25 × 25 m at Magdalena river basin, they explore the relationship among coupled factors. Their SEM analyses suggest that plant regeneration, a measure of ecosystem function, is only significantly predicted by human activities, rather than other ecosystem metrics or abiotic factors indicators.
A second methodology that can support a complex systems approach is digital simulation models. One such simulation approach is agent-based modeling (ABM). ABM is an object-oriented program that represents all related entities and subsystems as agents at various, often hierarchical levels. Model components, including agents, follow flexible rules to mimic complex and often nonlinear relationships and interactions. ABM approaches have been used to understand system structure and function and can support the development of relevant actions to effectively manage complex systems (Axelrod and Cohen Citation1999). For example, local households or individual people can be modeled as spatially explicit agents at specific locations with associated behaviors at/near ecosystems, which in turn can be modeled as a dynamic, updatable, pixelized world. All the resource or land-use activities (e.g., farming, extraction of fuelwood) are simulated in the local ecosystems, providing an opportunity to monitor changes in local ecosystems or social systems to capture nature of the reciprocal relationships denoted in .
Recent studies have used ABM to explore human–environment interactions that are shaped by PES programs and evaluate the effectiveness of PES (Sun and Müller Citation2013). For instance, Smajgl et al. (Citation2015) use ABMs to consider PES programs success without monitoring or regulatory mechanisms; they found that PES schemes may not achieve the expected objectives if appropriate monitoring and regulatory mechanisms are not enforced. In evaluating China’s NFCP, Chen et al. (Citation2014) use ABM to consider how electricity payments, rather than cash payments, would impact the NFCP and found that if the cash payment can be replaced with an electricity payment, the effectiveness can be improved by 39%. ABM has also been used to understand the alternative design and implementation of PES schemes. In another example, Chen et al. (Citation2012) simulated the evolution and impacts of social norms on the PES participation. They found that more than 15% additional land can be enrolled at the same price in China’s GTGP due to the effects of social norms if repeated interactions among landholders regarding their land-use decisions are allowed.
Finally, the CHANS framework can account for a temporal dimension which is essential in PES research. Due to data limitation, researchers use cross-sectional data to explore various relationships in the above diagram. But without longitudinal data and observations, many of the putative relationships cannot be tested and established (Guo and Hippo 2004). There is a clear need in PES-related research for integrated socio-ecological data collection, monitoring, and evaluation over relatively longer time scales. Once longitudinal data have been collected in a PES system, latent trajectory models (LTMs) can be used to support an exploration of coupled socio-ecological temporal patterns, i.e., how does slope of land parcels influence PES participation and how successful are PES programs in reducing erosion, retain topsoil, or improving water quality of areas of high slopes (An et al. Citation2016)?
Conclusions
As threats to human and ecological sustainability mount, the need for programs and policies that are designed to simultaneously support human well-being and ecological composition, process, and function intensifies. Conceptually, PES embodies a CHANS approach by recognizing the fundamental reciprocal relationships among human activities, human well-being, and ecological conditions. Despite this, the research and implementation of PES continues to focus largely on socioeconomic or demographic and ecological factors independently, with limited research on the complex, interrelationship among socioeconomic, demography, and ecological metrics, as represented in . Ecosystem services within PES program evaluation are largely limited to LULC as a proxy for ecosystem services (but see Tuanmu et al. Citation2016). To resolve these conceptual and empirical gaps will require study of a more representative range of ecological responses to PES, building on and drawing from the rich ES literature (Harrison et al. 2014; Schröter et al. Citation2014; Balvanera et al. Citation2016). Continued exploration of ES elasticity (Daw et al. Citation2016) and how ES elasticity changes over space and time will also be needed to support the evolution of PES both in terms of conceptual development and empirical application. From an analytical perspective, adopting a more integrated CHANS framework for PES programs would provide field practitioners with tools and information that can support PES program relevance to context-dependent management needs. With a more comprehensive understanding of the complex linkages among social, cultural, demographic, and ecological dimensions, PES programs may be improved and fine tuned to adapt to inherent dynamics that govern both social and ecological subsystems.
