Abstract
The CRISPR-Cas9 system, which uses sgRNA for targeting and the nuclease Cas9 for cleavage, has emerged as a versatile and efficient tool for genome engineering; the system has overcome the limitations of previous technologies and implements various gene editing strategies. The large-scale loss-of-function (LOF) gene scanning technology based on the CRISPR-Cas9 system can be utilized to reveal associations between the genotype and phenotype by inducing efficient and scalable gene perturbations throughout the whole genome. This technology is playing a breakthrough role in the exploration of genes associated with tumors and viruses and its expansion together with RNA sequencing (RNA-seq) can be used to assess multiplexed gene interactions as well as more complicated phenotypes. Here, we introduce LOF genetic screens based on CRISPR knockout (CRISPR ko) and its applications in synthetic lethality and virus-host interactions. We highlight the recent progress on combining this technique with single cell RNA-seq. We also compare the advantages and pitfalls of CRISPR variants, discuss the future perspectives of gene therapy and raise important considerations regarding off-target effects.
KEYWORDS:
CRISPR ko-mediated loss-of-function screens
Genetic screens aim to uncover the relationship between the genotype and phenotype by introducing perturbations into genes at a large scale. Loss-of-function (LOF) analysis elucidates the function of target genes by reducing or ablating the original genes, mRNA or proteins. By analyzing the changes in the phenotype that are induced by these perturbations, the functions of the wild-type genes can be deduced. LOF approach-based screens have opened unprecedented opportunities for discovering genes of interest. Genetic screens in Caenorhabditis elegans (Brenner Citation1974) and Drosophila melanogaster (Gans et al. Citation1975; Nüsslein-volhard and Wieschaus Citation1980) demonstrated the potential and efficiency of this technique for identifying genes that are essential for specific biological functions among large groups of genes through saturation mutagenesis.
A decade ago, the discovery of RNA interference (RNAi) technology suggested the possibility of silencing targeted genes in an individual. With the production of RNAi libraries, RNAi technology was developed for genome-wide LOF screening. Discoveries in C. elegans, Drosophila, and even human cells have been made with RNAi-based screening technologies (Conte and Mello Citation2003; Ali et al. Citation2009) Nevertheless, as the cleavage site is located in RNA rather than DNA, RNAi-based genetic screens lead to only partial and short-term suppression of genes rather than complete LOF mutation and the high off-target effects (Sigoillot et al. Citation2012; Khorashad et al. Citation2015) may cause false-positive observations. Thus, exploitation of a newer technology with a better targeting efficiency as well as fewer off-target effects is needed.
Accompanied by the emergence of clustered regularly interspaced short palindromic repeat (CRISPR) -Cas9 technology, by which a focused genomic locus can be precisely targeted, LOF screens represent a new era of gene knockout technology. The technology and its variants offer flexible and versatile platforms to utilize numerous genome editing strategies as well as genetic screens. Here we summarize the current CRISPR technologies for genetic pooled screens, focusing on the screening strategies, crucial steps and recent applications in cancer synthetic lethality and virus-host interactions of CRISPR ko technology, highlighting the important progress of RNA-seq based CRISPR screening.
CRISPR-Cas9 genome editing
The CRISPR-Cas9 system is regarded as an RNA-based adaptive immune system that evolved from bacteria and archaea and functions by using RNA-guided nucleases to attack invading viruses and plasmids (Horvath and Barrangou Citation2010; Wiedenheft et al. Citation2012). Three types of the CRISPR system (I-III) are found in bacteria and archaeal hosts, and all comprise a cluster of CRISPR-associated (Cas) genes, a regulatory leader sequence and a CRISPR RNA (crRNA) array. The crRNA array is formed by a distinctive array of repetitive elements interspaced by short variable sequences known as the ‘spacers’ that are derived from the invading pathogens.
The typeII CRISPR system exhibits a simple structure and easy design, consisting of a single guide RNA (sgRNA) fused by crRNA encoded by the crRNA array, an auxiliary transactivating crRNA (tracrRNA) and a Cas9 protein for cleavage. The mechanism consists of three steps: acquisition, expression and maturation, and interference. In the acquisition step, target DNA sequences are integrated as new CRISPR spacers into the CRISPR array to store the immunological memory. In the expression and maturation step, a pre-CRISPR RNA transcript (pre-crRNA) is transcribed from the CRISPR locus which also encodes a transactivating RNA (tracrRNA) with complementarity to the repeat regions of pre-crRNA. The maturation of the active crRNA is directed by the tracrRNA that induces the activities of the Cas proteins and the widely conserved endogenous RNase III to facilitate the cleavage of pre-crRNA into crRNA (Deltcheva et al. Citation2011). Furthermore, the Cas9 gene expresses the double-stranded DNA endonuclease that is combined with sgRNA to form the sgRNA-Cas9 complex. In the interference stage, the sgRNA can specifically target foreign genes through crRNA recognizing the complementary nucleic acid by base-pairing and guides Cas9 proteins for cleavage. Specifically, the essential cleavage depends on the recognition of a specific DNA array, termed the protospacer adjacent motif (PAM), located near the exogenous target sequence known as the protospacer, which does not exist in the host genome, preventing the endogenous cleavage of self-DNA.
In the type II CRISPR system, the specific nuclease Cas9 functions as a molecular scissor to induce a double-strand break (DSB) at the target genomic locus, which is then repaired via nonhomologous end-joining (NHEJ) and homology directed repair (HDR) (Jinek et al. Citation2012). Generally, NHEJ occurs more frequently than HDR because a homologous template is required to exchange or insert genes at the target site by HDR, which may make precise gene replacement possible. However, NHEJ is often error prone and small insertions or deletions can be induced that potentially result in frameshift indel mutations and abolish gene function. Thus, the CRISPR-Cas9 system has laid the foundation for the LOF analysis of individual gene and exploration of gene functions. (Jiang et al. Citation2013; Wang et al. Citation2013; Cong and Zhang Citation2015).
