ABSTRACT
Forest stand structure is not only a crucial factor for regulating forest functioning but also an important indicator for sustainable forest management and ecosystem services. Although there exists a few national/global structure databases for natural forests, a country-wide synthetic structure database for plantation forests over China, the world’s largest player in plantation forests, has not been achieved. In this study, we built a country-wide synthetic stand structure database by surveying more than 600 peer-reviewed literature. The database covers tree species, mean stand age, mean tree height, stand density, canopy coverage, diameter at breast height, as well as the associated ancillary in-situ topographical and soil properties. A total of 594 published studies concerning diverse forest stand structure parameters were compiled for 46 tree species. This first synthesis for stand structure of plantation forests over China supports studies on the evolution/health of plantation forests in response to rapid climate change and intensified disturbances, and benefits country-wide sustainable forest management, future afforestation or reforestation planning. Potential users include those studying forest community dynamics, regional tree growth, ecosystem stability, and health, as well as those working with conservation and sustainable management. This dataset is freely accessible at http://www.doi.org/10.11922/sciencedb.j00076.00091.
1. Introduction
Plantation forests are typically characterized by single dominant species and even-aged trees, as well as regularly spaced stands (FAO, Citation2006). They provide crucial ecosystem services at various spatial scales by reducing sediment, sandstorm, and soil erosion, as well as by improving water quality, promoting timber stocks, and carbon sequestration. At the same time, they play an important role in land-atmosphere biogeochemical and biophysical interactions and feedbacks (Beer et al., Citation2010; Crowther et al., Citation2015; Pan et al., Citation2011).
China has launched several afforestation programs since the end of the 1970s (Cao, Sun, et al., Citation2011a; Zhang et al., Citation2016). With more than 79 million hectares, China has the largest plantation area in the world (National Forestry and Grassland Administration of China, Citation2019). Meanwhile, the country has committed to conserve and expand forests with the goal of mitigating land degradation, air pollution, and climate change in the coming decades (Chen et al., Citation2019). China’s goal is to increase forest cover to 26% by 2050 through the largest tree-planting programs over the world (Wang, Innes, Lei, Dai, & Wu, Citation2007). The six key forestry programs cover more than 97% of China’s counties and target 76 million hectares of land for afforestation (Wang et al., Citation2007). Widespread afforestation in China is playing an increasingly significant role in global greening trend and CO2 mitigation (Chen et al., Citation2020, Citation2019). Additionally, afforestation tends to neutralize soil pH and has important cascading effects on terrestrial ecosystem structure and function (Hong et al., Citation2018).
Much of the initial concerns in the last two decades focused on the optimization of the efficiency of wood production, mostly for timber, pulp, and fuel (Carnus et al., Citation2006) in the case of commercial forests; or focused on the maximization of forest cover in the case of protection forest. This notion forms regular planting patterns, without considering the consequently natural succession of plantation ecosystems. Compared with natural forests, plantation forests lack intrinsic self-regulation, and generally have lower species diversity, less resistance to diseases (Pautasso, Holdenrieder, & Stenlid, Citation2005) and insect pest attack (Jactel, Brockerhoff, & Duelli, Citation2005). Over recent years, awareness has been raised concerning the unintended environmental, ecological, and socio-economic consequences triggered by plantation forests (Cao, Chen, et al., Citation2011; Cao, Sun, et al., Citation2011). In some cases, this massive “greening” effort has been less effective than expected, and has failed to achieve the desired ecological benefits (Cao, Sun, et al., Citation2011), due mainly to inappropriate afforestation strategy and management (e.g. species selection, stand density setting). Given the limited and slow improvement of species diversity management in plantations due to both economic and technological constraints, a systematic understanding of the resistance and resilience of plantations in response to climate perturbation and intensified disturbance is necessary. This need together with the need for an accurate assessment of their health, emerges as a core concern for both the scientific community and the administrative sector.
These newly planted forests not only contribute to increased carbon storage and forest cover but also alter local/regional albedo and turbulent energy fluxes, which offers additional local/regional climate feedback (Li et al., Citation2018; Peng et al., Citation2014). Complex and non-linear interactions between plantations and the atmosphere have been reported. These affect land-atmosphere energy exchanges, hydrological cycle and atmospheric composition through physical, chemical, and biological processes (Bonan, Citation2008; Forzieri, Alkama, Miralles, & Cescatti, Citation2017; Li et al., Citation2018; Peng et al., Citation2014). The stability and resilience of plantations in response to rapidly changing climates, combined with other disturbances including fire, pest/pathogen attacks, climate extremes, and management presents a major challenge (Sun et al., Citation2018a; Rowland et al., Citation2015; Allen et al., Citation2010).
One main constraint for our comprehensive understandings of changes in both the productivity and health/resilience of plantation ecosystems in face of a rapid changing environment is the lack of detailed forest stand structure information, including the community traits (e.g. tree species, mean stand age, stand density, mean tree height, canopy coverage, etc.), and the ancillary topographic and soil properties. This constraint prohibits us from achieving a comprehensive picture of vulnerability of plantation forests in response to a warmer and more extreme climate regime. A key question to be answered is how forest stand structure contributes to the climate response/sensitivity of the forest growth/health.
