ABSTRACT
Wildlife trafficking is a global phenomenon posing many negative impacts on socio-environmental systems. Scientific exploration of wildlife trafficking trends and the impact of interventions is significantly encumbered by a suite of data reuse challenges. We describe a novel, open-access data directory on wildlife trafficking and a corresponding visualization tool that can be used to identify data for multiple purposes, such as exploring wildlife trafficking hotspots and convergence points with other crime, discovering key drivers or deterrents of wildlife trafficking, and uncovering structural patterns. Keyword searches, expert elicitation, and peer-reviewed publications were used to search for extant sources used by industry and non-profit organizations, as well as those leveraged to publish academic research articles. The open-access data directory is designed to be a living document and searchable according to multiple measures. The directory can be instrumental in the data-driven analysis of unsustainable illegal wildlife trade, supply chain structure via link prediction models, the value of demand and supply reduction initiatives via multi-item knapsack problems, or trafficking behavior and transportation choices via network interdiction problems.
1. Introduction
The illegal trade in, and movement of, wild fauna and flora is a global phenomenon posing direct, as well as second and third order impacts on socio-environmental systems. Wildlife trafficking directly threatens species’ persistence (Guynup et al., Citation2020), socio-environmental security (Dalpane & Baideldinova, Citation2022; Felbab-Brown, Citation2018), and undermines the rule of law (Session, Citation2018). Trafficking harms ecosystem integrity (Sanjurjo-Rivera et al., Citation2021), the viability of nature-based solutions (Price, Citation2018), sustainable use of wildlife, and the carbon capture potential of forests (Hayek et al., Citation2021), while creating new exposure pathways for zoonotic disease transmission (Felbab-Brown, Citation2021), social injustices (Gianopoulos, Citation2020), and exploitation of marginalized groups (Agu & Gore, Citation2020). There are no socio-environmental systems in the world untouched by wildlife trafficking (The World Bank Group, Citation2022). The knowledge base about poaching – an early step in the wildlife trafficking supply chain – is substantial and overwhelmingly case-based (e.g. species and products such as elephant tusks, helmeted hornbills casques) (Vigne & Nijman, Citation2022). The poaching literature elaborates on the drivers of poaching, the social contexts within which poaching occurs, and essential issues of contested legality, fragility and conflict, social and environmental safeguards, the militarization of conservation, and restorative justice.
Scientific exploration of wildlife trafficking trends and the impact of interventions – and drawing of inferences to inform decision-making – is significantly encumbered by a suite of data reuse challenges (Gore et al., Citation2022; The World Bank Group, Citation2022) including the challenge of identifying relevant studies/data sets with reliable and high-quality data that is regularly updated and organized for accessibility. Beyond supporting the need of donors to evaluate, direct, and monitor investments to combat wildlife trafficking and conserve biodiversity, secondary analysis using multiple datasets portends broad application to scientific exploration across disciplines. Secondary data analysis increases the ability of science teams including experts beyond conservation to detect subtle and complex associations, which is not possible with individual, discipline-focused studies (Pan et al., Citation2022). For example, operations researchers could apply data-driven research methods to uncover and understand the supply chain structure, operations, and drivers of these illicit networks to offer insights on network interdiction, allocation of scarce resources, and prediction of adversaries’ behavior (Keskin et al., Citation2022). [Illicit networks can be physical, financial, social, or a combination thereof and the terms illegal and illicit are often used interchangeably. We differentiate illicit as being the preferred broader term. Illegal is an explicit communication of being a violation of a public law. Illicit incorporates the term “illegal” and adds the contexts of a grey area of commerce (Shelley, Citation2018), intentional covertness, and/or often a social perception of right and wrong (Van Schendel & Abraham, Citation2005).] Computer scientists can leverage limited available data about wildlife trafficking routes to predict previously unknown paths that may be used to transport illegally obtained wildlife products. Data scientists can predict illegal fishing and transshipment from ship location data combined with a historical criminal registry and exclusive economic zone data (Miller et al., Citation2018). Finally, computer science has proven useful in detecting wildlife trafficking online (Sharma, Citation2020; Xu et al., Citation2019), and by combining these detections with intervention and demographic information, stakeholders can quantitatively identify what tools will be most effective in different settings. These perspectives can complement existing classical conservation efforts dedicated to protecting species and their habitats (NatureServe, Citation2023).
