ABSTRACT
This study examined the influence of tree species and structural diversity on the production of high-quality logs. The data were from the regional forest inventory of the University Forest Enterprise, Czech Republic, performed from 2009 to 2011 on 1188 sample plots. For every sample plot, we quantified 38 diversity indicators. The plots were divided into four age groups (young, middle-aged, old and uneven-aged stands). The anticipated proportion of high-quality logs was determined using local assortment tables. For each age group, the impact of species and structural diversity indicators on the volumetric proportion of high-quality logs was assessed using backwards multiple regression. The relationship between diversity measures and log quality changed with stand age. In old stands, horizontal structure had a more profound effect on the proportion of high-quality logs. In young stands, species diversity and vertical structure were more influential. In middle-aged stands, the impact of stand diversity on log quality was most complex. In uneven-aged stands, vertical structure was the diversity component most affecting the proportion of high-quality logs. Overall, the proportion of best quality logs increased with the increasing stand diversity in all age classes, suggesting that timber production and stand diversity are not contradictory management goals.
EDITED BY Sheila Ward
1. Introduction
Timber production and economic yield have always been primary forestry objectives. However, demands for timber and timber products are often considered to be in conflict with demands to maintain biodiversity (Fox et al. Citation2006). Forest production research has mostly dealt with the relationship between timber yields and species diversity (e.g. Belote et al. Citation2011; Pretzsch Citation2005; Liang et al. Citation2007; Wang et al. Citation2011). Recently, the impact of stand structural diversity (i.e. spatial and size differentiation of forest stands, Gadow & Hui Citation1999) on timber production has also received attention (e.g. Liang et al. Citation2007; Lei et al. Citation2009; Long & Shaw Citation2010; Wang et al. Citation2011).
Although stand productivity and assortment structure (i.e. quantity of logs of differing quality that can be produced from a stand and used for different products; Forest Research Agency Citation2015) are closely related, higher productivity does not necessarily generate better quality wood. Stands with similar timber stocks may significantly differ in the quality of the timber produced (i.e. in the assortment structure), which affects the income from forests, because the prices of fuel timber are several times lower than the prices of veneer or sawlogs (Suchomel et al. Citation2012). Therefore, one of the main goals of forest management is to produce high-quality timber assortments (assortments being pieces of timber of different quality into which a tree may be converted; Oxford English Dictionary Citation2015).
Stand assortment structure is influenced by a number of factors (Danilović Citation2008; Prka & Krpan Citation2010; Prka Citation2012). One of the main factors affecting the distribution of timber assortments in individual trees is the diversity of tree habitus (stem and crown shape; Prka Citation2012), which is largely influenced by forest structure. This was demonstrated by Liang et al. (Citation2007), who showed that trees of the same size may be of a very different quality due to stand characteristics, such as density, spatial distribution of trees and species composition. However, information about the relationship between stand species and structural diversity, and timber quality is limited. Liang et al. (Citation2007) reported that stands with greater species and structural diversity contained a higher percentage of high-quality timber. Similarly, Guldin and Fitzpatrick (Citation1991) found better log quality in uneven-aged stands than in even-aged stands, while Macdonald et al. (Citation2010) indicated insignificant differences between timber quality of even-aged homogeneous stands and structurally more diverse forests.
Since studies dealing with this issue are scarce, our goal was to fill this gap by analysing relationships between indicators of tree species and structural diversity and timber quality in Central European forest stands. We searched for the answers to the following questions: (1) How is the timber quality of a stand influenced by tree species and structural diversity? (2) What diversity elements () have the most impact on timber quality? (3) Is the effect of diversity on log quality independent of stand age? We focused upon high-quality, economically most profitable timber (Suchomel et al. Citation2012) which is most valuable for the wood-processing industry. We hypothesised that the relationship between stand diversity and timber quality is positive, that management goals to achieve high stand diversity and high-quality timber are not contradictory, but complementary to each other. We also expected that their relationship is age-related, that is, the elements of diversity that affect timber quality are not constant over time, but change with age.
Table 1. Species and structural diversity indicators used in this study.
2. Materials and methods
Data was collected during the regional forest inventory of University Forest Enterprise ‘Kostelec nad Černými lesy’ of the Czech University of Life Sciences () performed from 2009 to 2011 (Merganič, Marušák et al. Citation2012). The total area of the enterprise is 6581 ha, 95.4% of which is covered with forests. The majority of the forests in the enterprise are managed, so their development is influenced to a large extent by human activities. Generally, small-scale clear-cutting is used in areas not exceeding 1 ha. Elevations range from 220 to 560 m a.s.l. The average length of the growing season is 153 days. Mean annual temperature varies from 7.0°C to 7.5°C, and mean temperature during the growing season ranges between 13.0°C and 13.8°C. Mean annual precipitation fluctuates from 600 to 650 mm. According to Czech typology (Plíva Citation1987), six forest types occur in the area of the enterprise. The most common types are Querceto-Fagetum acidophilum and Querceto-Fagetum mesotrophicum that cover almost 40% of the total area of the enterprise. Since these forest types are the most common in the Czech Republic (ÚHÚL Citation2007), and the tree species composition of the enterprise is similar to the species composition of Czech forests (Krejzar et al. Citation2015), the enterprise represents well the country’s forests.