Forest stand structure is a useful indicator for assessing the functioning and health status of both natural and planted forests (Bell et al., Citation2014; Dobrowski, et al., Citation2015; Millar & Stephenson, Citation2015; McIntyre et al., Citation2015; Pan, McCullough, & Hollinger, Citation2018; Zhang, Huang, & He, Citation2015; Zhou et al., Citation2006). Choosing the right species is an important precondition to sustain the stability and resilience of the forest ecosystem (Paquette, Vayreda, Coll, Messier, & Retana, Citation2018; Van de Peer, Verheyen, Kint, Cleemput, & Muys, Citation2017). In China, only a small range of tree species have been used for afforestation despite the highly variable climatic/site conditions (Liu, Li, Ouyang, Tam, & Chen, Citation2008). The plantations constructed so far are dominated by monoculture forest. Single-species dominant plantation forests are reported to be vulnerable and susceptible to both climate variations and multiple disturbances, due to the lack of enough diversity-mediated compensation effects on ecosystem stability (Musavi et al., Citation2017). High planting density has also been encouraged, and no timely thinning due to a long-term strict log ban policy has been implemented, to quickly purchase a high forest cover and/or high timber stock, which unfortunately could be approaching or even beyond the sustainable limit for effective vegetation evolution, as exemplified in the dryland region of the Loess Plateau (Feng et al., Citation2016; Zhang et al., Citation2018). Tree age and stand density are significant factors influencing the productivity of plantations (Pei, Lei, Xiang, Ouyang, & Xu, Citation2018) and can also be meaningful metrics to guide forest management practices (Sun et al., Citation2018b). Diameter at breast height (DBH), tree height, and forest canopy are informative parameters to characterize the health status of trees/forest, as well as the main indicators for biomass calculation (Dong, Zhang, & Li, Citation2016; Wang, Zhao, Liu, Yang, & Teskey, Citation2018). The ancillary topographical factors (altitude, slope, and aspect) affect the productivity of plantations through controlling microclimate (Ren, Dai, Yang, Ma, & Zou, Citation2018; Xu, Zhang, Chen, Zhu, & Kang, Citation2017), while soil factors are closely related to nutrients (e.g. C, N, P, etc.) and water cycles, which in turn regulates tree growth in plantations (Cai et al., Citation2018). Taken together, these parameters formed the core stand structure traits of plantations, which can affect the plantation functioning and ecosystem services (Lin et al., Citation2021). However, little is known about the spatial patterns in stand structure for these new planted forests over China, and its consequences for the functioning and future evolution of these ecosystems.
Several methods can be used to retrieve forest stand structures, including field survey, remote sensing monitoring, and data-model integration. However, none of them can provide enough and accurate data at large spatial scales (e.g. nationwide) due to specific limitations (Glick et al., Citation2016; Fang et al., Citation2013). The sample survey is relatively simple to implement and has been widely used for forest stand structure investigation at local and regional scales (Fang et al., Citation2001). However, this method is usually time and manpower costing. Remote sensing monitoring, particularly the rapid development of LiDAR system, emerges as an effective tool for investigating the forest stand structure across diverse spatial and temporal scales. However, restricted by the perturbations of remote sensing signals and the uncertainties in key parameters in remote sensing inversion models (Donoghue, Watt, Cox, & Wilson, Citation2007; Fagan et al., Citation2018), this method is still limited for application at large scales. Data-driven model simulations for specific structure parameters are susceptible to multiple constraints, including tree species, model structures, spatial heterogeneity, and forcing data availability.
Alternatively, meta-analysis has become popular in the few last decades. Meta-analysis involves statistical analysis of large collection of information from existing studies for the purpose of integrating the findings. Reasons for the increasing popularity of these approaches are that it can make up for some constraints (such as time or object) with a large number of observations and can reconcile the observations of specific conditions (Fleiss & Gross, Citation1991; Wang, Fan, Jing, Cheng, & Ruan, Citation2016). Here, we construct a nation-wide database with multiple forest stand structure parameters for Chinese plantations, and aim to provide a sound and solid basis for forest stand structure and its linkage to forest functioning analysis, which will in return aid our understanding of these unique and rapidly changing ecosystems.
2. Materials and methods
2.1. Data sources
A meta-analysis approach was applied in this study to obtain the basic and harmonized stand structure parameters for plantation forests at national scale. We firstly conducted literature survey by Boolean retrieval from multiple sources including CNKI (China National Knowledge Infrastructure), Web of Science, and Google Scholar, by any combination of Chin* and plantation* or artificial forest* or planted forest*, with one or more keywords as followings, including tree age, canopy/tree height, canopy coverage, diameter at breast height (DBH), stand/forest density. A total of 1,152 literature published between 1948 and 2018, with 35% and 53% within the period of 2000–2010 and 2011–2018, respectively, were originally obtained ().
2.2. Data processing
The obtained literature were further screened to meet with the following criteria: 1) observations for natural forests and mixed forests (for comparison study in some cases) were excluded; 2) with relatively precise records for geographical locations of study plots (normally with size of 30 m × 30 m or 25 m × 25 m, which is individual study dependent), that is with precise coordinates or locating in a relatively small range with the latitudinal and longitudinal ranges <30”; 3) observations from forest stands suffering from obvious human disturbances (including harvesting, fire, fertilization, managed thinning, and recruitment, etc.) are excluded; 4) records for plots from marginal of forest patches are further excluded by visual interpretation on Google Earth (https://www.google.com/earth/). Consequently, the remaining studies are with accurate geographical locations, concrete tree species, least human disturbances, and good-quality records for core stand structure variables as well as the ancillary soil and topographical properties at plot scale. After filtering, we recorded 4,721 observations for 16 core forest stand structure parameters from 594 studies. The ancillary topographical and soil properties were accordingly extracted for all the chosen sites.
