Big Earth data are increasingly used in a variety of applications. At the same time, technological developments happen rapidly and include Earth observation data cubes, analysis-ready data (ARD), the need to access distributed systems and data to avoid replicating datasets, searching and finding datasets, or visualization of data and information in a comprehensive way.
The current pace in which technology and methodology using big Earth data is developed is high, but this should be seen as an opportunity to strive for flexible and innovative solutions. Those solutions and approaches may even come from other domains and disciplines as remote sensing or Earth observation (EO) in general and they should be embraced as a facilitator of the multi- and interdisciplinary nature that is inherent to big Earth data science and research.
Although worthwhile to envision, it would be a challenging or even impossible task to get a full and comprehensive overview over the state-of-the-art, developments, and current research agendas of all disciplines that are contributing to big Earth data. In this special issue, we aimed to curate contributions that can be seen as a snapshot of “emerging trends” instead of providing a comprehensive overview, which is hardly possible in such a highly dynamic field. Seven papers illustrate the variety of topics, different available solutions, and challenges that lie ahead. The contributions are as varied as the topics and range from technical notes as a state-of-the-art report to very detailed, comprising articles.
The contributions can be categorised into four sub-topics: data sources, data management, data analytics, and data visualization. However, the boundaries of these categories cannot be strictly drawn, and investigations of individual solutions provided in the articles can be put into their contexts within a bigger big Earth data workflow.
Baraldi et al. (Citation2022a, Citation2022b) investigate the concept of ARD in two papers (part 1 and part 2), and proposes a new workflow for generating ARD. These papers are technologically dense, but provide concepts and ideas for quality indicators, while also considering data storage and querying. Considering that the quality of subsequent analyses depends largely on the quality of input data, providing high-quality ARD is of interest for the entire community.
Backeberg et al. (Citation2022) describe technical solutions for federated big Earth data management and processing. With the objective to overcome limitations of requirements to keep all data within one system, different providers may share responsibilities and technical implementations of concepts within the overall system. For users, such a system is aimed to be transparent and usable without technical barriers. Such federated systems provide different access points to data and computing infrastructure, which, overall, complements the technical landscape.
Sudmanns et al. (Citation2022) investigate local EO data cubes and how they contribute to the Digital Earth vision. Based on four examples (Swiss Data Cube, Sen2Cube.at semantic EO data cube for Austria, Digital Earth Africa, and Virginia Data Cube) and five perspectives (science, business and industry, government and policy, education, communities and citizens), different advantages and disadvantages are highlighted. Local EO data cubes can be often seen as an alternative to global, monolithic solutions. Considering recent implementations of local, regional, or national data cube initiatives all over the globe, this paper approaches the question whether these are worthwhile efforts and comes to the conclusion that the Digital Earth vision benefits from multiple access points for data and computing infrastructure provided to users and their specific needs.
Wang et al. (Citation2022) provide a data classification system for big Earth data with the goal of associating datasets to hierarchical semantic labels. The automatically extracted labels include different domains on the first level and sustainable development goals (SDGs) on a second level. This contribution towards a better and more effective classification of big Earth datasets is an important intermediate step to support users in searching and finding the datasets that they need, but may also be used to identify spatial, temporal, and thematic gaps in datasets.
Zhang et al. (Citation2023) tackle the problem of data scarcity, which still exists in the big EO data era. They provide a high-resolution daily dataset of the Standardized Precipitation Index (SPI) and Standardized Precipitation Evapotranspiration Index (SPEI) for China calculated from ground stations. The value of this dataset is shown using the example of droughts. This topic, and additional climate-change relevant indicators can benefit from this dataset, while similar datasets could be provided for other regions of the world as well. The dataset provided here is open access and can be downloaded by anyone who is interested.
Narvaez et al. (Citation2023) develop and describe the Solar Atlas of Colombia as an interactive tool for data visualization to allow users to explore the solar photovoltaic potential. Users of the Solar Atlas can use historical data from satellite observations and outputs from climate change scenarios as well as models for simulating the potential power production of solar panel installations. Reaching out to end users and providing tailored, easy-to-use interfaces with comprehensive visualizations is essential to create an impact from big Earth data. This contribution demonstrates an example with validated datasets, a graphical user interface, and an important use-case as application.
All contributions to this issue clearly demonstrate the worth and potential of big Earth data for real-world applications that already have transcended from research into operational applications. They also discuss research questions that remain open, showing the need to continue with research in different disciplines and angles, with considerations including data availability and data quality, technical issues around data management, data searches, data visualisation, and societal and environmental concerns from local level to global SDGs goals.
We can conclude from this special issue that solid scientific foundations need to be established – where not already done – on all aspects of big Earth data and that the individual developments build on top of each other. The curated snapshot of currently emerging trends shows that we are on track to create such a pathway now but also as a substantial basis for future work.
Disclosure statement
No potential conflict of interest was reported by the author(s).
Additional information
Notes on contributors
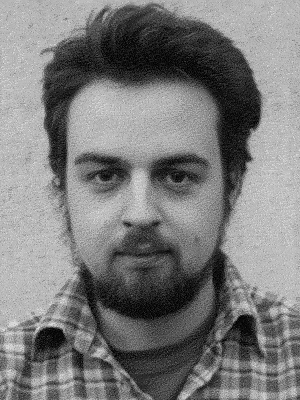
Martin Sudmanns
Martin Sudmanns, Dr., is postdoctoral researcher at the Paris Lodron University Salzburg, Department of Geoinformatics, Austria, and co-head of the research area EO Analytics. His research focuses on Geoinformatics, computer-based representation of natural phenomena in spatial data models, spatio-temporal Earth observation analysis in the context of data cubes and big EO data.