Figure 1. The area of the forest management unit of University Forest Enterprise ‘Kostelec nad Černými lesy’ and its location in the Czech Republic (inset map).
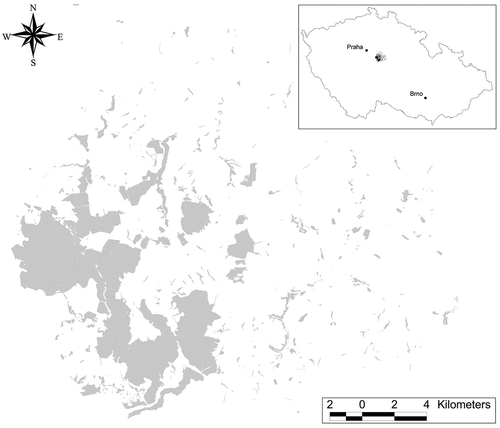
In total, 1188 inventory sample plots were established over the whole forested area of the forest enterprise using a stratified sampling design (Merganič, Marušák et al. Citation2012). The sample plots were of a circular shape with an area of 500 m2. On each plot, approximately 100 variables describing site conditions (e.g. slope, aspect, soil moisture), stand development (e.g. stand age, canopy cover, number of layers, level of tree aggregation and mixture) and tree status (e.g. tree species, tree diameter at breast height (DBH), tree height, stem quality, health condition) were assessed. Canopy cover was defined as a ratio of the sum of tree crown projections (i.e. the area of the vertical projections of the outermost perimeter of the crowns on the horizontal plane, Gschwantner et al. Citation2009) with canopy overlaps removed, to the total area of the sample plot. Hence, the maximum value of canopy cover is 100%. Canopy cover was visually estimated in the field as per cent cover at 5% intervals, separately for trees with DBH less than and greater than 7 cm (hereafter referred to as young and old trees, respectively). Tree canopy layers (N0Lay, ) were identified after Zlatník (Citation1976) as (1) dominant (significantly above the trees that form the main canopy), (2) co-dominant (trees that form the main canopy), (3) subdominant (trees that are higher than a half of the mean height of dominant trees, but do not reach the main canopy), (4) intermediate (trees and shrubs with a height greater than 1.30 m and smaller than a half of the mean height of dominant trees) and (5) undergrowth (trees smaller than 1.3 m). Undergrowth was further divided into three layers: (5a) trees between 20 cm and 1.30 m in height, (5b) seedlings smaller than 20 cm but older than 1 year and (5c) seedlings up to a year old.
Overall, 29 tree species were identified within the inventory, all occurring in the class of old trees and 22 occurring in the class of young trees. Norway spruce (Picea abies L. Karst.) was the most common tree species in both young and old trees (26% and 53% calculated from the number of young trees and stand volume of old trees, respectively). Other common tree species were silver fir (Abies alba), Scots pine (Pinus sylvestris), European larch (Larix decidua), common beech (Fagus sylvatica), oak (Quercus spp.), hornbeam (Carpinus betulus) and birch (Betula pendula syn. Betula verrucosa). The proportion of some light-demanding species (e.g. Scots pine or larch) was lower in young trees than in old trees, while the proportion of silver fir, which is a shade-tolerant species, was greater in young than in old trees.
In the study, we included stands of all ages because they all represent potential sources of timber, and because their current state affects their future quality, and hence future income from the forests. Since all growth parameters of trees are age-dependent and they do not develop linearly over time, for the analysis, sample plots were divided into four age groups (young, middle-aged, old and uneven-aged stands). Young stands had a mean tree age less than 40 years, middle-aged stands had trees with a mean age between 40 and 100 years and old stands had trees with a mean age greater than 100 years. Stands were classified as uneven-aged if the crown cover of young trees with diameters less than 7 cm exceeded 30%. From all inventory plots, 265, 530, 275 and 118 plots represented young, middle-aged, old and uneven-aged stands, respectively. These age groups enabled the analysis of the relationship between diversity and quality while excluding the non-linear impact of stand age. At the same time, it allowed us to examine if and how the relationship changes with stand age.