LOF genetic screens using CRISPR knockout
CRISPR-Cas9 technology not only deletes specific single-loci of interest in prokaryotic organism or mammalian animals but also allows more efficient access to genetic screens with fewer off-targets. In wild-type (wt)-CRISPR-Cas9-mediated screens, an sgRNA composed of crRNA and tracrRNA can be designed for any target of interest and coinjected with a designed Cas9 into host cells via vectors (Cho et al. Citation2013; Habib et al. Citation2013). The Cas9 protein can be directed towards the target sequence near a specific PAM sequence for cleavage. The system causes mutation at the DNA level, resulting in permanent and heritable gene knockout, thereby leading to a clear phenotype for LOF screening.
Generally, two screening formats are used: an arrayed screen and a pooled screen. In an arrayed screen, each cell well contains a specific CRISPR reagent targeting an individual gene whose sequence is known in advance. It is easy to speculate the gene function which is correlated with the observed cell phenotype. Such a screen allows researchers to acquire comprehensive and reliable details regarding the gene perturbation effects. However, to perform large-scale LOF screening, arrayed formats can be used as a low-efficiency method at the expense of financial and material resources.
In contrast, in a pooled array, each cell line is randomly transfected with a sgRNA library to introduce diverse gene mutations, and a low multiplicity of infection (MOI, usually 0.4∼0.6) transduction (Shalem et al. Citation2014; Wang et al. Citation2014) is used to ensure that each cell is targeted by only a single sgRNA. The library is capable of carrying thousands of sgRNAs. In practice, researchers must choose libraries according to experimental purposes. An available pre-made library, such as the most widely used KO library GeCKO designed by Zhang et al. (Shalem et al. Citation2014), may not ideally match the cell lines of interest, while developing a new library for genes of interest in cell lines is a laborious and time-consuming process. For example, Zhu et al. used paired sgRNAs targeting approximately 700 human lncRNA genes to identify functional cancer long noncoding RNAs (lncRNAs) (Zhu et al. Citation2016). Methods of optimizing libraries have recently been elucidated. In a recent study, Sanson et al. (Citation2018) developed a more effective library for essential gene depletion called Brunello comprising 77441 sgRNAs and 1000 non-targeting controls. In a gold-standard test to target 1580 essential genes as well as 927 non-essential genes, the area under the curve (AUC) was calculated to perform evaluation. (Hart et al. Citation2015, Citation2018). The Brunello library showed an AUC of 0.8 (usually >0.5) when targeting essential genes but an AUC of 0.42 (usually ≤0.5) when targeting non-essential genes, outperforming many other libraries (Hart et al. Citation2015; Tzelepis et al. Citation2016; Aguirre et al. Citation2017; Wang et al. Citation2017). Additionally, Brunello effectively modulated targeted genes with only 4 sgRNAs per gene to provide sufficient screening information, while the figures for GeCKOv2 and GeCKOv1 are 6 and 3–4 respectively, showing the high efficiency of Brunello (Sanson et al. Citation2018).
Under a phenotypic LOF screen, negative or positive selection can be utilized to select cells with a phenotype of interest. sgRNAs negatively selected will be depleted in the selected cells, and this strategy can be used to select drug-targeting genes for cancer treatment. In contrast, positive selection identifies genes in survival cells whose perturbations are protective, thereby conferring resistance to a selective environment, such as that induced by puromycin treatment. After phenotypic selection, genomic DNA will be extracted from selected cells, and sgRNA domains will be amplified by PCR. Each sgRNA will typically be sequenced by Next Generation Sequencing (NGS), and their frequencies in different periods for example, the beginning or end of the screen will be compared to identify the most depleted or enriched sgRNAs (Figure ). To identify negatively or positively selected sgRNAs, several algorithms are used to calculate the readout. MAGeCK developed for CRISPR-Cas9 knockout screens has been the most common way to dissect essential genes, taking advantage of controlling the false discovery rate (FDR) as well as a high sensitivity (Li et al. Citation2014). Recently a new protocol named MAGeCKFlute combining MAGeCK and MAGeCK-VISPR was shown to feature multiple functions, including quality control, beta score normalization, bias correction and identification and analysis of hit genes. This protocol requires ∼3 h to finish all the calculations and shows a comprehensive analytical ability (Wang et al. Citation2019). When completing hit identification, some follow-up studies, such as an in vivo test or a second screen (Wang et al. Citation2019), will be performed to validate the functions of the top-depleted or top-enriched genes. Saving time and effort, massive pooled CRISPR screening appears to be used more widely than the arrayed format.
Exploration of synthetic lethal genes
The occurrence and development of tumors is a highly complicated process due to the interactions of cancer-related genes and their multiple pathways. With advances in tumor genome sequencing and gene editing technologies being used to identify essential tumor genes, the mechanisms of cancer occurrence, development, metastasis and drug resistance are more clearly and deeply understood. The emerging high-throughput genetic screens with CRISPR-Cas9 can be used to dissect associations between tumor genotypes and phenotypes, leading to improvements in disease diagnosis, tumor treatment and drug resistance.
Precise cancer treatments focusing on targeting cancer phenotypes outperform standard cancer therapies with the advantages of individuation, accuracy and low side effects, becoming the first choice for applicable patients, and the targeted drug imatinib serves as an example. In fact, imatinib effectively kills tumor cells in patients with gastrointestinal stromal tumors (GIST) or chronic myelogenous leukemia (CML) due to the ‘oncogene addiction’ (Pagliarini et al. Citation2015) phenomenon, in which genes such as KIT in GIST or BCR/ABL in CML are indispensable for cancer cell survival. Thus, targeting these oncogenes can suppress tumor growth. However, some of these targeted genes undergo ‘oncogene escape’ to alter their addictive characteristic after a period of treatment, resulting in drug resistance, but the mechanisms underlying this phenomenon remain incompletely understood. To overcome this problem, targeting an essential gene at a second site to cause both oncogenic and non-oncogenic mutations is important. The term ‘synthetic lethality’ refers to the use of perturbations in combination to induce more effective defects in the cell or organism compared to those achieved with each perturbation alone. In the context of tumors, the survival of a cancer cell with a specific gene mutation is dependent on the viability of a synthetic lethal partner gene (Pagliarini et al. Citation2015) (Figure ). This concept was first proposed 20 years ago and provided a way to find new cancer target sites and to block drug resistance. Poly(ADP-ribose) polymerase (PARP) inhibitors are the first tumor drugs based on the concept of synthetic lethality, which function by the synthetic lethal interaction of poly(ADP-ribose) polymerase (PARP) and the mutated tumor suppressor genes BRCA1 and BRCA2 in breast or ovarian cancers, now widely used in clinical (Fong et al. Citation2009; Hutchinson Citation2010).