Identifying the relevant data to include in datasets for secondary analysis from publicly available data repositories is challenging in part because of the unstructured nature of variable descriptions (Pan et al., Citation2022). There remains a lack of data integration across disciplines on current efforts to combat wildlife trafficking, at times because of the diversity of sectors and stakeholders collecting data on different aspects of the problem. The limitation of integrated data decreases researchers’ ability to use analytical methods of pattern recognition, anomaly detection, forecasting, problem-solving, and decision-making in support of efforts to determine the effectiveness of interventions, strengthen wildlife crime investigations, and manage records.
In this paper, we describe a novel directory on wildlife trafficking (available at Zenodo open repository) (Gore et al., Citation2023) and its integration with a corresponding visualization tool that can be used to search for and identify data sources for multiple purposes, such as exploring wildlife trafficking hotspots, finding convergence points with other crime, discovering key drivers or deterrents of wildlife trafficking, and uncovering structural patterns beyond those considered by classical conservation biology. The data directory can be instrumental for data-driven analysis of supply chain structure via link prediction models, the value of demand and supply reduction initiatives via multi-item knapsack problems, or trafficking behavior and transportation choices via network interdiction problems. Our motivation to produce this directory was sparked when we attempted to apply operations research and analytics techniques to the problem of wildlife trafficking and discovered such a database did not exist. We also recognized the fragmented nature of existing data sources and the benefit of augmenting classical conservation biology approaches.
2. Methods
The content of the data directory was informed by snowball keyword searches on Google USA and Google Scholar (Aliyu, Citation2017) and expert elicitation about online datasets and other data sources (Hemming et al., Citation2018). We searched for current tools and data used by industry and non-profit organizations, as well as those leveraged to publish academic research articles (Barrett, Citation1993). To avoid restricting our search to a specific species, we used general terms such as “wildlife,” “flora,” “fauna,” “animal,” “species.” To ensure the data was focused on illegal trade, we ensured at last one of the following terms was included: “illegal,” “illicit,” “illegitimate,” “banned,” “prohibited,” “unsanctioned,” “smuggle” as well as one of these terms “trade,” “sale,” “market,” “exchange.” To avoid confining results to a specific geographic region, we omitted the use of spatially restricting criteria in our search and included one of the following terms in each search: “global,” “world,” “international.” To ensure each of the entries in our directory were explicitly related to illegal wildlife trade, we conducted a cross-check review of all sources with two members of our research term and discarded any extraneous sources. For example, we excluded articles which reused data source data and not modified; in these instances, we included the original source as an entry in the directory. The data directory was designed to be a living directory. We encourage others to contribute new sources of data which are more targeted to a given geographic area, species, or otherwise to facilitate effective collaboration and advancement of research. In this regard, the data directory involves collaborative record-keeping and downstream analysis (Henson et al., Citation2020).
To confirm content was not excluded, the list of sources in the directory was collaboratively reviewed and revised by a larger, multi-disciplinary science team composed of conservation biologists, geologists, supply chain and logistics experts, operations researchers, and computer scientists. With this integrative perspective, the larger research team identified the most appropriate descriptive information to include with each data source and its corresponding title. This content-derivation process encouraged us to generate an open-access directory with a searchable visualization (Ferber et al., Citation2023) to allow any online user to search and filter information based on data source categorization, data type, accessibility, geography, and species of flora or fauna. We ultimately organized the directory along dimensions identified as useful for researchers seeking to understand wildlife trafficking in a network context AND for which information across all sources. In this regard, the organizational schema for the directory was restricted by the content of the sources. Although researchers may desire information, it may not be available. The data were organized according to the type of data (e.g. article, dashboard), its accessibility for researchers (e.g. limited access, publicly available), and relevant disciplinary categories (e.g. geospatial, legal) (). The disciplinary category serves several purposes, firstly to record which disciplines have found these datasets useful in the past (not sure about this), as well as to help point researchers in discrete disciplines to datasets that might be immediately useful for them. The level of disaggregation was intended to support diverse researchers and stakeholders accessing our directory and attempting to avoid domain-specific terminology or jargon (e.g. deep learning, inverse optimization, recidivism).