2.1. Quantification of plant diversity
Stand diversity was quantified using different indicators representing plant species and structural diversity. Species diversity is based on species composition that refers to the identity and variety of elements in a group (Noss Citation1990). Depending on how the species composition is evaluated, species diversity indicators can be placed in three groups (Ludwig & Reynolds Citation1988; Krebs Citation1989): (1) species richness expressed as a number of species in a stand (Krebs Citation1989), (2) species evenness referring to the equality in species composition in a stand (Bruciamacchie Citation1996) and (3) species heterogeneity encompassing both species richness and evenness (Bruciamacchie Citation1996). In addition to these three categories, we identified the fourth group of species diversity indicators representing species similarity between young and old trees in a stand (Magurran Citation2004) (). Structural diversity of a stand is defined as the specific arrangement of stand components (Gadow Citation1999). In our analysis, we identified four groups of structural indicators: (1) horizontal structure (i.e. spatial distribution of the elements in the stand; Neumann & Starlinger Citation2001), (2) vertical structure (i.e. vertical differentiation of the stand; Kint et al. Citation2000), (3) structural similarity between young and old trees in a stand (Ludwig & Reynolds Citation1988; Krebs Citation1989) and (4) woody debris (Humphrey & Watts Citation2004; Woodall & Williams Citation2005) ().
The selection of the individual indicators in each group was based on review of the literature (Ludwig & Reynolds Citation1988; Humphrey & Watts Citation2004; Woodall & Williams Citation2005; Merganič, Merganičová et al. Citation2012). The selected indicators are presented in .
2.2. Calculation of tree volume
Tree volume was calculated using two-parameter regressions derived for volumetric tables of Czechoslovakia by Petráš and Pajtík (Citation1991) for the most common 11 tree species: 7 broadleaved (beech, oak, hornbeam, birch, ash, poplar, poplar clone, alder) and 4 coniferous tree species (spruce, fir, pine, larch). The equations were derived from felled sample trees measured in 2 m sections, including stem and crown woody parts. The mathematical form and the regression coefficients differed for individual tree species because each tree species is characterised by a different tree habitus. The specific formulations for individual species can be found in Petráš and Pajtík (Citation1991).
The general form of the relationship was
where v is total volume in cubic metres, dbh is the tree DBH in centimetres and h is the tree height in metres.
2.3. Log grading
Log grading was performed using assortment tables of Petráš and Nociar (Citation1990, Citation1991) that quantify the proportion of six different product classes ranging from logs of the highest quality that can be used for veneer production to low-quality fuel wood. Log grading of each tree is dependent on the region, tree species, age, tree DBH, stem quality and stem damage assessed in the field. For the purposes of this study, for each stand, we used the proportion of the two top assortment classes containing the highest quality logs, which can be used for veneer, musical instruments, sport equipment and barrels. For each plot, an indicator of anticipated production of high-quality logs, or quality log proportion (QLP), was quantified as the volumetric proportion of the two best assortment classes from the total timber volume of the plot. The proportion of the logs was chosen instead of the absolute volume to ensure the comparability of the individual plots, because the coefficient of variation of volume was high (61%). We focused upon this high-quality timber because it is economically the most profitable and most valuable for the wood-processing industry. Our preliminary analysis showed that the mean proportion of this high-quality timber in our region was 26%, indicating that the two top assortment classes represented one-fourth of the whole timber stock of the enterprise.
2.4. Multiple regression between diversity indicators and indicators of anticipated log quality
The analysis was carried out in several steps. First, plots were segregated into the four age groups previously discussed to exclude the impact through time of non-linear development of the proportion of high-quality timber. Then, diversity indicators were divided into eight groups characterising species richness, species evenness, species heterogeneity, species similarity, horizontal structure, vertical structure, structural similarity and woody debris (). This was done because the high number of indicators and their multi-collinearity would cause difficulty in estimating variable coefficients.
For each stand age group, multiple linear regression was then performed separately for each group of diversity indicators, using all the indicators in that category, to determine which indicators had the greatest effect on the volumetric proportion of high-quality logs (QLP):
where a and b are regression coefficients of the linear regression and i is the index for n diversity indicators (ranging from 1 to n) for a given category of diversity indicators. In addition to diversity indicators, these regressions included five covariates as independent variables: total volume of living trees (VolTotal), mean age of young and old trees per stand (AgeYoung, AgeOld) and canopy cover of young and old trees per stand (CanopyYoung, CanopyOld). The selection of these covariates was based on factors known to affect forest growth and yield processes (Pretzsch Citation2009) and previous analyses of our data which showed that stand age, stocking and forest type had a significant impact on both species richness and the economic value of timber in the stands of the forest enterprise (Merganič, Marušák et al. Citation2012). Stand volume and canopy cover can be considered as indirect measures of site conditions (i.e. of forest types).