Disclosure statement
No potential conflict of interest was reported by the authors.
Additional information
Funding
References
- An, L. 2012. “Modeling Human Decisions in Coupled Human and Natural Systems: Review of Agent-Based Models.” Ecological Modelling 229 (24): 25–11. doi:10.1016/j.ecolmodel.2011.07.010.
- An, L., A. Mertig, and J. Liu. 2003. “Adolescents’ Leaving Parental Home in Wolong Nature Reserve (China): Psychosocial Correlates and Implications for Panda Conservation.” Population and Environment: A Journal of Interdisciplinary Studies 24: 415–444. doi:10.1023/A:1023694924954.
- An, L., A. Zvoleff, J. Liu, and W. Axinn. 2014. “Agent Based Modeling in Coupled Human and Natural Systems (CHANS): Lessons from a Comparative Analysis.” Annals of the Association of American Geographers 104 (4): 723–745. doi:10.1080/00045608.2014.910085.
- An, L., G. He, Z. Liang, and J. Liu. 2006. “Impacts of Demographic and Socioeconomic Factors on Spatio-Temporal Dynamics of Panda Habitats.” Biodiversity and Conservation 15: 2343–2363. doi:10.1007/s10531-004-1064-6.
- An, L., M. Linderman, J. Qi, A. Shortridge, and J. Liu. 2005. “Exploring Complexity in a Human-Environment System: An Agent-Based Spatial Model for Multidisciplinary and Multiscale Integration.” Annals of the Association of American Geographers 95: 54–79. doi:10.1111/j.1467-8306.2005.00450.x.
- An, L., M.-H. Tsou, B. Spitzberg, J. M. Gawron, and D. K. Gupta. 2016. “Latent Trajectory Models for Space-Time Analysis: An Application in Deciphering Spatial Panel Data.” Geographical Analysis 48 (3): 314–333. doi:10.1111/gean.2016.48.issue-3.
- Andam, K. S., P. J. Ferraro, A. Pfaff, G. A. Sanchez-Azofeifa, and J. A. Robalino. 2008. “Measuring the Effectiveness of Protected Area Networks in Reducing Deforestation.” Proceedings of the National Academy of Sciences of the United States of America 105: 16089–16094. doi:10.1073/pnas.0800437105.
- Antwi-Agyei, P., E. D. G. Fraser, A. J. Dougill, L. C. Stringer, and E. Simelton. 2012. “Mapping the Vulnerability of Crop Production to Drought in Ghana Using Rainfall, Yield and Socioeconomic Data.” Applied Geography 32 (2): 324–334. doi:10.1016/j.apgeog.2011.06.010.
- Arthur, W. B. 1999. “Complexity and the Economy.” Science 284: 107–109. doi:10.1126/science.284.5411.107.
- Asner, G. P., D. E. Knapp, A. N. Cooper, M. M. Bustamante, and L. P. Olander. 2005. “Ecosystem Structure Throughout the Brazilian Amazon from Landsat Observations and Automated Spectral Unmixing.” Earth Interactions 9 (7): 1–31. doi:10.1175/EI134.1.
- Asquith, N. M., M. T. Vargas, and S. Wunder. 2008. “Selling Two Environmental Services: In-Kind Payments for Bird Habitat and Watershed Protection in Los Negros, Bolivia.” Ecological Economics 65: 675–684. doi:10.1016/j.ecolecon.2007.12.014.
- Axelrod, R., and M. D. Cohen. 1999. Harnessing Complexity: Organizational Implications of a Scientific Frontier. New York: Free Press.
- Balvanera, P., I. Siddique, L. Dee, A. Paquette, F. Isbell, A. Gonzalez, J. Byrnes, M. I. O’Connor, B. A. Hungate, and J. N. Griffin. 2014. “Linking Biodiversity and Ecosystem Services: Current Uncertainties and the Necessary Next Steps.” BioScience, no. 1: 49–57. doi:10.1093/biosci/bit003Balvanera.