Table 1. Records in the directory on worldwide wildlife trafficking are alphabetically organized by data types. Records were assigned one of the eight data types.
Table 2. Records in the directory on worldwide global wildlife trafficking are alphabetically organized by degree of accessibility. Records were assigned into one of the five degrees of accessibility.
Table 3. Records in the directory on worldwide wildlife trafficking are alphabetically organized by source categories. Records were assigned one of the 11 data categories.
Expert elicitation about data sources (Hemming et al., Citation2018) and interdisciplinary team science principles (Henson et al., Citation2020) were used to validate the technical quality of the directory. Computer scientists, operations engineers, supply chain management, conservation biology, and human geography faculty, graduate, and undergraduate students at five large research universities in the United States independently and collectively reviewed database content and organizational structure over multiple Zoom meetings. When inconsistencies, logical fallacies, or misunderstandings were identified during a meeting, collective revisions to the directory were made. A final test was completed and published by computer science and operations research scientists using sources noted in the data directory to analyze wildlife trafficking supply chains (Gore et al., Citation2023).
3. Data records
Our directory for worldwide wildlife trafficking is designed to be searchable according to multiple measures. The data are available at https://tinyurl.com/mr2bh8uk.Records and are organized according to species of flora and fauna as defined by the International Union for Conservation of Nature (IUCN Red List, Citationn.d.) and as modified by the Intergovernmental Platform on Biodiversity and Ecosystem Services. Geography is organized according to the United Nations Geoscheme (EMIM, Citationn.d.) which divides 249 countries and territories in the world into 6 regional, 17 subregional and 9 sub-regional groups. Data records are labeled as “global,” permitting they included at least one country from each continent. We labeled data records as “searchable” when they did not retain a visible collection of species or countries, and instead required input. Records labeled as “n/a” indicate the species or countries are not explicitly listed. Records are organized according to 8 data types (), 5 degrees of accessibility (), and 11 data categories ().
4. Discussion
Upon aggregating the data sources and descriptive information into a shared Microsoft Excel spreadsheet, we sought an option for cloud-based data aggregation and visualization. We uploaded the directory into Power BI because the platform enables broad data accessibility and analysis for diverse professionals and academics (Keskin et al., Citation2022). Compared to other types of data visualization software, our team found Power BI easy to integrate with Microsoft Office. Knowing many people use Microsoft Office, we felt this option would be accessible to a wide range of users and help minimize potential data loss due to compatibility with Excel, which was the original format of the data directory. Power BI has been used in prior research focused on illegal wildlife trade, primarily as a visualization tool for mapping import and export data of wild flora and fauna (Keskin et al., Citation2022). In this way, Power BI is well equipped to maximize the use of geospatially enabled data, as it can process inputs from software such as R, a GIS, and other spatial files to ease accessibility and shareability. This capability allows multiple types of data sources, as well as visualization by relying on the geography field as input.
Our science team found Power BI useful; it allowed for efficient searching and filtration of a central database to narrow down choices for a specific purpose (e.g. all the five sources with “on request” data, how many include reptiles?). Power BI enabled embedding of multiple hyperlinks, thus maximizing the potential for continuity of accessing external data sources. For instance, operations research and supply chain management researchers may desire more quantitative seizure data at ports for interdiction purposes, while the criminology researchers may need qualitative interview responses from convicted traffickers found in reports. Conservationists may focus on specific species or products. Given that global wildlife trafficking data remains disparate, minimally interoperable and of varied quality, the research desires are multi-faceted. It is crucial to have a main directory that centralizes information used by the relevant disciplines if we wish to encourage interdisciplinary and cross disciplinary research on wildlife trafficking. Search and filtration functionalities become a necessity with the respective focus areas of an interdisciplinary research team.