In the next step, independent variables included in the preliminary analyses (both diversity indicators and covariates) were excluded from the regression using backwards selection based on their tolerance, variance inflation factor (VIF) and t value. The tolerance of each indicator should be above 0.1 and the higher the value of tolerance the better (Quinn & Keough Citation2002). VIF should be below 10, preferably as low as possible, to avoid strong multi-collinearity between the independent variables (Marquardt Citation1970). Diversity indicators should also have t values greater than or equal to 1.96 (for and
) to significantly contribute to explaining the variance of QLP, the dependent variable.
In the final step, for each stand age group, all significant diversity indicators from each diversity group and all covariates were included in one multiple regression and those that were insignificant and/or showed strong collinearity to other independent variables were excluded using backwards selection. The final multiple regressions for each stand age group comprised only significant independent variables that were not collinear.
3. Results
For the evaluated stands, plant species and structural diversity had a significant impact on the anticipated production of high-quality logs (QLP). However, the adjusted R-squared of the derived multiple regressions did not exceed 0.5, indicating that the combination of significant diversity indicators and covariates explained less than 50% of the total variance in QLP for a given stand age group. The highest correlation coefficient was obtained for the age group of uneven-aged stands (R = 0.68), and the lowest for old stands (R = 0.38).
The number of indicators included in the regressions and their combination differed between the age groups. The highest number of independent variables was in the regression of middle-aged stands (8), for which we found the second highest correlation (R = 0.62). The minimum number of independent variables (3) was in the regression derived for uneven-aged stands ().
Table 2. Statistics of multiple linear regressions calculated for age groups.
Every regression contained at least one covariate variable (). In old and young stands, the age of young trees (AgeYoung) had a negative significant impact on the proportion of best quality logs, while in middle-aged and uneven-aged stands it was the age of old trees (AgeOld) which had a positive significant relation to QLP (). Stand volume (VolTotal) as another covariate was included in two regression models for middle-aged and young stands, in which it had the greatest positive impact on the proportion of best quality logs (, ). Another covariate, canopy cover of old trees (CanopyOld), remained only in the regression for old stands (), where it was the second most influential indicator and had a positive relation with QLP.
Figure 2. Pareto graph of independent variables included in the final multiple linear regressions derived for best log quality in four age groups: old stands (top left), middle-aged stands (top right), young stands (bottom left) and uneven-aged stands (bottom right). t-Values are given for the significant independent variables remaining in the regressions after backwards elimination.
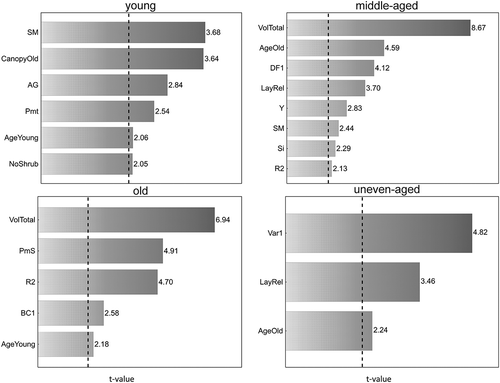
All significant species diversity indices except for BC1 had a positive relation to the proportion of best quality logs in all regressions (). Species diversity indicators were included in all regressions except in the equation for uneven-aged stands. Three species diversity indicators were included in the regressions for middle-aged stands, one representing species richness (R2), one species heterogeneity (Si) and one species similarity between young and old trees (Y) (). In young stands, the proportion of high-quality timber was significantly correlated with two species diversity indicators representing species richness and species similarity between young and old trees (R2 and BC1, respectively). In the regression derived for old stands, the number of shrub species representing species richness (N0Shrub) was the only species diversity indicator with significant impact on QLP ().
All regressions contained structural diversity indicators (), but their character differed among stand age groups. Indicators of vertical structure occurred in the regressions for the uneven-aged, young, and middle-aged stands. Indicators of horizontal structure had significant impacts on QLP in middle-aged and old stands, and woody debris had a significant impact on QLP in old stands (). All indicators of structural diversity were positively correlated with QLP except for the ratio of volume of fine to coarse woody debris (PmT) ().
For old forest stands, six independent variables entered the final multiple regression model (), of which two were covariates (canopy cover of old trees [CanopyOld] and stand age of young trees [AgeYoung]), one represented species richness (number of shrub species [N0Shrub]) and two represented horizontal structural diversity (species aggregation [AG], species mixture [SM]) and one represented dead wood (ratio of volume of fine woody debris to coarse woody debris [PmT]). SM had the highest impact on QLP followed by canopy cover of old trees and AG (see Pareto graph, ).