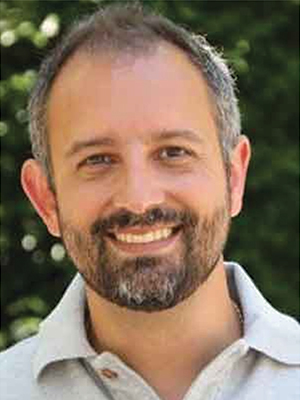
Gregory Giuliani
Gregory Giuliani, Dr., is the Head of the Digital Earth Unit and Swiss Data Cube Project Leader at GRID-Geneva of the United Nations Environment Programme (UNEP) and a Senior Lecturer at the University of Geneva’s Institute for Environmental Sciences. He is a geologist and environmental scientist who specialises in Remote Sensing, Geographical Information Systems (GIS) and Spatial Data Infrastructures (SDI). He also works at GRID-Geneva of the United Nations Environment Programme (UNEP) since 2001, where he was previously the focal point for Spatial Data Infrastructure (SDI) and is currently the Head of the Digital Earth Unit. Dr. Giuliani's research focuses on Land Change Science and how Earth observations can be used to monitor and assess environmental changes and support sustainable development.
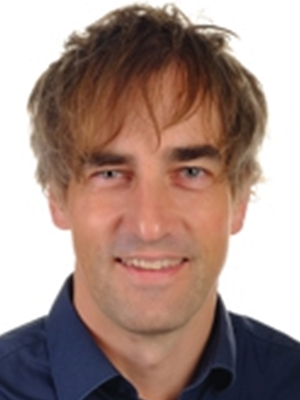
Dirk Tiede
Dirk Tiede, Dr., is Associate Professor and Deputy Head of the Department of Geoinformatics – Z_GIS, University of Salzburg, Austria, and co-head of the research lab EO Analytics. His research focuses on methodological developments in image analysis using optical EO data, object-based methodologies and process automation in the context of Big EO data analysis. Research fields include environmental monitoring and support of humanitarian relief operations, for which he received the Christian-Doppler-Award of the Federal State of Salzburg in 2014.
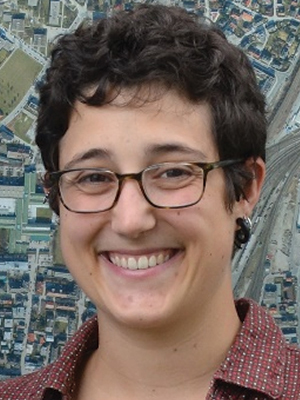
Hannah Augustin
Hannah Augustin, MSc, is a PhD researcher in Earth observation (EO) Analytics at the Paris Lodron University of Salzburg, Department of Geoinformatics, Austria with a research focus on semantic EO data cubes, and automated and transferable processes for generating EO-informed indicators from big optical EO imagery.
References
- Backeberg, B., Šustr, Z., Fernández, E., Donchyts, G., Haag, A., Oonk, J. B. R., Venekamp, G., Schumacher, B., Reimond, S., & Chatzikyriakou, C. (2022). An open compute and data federation as an alternative to monolithic infrastructures for big Earth data analytics. Big Earth Data, 7(3), 812–830. https://doi.org/10.1080/20964471.2022.2094953
- Baraldi, A., Sapia, L. D., Tiede, D., Sudmanns, M., Augustin, H. L., & Lang, S. (2022a). Innovative Analysis Ready Data (ARD) product and process requirements, software system design, algorithms and implementation at the midstream as necessary-but-not-sufficient precondition of the downstream in a new notion of Space Economy 4.0 - Part 1: Problem background in Artificial General Intelligence (AGI). Problem Background in Artificial General Intelligence (AGI), Big Earth Data, 7(3), 455–693. https://doi.org/10.1080/20964471.2021.2017549
- Baraldi, A., Sapia, L. D., Tiede, D., Sudmanns, M., Augustin, H., & Lang, S. (2022b). Innovative Analysis Ready Data (ARD) product and process requirements, software system design, algorithms and implementation at the midstream as necessary-but-not-sufficient precondition of the downstream in a new notion of Space Economy 4.0 - Part 2: Software developments. Big Earth Data, 7(3), 694–811. https://doi.org/10.1080/20964471.2021.2017582
- Narvaez, G., Felipe Giraldo, L., Bressan, M., Guillen, C. A., Pabón, M. A., Díaz, N., Felipe Porras, M., Herney Medina, B., Jiménez, F., Jiménez-Estévez, G., Pantoja, A., & Alonso, C. (2023). An interactive tool for visualization and prediction of solar radiation and photovoltaic generation in Colombia. Big Earth Data, 7(3), 904–929. https://doi.org/10.1080/20964471.2023.2185920
- Sudmanns, M., Augustin, H., Killough, B., Giuliani, G., Tiede, D., Leith, A., Yuan, F., & Lewis, A. (2022). Think global, cube local: An Earth observation data cube’s contribution to the digital Earth vision. Big Earth Data, 7(3), 831–859. https://doi.org/10.1080/20964471.2022.2099236
- Wang, J., Kun, B., Yan, D., Wang, J., Duan, B., Zhang, M., & Guojin, H. (2022). Classification framework and semantic labeling for Big Earth Data. Big Earth Data, 7(3), 886–903. https://doi.org/10.1080/20964471.2022.2123946
- Zhang, R., Bento, V. A., Qi, J., Xu, F., Wu, J., Qiu, J., Li, J., Shui, W., & Wang, Q. (2023). The first high spatial resolution multi-scale daily SPI and SPEI raster dataset for drought monitoring and evaluating over China from 1979 to 2018. Big Earth Data, 7(3), 860–885. https://doi.org/10.1080/20964471.2022.2148331