Figure 2. The concept of synthetic lethality In the simplest form, the simultaneous perturbation of two genes (shown here as gene A and B) leads to cell death, while perturbation of single gene does not. In the context of tumors, gene A can represent an oncogene and gene B can be identified as its synthetic lethal partner gene. The red crosses denote a mutation or a pharmacological inhibition.
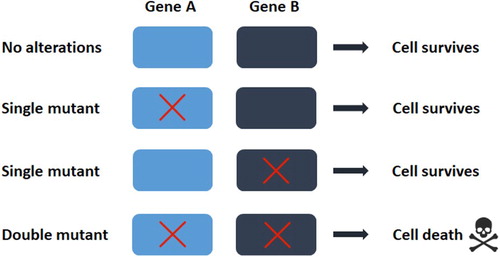
In a genome-wide screens using CRISPR-Cas9 for synthetic lethality, a library of sgRNAs designed to target various DNA sequences will be infected through lentivirus into both a wild-type cell line and a mutant-type cell line that contains mutant or inactive genes of interest. The two cell lines will be cultivated, and the DNA sequences of the next generation will be isolated and amplified by PCR. Compared with the wild-type cell group, the cell underrepresented or dead are the candidate cells whose DNA targeted by sgRNAs are the candidates for the synthetic lethal partners of the genes of interest.
In fact, synthetic enhancers and synthetic lethal phenomena can occur in metabolic genes, which promote tumorigenesis and tumor proliferation by affecting the metabolic pathways and the tumor environment. The RAS family of oncogenes (KRAS, NRAS, and HRAS) is one of the most common and lethal genes that causes mutations and subsequent tumor occurrence. In the past ten years, researchers have utilized various methods to target the RAS downstream proteins, because of the difficulty in targeting RAS members. Recently, researchers constructed a KRAS mutant colorectal cancer cell model (HCT116mut) and then transduced HCT116wt and HCT116mut cells with the human GeCKOv2 library to conduct a whole genome LOF screening for genes of synthetic lethal potential. Inactivating both NADK and KHK metabolic enzyme genes reduced the growth of mutant cancer cells in mice by 50% (Yau et al. Citation2017), showing the effectiveness of CRISPR ko screening for the elucidation of therapeutic targets of cancers driven by oncogenes.
Screening for possible synthetic lethality sites used for antitumor targeting drugs has been performed for the past 20 years. CRISPR-Cas9 technology has advanced the methodologies used to reduce drug resistance. In a recent study, Hinze et al. found a synthetic lethal interaction between asparaginase and Wnt pathway activation in asparaginase-resistant acute leukemias. Asparaginase is regarded as an effective antilymphoma drug due to its ability to deaminate asparagine, which is essential for the survival of acute leukemia cells (New and Medical Citation1963). Nevertheless, when tumor cells develop drug resistance to asparaginase, patients have to face the situation of no cure and poor prognosis. It is therefore imperative to identify a synergistic gene whose deletion will improve the antitumor effect of the enzyme. Hinze et al. (Citation2019) have screened a genome wide CRISPR-Cas9 library against the most asparaginase-resistant T cell acute lymphoblastic leukemia (T-ALL) cell line CCRF-CEM to perform LOF screen to identify therapeutic targets for drug-resistant ALL. A GeCKO v2 human library and a lentiCas9-blast vector containing a self-excising GFP construct used for the Cas9 activity reporter were induced in each CCRF-CEM cell. Then the authors treated the cell lines with 10 U/L asparaginase for 5 days and then extracted DNA. sgRNA read counts were obtained by NGS (NextSeq 500) and calculated by MAGeCK software. Two negative regulator genes of Wnt signaling, NKD2 and LGR6, were the most significantly depleted genes in addition to the asparagine synthetase gene, and their LOF leading to sensitivity to asparaginase was confirmed by shRNAs knockdown. Hinze et al. documented that Wnt pathway activation plays a critical role in asparaginase sensitivity and tested whether the LOF of glycogen synthase kinase 3 (GSK3) has the same antidrug-resistant action as that of NKD2 and LGR6 due to the fact that repression of GSK3 is a key process by which Wnt induces downstream signals. The team carried out a series of follow-up experiments to demonstrate that inhibiting the alpha isoform of GSK3 can simulate the Wnt-dependent stabilization of proteins (Wnt/STOP) to reduce asparaginase resistance. While CRISPR-Cas9 LOF screens offer an effective strategy for identification of synergistic gene interactions, Hinze et al. noted that some asparaginase sensitization genes, such as GSK3A, EIF2AK4 and ATF4, are not among the top hits in the screen because of the coverage of the sgRNA library, which contains relatively few sgRNAs that target genes encoding functional proteins. Thus, additional efforts to design a more comprehensive library should be made.
Nevertheless, as a deep understanding of important genetic interactions is lacking, very few drugs based on synthetic lethal gene interactions progress to clinical trials. In fact, synthetic lethal gene pairs are rare, and their interaction phenotypes vary by cellular conditions as well as cancer types, which makes genome-wide screening with one gene mutation per cell a large project. Shen et al. developed a library targeting 73 cancer genes with dual-sgRNAs to map genetic interactions (Shen et al. Citation2017). From the same angle, two other strategies, termed CombiGEM (Wong et al. Citation2016) and CDKO (Han et al. Citation2017) constructed vector with double sgRNA libraries. However, to explore genetic interactions in cancer cells, this type of screen can assess only the impacts of fitness on cell groups. With the emerging approach of combining RNA-seq and CRISPR pooled screens, we may be able to clearly identify multiple gene interactions under different cell environments on an individual cell basis in the future.