Power BI’s drag-and-drop feature was used to place the data sources from the Fields pane into the Filters pane of the Report. This created a table depicting only the selected data associated with the selected fields queried by a user. This table is in Power BI’s report space, which is where the resulting data from the selected fields can be viewed. There are multiple search boxes located in the Filters pane on the right-hand side of the report. The boxes search for entered strings in each column assigned to a data field across the table. The Basic filter function allows a user to search a singular term. Using this function, the boxes with checkmarks below the search boxes are assigned to each column of the table to allow a user to filter or search within a single column. The results of these search and filter options are a viewable table of specific data sources that a user can then access via the data directory (). Alternatively, the Advanced filtering function allows a user to create a string of terms, which are connected via multiple keywords (i.e. AND, OR) or specifications (e.g. contains, does not contain, starts with, does not start with). While using the Advanced filtering function, users are able to explicitly filter by multiple subcategories of either species or geographic regions.
Figure 1. A screenshot of the IWT Data Directory landing page shows the multiple columns used to organize records such as title, type of data, accessibility. Each column can be used to search the directory using the filter function.
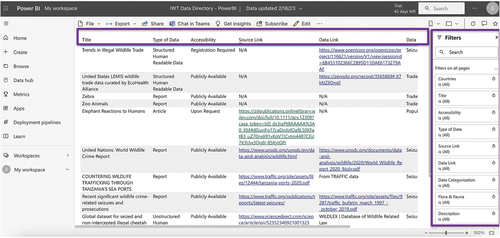
We acknowledge limitations to the data directory. First, many of the datasets and species included in the directory are of African origin. Although the directory itself exists on a global scale, often with hundreds of different species, due to the nature of charismatic megafauna, African species tend to be studied more comprehensively than many non-African species. Due to this bias, the availability of publicly accessible data will remain consistent with this trend. However, we are hopeful that encouraging collaboration in the conservation community and on an interdisciplinary level will aid in developing a more comprehensive collection of species data. Second, our search relied heavily on Google, which may miss datasets and sources that are not highly promoted or are simply not indexed by Google, such as those in PDFs, on websites with restricted access, or websites in countries not accessible by Google. Furthermore, by conducting the search in English we can verify the discovered data sources at the expense of potentially missing data sources in other relevant languages like French, Arabic, or Mandarin. We hope that by building our directory as a living document and incorporating experts from other countries and backgrounds, we can grow the directory to include data sources that are in other languages, or which might be inaccessible through Google but made accessible through a network of scholars. Lastly, we were only able to discover datasets and sources that currently existed rather than proactively generate or call for datasets that may not currently exist, but which are relevant to wildlife trafficking. We hope that by helping to determine which data sources exist, we can also identify the gaps in our current knowledge base to promote the creation of useful datasets, such as data about wildlife trafficking enforcement, wildlife consumer behavior data, or financial data tied to wildlife trafficking.
We intended for the data directory to be a living document. Ideally, researchers contribute to and leverage the data sources to perform a range of research and analyses. Researchers currently studying wildlife trafficking can scale classical conservation biology-related insights in a more strategic fashion (e.g. multiple species, multiple protected areas), integrating different knowledge and bringing more complex and complete understanding of the phenomenon as opposed to creating a new niche development for research. More granular insights can be derived from triangulating data using repeated measures. Most importantly, new research questions that have not been anticipated may be posed and answered.
Open Scholarship
This article has earned the Center for Open Science badge for Open Data. The data are openly accessible at https://doi.org/10.15482/USDA.ADC/1524754.
Acknowledgements
The information contained herein does not represent the opinions of the U.S. Government or any author affiliations.
Disclosure statement
No potential conflict of interest was reported by the authors.
Data availability statement
There is no custom code associated with this research. The data directory is available at Zenodo open repository (https://doi.org/10.5281/zenodo.7096921). The visualization is available at http://conservationcriminology.com/iwt-data-directory.