- Balvanera, P., S. Quijas, B. Martin Lopez, E. Barrios, L. Dee, F. Isbell, I. Durance, P. White, R. Blanchard, and R. de Groot. 2016. The Links between Biodiversity and Ecosystem Services, 45–59. London: Routledge Handbook on Ecosystem Services.
- Bankes, S. C. 2002. “Tools and Techniques for Developing Policies for Complex and Uncertain Systems.” Proceedings of the National Academy of Sciences 99 (3): 7263: 7266. doi:10.1073/pnas.092081399.
- Bennett, M. T. 2008. “China’s Sloping Land Conversion Program: Institutional Innovation or Business as Usual?” Ecological Economics 65: 699–711. doi:10.1016/j.ecolecon.2007.09.017.
- Bian, L. 1997. “Multiscale Nature of Spatial Data in Scaling up Environmental Models.” In Scale in Remote Sensing and GIS, edited by D. A. Quattrochi and M. F. Goodchild, 13–26. New York: Lewis Publishers.
- Bollen, K. A., and P. J. Curran. 2006. Latent Curve Models: A Structural Equation Perspective. Hoboken, NJ: Wiley-Interscience.
- Brouwer, R., A. Tesfaye, and P. Pauw. 2011. “Meta-Analysis of Institutional-Economic Factors Explaining the Environmental Performance of Payments for Watershed Services.” Environmental Conservation 38 (4): 380–392. doi:10.1017/S0376892911000543.
- Cardinale, B. J., J. E. Duffy, A. Gonzalez, D. U. Hooper, C. Perrings, P. Venail, A. Narwani, et al. 2012. “Biodiversity Loss and Its Impact on Humanity.” Nature 486: 59–67. doi:10.1038/nature11148.
- Chen, X. D., A. Viña, A. Shortridge, L. An, and J. G. Liu. 2014. “Assessing the Effectiveness of Payments for Ecosystem Services: An Agent-Based Modeling Approach.” Ecology and Society 19: 7. doi:10.5751/ES-05578-190107.
- Chen, X. D., F. Lupi, G. M. He, and J. G. Liu. 2009a. “Linking Social Norms to Efficient Conservation Investment in Payments for Ecosystem Services.” Proceedings of the National Academy of Sciences of the United States of America 106: 11812–11817. doi:10.1073/pnas.0809980106.
- Chen, X. D., F. Lupi, G. M. He, Z. Y. Ouyang, and J. G. Liu. 2009b. “Factors Affecting Land Reconversion Plans following a Payment for Ecosystem Service Program.” Biological Conservation 142: 1740–1747. doi:10.1016/j.biocon.2009.03.012.
- Chen, X. D., F. Lupi, L. An, R. Sheely, A. Viña, and J. G. Liu. 2012. “Agent-Based Modeling of the Effects of Social Norms on Enrollment in Payments for Ecosystem Services.” Ecological Modelling 229: 16–24. doi:10.1016/j.ecolmodel.2011.06.007.
- Cooper, J. C., and C. T. Osborn. 1998. “The Effect of Rental Rates on the Extension of Conservation Reserve Program Contracts.” American Journal of Agricultural Economics 80: 184–194. doi:10.2307/3180280.
- Crawford, T. W., J. P. Messina, S. M. Manson, and D. O’Sullivan. 2005. “Complexity Science, Complex Systems, and Land-Use Research.” Environment and Planning B: Planning and Design 32 (6): 792–798. doi:10.1068/b3206ed.
- Daw, T., K. Brown, S. Rosendo, and R. Pomeroy. 2011. “Applying the Ecosystem Services Concept to Poverty Alleviation: The Need to Disaggregate Human Well-Being.” Environmental Conservation 38: 370–379. doi:10.1017/S0376892911000506.