The selected studies were further stratified into three levels according to the accuracy in records of both geographical locations of study plots and the corresponding observations of structure parameters (). The Level One is named as “most accurate,” that is, the sampling plots are with precise geographical coordinates, and the corresponding core structure parameters are clearly recorded. The Level Two is named as “moderate accurate.” There are two cases in Level 2. One has precise geographical location information but has multiple records for specific targeting stand structure parameters. The other one lacks precise geographical location information (i.e. not showing the precise coordinates for plots rather than a small region), but documents the sole record for each of the targeting structure parameters. The Level Three is named as “less accurate.” In this case, the studies do not provide precise geographical location rather than a small range (<30”), and there are multiple records (attributed to survey of multiple plots within a small study region) for each of the targeting structure parameters. For cases without precise geographical coordinate records, Google Earth was used for visual interpretation to determine the geographic coordinates manually as the center of the small study region. For those studies with multiple records for each of the targeted structure parameters, the mean value for the core parameter was calculated, and it is then regarded as the representative stand structure variable in this small study site/region (). Notably, for mean stand age, we first documented the year of field survey for each study. We then unified the mean stand age to the year of 2016, by adding/subtracting the corresponding number of years between the field survey and the year of 2016. Finally, we grouped these diverse records for forest stand structure into three major groups termed as structure variables, soil properties, and topographical parameters ().
Figure 2. Flow chart of data processing and classification. The visual interpretation of forest stands on Google Earth is shown on the right.
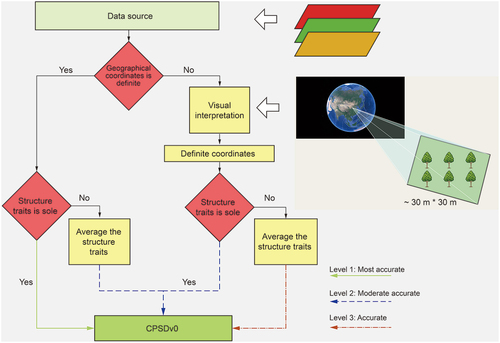
Table 1. Three levels of data quality and the corresponding data processing procedures.
Table 2. The core stand structure variables of plantation forests over China and the ancillary topographical and soil parameters.
3. Results and discussions
Results based on filtered studies are recorded and analyzed. This synthesis represented the forested regions over China well (). In what follows, we show the key statistics of the collected information.
Figure 3. The spatial distribution of major land cover types (a) and the geographical locations of 594 studies involved in our final analyses (b). Different planting tree species were marked by different colors. The major land covers are derived from Moderate Resolution Imaging Spectroradiometer dataset (MCD12Q1, https://e4ftl01.cr.usgs.gov/MOTA/MCD12Q1.051/).
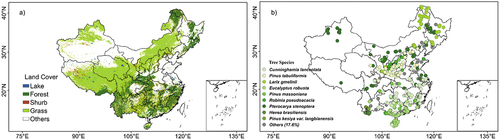
3.1. Stand structure traits
This dataset compiles six most important, widely-used, and easily accessible stand structure variables, including planting tree species, mean stand age, stand density, canopy coverage, tree height, and diameter at breast height (DBH).
3.1.1. Tree species
This dataset covers 46 tree species. The spatial distribution of the top nine widespread tree species on record is displayed in . The tree species of Cunninghamia lanceolata has the largest number of observations (~20.0%) among all tree species, followed by Pinus tabuliformis (10.8%), Larix gmelinii (10.8%), and Eucalyptus robusta (9.3%).
3.1.2. Mean stand age
Tree age is an important factor in regulating forest growth and productivity. Previous studies have found that the productivity of plantations grows rapidly at a young age, but slows down or even declines at a mature age (Zhang et al., Citation2018). However, a comprehensive synthesis of mean stand age for Chinese plantations across diverse bioclimatic regions has until now not been achieved.
In our synthesis, we normalized mean stand age records to the year of 2016 for all observations. According to the classification criteria of forest growth stages of the State Forestry Administration of China (Citation2018), we grouped the Chinese plantations into three major growth stages, including immature, mature, and over-mature ()). Our results clearly show an obvious latitudinal pattern in mean stand age, with younger plantations found in the south (<40° N) and older plantations in the north (>40° N), respectively ()), which may be attributed to differences in tree species planted, and the corresponding life cycles dependent on the targeted service of plantations. Normally, plantations in northern China are targeting towards environmental protection and ecological conservation, whereas the multilateral functions of plantations in southern China include wood production besides the ecological benefits. Most of the planting species in southern China were fast-growing economic species targeting both wood production and ecological restoration with short and rapid growth cycle, while plantations across northern China benefited from rigorous protection policies.
Figure 4. The spatial distribution of the mean stand age of plantations over China, grouped according to the age classes of major tree species and the classification standards by the State Forestry Administration of China (Citation2018).
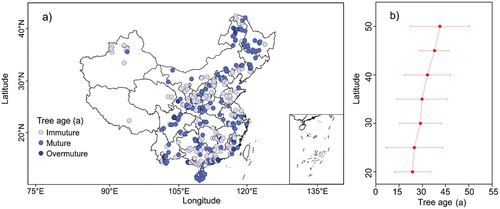
3.1.3. Stand density
The stand density plays a crucial role in shaping the community structure (Pretzsch, Biber, Schütze, Uhl, & Rötzer, Citation2014) and affecting energy partitioning, recruitment, and water/nutrient uptake competition of forest ecosystems (Kerhoulas, Kolb, & Koch, Citation2013), and thus exert significant impacts on forest productivity/growth and climate vulnerability (D’Amato, Bradford, Fraver, & Palik, Citation2013; Giuggiola, Bugmann, Zingg, Dobbertin, & Rigling, Citation2013; McDowell & Allen, Citation2015; Pillet et al., Citation2018; Zhang et al., Citation2015). Stand density is also a core parameter in nearly all kinds of Dynamic Global Vegetation Models (DGVMs) to simulate the future growth and evolution of forest ecosystems (Bellassen, Le Maire, Dhôte, Ciais, & Viovy, Citation2010; Sato, Kobayashi, & Delbart, Citation2010). Based on our synthesis, the mean stand density is 1717 ± 1026 trees/ha, ranging from 33 to 6947 trees/ha across diverse plantations over China (). Different from the tree age, the stand density did not show a clear latitudinal gradient, that is, there is no obvious increasing or decreasing trend in stand density along with increasing latitudes over China ()). Relatively higher stand density was commonly found in mountainous regions, including the Great Xing-Zan Mountains in northern China, Qin Mountain in central China and mountains in southeastern China ()).