In the multiple regression derived for middle-aged forest stands, stand volume (VolTotal) was the variable with the greatest effect on QLP (), followed by age of old trees (AgeOld), and an index of structural similarity between young and old trees (DFI). This regression contained indicators that represented six out of the eight groups of diversity indicators (only species evenness and woody debris were not included).
The multiple regression for young forest stands contained five significant variables, of which two were covariates (stand volume [VolTotal], age of young trees [AgeYoung]), one represented species richness (R2), one species similarity between young and old trees (BC1) and one vertical structure (PmS). Stand volume had the greatest effect on QLP, as was also the case for middle-aged stands (). In the uneven-aged stands, the range of tree heights (Var1) had the greatest impact on QLP (). This regression contained only diversity indicators representing vertical structure ().
4. Discussion
Our results indicate that structural diversity had a greater effect on the proportion of best quality timber than species diversity, since the regressions comprised more structural indicators than species indicators (, ). In addition, species diversity indices had usually a lower impact on the proportion of best quality logs than structural diversity indicators, since their beta coefficients were smaller compared to those for structural diversity indicators (). The results also confirmed our hypothesis that the impact of species and structural diversity changed with stand age. In young stands, species diversity seemed to be more important for generating timber of high quality, while in old stands horizontal structure of the stands was more influential. In middle-aged stands, the impact of species and structural diversity on the proportion of high-quality logs was the most complex, because the derived regression contained indicators representing all groups of diversity except for species evenness and woody debris (). In uneven-aged stands, vertical structure seemed to be the diversity feature controlling log quality, since the regression comprised only indicators of vertical diversity (number of tree layers and range of tree heights) and a covariate ().
Most diversity indicators had a positive impact on the proportion of high-quality logs (), which indicated that promoting greater stand species and structural diversity can, at the same time, result in better timber quality. All significant vertical diversity indicators included in the regressions were positively correlated with the proportion of best quality logs (). This is in accordance with silvicultural experiments that have shown the positive impact of multi-layer vertical structure on overall stand productivity (Coomes et al. Citation2014; Pretzsch Citation2014; Jucker et al. Citation2015).
Horizontal structure had the most significant impact on QLP in old stands, where it was represented by two indicators (, ): SM and AG. Both positively affected the proportion of best quality logs. The values of these two diversity indicators increase with increasing mixture and intermingling of tree species in the stand (). This result suggested that better log quality can be expected in the old stands characterised by greater intermingling of species in the stand. This positive effect can be explained by so-called complementary demands for resources of different tree species, which has been demonstrated in different studies (e.g. Morin et al. Citation2011; Jucker et al. Citation2015) and has been shown to increase with age (Cardinale et al. Citation2007). Similarly, Liang et al. (Citation2007) found that timber quality depends on the spatial distribution of trees.
The last structural diversity indicator in the regression for old stands was the ratio of volume of fine woody debris to coarse woody debris (PmT) (, ), which had a negative impact on QLP. The group of old stands in our study included stands in the regeneration period. More fine woody debris in a stand suggested that when coarse timber was extracted, fine material was left on the site. The previous felling may have removed higher quality trees, leaving those of lower quality for the next felling. In addition, overstory trees remaining in a stand develop, creating larger crowns in reaction to changes in the local competitive environment induced by canopy openings (Jucker et al. Citation2015), with proportionately higher investment in crowns or fine material than in high-quality boles. Hence, the next felling may generate more fine woody debris that usually remains in the stand. Thus, the combination of extraction of higher quality trees during first rotation fellings and the subsequent increase in crown biomass on remaining trees may result in a negative relationship between PmT and QLP.
The positive relations of all significant diversity indicators to the proportion of high-quality logs in middle-aged stands () indicated that the timber quality was higher in the stands that were more differentiated in their species composition and stand structure. Our results suggest that promoting species and structural diversity by thinning may increase the quality of the remaining stand and generate more future income from the mature forest. In middle-aged stands, future timber production is improved by thinning treatments that influence the growth of individual trees (Pretzsch Citation2009). Several authors (Danilović Citation2008; Prka & Krpan Citation2010) have shown thinning may greatly improve final timber quality, as this changes the final habitus of individual trees, which improves log quality for a given tree (Prka Citation2012). In addition, species diversity may help increase forest productivity by promoting denser and more structurally complex canopies (Jucker et al. Citation2015). Hence, thinning treatments that increase species and structural diversity of stands may generate both higher timber production and quality.
Four out of five significant species diversity indices for the even-aged stands were positively correlated with the proportion of best quality logs (). This suggested that when species diversity was higher, the proportion of best quality logs was greater for such stands. This is in accordance with Liang et al. (Citation2007), who found that the proportion of peeler logs was positively correlated with species diversity. This finding may help to promote mixed forest stands for forestry, although the timber yields of mixtures may not always reach the timber yields of pure stands (Knoke et al. Citation2008).