- Daw, T. M., C. Hicks, K. Brown, T. Chaigneau, F. Januchowski-Hartley, W. Cheung, S. Rosendo, et al. 2016. “Elasticity in Ecosystem Services: Exploring the Variable Relationship between Ecosystems and Human Well-Being.” Ecology and Society 21 (2): 11. doi:10.5751/ES-08173-210211.
- de Groot, R. S., J. R. M. Alkemade, L. Braat, L. G. Hein, and L. L. J. M. Willemen. 2010. “Challenges in Integrating the Concept of Ecosystem Services and Values in Landscape Planning, Management and Decision-Making.” Ecological Complexity 7: 260–272. doi:10.1016/j.ecocom.2009.10.006.
- Dikgang, J., and E. Muchapondwa. 2012. “The Valuation of Biodiversity Conservation by the South African Khomani San Bushmen Community.” Ecological Economics 84: 7–14. doi:10.1016/j.ecolecon.2012.09.001.
- Eakin, H., and A. L. Luers. 2006. “Assessing the Vulnerability of Social-Environmental Systems.” Annual Review of Environment and Resources 31 (1): 365–394. doi:10.1146/annurev.energy.30.050504.144352.
- Engel, S., S. Pagiola, and S. Wunder. 2008. “Designing Payments for Environmental Services in Theory and Practice: An Overview of the Issues.” Ecological Economics 65 (4): 663–674. doi:10.1016/j.ecolecon.2008.03.011.
- Farley, J., and R. Costanza. 2010. “Payments for Ecosystem Services: From Local to Global.” Ecological Economics 69 (11): 2060–2068. doi:10.1016/j.ecolecon.2010.06.010.
- Ferraro, P. J., and A. Kiss. 2002. “Ecology—Direct Payments to Conserve Biodiversity.” Science 298: 1718–1719. doi:10.1126/science.1078104.
- Grieg-Gran, M., I. Porras, and S. Wunder. 2005. “How Can Market Mechanisms for Forest Environmental Services Help the Poor? Preliminary Lessons from Latin America.” World Development 33: 1511–1527. doi:10.1016/j.worlddev.2005.05.002.
- Guo, G., and J. Hipp. 2004. “Longitudinal Analysis for Continuous Outcomes.” In The Handbook of Data Analysis, edited by M. Hardy and A. Bryman, 347–368. Los Angeles: SAGE Publications.
- Hall, J. M., T. Van Holt, A. E. Daniels, V. Balthazar, and E. F. Lambin. 2012. “Trade-Offs between Tree Cover, Carbon Storage and Floristic Biodiversity in Reforesting Landscapes.” Landscape Ecology 27: 1135–1147. doi:10.1007/s10980-012-9755-y.
- Hansen, M. C., P. V. Potapov, R. Moore, M. Hancher, S. A. Turubanova, A. Tyukavina, D. Thau, et al. 2013. “High-Resolution Global Maps of 21st-Century Forest Cover Change.” Science 342: 850–853. doi:10.1126/science.1244693.
- Harrison, P. A., M. Vandewalle, M. T. Sykes, P. M. Berry, R. Bugter, F. de Bello, C. K. Feld, et al. 2010. “Identifying and Prioritising Services in European Terrestrial and Freshwater Ecosystems.” Biodiversity and Conservation 19: 2791–2821. doi:10.1007/s10531-010-9789-x.
- Harrison, P. A., P. M. Berry, G. Simpson, J. R. Haslett, M. Blicharska, M. Bucur, R. Dunford, et al. 2014. “Linkages Between Biodiversity Attributes and Ecosystem Services: A Systematic Review.” Ecosystem Services 9: 191-203.
- Johnson, A. C., R. T. Edwards, and R. Erhardt. 2007. “Ground-Water Response to Forest Harvest: Implications for Hillslope Stability.” Journal of the American Water Resources Association 43 (1): 134–147. doi:10.1111/j.1752-1688.2007.00011.x.