Investigation of gene functions in virus-host interactions
The fight against viruses has long been a hot topic worldwide. Viruses recognize cell surface receptors and are absorbed by and then enter the hosts through three major mechanisms: endocytosis, penetration and transposition. In the cytoplasm, after uncoating, the virus interacts with the host and completes the processes of biosynthesis, assembly and release to complete the viral replication cycle. Because this process ultimately induces cytolytic death or chronic stable infection in the host, exploration of the cell surface receptors and signaling pathways that may act as promoters or suppressors is needed. For example, the cell surface protein CCR5 has been found to be a critical accessory receptor of HIV-infecting lymphocytes (Tebas et al. Citation2014). In some Europeans, the CCR5 gene lacks 32 bp, which prevents them from being infected by HIV (Liu et al. Citation1996). Therefore, studying the host factors has substantial value. Large-scale gene editing by insertional mutagenesis in haploid cells and RNAi have played important roles in elucidating the host’s role in viral infection, but this method is still limited by serious off-target effects. Today, CRISPR-Cas9 has become a new method for studying host gene phenotypes, providing a new foundation for antiviral therapy.
To identify promoter factors, we can conduct the gain-of-function strategy in nonpermissive host cells and the LOF strategy in permissive host cells. In contrast, to identify suppressor factors, we can utilize the LOF strategy in nonpermissive host cells and the gain-of-function strategy in permissive host cells. In LOF screens, the pooled edited cell population is subjected to a virus infection assay, using the wild-type virus, control virus or reporter virus for fluorescence analysis. We then perform phenotype selection according to the survival or death of the host cells. In particular, when faced with a non-cytolytic virus, host cells need to be analyzed for the abundance of fluorescence carried by the virus of interest to determine the positive phenotype rather than the state of survival. NGS will identify the candidate genes targeted by sgRNAs through comparison of the wild-type and control groups, while the gain-of-function strategy utilizes CRISPRa and ectopic overexpression more often.
To date, researchers have elucidated a variety of relationships between hosts and viruses using CRISPR-Cas9 screens. For example, hepatitis C is an infectious disease caused by hepatitis C virus (HCV) that primarily affects the liver. ELAVL1, an RNA-binding protein encoded by the ELAVL1 gene, was found to be a critical receptor for HCV entry (Marceau et al. Citation2017). Furthermore, a research team constructed a sgRNA plasmid library containing 30,840 pairs of oligonucleotides targeting 10,280 human genes, which were cotransduced with plasmids containing Cas9 into human cells. These human cells were then treated with a human pathogenic virus termed enterovirus D68 (EV-D68) to identify essential genes for viral infection and replication. Researchers identified and confirmed authentic mutations of genes targeted by sgRNAs through whole genome sequencing, and the colonies with two disrupted genes, the ST3GAL4 gene encoding ST3 β-galactoside α-2,3-sialyltransferase 4 and the COG1 gene encoding a component of the oligomeric Golgi complex 1 were shown to be virus-resistant. In addition, the other two colonies harboring mutations in the MGAT5 gene, which encodes the mannosyl (α-1,6-)-glycoprotein β-1,6-N-acetyl-glucosaminyltransferase, and the COG5 gene, which encodes a component of the oligomeric Golgi complex 5, were also resistant to EV-D68 virus entry. In view of Baggen’s discovery, the ST3GLA4 and MGAT5 genes are critical for EV-D68 infection by facilitating the sialic conjugation needed for EV-D68 entry, and the conserved oligomeric Golgi (COG) complex is proposed to function in the cell glycosylation process (Baggen et al. Citation2016); researchers deduced that the COG1 and COG5 genes might also play an important role in the sialic conjugation process and finally demonstrated their role in presenting sialic acid on the cell surface (Kim et al. Citation2017). A CRISPR-Cas9 genome-wide scan performed to elucidate the relationship between hosts and viruses has narrowed the search scope and highlighted a more efficient direction for furthering antivirals therapeutics.
CRISPR variations
As researchers have a deeper understanding of CRISPR-Cas9 technology, the applications are not limited to simple DNA sequence editing. Emerging dead-Cas 9 (dCas9) technology has become an effective, nonmutagenic gene regulatory method. Researchers have induced site-directed mutations of H840A and D10A, which superlatively belong to the two endonuclease-active domains HNH and RuvC of the Cas9 protein to prevent cleavage of the gene of interest by Cas9. Although the dCas9 protein is unable to cleave DNA, it can still be localized to the target gene by sgRNA and activate (CRISPRa) or inhibit (CRISPRi) target genes.
In a CRISPRi system, the catalytically dead Cas9 protein, which is fused to transcriptional repressors (e.g. KRAB), is recruited to the transcription start site (TSS) of a specific sgRNA targeting gene. Unlike the knockout of genes, CRISPRi suppresses genes in a nonpermanent, reversible gene manner through two methods: (1) inhibition of transcription initiation by blocking the binding of RNA polymerase to DNA promoters and (2) inhibition of transcriptional elongation via steric hindrance. Although this method was first demonstrated in E. coli and shown to have substantial inhibitory activity (Ji et al. Citation2014), researchers have now successfully repressed endogenous mammalian genes, such as those encoding tumor protein 53 and the transferrin receptor CD71 (Gilbert et al. Citation2013). CRISPRi allows genome-wide unbiased screening for essential genes especially for lncRNAs. lncRNAs are defined as RNA transcripts >200 nucleotides long that have no obvious protein-coding capacity, and cannot be easily targeted by RNAi or CRISPR ko for relatively small deletions (Shalem et al. Citation2014; Wang et al. Citation2014; Shi et al. Citation2015). CRISPRi with larger genetic deletions can be high efficient to cause perturbations (Yin et al. Citation2015; Groff et al. Citation2016; Paralkar et al. Citation2016; Zhu et al. Citation2016),. In one study a non-coding sgRNA library called ‘CRiNCL’ was used to target 16401 lncRNAs in 7 distinct cell lines, and 499 essential genes were found to be critical for cell growth, demonstrating that CRISPRi is a highly efficient screening protocol despite its off-targets effects (Liu et al. Citation2017). Some new technologies combining CRISPRi and RNA-seq have emerged, such as Mosaic-seq (Xie et al. Citation2017) and CRISPRi-seq (Adamson et al. Citation2016), and both of these strategies employ a barcode strategy and a droplet approach to screen with dCas9-KRAB libraries. CRISPRi strongly repress genes with a high efficiency (Dominguez et al. Citation2016) and has advantages over RNAi that include fewer false-positive results (Smith et al. Citation2017a; Smith et al. Citation2017b) and off-target effects (Gilbert et al. Citation2014). Recently, the powerful technology called Mosaic-seq combined with CRISPRi and single-cell RNA-seq was able to perform a high-throughput assessment of the role of enhancers in gene regulation and their links to promotors, showing the bright the future of dissecting complicated gene interactions with CRISPRi.