The only exception was the BC1 species similarity index between young and old trees in the regression for young stands, which had a negative relationship with the proportion of best quality logs (). The value of this indicator is 0 if the species composition of the two classes of trees is the same, and increases as the differences in the species composition of the tree classes increase, to a maximum value of 1. Hence, its negative impact suggested that the proportion of high-quality logs was greater in stands with fewer species. A slight tendency towards lower stem quality with increasing tree species diversity on the stand level was also reported by Benneter and Bauhus (Citation2014). Nevertheless, the negative effect of BC1 was smaller than the positive influence of the ratio between the numbers of old trees and young trees representing vertical structure and the R2 index of species diversity, since the absolute value of the beta coefficient of BC1 was smaller than the beta coefficients of the other two diversity indicators (). Hence, overall we can state that in young stands the proportion of high-quality logs increased with increasing stand species and structural diversity.
The present study indicated that under the conditions encountered in Central Europe, species and structural diversity and quality of timber production are not in conflict to each other. Hence, promoting both biologically and structurally diverse stands may enhance the economic value of the final timber produced from temperate forests of Central Europe. Similarly, Benneter and Bauhus (Citation2014) showed that diverse stands are capable of providing highly valuable timber while at the same time providing other ecosystem services.
The fact that species and structural diversity indicators explained less than 50% of the total variance in QLP points to the influence of other factors on log quality. In addition to already mentioned management treatments, site conditions also play an important role (Danilović Citation2006). For example, Jucker et al. (Citation2014) presented that the productivity of mixed stands may be significantly hampered by drought. More research is needed on the relationship between log quality, diversity and other factors. Such studies provide us with information needed to modify modern forest management to support sustainability.
Disclosure statement
No potential conflict of interest was reported by the authors.
Additional information
Funding
References
- Baroni-Urbani C, Buser MW. 1976. Similarity of binary data. Syst Zool. 25:251–259.
- Belote RT, Prisley S, Jones RH, Fitzpatrick M, de Beurs K. 2011. Forest productivity and tree diversity relationships depend on ecological context within mid-Atlantic and Appalachian forests (USA). For Ecol Manage. 261:1315–1324.
- Benneter A, Bauhus J. 2014. Effects of tree species diversity on stem quality: plot and tree level results from six European regions. Int For Rev. 16:141. ISSN: 1465-5498 (print), 2053-7778 (online).
- Berger WH, Parker FL. 1970. Diversity of planktonic Foraminifera in deep sea sediments. Science. 168:1345–1347.
- Boyce R. 2003. Choosing the best similarity index when performing fuzzy set ordination on abundance data. Poster Session 2: Statistical Ecology and Ecological Modeling.ESA annual Meeting. [cited 2015 Feb 28]. Available from: http://www.nku.edu/~boycer/ESA03b.pdf
- Bray JR, Curtis JT. 1957. An ordination of the upland forest communities of southern Wisconsin. Ecol Monogr. 27:325–349.
- Brillouin L. 1956. Science and information theory. New York (NY): Academic Press.
- Bruciamacchie M. 1996. Comparison between indices of species diversity. Munich. 3/96:14.
- Cardinale BJ, Wright JP, Cadotte MW, Carroll IT, Hector A, Srivastava DS, Loreau M, Weis JJ. 2007. Impacts of plant diversity on biomass production increase through time because of species complementarity. Proc Natl Acad Sci U S A. 104:18123–18128.
- Coomes DA, Flores O, Holdaway R, Jucker T, Lines ER, Vanderwel MC. 2014. Wood production response to climate change will depend critically on forest composition and structure. Glob Chang Biol. 20:3632–3645.
- Czekanowski J. 1909. Zur differential Diagnose der Neandertalgruppe. Korrespondenz-Blatt Der Deutschen Gesellschaft Für Anthropologie. 40:44–47.
- Danilović M. 2008. Assessment of the effect of quality factors on the assortment structure in poplar plantations. Bull Fac For. 97:127–145.
- Danilović M. 2006. Effect of quality factors especially of sweep of poplar trees on assortment structure. Glasnik Schumarskog fakulteta. Beograd BIBLID. 94:135–150.
- Forest Research Agency. 2015. [cited 2015 Nov 24]. Available from: http://www.forestry.gov.uk/fr/glossary
- Fox TR, Haas CA, Smith DW, Loftis DL, Zedaker SM, Jones RH, Hammett AL. 2006. Alternative silvicultural practices in appalachian forest ecosystems: implications for species diversity, ecosystem resilience, and commercial timber production. In: Buckley DS, Clatterbuck WK, editors. Proceedings of 15th Central Hardwood Forest Conference held at the University of Tennessee; February 27 – March 1, 2006. Asheville (NC): U.S. Department of Agriculture Forest Service, Southern Research Station; p. 276–280.