Additional information
Funding
Notes on contributors
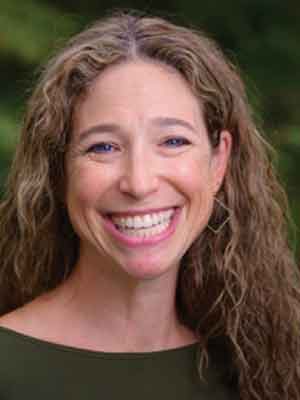
Meredith L. Gore
Meredith L. Gore is an Associate Professor of Human Dimensions of Global Environmental Change in the Department of Geographical Sciences at University of Maryland, College Park. She received her PhD from Cornell University.
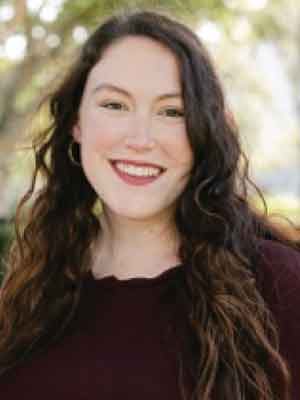
Rowan Hilend
Rowan Hilend is a PhD candidate of Logistics in the Department of Supply Chain Management at Michigan State University’s Broad College of Business.
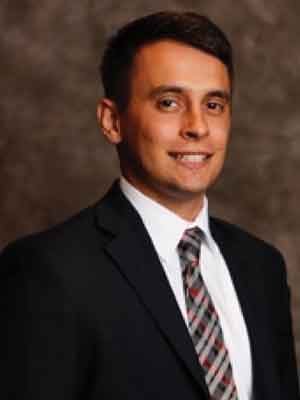
Jonathan O. Prell
Jonathan O. Prell is an MBA Candidate in the Manderson Graduate School at the University of Alabama’s Culverhouse College of Business.
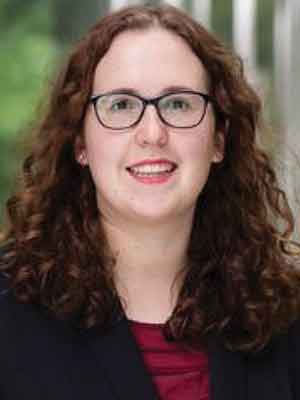
Emily Griffin
Emily Griffin is an Assistant Professor of Operations Management in the Operations and Information Management Division at Babson College. She received her PhD from University of Alabama.
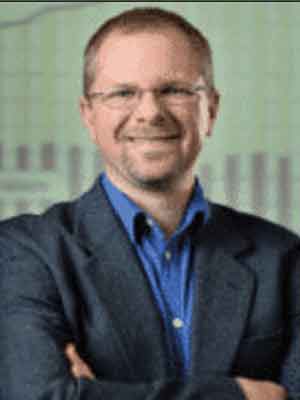
John R. Macdonald
John R. Macdonald is an Associate Professor of Supply Chain Management and Logistics in the Department of Management at Colorado State University. He received his PhD from University of Maryland, College Park.
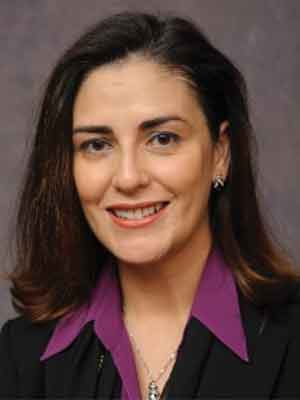
Burcu B. Keskin
Burcu B. Keskin is a Professor of Operations Management, Reese Phifer Fellow in Operation Management and Associate Department Head in the Department of Information Systems, Statistics and Management Science at the University of Alabama Culverhouse College of Business. She received her PhD from Texas A&M University.
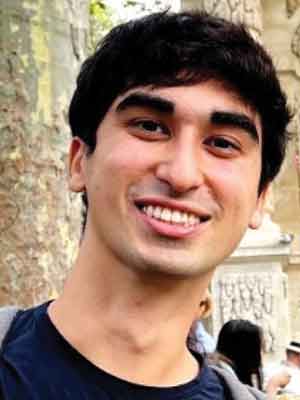
Aaron Ferber
Aaron Ferber is a PhD candidate of Computer Science in the University of Southern California Viterbi School of Engineering.