CRISPR-dCas9 can promote the transcription of target genes facilitated by dCas9-sgRNA complex binding activators, which promotes the binding of RNA polymerase to promotors. In mutant E. coli lacking the ω-subunit of RNA polymerase, the fused expression of dCas9-ω and its corresponding sgRNA boosts the binding of RNA polymerase to promotors (Bikard et al. Citation2013), thereby enhancing the transcription of target genes. CRISPRa was first used to search for promotors demonstrating its potential for robust genome gain-of-function scanning. Initial gene activations induced by CRISPRa are not sufficient. However, the binding of dCas9 to more than one activiator (VPR SYSTEM) (Chavez et al. Citation2015) or synergistic activation (SAM SYSTEM) (Konermann et al. Citation2015) led to robust activation, showing its potential for large scale gain-of-function screening. Recently, a group of scientists applied CRISPRa to identify the enhancers involved in T cell immune functions. The IL2RA gene can modulate T cells to either play a normal anti-inflammatory role or to attack our own tissues and organs, leading to autoimmune diseases such as Crohn's disease and ulcerative colitis. To track the location of IL2RA enhancers, researchers transduced a library of more than 20000 sgRNAs into Jurkat-dCas9-VP64 cells, finding key sites for regulating T cells (Simeonov et al. Citation2017).
Unlike CRISPR ko screens, perturbations induced by CRISPRi and CRISPRa are inducible and reversible, providing a way to dissect gene functions under different spatiotemporal backgrounds. CRISPRa has advantages over cDNA expression such as accurate endogenous gene regulation and not requiring initial cloning of the cDNA of interest. Nevertheless, one problem associated with both of CRISPRi and CRISPRa is their dependence on TSS. They may silence or activate both genes when TSS targeted regulates dual promotors (Rosenbluh et al. Citation2017). They may take a risk of off-target effects.
CRISPR screening combined with RNA-sequencing
Arrayed screens and pooled screens are widely used powerful strategies to systematically analyze gene function. Arrayed screens, which induce a single perturbation at a time, make it possible to apply RNA-seq to offer detailed and complex information such as transcriptome profiling. However, arrayed screens are limited by high costs and availability of labor because cells transduced with various sgRNAs have to be isolated and cultured. Pooled screens, which can perform more efficient and scalable parallel cell perturbations in thousands of cells, allow the selection of only crude phenotypes, such as cell survival or reporter gene expression. These low-content readouts lose information at molecular level which is more informative results of cellular response (Rédei Citation2007) and lead to high false-positive rates.
In the past decade, the rapid-development of single cell technology has enabled us to gain knowledge of genome-wide quantification of mRNA transcripts at the single-cell level, which furthers our ability to acquire rich information for characterizing cellular status and molecular circuitries (Dunham et al. Citation2012). However, such technology can reason only natural cellular response, lacking the ability to elucidate causal gene perturbations. Several studies termed CRISP-seq (Jaitin et al. Citation2016), PERTURB-seq (Chen et al. Citation2016) and CROP-seq (Datlinger et al. Citation2017) report new screening methods that combine CRISPR-based gene perturbations with single-cell RNA sequencing to obtain a rich genomic readout, which not only perform gene knockout or repression but also realize specific analysis of sgRNA-induced transcriptional profiles.
Dixit et al. (Chen et al. Citation2016) developed Perturb-seq to associate sgRNA-induced perturbations by an expressed guide barcode (GBC) with individual cell transcriptome tagged by a unique cell barcode (CBC) and a unique molecular identifier (UMI). They used this method to complete the CRISPR-Cas9 knockout analyzing 200,000 immune cells and cell lines; in a companion study on the mammalian unfolded protein response (UPR), Adamson et al. combined Perturb-seq with CRISPRi screening (Adamson et al. Citation2016). To perform Perturb-seq (Chen et al. Citation2016), a lentivirus backbone is engineered first, which includes an ORF encoding a BFP-T2A-Puromycin, an sgRNA and its U6 promoter (Figure A). In addition, the GBC expression cassette is inserted to identity its corresponding sgRNA, and their association is determined by Sanger sequencing. To prepare a single cell library, an individual mRNA is paired with CBC and UMI based on a droplet microfluidics method named DROP-seq (Zheng et al. Citation2017). In the droplets, CRISPR perturbed cells transduced with sgRNAs and paired GBCs are lysed under the action of cell lysis buffer, and the mRNA molecules from the individual cells are reverse transcribed with barcoded oligo-dT reverse transcription (RT) primers to form CBC/GBC cDNA libraries, which are then generated by NGS to associate the single-cell transcriptomes with GBC identities (Chen et al. Citation2016) (Figure ). Jaitin et al. (Citation2016) termed their approach CRISP-seq, similarly using transcribed barcodes for sgRNAs identification. To construct a CRISP-seq vector, a poly-adenylated unique guide index (UGI) to mark sgRNA and a fluorescent selection marker that allows for cell sorting and subsequent analysis of genotype-phenotype relationships are included.