- Gadow K. 1999. Waldstruktur und Diverzität. Afjz. 170:117–121.
- Gadow K, Hui G. 1999. Modelling forest development. Dordrecht: Kluwer Academic.
- Gschwantner T, Schadauer K, Vidal C, Lanz A, Tomppo E, Di Cosmo L, Robert N, Englert Duursma D, Lawrence M. 2009. Common tree definitions for national forest inventories in Europe. Silva Fennica. 43:303–321.
- Guldin JM, Fitzpatrick MW. 1991. Comparison of log quality from even-aged and uneven-aged Loblolly pine stands in south Arkansas. South J App For. 15:10–17.
- Heip C. 1974. A new index measuring evenness. J Marine Biol Assoc. 54:555–557.
- Hill MO. 1973. Diversity and evenness: a unifying notation and its consequences. Ecology. 54:427–432.
- Humphrey JW, Watts K. 2004. Biodiversity indicators for UK managed forests: development and implementation at different spatial scales. EFI-Proceedings. 51:79–89.
- Jucker T, Bouriaud O, Avacaritei D, Dănilă I, Duduman G, Valladares F, Coomes DA. 2014. Competition for light and water play contrasting roles in driving diversity-productivity relationships in Iberian forests. J Ecol. 102:1202–1213.
- Jucker T, Bouriaud O, Coomes DA. 2015. Crown plasticity enables trees to optimize canopy packing in mixed-species forests. Funct Ecol. 29:1078–1086.
- Kint V, Lust N, Ferris R, Olsthoorn AFM. 2000. Quantification of forest stand structure applied to scots pine (Pinus sylvestris L.). forests. Invest Agr: Sist Recur For: Fuera de Serie. 1:17.
- Knoke T, Ammer C, Stimm B, Mosandl R. 2008. Admixing broadleaved to coniferous tree species: a review on yield, ecological stability and economics. Eur J Forest Res. 127:89–101.
- Krebs CJ. 1989. Ecological methodology. New York (NY): Harper and Row; 471 p.
- Krejzar T, Bělská M, Bezděčková L, Bílý J, Buchta N, Činka M, Daňhelka M, Dvořák P, Fabiánek P, Hána J, et al. 2015. Zpráva o stavu lesa a lesního hospodářství České republiky v roce 2014. Report on the State of Forests and Forestry in the Czech Republic. Ministerstvo zemědělství Praha. [cited 2015 Nov 24]. Available from: http://www.uhul.cz/ke-stazeni/informace-o-lese/zelene-zpravy-mze.
- Lance GN, Williams WT. 1966. Computer programs for hierarchical polythetic classification (“similarity analysis”). Computer J. 9:60–64.
- Lei X, Wang W, Peng C. 2009. Relationships between stand growth and structural diversity in spruce-dominated forests in New Brunswick, Canada. Can J For Res. 39:1835–1847.
- Liang J, Buongiorno J, Monserud RA. 2007. Optimizing wood quality and stand diversity in uneven-aged forest management. In: Dykstra DP, Monserud RA, editors. Forest Growth and Timber Quality: Crown Models and Simulation Methods for Sustainable Forest Management. Proceedings of an International Conference, 2007 Aug 7–10; Portland, OR. Gen. Tech. Rep. PNW-GTR-791. Portland (OR): U.S. Department of Agriculture, Forest Service, Pacific Northwest Research Station; p. 169–176.
- Long JN, Shaw JD. 2010. The influence of compositional and structural diversity on forest productivity. Forestry. 83:121–128.
- Ludwig JA, Reynolds JF. 1988. Statistical ecology a primer on methods and computing. New York (NY): John Willey & Sons.
- Macdonald E, Gardiner B, Mason W. 2010. The effects of transformation of even-aged stands to continuous cover forestry on conifer log quality and wood properties in the UK. Forestry. 83:1–16.
- Magurran AE. 2004. Measuring biological diversity. Oxford: Wiley-Blackwell.
- Margalef R. 1958. Information theory in ecology. Gen Systematics. 3:36–71.
- Marquardt DW. 1970. Generalized inverses, ridge regression, biased linear estimation, and nonlinear estimation. Technometrics. 12:591–256.
- McIntosh RP. 1967. An index of diversity and the relation of certain concepts to diversity. Ecology. 48:392–404.
- Menhinick EF. 1964. A comparison of some species – individuals diversity indices applied to samples of field insects. Ecology. 45:859–861.
- Merganič J, Marušák R, Merganičová K, Tipmann L, Stolariková R, Audolenská V. 2012. Sampling design for integrated noncash and economic valuation of biodiversity – case study. Scientia Agriculturae Bohemica. 43:145–152.