Figure 5. The spatial pattern in stand density for Chinese plantations (a) and the correspondingly latitudinal pattern in stand density shown in latitudinal interval of five degree (b). The inlet in a) shows the frequency distribution of stand density (green bars) and the fitted probability density function (dark orange line).
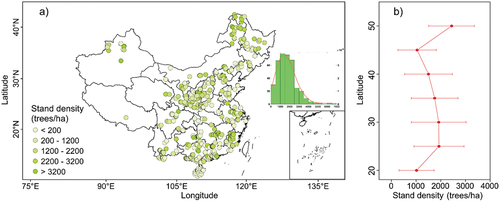
3.1.4. Canopy coverage, DBH, and tree height
Canopy coverage, mean DBH, and mean tree height are also core parameters in shaping forest stand structure (Klein, Randin, & Körner, Citation2015; Tao, Guo, Li, Wang, & Fang, Citation2016) and act as critical parameters for DGVMs and ecosystem models at different scales (Hurtt et al., Citation2004). Nevertheless, demographic traits and processes are not well parameterized and validated in many DGVMs and ecosystem models, given that such kind of data with high accuracy is hard to obtain, particularly at national and continental scales. The mean canopy coverage for plantation forests over China is 68% ± 16%, ranging from 30% to nearly 100%, despite the great spatial variations. The mean DBH and tree height are 15.0 ± 8.4 cm and 11.5 ± 5.9 m across Chinese plantation forests, respectively ().
3.2. Topographic parameters
The most important topographic parameters recorded in field survey include elevation, slope, and aspect. In total, we documented 391, 236, and 134 records for stand elevation, slope, and aspect from literature survey. Elevation records are more than that for slope and aspect. Chinese plantations are distributed at elevations ranging from c. 4 m to 3750 m. The plantations at higher elevations (e.g. > 1212 m of 75th percentile of all elevation records) were mainly distributed in mountainous regions at central and southwest China (). The Chinese plantations were distributed in locations with slopes ranging from 2.3° to 65.0° (). Aspect of forest stands could exert important influence on local energy balance and hydrothermal conditions which play crucial role for tree growth/productivity, ecohydrological processes, and biophysical feedbacks (Bale, Williams, & Charley, Citation1998). The records of stand aspect show that a large part (52%) of Chinese plantation forests are planted on the southeast, southwest and northwest slopes, followed by north and northeast ().
3.3. Soil properties
Ancillary soil properties, including the soil organic carbon content (SOC), total nitrogen, phosphorus, and kalium content (TN, TP, TK, respectively), available nitrogen, phosphorus, and kalium concentrations (AN, AP, and AK, respectively), as well as soil pH value, were also collected when available in the selected studies. Soil nutrients and pH play an important role in affecting forest growth and an important linkage with lithospheric cycles. The abovementioned soil properties were used to characterize the background soil conditions for plantations. However, the records for these soil properties are much less than other variables. Among these soil properties, TN, TP, and AP show strong aggregation in data distribution, in other words, these three indicators show relative consistency in content across different study sites.
3.4. Uncertainty, validation, and application of the database
This is the first synthesis, as far as we know, for forest stand structures of plantations over the whole of China, which is the world’s largest body of plantations with areas of more than 79 million hectares. Although we screened and processed the data records for forest stand structure traits from diverse individual studies strictly by meeting several rigorous criteria, there undoubtedly remains some uncertainties in this dataset that need further improvement.
Firstly, about 59% of the studies collected lack the precise geographical coordination information. Although we adopted a two-step rigorous procedure (in detail see data processing section, ) to identify the geographical locations of these study sites, this estimation approach could inevitably introduce some biases into the geographical coordinates of these study plots.
Second, the dataset was obtained from individual studies of plantations across the whole of China. These study sites could to varying degree suffer from some human disturbances such as logging, fire, fertilization, and silvicultural thinning, although we have tried to filter those studies with obvious records of any kind of human disturbances and discarded those study sites (e.g. those close to roads and settlements). But this filtering can only be done for the current situation, as we know little about the historic features in human disturbances. Silvicultural management such as partial logging, thinning, and fertilization potentially exist, and it will have a direct impact on the stand density and soil nutrients (Huang et al., Citation2013; Merino et al., Citation2005). However, we did not include these specific human managements into this forest structure dataset, due to the difficulties to record such information in a consistent way.
Thirdly, some uncertainty could also come from the problem of time period covered by different studies. We collected different experimental studies for forest stand structures of plantations performed in different years (depending on the field survey implemented in each study). This could introduce some bias into the core structure variables, which could temporarily change along with forest growth. This issue should not be critical for the ancillary variables concerning the topographical and some soil properties (e.g. TN and TP), which should not change significantly in such a short period.
Additionally, we further compared the stand density from our database with that derived from another independent global forest density dataset (Crowther et al., Citation2015), although our dataset solely focuses on plantations and not natural forests over China. Our comparison shows that results from two empirical statistical models (ecoregion-scale model and biome-level model, in detail see Crowther et al., Citation2015) largely underestimated the stand density for plantations of major tree species across China (). The global tree density map (Crowther et al., Citation2015) estimated that stand density for the top nine tree species ranges from 4 trees/ha to 876 trees/ha from biome-level models (ranging from 5 trees/ha to 1972 trees/ha from ecoregion-scale models), whereas our dataset recorded the corresponding stand density ranging from 37 trees/ha to 6947 trees/ha (, ).