Figure 3. A robust strategy combining CRISPR screening with RNA-sequencing Schematic of Perturb-seq vector and guide-mapping barcoded cDNA. CBC (cell barcode) index for cell. UMI (unique molecular identifier), index for mRNA counting to correct for duplicates. GBC (guide barcode), index for sgRNA. (B) Schematic of the Perturb-seq platform.
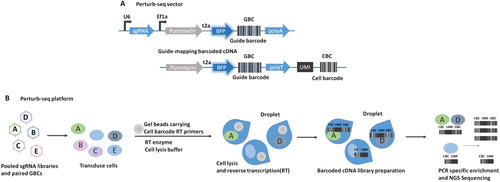
All the methods developed by Dixit et al. (Chen et al. Citation2016), Adamson et al. (Adamson et al. Citation2016), Datlinger et al. (Citation2017) and Jaitin et al. (Citation2016) are designed to overcome the technical problem of the sgRNA lacking a poly (A) tail and thus cannot be captured by most high-throughput single-cell RNA-seq methods which rely on the 3’-ends poly-A tail of captured mRNA. To address this problem, an RNA polymerase II-driven ‘‘sgRNAs identifier cassette’’ is inserted into both Perturb-vector (Chen et al. Citation2016) and Crisp-vector (Jaitin et al. Citation2016). Instead of using transcribed barcodes, the CROP-method (Datlinger et al. Citation2017) developed by Datlinger et al. has the key advantage of it directly reading RNA polymerase III-driven sgRNAs by integrating itself into a 400-bp lentivirus deletion site within the 3’ long terminal repeat (LTR). This study demonstrates that the hU6-sgRNAs insertion into the 3’ LTR can not only express functional sgRNAs but also be detected by RNA-seq using poly-A enrichment.
Each team performed a range of combination screens and interpreted the data from their studies to explain genotype and phenotype correlations by devising a computational framework. For example, Dixit et al. (Chen et al. Citation2016) perfomed Perturb-seq to analyze 200,000 cells by targeting 24 transcription factors (TFs) in bone-marrow derived dendritic cells (BMDCs) or 14 TFs and 10 cell cycle regulators in K562 lymphoblasts. They developed a regularized linear model called MIMOSCA (available online: https://github.com/asncd/MIMOSCA) to assess how gene expression is influenced by CRISPR perturbations. The model evaluates the impact of gene knockout by coefficient matrix β based on the correlation of expression matrix Y predicting each gene’s (log) expression level and design matrix X depicting the effects of sgRNAs or with other covariates, such as distinct cell-types and states. It is worth mentioning that, in CRISP-seq, Jaitin et al. (Jaitin et al. Citation2016) developed an algorithm using the k-Nearest Neighbors (kNN) graph based on an assumption of similar genotypes being closer in phenotypic position to overcome data outliers and refine the genotype labeling UGI. In brief, UGI is corrected according to the UGI of neighboring cells, thus efficiently reducing data noise and false-positive and false-negative cells.
Methods carried out single cell sequencing based on CRISPR-induced perturbations enable us to acquire more detailed and complex readouts rather than relatively simplified phenotypes, providing a perspective on exploring gene functions, genetic interactions, global effects, cell positive or negative feedback loops and transcriptional effects influenced by cell states or the microenvironment. For example Dixit et al. (Chen et al. Citation2016) modularized genes by their responses to perturbations and grouped similar regulatory TFs, finding that some TFs modules exerted opposite effects. Dixit et al. indicated that the opposing functions may come as a result of the cellular feedback pathway, which indicates that modularizing data may allow the assessment of high order effects in cells. To overcome the limitation of traditional pooled screening, these approaches showed the possibility of performing multiplexed targeting in complex cell populations. For example, by infecting bone marrow cells with gRNA targeting both response (mCherry/Rela) and developmental (BFP/Cebpb) regulators and then sorting CD11c+ myeloid cells by fluorescent markers, Jaitin et al. (Jaitin et al. Citation2016) revealed that DC subtypes and monocytes enriched for distinct gene knockout cells.
The strategy of fluorescent markers can form a visual and intuitive screen. In the CRISP-seq, Jaitin et al. (Jaitin et al. Citation2016) cloned a sgRNA targeting Itgam gene encoding for the CD11b integrin, and a blue fluorescent marker (BFP) into the backbone. In addition to the gRNA (CD11b)-BFP-UGI-lenti, a gRNA (Cebpb)-mCherry-UGI-lenti containing the mCherry fluorescent marker was infected into bone marrow cells harboring a GFP-Cas9-knockin. The cells were sorted by their UGI through sequencing after 5 days. The result showed a high high consistency of BFP signal intensity, CD11b perturbation and UGI. Jaitin et al. noted that fluorescent reporters are an effective way to sort and analyze cells with low data bias. By comparing clustering analyses and fluorescence distributions, correlations between myeloid cells subtypes and gene expressions under different circumstances, such as with or without lipopolysaccharide (LPS) stimulation can be dissected.
RNA-seq based pooled screens have outperformed CRISPR screens in some respects. When performing pooled CRISPR screens in bulk cell populations, gene functions can be assessed based on the false assumption that the cell populations are homogenous, which may lead to deviated or false result and cover individual cell differences. Researchers have suggested that combined screens can be practical and efficientand have validated their methods using in vitro or in vivo experiments. RNA-based screens require only a small number of cells, as Jaitin et al. found that ∼50–100 cells were sufficient to accurately dissect gene phenotypes (Jaitin et al. Citation2016).