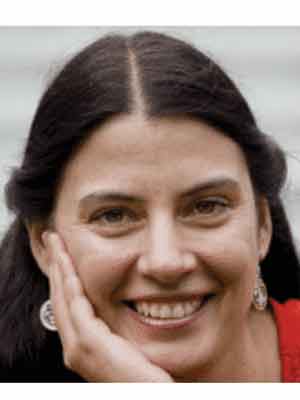
Bistra Dilkina
Bistra Dilkina is an Associate Professor of Computer Science in the University of Southern California Viterbi School of Engineering, Dr Allen and Charlotte Ginsburg Early Career Chair and Co-Director of the Center for AI in Society. She received her PhD from Cornell University.
References
- Agu, H. U., & Gore, M. L. (2020). Women in wildlife trafficking in Africa: A synthesis of literature. Global Ecology and Conservation, 23, e01166. https://doi.org/10.1016/j.gecco.2020.e01166
- Aliyu, M. B. (2017). Efficiency of boolean search strings for Information retrieval. American Journal of Engineering Research, 6(11), 216–222.
- Barrett, A. J., Evaluation, D., Validation, & Quality. (1993). ASTMManual on The Building of Material Databases. In H. Crystal (Ed.), ASTM Manual Series: MNL 19 (pp. 53–67).
- Dalpane, F., & Baideldinova, M. (2022). Poaching and Wildlife Trafficking as Threats to International Peace and Security. In S. Sayapin, R. Atadjanov, U. Kadam, G. Kemp, N. Zambrana-Tévar, & N. Quénivet (Eds.) International Conflict and Security Law. T.M.C. Asser Press, The Hague. https://doi.org/10.1007/978-94-6265-515-7_40
- EMIW. (n.d.). United Nations Geoscheme. https://www.emiw.org/fileadmin/emiw/UserActivityDocs/Geograph.Representation/Geographic-Representation-Appendix_1.pdf
- Felbab-Brown, V. (2018). Wildlife and drug trafficking, terrorism, and human security. Prism, 7(4), 124–137.
- Felbab-Brown, V. (2018). Wildlife and Drug Trafficking, Terrorism, and Human Security: Realities, Myths, and Complexities Beyond Africa. PRISM, 7(4), 124–137.
- Ferber, A., Griffin, E., Dilkina, B., Keskin, B., & Gore, M. L. (2023). Predicting Wildlife Trafficking Routes with Differentiable Shortest Paths. Proceedings of the Integration of Constraint Programming, Artificial Intelligence, and Operations Research: 20th International Conference, CPAIOR 2023. Nice, France, Springer International Publishing.
- Gianopoulos, K. M. Combating Wildlife Trafficking: Agencies Work to Address Human Rights Abuse Allegations in Overseas Conservation Programs. https://www.gao.gov/assets/gao-21-139r.pdf. (2020).
- Gore, M. L. (2023). A data directory to facilitate investigations on worldwide wildlife trafficking (2.0) [Data set]. Zenodo, https://doi.org/10.5281/zenodo.76476401
- Gore, M. L., Schwartz, L. R., Amponsah-Mensah, K., Barbee, E., Canney, S., Carbo-Penche, M., Cronin, D., Hilend, R., Laituri, M., Luna, D., Maina, F., Mey, C., Mumford, K., Mugo, R., Nduguta, R., Nyce, C., McEvoy, J., McShea, W., Mandimbihasina, A., & Naess, L. W. (2022). Voluntary consensus based geospatial data standards for the global illegal trade in wild fauna and flora. Scientific Data, 9(1), 267. https://doi.org/10.1038/s41597-022-01371-w
- Guynup, S., Shepherd, C. R., & Shepherd, L. (2020). The true costs of wildlife trafficking. Georgetown Journal of International Affairs, 21(1), 28–37. https://doi.org/10.1353/gia.2020.0023
- Hayek, M. N., Harwatt, H., Ripple, W. J., & Mueller, N. D. (2021). The carbon opportunity cost of animal-sourced food production on land. Nature Sustainability, 4(1), 21–24.