- Merganič J, Merganičová K, Marušák R, Audolenská V. 2012. Plant diversity of forests. In: Blanco JA, Lo YH, editors. 2012: Forest Ecosystems - More than Just Trees. InTech publisher; 464 p. p. 3–28. ISBN: 978-953-51-0202-1. [cited 2015 Nov 24]. Available from: http://www.intechopen.com/books/forest-ecosystems-more-than-just-trees.
- Morin X, Fahse L, Scherer-Lorenzen M, Bugmann H. 2011. Tree species richness promotes productivity in temperate forests through strong complementarity between species. Ecol Lett. 14:1211–1219.
- Neumann M, Starlinger F. 2001. The significance of different indices for stand structure and diversity in forests. For Ecol Manage. 145:91–106.
- Noss RF. 1990. Indicators for monitoring biodiversity: a hierarchical approach. Conservation Biol. 4:355–364.
- Oxford English Dictionary. 2015. [cited 2015 Nov 24]. Available from: http://findwords.info/term/assortment.
- Petráš R, Nociar V. 1990. Nové sortimentačné tabuľky hlavných listnatých drevín. Lesnícky Časopis. 36:535–552.
- Petráš R, Nociar V. 1991. Nové sortimentačné tabuľky hlavných ihličnatých drevín. Lesnícky Časopis. 37:377–392.
- Petráš R, Pajtík J. 1991. Sústava česko-slovenských objemových tabuliek drevín. Lesnícky Časopis. 37:49–56.
- Pielou EC. 1975. Ecological diversity. New York (NY): Wiley.
- Pielou EC. 1977. Mathematical ecology. New York (NY): Wiley.
- Plíva K. 1987. Typologický klasifikační systém ÚHÚL. Brandýs nad Labem: ÚHÚL.
- Pretzsch H. 2005. Diversity and productivity in forests: evidence from long-term experimental plots. In: Scherer-Lorenzen M, Körner C, Schulze ED, editors. Forest diversity and function: temperate and boreal systems. Berlin (Germany): Springer; p. 41–64.
- Pretzsch H. 2009. Forest dynamics, growth and yield. From measurement to model. Berlin: Springer-Verlag.
- Pretzsch H. 2014. Canopy space filling and tree crown morphology in mixed-species stands compared with monocultures. For Ecol Manage. 327:251–264.
- Prka M. 2012. Impact of sustainable management of natural even-aged beech stands on assortment structure of beech in Croatia. In: Oteng-Amoako, AA, editor. New Advances and Contributions to Forestry Research. InTech. [cited 2015 Feb 28]. Available from: http://www.intechopen.com/books/new-advances-and-contributions-to-forestry-research/impact-of-sustainablemanagement-of-natural-even-aged-beech-stands-on-assortment-structure-of-beech.
- Prka M, Krpan APB. 2010. Impact of tending measures on assortment structure of fellings in central Croatian beech stands. Acta Silv Lign Hung. 6:171–182.
- Quinn GP, Keough MJ. 2002. Experimental design and data analysis for biologists. New York (NY): Cambridge University Press.
- Shannon C, Weaver W. 1949. The mathematical theory of communication. Urbana (IL): University of Illinois Press.
- Simpson EH. 1949. Measurement of diversity. Nature. 163:688.
- Šmelko Š. 2007. Dendrometria. Zvolen: Vydavateľstvo TU Zvolen.
- Šmelko Š. 2010. New methodical procedures for the quantification of deadwood and its components in forest ecosystems. Lesn Čas – Forestry J. 56:155–175.
- Sørensen T. 1948. A method of establishing groups of equal amplitude in plant sociology based on similarity of species and its application to analyses of the vegetation on Danish commons. Biologiske Skrifter/Kongelige Danske Videnskabernes Selskab. 5:1–34.
- Suchomel J, Gejdoš M, Ambrušová L, Šulek R. 2012. Analysis of price changes of selected roundwood assortments in some Central Europe countries. J For Sci. 58:483–491.
- ÚHÚL. 2007. National forest inventory in the Czech Republic 2001–2004. Introduction, methods, results. Brandýs nad Labem: ÚHÚL.
- Wang W, Lei X, Ma Z, Kneeshaw DD, Peng C. 2011. Positive relationship between aboveground carbon stocks and structural diversity in spruce-dominated forest stands in New Brunswick, Canada. For Sci. 57:506–515.
- Woodall C, Williams MS. 2005. Sampling protocol, estimation, and analysis procedures for the down woody materials indicator of the FIA program. General-Technical-Report-North-Central-Research-Station,-USDA-Forest-Service. (NC-256). Newtown Square: USDA Forest Service, Northern Research Station.
- Zlatník A. 1976. Lesnická fytocenologie. Praha: SZN.