Although the era of combining single cell RNA-seq with CRISPR-Cas9 screening is only just beginning and holds great promise for functional genomics, the technology still has many possibilities and more improvements can be made. First, the methods can take scRNA-seq characteristics into consideration according to experimental objectives. For example, CRISP-seq base on MARS-seq (Adhemar Citation2014) which is more effective for profiling only a few cells, and both CROP-seq and Perturb-seq combine with a droplet approach which is more cost-efficient for the transcriptomic quantification of many cells (Ziegenhain et al. Citation2017). Similarly, perturbations other than gene-knockout should be assessed. Second, in addition to using CRISPRi for target gene repression, which was carried out by Adamson et al., using CRISPRa with scRNA-seq to perform gain-of-function screening or induce perturbations at the RNA level can be useful. Third, to increase data confidence, the identification of sgRNAs markers such as UGI or GBC should be more accurate, and more ideal software for analysis are in desperate need.
Future challenges
Minimizing off-target effects
Recent studies have shown that CRISPR screens are more efficient than other methods used in the past. However, the evaluation and improvement of off-target effects is an urgent problem that needs to be solved. Off-target effects are caused by mismatch tolerance, which is advantageous to bacteria to defend against viruses that tend to mutate frequently. In general, an ∼20 nt sgRNA sequence that is complementary to the target DNA followed by a PAM sequence will be designed to match the target gene for cleavage. However, the sgRNA may partially match the nontarget DNA sequence, leading to nonspecific gene cleavage. One case of this phenomenon is when some base mismatches exist between the sgRNA and the target gene sequence, and another case is the creation of a ‘DNA bulge’ or ‘RNA bulge’ when sgRNA and DNA are nonsymmetrically paired (Lin et al. Citation2014).
Several factors impact the off-target effects of the CRISPR-Cas9 system. According to a previous study, the target site recognition specificity depends mainly on the base pairing between the sgRNA and 10∼12 bp (seed region) near the PAM region, while mismatches located at the 5′ end of the protospacer region of the target sequence are better tolerated. Ren et al. demonstrated that the sgRNA GC content in the seed region has a positive correlation with the mutagenesis efficiency (Ren et al. Citation2014). In addition, the length of sgRNA is also closely related to the specificity. Zhang et al. documented that simply extending the length of the sgRNA failed to improve binding specificity (Ran et al. Citation2013), while Fu et al. demonstrated that an sgRNA truncated to 17 or 18 complementary nucleotides showed a target efficiency comparable to that of the full-length sgRNA but exerted fewer off-target effects (Fu et al. Citation2014). Furthermore, Pattanayak et al. used high-throughput sequencing to detect five potential off-target sites after genome cleavage in HEK239T cells, demonstrating that sgRNAs that were shorter and less active were more specific than those that were longer and more active and that a high sgRNA-Cas9 complex concentration could cleave sites near or within the PAM sequence, thus exerting off-target effects (Pattanayak et al. Citation2013). Thus, sgRNA design strategies that minimize off-target effects can be developed, including increasing the GC content in the seed region (40–60%), shortening the sgRNA properly and minimizing the number of paired bases between sgRNA and the predicted off-target sequence. In addition, the concentration of the sgRNA-Cas9 complex should also be carefully controlled.
Furthermore, strategies based on ‘double-nicking’ have been adopted to reduce off-target cleavage. This approach involves transformation of the Cas9 protein into a mutant Cas9n that can make only a single-stranded cut. When a pair of sgRNAs are designed to target different DNA loci on each strand that are physically close to each other, a DSB can be formed (Ran et al. Citation2013). In parallel with this approach, the FokI-dCas9 system is based on the concept that cutting is performed only when two monomers of FokI combined with dCas9 are physically close enough to form a dimer (Bitinaite et al. Citation1998; Guilinger et al. Citation2014).
The current approach to defining off-target effects is to detect DSBs in the whole genome. Whole genome sequencing (WGS) can be performed for single-cell clones but is limited by its low sensitivity at low off-target frequencies. Genome-wide unbiased identification of DSBs enabled by sequencing (GUIDE-seq) (Tsai et al. Citation2015) that depends on the capture of double-stranded oligodeoxynucleotides into DSBs has recently been developed for the sensitive detection of off-targets in living cells. This method is sufficiently sensitive to detect frequencies lower than 0.1% in a cell population, but the integration efficiency of the double-stranded oligodeoxynucleotides is low. For in vitro genome-wide assays, digested genome sequencing (Digenome-seq) (Kim et al. Citation2015) is an in vitro method to detect Cas9-induced DSBs and can even detect very weakly cleaved sites, while sgRNA testing costs are high, and the depth of sequencing multiple sgRNAs is limited. Many online websites are now able to provide sgRNA designs and off-target predictions. For example, CRISPOR.org (http://crispor.org) is an online tool that can rank sgRNAs by evaluating potential off-targets in the genome of interest, and the genome species continue expanding (Concordet and Haeussler Citation2018). Other web tools, such as CHOPCHOP (http://chopchop.cbu.uib.no), (Labun et al. Citation2016) provide support for custom length sgRNAs, and Cas-OFFinder (http://www.rgenome.net/cas-offinder) develops an algorithm that has no limitation of the number of mismatches (Bae et al. Citation2014).
Off-target effects in CRISPR-Cas9 screens
Off-target effects have been recognized as the dominant confounding factor for the CRISPR-Cas9 screens. sgRNA binding to the off-target sequence can generate DSB by guiding Cas9 endonuclease, which then results in the decrease of cell viability through activating DNA damage response and apoptosis. Therefore, the analysis of gene essentiality can be misguided because of the confounded phenotypic readouts in a LOF screen. In a recent study, Fortin et al. (Fortin et al. Citation2019) investigated the impact of single-mismatch tolerant sgRNAs in the genome-wide screens. He confirmed that a single-mismatch alignment in the sgRNA-DNA pairing can lead to the cell line-specific off-target toxicity, and the single-mismatches occurring in the PAM-proximal region are less tolerated compared to that in the PAM-distal region. More importantly, a cell-line dependent false-positive effect was demonstrated in a case that SOX9 was found to be an essential gene in the highly SOX10-expressing cell lines which in fact lacked the expression of SOX9. The reason was that the guides targeting SOX9 genes also have the single-mismatch alignments to SOX10, leading to the biased essentiality scores.