- Hemming, V., Burgman, M. A., Hanea, A. M., McBride, M. F., Wintle, B. C., & Anderson, B. (2018). A practical guide to structured expert elicitation using the IDEA protocol. Methods in Ecology and Evolution, 9(1), 169–180. https://doi.org/10.1111/2041-210X.12857
- Henson, V. R., Cobourn, K. M., Weathers, K. C., Carey, C. C., Farrell, K. J., Klug, J. L., Sorice, M. G., Ward, N. K., & Weng, W. (2020). A practical guide for managing interdisciplinary teams: Lessons learned from coupled natural and human systems research. Social Sciences, 9(7), 119. https://doi.org/10.3390/socsci9070119
- IUCN Red List. (n.d.). The IUCN Red List of Threatened Species. https://www.iucnredlist.org
- Keskin, B. B., Griffin, E. C., Prell, J. O., Dilkina, B., Ferber, A., MacDonald, J., Gore, M. L., Griffis, S., & Gore, M. L. (2022). Quantitative investigation of wildlife trafficking supply chains: A review. Omega, 115, 102780. https://doi.org/10.1016/j.omega.2022.102780
- Miller, N. A., Roan, A., Hochberg, T., Amos, J., & Kroodsma, D. A. (2018). Identifying global patterns of transshipment behavior. Frontiers in Marine Science, 5, 240. https://doi.org/10.3389/fmars.2018.00240
- NatureServe. (2023). Biodiversity in focus: United States edition. https://www.natureserve.org/sites/default/files/NatureServe_BiodiversityInFocusReport_medium.pdf
- Pan, H., Bakalov, V., Cox, L., Engle, M. L., Erickson, S. W., Feolo, M., Hamilton, C. M., Huggins, W., Hwang, S., Kimura, M., Krzyzanowski, M., Levy, J., Phillips, M., Qin, Y., Williams, D., Ramos, E. M., & Hamilton, C. M. (2022). Identifying datasets for cross-study analysis in dbGap using PhenX. Scientific Data, 9(1), 532. https://doi.org/10.1038/s41597-022-01660-4
- Price, R. (2018). The contribution of wildlife to the economies of sub-saharan Africa. K4D Helpdesk Report. Institute of Development Studies.
- Sanjurjo-Rivera, E., Mesnick, S. L., Ávila-Forcada, S., Poindexter, O., Lent, R., Felbab-Brown, V., Sainz, J. F., Squires, D., Sumaila, U. R., Munro, G., Ortiz-Rodriguez, R., Rodriguez, R., & Sainz, J. F. (2021). An economic perspective on policies to save the Vaquita: Conservation actions, wildlife trafficking, and the structure of incentives. Frontiers in Marine Science, 8, 644022. https://doi.org/10.3389/fmars.2021.644022
- Session, J. (2018, October 11). Statement on Behalf of the United States at the London Illegal Wildlife Trade Conference 2018. The United States Department of Justice. https://www.justice.gov/opa/speech/attorney-general-sessions-delivers-statement-behalf-united-states-london-illegal-wildlife
- Sharma, B. (2020). Descriptive analytics on the endangered species international trade. The Journal of Applied Business and Economics, 22(3), 150–158.
- Shelley, L. I. (2018). Dark commerce: How a new illicit economy is threatening our future. Princeton University Press.
- Van Schendel, W., & Abraham, I. (Eds.). (2005). Illicit flows and criminal things: States, borders, and the other side of globalization. Indiana University Press.
- Vigne, L., & Nijman, V. (2022). Elephant ivory, rhino horn, pangolin and helmeted hornbill products for sale at the Myanmar–Thailand–China border. Environmental Conservation, 49(3), 187–194. https://doi.org/10.1017/S0376892922000169
- The World Bank Group. (2022). The global wildlife program progress report 2021. World Bank. July, https://thedocs.worldbank.org/en/doc/094dc1a42acc69914fc259c593ab6d9f-0320052022/original/2021-GWP-Annual-Report.pdf
- Xu, Q., Li, J., Cai, M., & Mackey, T. K. (2019). Use of machine learning to detect wildlife product promotion and sales on Twitter. Frontiers in Big Data, 2, 28. https://doi.org/10.3389/fdata.2019.00028