Figure 10. Scatter plot of stand density derived for CPSDv0 dataset and another independent global stand density dataset (Crowther et al., Citation2015). We compared the stand density from CPSDv0 and that calculated using Ecoregion models (a) and biome-level model (b), respectively in (Crowther et al., Citation2015).
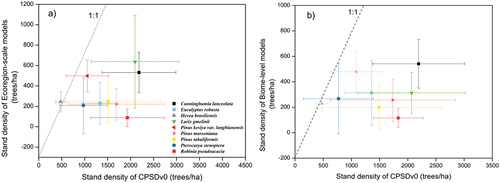
Albeit these potential uncertainties, this new and unique database of forest stand structure for Chinese plantations (Wu et al., Citation2020) will benefit comprehensive understandings of the biochemical and biophysical functioning of these man-made ecosystems, by linking the detailed records of forest stand structure to either national forest biomass inventory or regional scale land-atmosphere coupling modelling (Bellassen et al., Citation2010; Carnicer et al., Citation2011; Seidl, Schelhaas, & Lexer, Citation2011; Wolf et al., Citation2011). This high-quality, multiple-trait structure database could also provide valuable insight into sustainable forest management, particularly when facing a continuing trend towards more disturbance-prone conditions (Seidl et al., Citation2011).
First, our synthesis provides a fundamental and solid database of forest stand structures at plot scales with sizes of c. 30 m × 30 m or c. 25 m × 25 m but covering the majority of the forested areas over the whole China. This dataset can not only be used to formulate management plan for local afforestation practice, but also contribute with added valuable to the existing global datasets of forest structure (Hansen et al., Citation2013), like the global database of tree height and stand density (Crowther et al., Citation2015; Hansen et al., Citation2013).
Second, due to the extensive representation of diverse tree species and bioclimatic zones, this dataset can not only be applied to parameterize demographic processes in dynamic vegetation models, whereas this process has not been well embedded and validated due partly to limited data availability, but also be applied to other research for a variety of eco-physiological processes. For example, users can explore whether taller and older plantations play different roles in carbon storage and whether older trees are less/more sensitive to climate change (Carrer & Urbinati, Citation2004; Zhou et al., Citation2006). The documented stand density, canopy coverage, together with other ancillary information, can be potentially applied to capture the features in community competition and disentangle its impacts on forest growth (Gómez-aparicio, García-valdés, Ruíz-benito, & Zavala, Citation2011), carbon sequestration, water consumption, as well as forest health facing the rapid climate change (McDowell & Allen, Citation2015; McIntyre et al., Citation2015; Musavi et al., Citation2017).
Finally, this dataset provides a valuable basis for linking plot-scale forest stand structure traits, with high-resolution remote sensing derived products, such as vegetation indices (e.g. NDVI, EVI), vegetation productivity (e.g. SIF, NPP), biophysical parameters (e.g. land surface temperature, albedo, roughness), given that we clearly constrained/identified the spatial scale of individual studies in data surveying. Thus, this dataset has a great potential for studying forest structure-functioning and its climate effects at regional/national scale. It is also useful for data-model integration, multiple data streams inference, as well as land-atmosphere coupled model parameterization and validation.
4. Conclusions
In summary, we for the first time compiled a forest stand structure dataset for plantations of 46 tree species over the whole of China by surveying more than 600 peer-reviewed literature from multiple data sources. In this dataset, six most important forest stand structure traits, including dominant tree species, mean stand age, mean tree height, stand density, canopy coverage, and mean DBH, were systematically compiled, together with three topographical and seven soil biochemical parameters. This dataset provides an easily accessible and solid footstone of forest stand structure for dominant plantations and will thus facilitate prospective structure-based studies for forest dynamics/health and its biochemical and biophysical climate feedback. Aside from the potential applications in community structure studies, we also expect this freely accessible dataset to be broadly applicable to different research fields concerning land surface processes and land-atmosphere interactions, as well as for combining datasets from multidisciplinary fields such as ecology, hydrology, climate, and remote sensing. Finally, this advanced information about the forest stand structure of Chinese plantations provides critical ecological insights and opportunities for sustainable afforestation planning and management as well as sustainable ecosystem services.
Disclosure statement
No potential conflict of interest was reported by the author(s).
Data availability statement
The forest stand structure database of plantations in China is openly accessible at http://www.doi.org/10.11922/sciencedb.j00076.00091, https://doi.org/10.5281/zenodo.3731928, or alternatively at the Regional Climate Group of the Department of Earth Sciences, University of Gothenburg (http://rcg.gvc.gu.se/dc/, under LINKS directory).
Correction Statement
This article has been republished with minor changes. These changes do not impact the academic content of the article.
Additional information
Funding
Notes on contributors
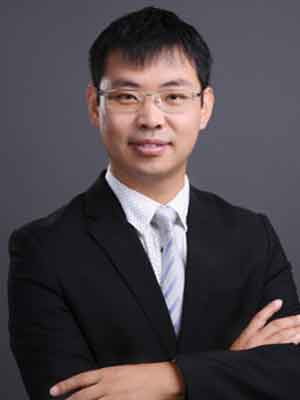
Xiuchen Wu
Xiuchen Wu is now a Professor of Faculty of Geographical Science, Beijing Normal University. He got a Ph.D. degree in 2011 from Peking University. He has postdoc research experience in both the Max-Planck-Institute of Biogeochemistry in Germany and the Laboratory for Sciences of Climate and Environment in France. His main research interests include the terrestrial-atmosphere interaction, climate change and forest resilience, extreme climate and ecosystem functioning, as well as climate feedback. He is in charge of some projects regarding the drought responses of vegetation growth from both NSFC and the Ministry of Science and Technology of China.