- Johnson, P. N., S. K. Misra, and R. T. Ervin. 1997. “A Qualitative Choice Analysis of Factors Influencing Post-Crp Land Use Decisions.” Journal of Agricultural and Applied Economics 29: 163–173. doi:10.1017/S1074070800007641.
- Kadafa, A. A. 2012. “Environmental Impacts of Oil Exploration and Exploitation in the Niger Delta of Nigeria.” Global Journal of Science Frontier Research Environment & Earth Sciences 12 (3): 19–28.
- Kanninen, M., D. Murdiyarso, F. Seymour, A. Angelsen, S. Wunder, and L. German. 2007. Do Trees Grow on Money? The Implications of Deforestation Research for Policies to Promote REDD. Bogor: CIFOR Forest Perspectives No. 4.
- Kroeger, T. 2013. “The Quest For The “Optimal” Payment for Environmental Services Program: Ambition Meets Reality, With Useful Lessons.” Forest Policy and Economics 37: 65-74. doi:10.1016/j.forpol.2012.06.007.
- Leavey, P. 2016. Essentials of Transdisciplinary Research: Using Problem-Centered Methodologies. London: Routledge Taylor, Francis Group.
- Levin, S. A., T. Xepapadeas, A.-S. Crépin, J. Norberg, A. de Zeeuw, C. Folke, T. Hughes, et al. 2013. “Social-Ecological Systems as Complex Adaptive Systems: Modeling and Policy Implications.” Environment and Development Economics 18 (2): 111–132. doi:10.1017/S1355770X12000460.
- Liu, J., S. Li, Z. Ouyang, C. Tam, and X. Chen. 2008. “Ecological and Socioeconomic Effects of China’s Policies for Ecosystem Services.” Proceedings of the National Academy of Sciences 105 (28): 9477–9482. doi:10.1073/pnas.0706436105.
- Liu, J., T. Dietz, S. R. Carpenter, M. Alberti, C. Folke, E. Moran, A. N. Pell, et al. 2007. “Complexity of Coupled Human and Natural Systems.” Science 317: 1513–1516. doi:10.1126/science.1144004.
- Liu, J., and W. Yang, 2013. "Integrated Assessments of Payments for Ecosystem Services Programs." Proceedings of The National Academy of Sciences 110 (41): 16297-16298.
- Liu, J. G., and J. Diamond. 2005. “China’s Environment in a Globalizing World.” Nature 435: 1179–1186. doi:10.1038/4351179a.
- MA. 2005. Ecosystems and Humanwell-Being: Synthesis. Millennium Ecosystem Assessment. Washington, DC: Island Press.
- Malanson, G. P., Y. Zeng, and S. J. Walsh. 2006a. “Complexity at Advancing Ecotones and Frontiers.” Environment and Planning A 38: 619–632. doi:10.1068/a37340.
- Malanson, G. P., Y. Zeng, and S. J. Walsh. 2006b. “Landscape Frontiers, Geography Frontiers: Lessons to Be Learned.” The Professional Geographer 58 (4): 383–396. doi:10.1111/j.1467-9272.2006.00576.x.
- Manson, S. M. 2001. “Simplifying Complexity: A Review of Complexity Theory.” Geoforum 32: 405–414. doi:10.1016/S0016-7185(00)00035-X.
- Manson, S. M. 2005. “Agent-Based Modeling and Genetic Programming for Modeling Land Change in the Southern Yucatán Peninsular Region of Mexico.” Agriculture, Ecosystems & Environment 111 (1–4): 47–62. doi:10.1016/j.agee.2005.04.024.
- Manson, S. M. 2008. “Does Scale Exist? An Epistemological Scale Continuum for Complex Human-Environment Systems.” Geoforum 39: 776–788. doi:10.1016/j.geoforum.2006.09.010.
- Matson, P. A., W. J. Parton, A. G. Power, and M. J. Swift. 1997. “Agricultural Intensification and Ecosystem Properties.” Science 277 (5325): 504–509. doi:10.1126/science.277.5325.504.