References
- Allen, C.D., Macalady, A.K., Chenchouni, H., Bachelet, D., McDowell, N., Vennetier, M., and Cobb, N. (2010). A global overview of drought and heat-induced tree mortality reveals emerging climate change risks for forests. Forest Ecology and Management, 259(4), 660–684.
- Bale, C. L., Williams, J. B., & Charley, J. L. (1998). The impact of aspect on forest structure and floristics in some Eastern Australian sites. Forest Ecology and Management, 110(1–3), 363–377.
- Beer, C., Reichstein, M., Tomelleri, E., Ciais, P., Jung, M., Carvalhais, N., … Papale, D. (2010). Terrestrial gross carbon dioxide uptake: Global distribution and covariation with climate. Science, 329(5993), 834–838.
- Bell, D. M., Bradford, J. B., & Lauenroth, W. K. (2014). Forest stand structure, productivity, and age mediate climatic effects on aspen decline. Ecology, 95(8), 2040–2046.
- Bellassen, V., Le Maire, G., Dhôte, J. F., Ciais, P., & Viovy, N. (2010). Modelling forest management within a global vegetation model—Part 1: Model structure and general behavior. Ecological Modelling, 221(20), 2458–2474.
- Bonan, G. B. (2008). Forests and Climate Change: Forcings, Feedbacks, and the climate benefits of forests. Science, 320(5882), 1444–1449.
- Cai, X., Lin, Z., Penttinen, P., Li, Y., Li, Y., Luo, Y., et al (2018). Effects of conversion from a natural evergreen broadleaf forest to a Moso bamboo plantation on the soil nutrient pools, microbial biomass and enzyme activities in a subtropical area. Forest Ecology and Management, 422, 161–171.
- Cao, S., Chen, L., Shankman, D., Wang, C., Wang, X., & Zhang, H. (2011). Excessive reliance on afforestation in China’s arid and semi-arid regions: Lessons in ecological restoration. Earth-Science Reviews, 104(4), 240–245.
- Cao, S., Sun, G., Zhang, Z., Chen, L., Feng, Q., Fu, B., … Wei, X. (2011). Greening China naturally. AMBIO, 40(7), 828–831.
- Carnicer, J., Coll, M., Ninyerola, M., Pons, X., Sanchez, G., & Penuelas, J. (2011). Widespread crown condition decline, food web disruption, and amplified tree mortality with increased climate change-type drought. Proceedings of the National Academy of Sciences, 108(4), 1474–1478.
- Carnus, J. M., Parrotta, J., Brockerhoff, E., Arbez, M., Jactel, H., Kremer, A., … Walters, B. (2006). Planted forests and biodiversity. Journal of Forestry. 104(2), 65–77.
- Carrer, M., & Urbinati, C. (2004). Age-dependent tree-ring growth responses to climate in Larix decidua and Pinus cembra. Ecology, 85(3), 730–740.
- Chen, C., Park, T., Wang, X., Piao, S., Xu, B., Chaturvedi, R. K., & Myneni, R. B. (2019). China and India lead in greening of the world through land-use management. Nature Sustainability, 2(2), 122–129.
- Chen, Y. Z., Chen, L. Y., Cheng, Y., Ju, W. M., Chen, H. Y. H., & Ruan, H. H. (2020). Afforestation promotes the enhancement of forest LAI and NPP in China. Forest Ecology and Management, 462, 117990.
- Crowther, T. W., Glick, H. B., Covey, K. R., Bettigole, C., Maynard, D. S., Thomas, S. M., & Bradford, M. A. (2015). Mapping tree density at a global scale. Nature, 525(7568), 201–205.
- D’Amato, A. W., Bradford, J. B., Fraver, S., & Palik, B. J. (2013). Effects of thinning on drought vulnerability and climate response in north temperate forest ecosystems. Ecological Applications, 23(8), 1735–1742.
- Dobrowski, S. Z., Swanson, A. K., Abatzoglou, J. T., Holden, Z. A., Safford, H. D., Schwartz, M. K., & Gavin, D. G. (2015). Forest structure and species traits mediate projected recruitment declines in western US tree species. Global Ecology and Biogeography, 24(8), 917–927.
- Dong, L., Zhang, L., & Li, F. (2016). Developing two additive biomass equations for three coniferous plantation species in Northeast China. Forests, 7(12)), 136.
- Donoghue, D. N. M., Watt, P. J., Cox, N. J., & Wilson, J. (2007). Remote sensing of species mixtures in conifer plantations using LiDAR height and intensity data. Remote Sensing of Environment, 110(4), 509–522.
- Fagan, M. E., Morton, D. C., Cook, B. D., Masek, J., Zhao, F., Nelson, R. F., & Huang, C. (2018). Mapping pine plantations in the southeastern U.S. using structural, spectral, and temporal remote sensing data. Remote Sensing of Environment, 216, 415–426.
- Fang, J.Y., Chen, A.P., Peng, C.H., Zhao, S.Q., & Ci, L.J. (2001). Changes in Forest Biomass Carbon Storage in China Between 1949 and 1998. Science, 292(5525), 2320–2322.
- Fang, J.Y., Shen, Z.H., Tang, Z.Y., Wang, X.P., Wang, Z.H., Feng, J.M., and Zheng, C.Y. (2013). Forest community survey and the structural characteristics of forests in China. Ecography, 35(12), 1059–1071.
- FAO. Global planted forests thematic study: Results and analysis. by A. Del Lugo, J. Ball and J. Carle, Planted Forests and Trees Working Paper 38. Rome. (2006).
- Feng, X., Fu, B., Piao, S., Wang, S., Ciais, P., Zeng, Z., … Wu, B.(2016). Revegetation in China’s Loess Plateau is approaching sustainable water resource limits. Nature Climate Change, 6(11), 1019–1022.
- Fleiss, J. L., & Gross, A. J. (1991). Meta-analysis in epidemiology, with special reference to studies of the association between exposure to environmental tobacco smoke and lung cancer: A critique. Journal of Clinical Epidemiology, 44(2), 127–139.
- Forzieri, G., Alkama, R., Miralles, D. G., & Cescatti, A. (2017). Satellites reveal contrasting responses of regional climate to the widespread greening of Earth. Scinence, 356(6343), 1140–1144.
- Giuggiola, A., Bugmann, H., Zingg, A., Dobbertin, M., & Rigling, A. (2013). Reduction of stand density increases drought resistance in xeric Scots pine forests. Forest Ecology and Management, 310, 827–835.
- Glick, H. B., Bettigole, C., Maynard, D. S., Covey, K. R., Smith, J. R., & Crowther, T. W. (2016). Spatially-explicit models of global tree density. Scientific Data, 3(1), 160069.
- Gómez-aparicio, L., García-valdés, R., Ruíz-benito, P., & Zavala, M. A. (2011). Disentangling the relative importance of climate, size and competition on tree growth in Iberian forests: Implications for forest management under global change. Global Change Biology, 17(7), 2400–2414.
- Hansen, M. C., Potapov, P. V., Moore, R., Hancher, M., Turubanova, S. A., Tyukavina, A., … Townshend, J. R. G. (2013). High-Resolution global maps of 21st-Century forest cover change. Science, 342(6160), 850.
- Hong, S. B., Piao, S. L., Chen, A. P., Liu, Y. W., Liu, L. L., Peng, S. S., … Zeng, H. (2018). Afforestation neutralizes soil pH. Nature Communications, 9(1), 520.
- Huang, Z., He, Z., Wan, X., Hu, Z., Fan, S., & Yang, Y. (2013). Harvest residue management effects on tree growth and ecosystem carbon in a Chinese fir plantation in subtropical China. Plant and Soil, 364(1–2), 303–314.
- Hurtt, G. C., Dubayah, R., Drake, J., Moorcroft, P. R., Pacala, S. W., Blair, J. B., & Fearon, M. G. (2004). Beyond potential vegetation: Combining Lidar data and a height-structured model for carbon studies. Ecological Applications, 14(873–883), 873–883.
- Jactel, H., Brockerhoff, E., & Duelli, P. (2005). “A test of the biodiversity-stability theory: Meta-analysis of tree species diversity effects on insect pest infestations, and re-examination of responsible factors,” in forest diversity and function: Temperate and boreal systems (pp. 235–262). (M. Scherer-Lorenzen, C. Körner, & E. Schulze, eds). Berlin, Heidelberg: Springer Berlin Heidelberg.
- Kerhoulas, L. P., Kolb, T. E., & Koch, G. W. (2013). Tree size, stand density, and the source of water used across seasons by ponderosa pine in northern Arizona. Forest Ecology and Management, 289, 425–433.
- Klein, T., Randin, C., & Körner, C. (2015). Water availability predicts forest canopy height at the global scale. Ecology Letters, 18(1311–1320), 1311–1320.
- Li, Y., Piao, S. L., Li, L. Z. X., Chen, A. P., Wang, X. H., Ciais, P., … Zhou, L. M. (2018). Divergent hydrological response to large-scale afforestation and vegetation greening in China. Science Advances, 4(5), eaar4182.
- Lin, S., Li, Y., Li, Y.H., Chen, Q., Wang, Q.L., & He, K.N. (2021). Influence of tree size, local forest structure, topography, and soil resource availability on plantation growth in Qinghai Province, China. Ecological Indicators, 120, 106957, doi:10.1016/j.ecolind.2020.106957
- Liu, J., Li, S., Ouyang, Z., Tam, C., & Chen, X. (2008). Ecological and socioeconomic effects of China’s policies for ecosystem services. Proceedings of the National Academy of Sciences, 105(28), 9477.
- McDowell, N. G., & Allen, C. D. (2015). Darcy’s law predicts widespread forest mortality under climate warming. Nature Climate Change, 5(7), 669–672.
- McIntyre, P. J., Thorne, J. H., Dolanc, C. R., Flint, A. L., Flint, L. E., Kelly, M., & Ackerly, D. D. (2015). Twentieth-century shifts in forest structure in California: Denser forests, smaller trees, and increased dominance of oaks. Proceedings of the National Academy of Sciences, 112(5), 1458–1463.
- Merino, A., Balboa, M.A., Soalleiro, R.R., and Álvarez González, J.G. (2005). Nutrient exports under different harvesting regimes in fast-growing forest plantations in southern Europe. Forest Ecology and Management, 207(3), 325–339.
- Millar, C., & Stephenson, N. L. (2015). Temperate forest health in an era of emerging megadisturbance. Science, 349(823–826), 823–826.
- Musavi, T., Migliavacca, M., Reichstein, M., Kattge, J., Wirth, C., Black, T. A., & Mahecha, M. D. (2017). Stand age and species richness dampen interannual variation of ecosystem-level photosynthetic capacity. Nature Ecology & Evolution, 1(2). doi:10.1038/s41559-016-0048
- National Forestry and Grassland Administration of China. (2019). China Forest Resources Report (2014-2018). Beijing: China Forestry Publishing House.
- Pan, Y., Birdsey, R. A., Fang, J., Houghton, R., Kauppi, P. E., Kurz, W. A., … Hayes, D. (2011). A large and persistent carbon sink in the world’s forests. Science, 333(6045), 988–993.
- Pan, Y., McCullough, K., & Hollinger, D. Y. (2018). Forest biodiversity, relationships to structural and functional attributes, and stability in New England forests. Forest Ecosystems, 5(1). doi:10.1186/s40663-018-0132-4
- Paquette, A., Vayreda, J., Coll, L., Messier, C., & Retana, J. (2018). Climate change could negate positive tree diversity effects on forest Productivity: A study across five climate types in Spain and Canada. Ecosystems, 21(5), 960–970.