- McMaster, D. G., and S. K. Davis. 2001. “An Evaluation of Canada’s Permanent Cover Program: Habitat for Grassland Birds?” Journal of Field Ornithology 72: 195–210. doi:10.1648/0273-8570-72.2.195.
- McNally, C., E. Uchida, and A. Gold. 2011. “The Effect of a Protected Area on the Tradeoffs between Short-Run and Long-Run Benefits from Mangrove Ecosystems.” Proceedings of the National Academy of Sciences 108: 13945–13950. doi:10.1073/pnas.1101825108.
- Mertz, O., H. M. Ravnborg, G. L. LöVei, I. Nielsen, and C. C. Konijnendijk. 2007. “Ecosystem Services and Biodiversity in Developing Countries.” Biodiversity and Conservation 16 (10): 2729–2737. doi:10.1007/s10531-007-9216-0.
- Miteva, D. A., S. K. Pattanayak, and P. J. Ferraro. 2012. “Evaluation of Biodiversity Policy Instruments: What Works and What Doesn’t?” Oxford Review of Economic Policy 28 (1): 69–92. doi:10.1093/oxrep/grs009.
- Muradian, R., E. Corbera, U. Pascual, N. Kosoy, and P. H. May. 2010. “Reconciling Theory and Practice: An Alternative Conceptual Framework for Understanding Payments for Environmental Services.” Ecological Economics 69: 1202–1208. doi:10.1016/j.ecolecon.2009.11.006.
- Nakayama, T., and D. Shankman. 2013. “Impact of the Three-Gorges Dam and Water Transfer Project on Changjiang Floods.” Global and Planetary Change 100: 38–50. doi:10.1016/j.gloplacha.2012.10.004.
- NRC (National Research Council). 2014. Advancing Land Change Modeling: Opportunities and Research Requirements. Washington, DC: National Academies Press.
- OECD (Organization for Economic Cooperation and Development). 1997. The Environmental Effects of Agricultural Land Diversion Schemes. Paris: OECD.
- Ostrom, E., 2007. "A Diagnostic Approach for Going Beyond Panaceas." Proceedings of The National Academy of Sciences 104 (39): 15181-15187. doi:10.1073/pnas.0702098104
- Ostrom, E. 2009. “A General Framework for Analyzing Sustainability of Social-Ecological Systems.” Science 325: 419–422. doi:10.1126/science.1172133.
- Pagiola, S., A. Arcenas, and G. Platais. 2005. “Can Payments for Environmental Services Help Reduce Poverty? An Exploration of the Issues and the Evidence to Date from Latin America.” World Development 33: 237–253. doi:10.1016/j.worlddev.2004.07.011.
- Pattanayak, S. K., S. Wunder, and P. J. Ferraro. 2010. “Show Me the Money: Do Payments Supply Environmental Services in Developing Countries?” Review of Environmental Economics and Policy 4: 254–274. doi:10.1093/reep/req006.
- Phillips, J. D. 1999. Earth Surface Systems: Complexity, Order, and Scale. Malden: Blackwell.
- Raudsepp-Hearne, C., G. D. Peterson, and E. M. Bennett. 2010. “Ecosystem Service Bundles for Analyzing Tradeoffs in Diverse Landscapes.” Proceedings of the National Academy of Sciences 107 (11): 5242–5247. doi:10.1073/pnas.0907284107.
- Rogan, J., J. Franklin, D. Stow, J. Miller, C. Woodcock, and D. Roberts. 2008. “Mapping Land-Cover Modifications over Large Areas: A Comparison of Machine Learning Algorithms.” Remote Sensing of Environment 112 (5): 2272–2283. doi:10.1016/j.rse.2007.10.004.
- Santibáñez-Andrade, G., S. Castillo-Argüero, E. V. Vega-Peña, R. Lindig-Cisneros, and J. A. Zavala-Hurtado. 2015. “Structural Equation Modeling as a Tool to Develop Conservation Strategies Using Environmental Indicators: The Case of the Forests of the Magdalena River Basin in Mexico City.” Ecological Indicators 54: 124–136. doi:10.1016/j.ecolind.2015.02.022.