- Pautasso, M., Holdenrieder, O., & Stenlid, J. (2005). Susceptibility to fungal pathogens of forests differing in tree diversity,” in forest diversity and function: Temperate and boreal systems (pp. 263–289). (M. Scherer-Lorenzen, C. Körner, & E. Schulze, eds). Berlin, Heidelberg: Springer Berlin Heidelberg.
- Pei, Y., Lei, P., Xiang, W., Ouyang, S., & Xu, Y. (2018). Effect of stand age on fine root biomass, production and morphology in Chinese fir plantations in subtropical China. Sustainability, 10(7), 2280.
- Peng, -S.-S., Piao, S., Zeng, Z., Ciais, P., Zhou, L., Li, L. Z. X., … Zeng, H. (2014). Afforestation in China cools local land surface temperature. Proceedings of the National Academy of Sciences, 111(8), 2915–2919.
- Pillet, M., Joetzjer, E., Belmin, C., Chave, J., Ciais, P., Dourdain, A., … Zhou, S. (2018). Disentangling competitive vs. climatic drivers of tropical forest mortality. Journal of Ecology, 106(3), 1165–1179.
- Pretzsch, H., Biber, P., Schütze, G., Uhl, E., & Rötzer, T. (2014). Forest stand growth dynamics in central Europe have accelerated since 1870. Nature Communications, 5(1). doi:10.1038/ncomms5967
- Ren, J., Dai, W., Yang, C., Ma, X., & Zou, C. B. (2018). Physiological regulation of poplar species to experimental warming differs between species with contrasting elevation ranges. New Forests, 49(3), 329–340.
- Rowland, L., Da Costa, A. C. L., Galbraith, D. R., Oliveira, R. S., Binks, O. J., Oliveira, A. A. R., … Meir, P. (2015). Death from drought in tropical forests is triggered by hydraulics not carbon starvation. Nature, 528(7580), 119–122.
- Sato, H., Kobayashi, H., & Delbart, N. (2010). Simulation study of the vegetation structure and function in eastern Siberian larch forests using the individual-based vegetation model SEIB-DGVM. Forest Ecology and Management, 259(3), 301–311.
- Seidl, R., Schelhaas, M., & Lexer, M. J. (2011). Unraveling the drivers of intensifying forest disturbance regimes in Europe. Global Change Biology, 17(9), 2842–2852.
- State Forestry Administration of China. Regulations for age-class and age-group division of main tree-species. (2018).
- Sun, D., Zhang, W., Lin, Y., Liu, Z., Shen, W., Zhou, L., … Fu, S. (2018a). Soil erosion and water retention varies with plantation type and age. Forest Ecology and Management, 422, 1–10.
- Sun, S., He, C., Qiu, L., Li, C., Zhang, J., & Meng, P. (2018b). Stable isotope analysis reveals prolonged drought stress in poplar plantation mortality of the three-north shelter forest in Northern China. Agricultural and Forest Meteorology, 252, 39–48.
- Tao, S. L., Guo, Q. H., Li, C., Wang, Z. H., & Fang, J. Y. (2016). Global patterns and determinants of forest canopy height. Ecology, 97(12), 3265–3270.
- Van de Peer, T., Verheyen, K., Kint, V., Cleemput, E. V., & Muys, B. (2017). Plasticity of tree architecture through interspecific and intraspecific competition in a young experimental plantation. Forest Ecology and Management, 385, 1–9.
- Wang, D., Fan, J., Jing, P., Cheng, Y., & Ruan, H. (2016). Analyzing the impact of climate and management factors on the productivity and soil carbon sequestration of poplar plantations. Environmental Research, 144, 88–95.
- Wang, G., Innes, J., Lei, J., Dai, S., & Wu, S. (2007). China’s Forestry Reforms. Science, 318(5856), 1556–1557.
- Wang, X., Zhao, D., Liu, G., Yang, C., & Teskey, R. O. (2018). Additive tree biomass equations for Betula platyphylla suk. plantations in Northeast China. Annals of Forest Science, 75(1), 1–9.
- Wolf, A., Ciais, P., Bellassen, V., Delbart, N., Field, C. B., & Berry, J. A. (2011). Forest biomass allometry in global land surface models. Global Biogeochemical Cycles, 25(4), n/a–n/a.
- Wu, X., Jiang, X., Liu, H., Allen, C., Li, X., Wang, P., … Chen, D. (2020). Forest structure database for plantation forests over China-CPSDv0. doi:10.5281/zenodo.3731928
- Xu, H., Zhang, Z., Chen, J., Zhu, M., & Kang, M. (2017). Cloudiness regulates gross primary productivity of a poplar plantation under different environmental conditions. Canadian Journal of Forest Research, 47(5), 648–658.
- Zhang, J., Huang, S., & He, F. (2015). Half-century evidence from western Canada shows forest dynamics are primarily driven by competition followed by climate. Proceedings of the National Academy of Sciences, 112(13), 4009–4014.
- Zhang, S., Yang, D., Yang, Y., Piao, S., Yang, H., Lei, H., … Fu, B. (2018). Excessive afforestation and soil drying on China’s loess plateau. Journal of Geophysical Research: Biogeosciences, 123(3), 923–935.
- Zhang, Y., Peng, C., Li, W., Tian, L., Zhu, Q., Chen, H., … Xiao, X. (2016). Multiple afforestation programs accelerate the greenness in the ‘Three North’ region of China from 1982 to 2013. Ecological Indicators, 61(2), 404–412.
- Zhou, G., Liu, S., Li, Z., Zhang, D., Tang, X., Zhou, C., … Mo, J. (2006). Old-growth forests can accumulate carbon in soils. Science, 314(5804), 1417.