- Schröter, M., E. H. Zanden, A. P. Oudenhoven, R. P. Remme, H. M. Serna‐Chavez, R. S. Groot, and P. Opdam. 2014. “Ecosystem Services as a Contested Concept: A Synthesis of Critique and Counter‐Arguments.” Conservation Letters 7 (6): 514–523. doi:10.1111/conl.12091.
- Schuster, R. L., and L. M. Highland. 2007. “The Third Hans Cloos Lecture. Urban Landslides: Socioeconomic Impacts and Overview of Mitigative Strategies.” Bulletin of Engineering Geology and the Environment 66 (1): 1–27. doi:10.1007/s10064-006-0080-z.
- Scullion, J., C. W. Thomas, K. A. Vogt, O. Pérez-Maqueo, and M. G. Logsdon. 2011. “Evaluating the Environmental Impact of Payments for Ecosystem Services in Coatepec (Mexico) Using Remote Sensing and On-Site Interviews.” Environmental Conservation 38 (4): 426–434. doi:10.1017/S037689291100052X.
- Sierra, R., and E. Russman. 2006. “On the Efficiency of Environmental Service Payments: A Forest Conservation Assessment in the Osa Peninsula, Costa Rica.” Ecological Economics 59: 131–141. doi:10.1016/j.ecolecon.2005.10.010.
- Smajgl, A., J. Xu, S. Egan, Z. Yi, J. Ward, and Y. Su. 2015. “Assessing the Effectiveness of Payments for Ecosystem Services for Diversifying Rubber in Yunnan, China.” Environmental Modelling & Software 69: 187–195. doi:10.1016/j.envsoft.2015.03.014.
- Smith, R. B. W. 1995. “The Conservation Reserve Program as a Least-Cost Land Retirement Mechanism.” American Journal of Agricultural Economics 77: 93–105. doi:10.2307/1243892.
- Sun, Z., and D. Müller. 2013. “A Framework for Modeling Payments for Ecosystem Services with Agent-Based Models, Bayesian Belief Networks and Opinion Dynamics Models.” Environmental Modelling & Software 45: 15–28. doi:10.1016/j.envsoft.2012.06.007.
- Tscharntke, T., A. M. Klein, A. Kruess, I. Steffan-Dewenter, and C. Thies. 2005. “Landscape Perspectives on Agricultural Intensification and Biodiversity – Ecosystem Service Management.” Ecology Letters 8 (8): 857–874. doi:10.1111/j.1461-0248.2005.00782.x.
- Tuanmu, M.-N., A. Viña, W. Yang, X. Chen, A. M. Shortridge, and J. Liu. 2016. “Effects of Payments for Ecosystem Services on Wildlife Habitat Recovery.” Conservation Biology 30 (4): 827–835. doi:10.1111/cobi.2016.30.issue-4.
- Turner, B. L., P. A. Matson, J. J. McCarthy, R. W. Corell, L. Christensen, N. Eckley, G. K. Hovelsrud-Broda, et al. 2003. “Illustrating the Coupled Human–Environment System for Vulnerability Analysis: Three Case Studies.” Proceedings of the National Academy of Sciences of the United States of America 100 (14): 8080–8085. doi:10.1073/pnas.1231334100.
- Uchida, E., S. Rozelle, and J. T. Xu. 2009. “Conservation Payments, Liquidity Constraints, and Off-Farm Labor: Impact of the Grain-For-Green Program on Rural Households in China.” American Journal of Agricultural Economics 91: 70–86. doi:10.1111/ajae.2009.91.issue-1.
- Vitousek, P. M., H. A. Mooney, J. Lubchenco, and J. M. Melillo. 1997. “Human Domination of Earth’s Ecosystems.” Science 277 (5325): 494–499. doi:10.1126/science.277.5325.494.
- Vogt, J. M., G. B. Epstein, S. K. Mincey, B. C. Fischer, and P. McCord. 2015. "Putting the "E" in Ses: Unpacking the Ecology in the Ostrom Social-ecological System Framework." 20: (1): 55. doi: 10.5751/ES-07239-200155.
- von Bertalanffy, L. 1968. General System Theory: Foundations, Development, Applications. New York: George Braziller.
- Walsh, S. J., J. P. Messina, C. F. Mena, G. P. Malanson, and P. H. Page. 2008. “Complexity Theory, Spatial Simulation Models, and Land Use Dynamics in the Northern Ecuadorian Amazon.” Geoforum 39: 867–878. doi:10.1016/j.geoforum.2007.02.011.
- Walsh, S. J., T. P. Evans, W. F. Welsh, B. Entwisle, and R. R. Rindfuss. 1999. “Scale-Dependent Relationships between Population and Environment in Northeastern Thailand.” Photogrammetric Engineering & Remote Sensing 65 (1): 97–105.
- Wandersee, S. M., L. An, D. López-Carr, and Y. Yang. 2012. “Perception and Decisions in Modeling Coupled Human and Natural Systems: A Case Study from Fanjingshan National Nature Reserve, China.” Ecological Modelling 229: 37–49. doi:10.1016/j.ecolmodel.2011.08.004.
- Warren, K., C. Franklin, and C. L. Streeter. 1998. “New Directions in Systems Theory: Chaos and Complexity.” Social Work 43 (4): 357–372. doi:10.1093/sw/43.4.357.
- Weeks, J., A. Getis, A. G. Hill, T. Rashed, and M. S. Gadalla. 2004. “The Fertility Transition in Egypt: Intra-Urban Patterns in Cairo.” Annals of the Association of American Geographers 94: 74–93. doi:10.1111/j.1467-8306.2004.09401005.x.
- Woodroffe, R., S. Thirgood, and A. Rabinowitz. 2005. People and Wildlife, Conflict or Co-Existence? edited by R. Woodroffe, S. Thirgood, and A. Rabinowitz. Cambridge, UK: Cambridge University Press.
- Wunder, S. 2007. “The Efficiency of Payments for Environmental Services in Tropical Conservation.” Conservation Biology 21: 48–58. doi:10.1111/cbi.2007.21.issue-1.
- Wunder, S. 2008. “Payments for Environmental Services and the Poor: Concepts and Preliminary Evidence.” Environment and Development Economics 13: 279–297. doi:10.1017/S1355770X08004282.
- Xu, Z. G., J. T. Xu, X. Z. Deng, J. K. Huang, E. Uchida, and S. Rozelle. 2006. “Grain for Green versus Grain: Conflict between Food Security and Conservation Set-Aside in China.” World Development 34: 130–148. doi:10.1016/j.worlddev.2005.08.002.
- Yin, R., T. Liu, S. Yao, and M. Zhao. 2013. “Designing and Implementing Payments for Ecosystem Services Programs: Lessons Learned from China’s Cropland Restoration Experience.” Forest Policy and Economics 35: 66–72. doi:10.1016/j.forpol.2013.06.010.
- Zbinden, S., and D. R. Lee. 2005. “Paying for Environmental Services: An Analysis of Participation in Costa Rica’s PSA Program.” World Development 33: 255–272. doi:10.1016/j.worlddev.2004.07.012.
- Zheng, H., B. E. Robinson, Y.-C.. Liang, S. Polasky, D.-C. Ma, F.-C. Wang, M. Ruckelshaus, Z.-Y. Ouyang, and G. C. Daily, 2013. "Benefits, Costs, and Livelihood Implications of a Regional Payment for Ecosystem Service Program." Proceedings of the National Academy of Sciences 110 (41): 16681-16686. doi:10.1073/pnas.0813417106
- Zilberman, D., L. Lipper, and N. McCarthy. 2008. “When Could Payments for Environmental Services Benefit the Poor?” Environment and Development Economics 13: 255–278. doi:10.1017/S1355770X08004294.