In addition to the false positives caused by the single-mismatch tolerance, the off-target cuts may result in the biased essentiality scores especially when corporate with on-target effects. This is because a strong correlation of the number of cuts induced by CRISPR-Cas9 and the cell proliferation exists, and this correlation is independent of the LOF of the target gene (Aguirre et al. Citation2017). The number of off-target cuts may effect a cumulative toll on the decrease of cell viability. These off-target effects highlight the importance of an optimal library design, including guides with less off-target and better on-target activity.
In fact, CRISPR-Cas9 technology has opened a path to uncovering the nature of genes; however, both the technology itself and its use in large-scale systemic gene screening can be dramatically improved. Increasingly sophisticated methods for WGS with more accurate sgRNA libraries and more versatile and specific Cas9 proteins will be developed. Resolving and minimizing off-target effects are the most important factors for improving the efficiency of genetic screens and the safety of gene therapy, and much more work needs to be conducted on the strategies described herein.
Disclosure statement
No potential conflict of interest was reported by the authors.
ORCID
Additional information
Funding
References
- Adamson B, Norman TM, Jost M, Parnas O, Regev A, Weissman JS. 2016. A multiplexed single-cell CRISPR screening platform enables systematic dissection of the unfolded protein response. Cell. 67(7):1867–1882.e21. doi: 10.1016/j.cell.2016.11.048
- Adhemar D. 2014. Massively parallel single-cell RNA-seq for marker-free decomposition of tissues into cell types. Science. 343(6172):776–779. doi: 10.1126/science.1247651
- Aguirre AJ, Meyers RM, Weir BA. 2017. Genomic copy number dictates a gene-independent cell response to CRISPR-Cas9 targeting. Cancer Discov. 6(8):914–929. doi: 10.1158/2159-8290.CD-16-0154
- Ali N, Karlsson C, Aspling M, Larsson J. 2009. Forward RNAi screens in primary human hematopoietic stem/progenitor cells. Blood. 113(16):3690–3695. doi: 10.1182/blood-2008-10-176396
- Bae S, Park J, Kim JS. 2014. Cas-OFFinder: a fast and versatile algorithm that searches for potential off-target sites of Cas9 RNA-guided endonucleases. Bioinformatics. 30(10):1473–1475. doi: 10.1093/bioinformatics/btu048
- Baggen J, Jan H, Staring J. 2016. Enterovirus D68 receptor requirements unveiled by haploid genetics. Proc Natl Acad Sci USA. 113(5):1399–1404. doi: 10.1073/pnas.1524498113
- Bikard D, Jiang W, Samai P, Hochschild A, Zhang F, Marraffini LA. 2013. Programmable repression and activation of bacterial gene expression using an engineered CRISPR-Cas system. Nucleic Acids Res. 41(15):7429–7437. doi: 10.1093/nar/gkt520
- Bitinaite J, Wah DA, Aggarwal AK, Schildkraut I. 1998. FokI dimerization is required for DNA cleavage. Proc Natl Acad Sci USA. 95(18):10570–10575. doi: 10.1073/pnas.95.18.10570
- Brenner S. 1974. The genetics of Caenorhabdztzs elegans. Genetics. 77:71–94.
- Broome JD. 1963. Evidence that the L-asparaginase activity of Guinea pig serum is responsible for its antilymphoma effects. J Exp Med. 118:99–120. doi: 10.1084/jem.118.1.99
- Chavez A, Scheiman J, Vora S. 2015. Highly efficient Cas9-mediated transcriptional programming. Nat Methods. 12(4):326–328. doi: 10.1038/nmeth.3312
- Cho SW, Kim S, Kim JM, Kim J. 2013. Targeted genome engineering in human cells with the Cas9 RNA-guided endonuclease. Nat Biotechnol. 31(3):230–232. doi: 10.1038/nbt.2507
- Concordet JP, Haeussler M. 2018. CRISPOR: intuitive guide selection for CRISPR/Cas9 genome editing experiments and screens. Nucleic Acids Res. 46(W1):W242–W245. doi: 10.1093/nar/gky354
- Cong L, Zhang F. 2015. Genome engineering using the CRISPR-Cas9 system. Methods Mol Biol. 1239:197–217. doi: 10.1007/978-1-4939-1862-1_10
- Conte D, Mello CC. 2003. RNA interference in Caenorhabditis elegans. Curr Protoc Mol Biol. 109:26.3.1–30.
- Datlinger P, Rendeiro AF, Schmidl C. 2017. Pooled CRISPR screening with single-cell transcriptome read- out. Nat Methods. 14(3):297–301. doi: 10.1038/nmeth.4177
- Deltcheva E, Chylinski K, Sharma CM. 2011. CRISPR RNA maturation by trans-encoded small RNA and host factor RNase III. Nature. 471(7340):602–607. doi: 10.1038/nature09886
- Dixit A, Parnas O, Li B. 2016. Perturb-seq: dissecting molecular circuits with scalable single cell RNA profiling of pooled genetic screens. Cell. 167(7):1853–1866.e17. doi: 10.1016/j.cell.2016.11.038
- Dominguez AA, Lim WA, Qi LS. 2016. Beyond editing: repurposing CRISPR–Cas9 for precision genome regulation and interrogation. Nat Rev Mol Cell Biol. 17(1):5–15. doi: 10.1038/nrm.2015.2
- Dunham I, Kundaje A, Aldred SF. 2012. An integrated encyclopedia of DNA elements in the human genome. Nature. 489(7414):57–74. doi: 10.1038/nature11247
- Fong PC, Boss DS, Yap TA. 2009. Inhibition of poly(ADP-ribose) polymerase in tumors from BRCA mutation carriers. N Engl J Med. 361(2):123–134. doi: 10.1056/NEJMoa0900212
- Fortin JP, Tan J, Gascoigne KE. 2019. Multiple-gene targeting and mismatch tolerance can confound analysis of genome-wide pooled CRISPR screens. Genome Biol. 20(1):21. doi: 10.1186/s13059-019-1621-7
- Fu Y, Sander JD, Reyon D, Cascio VM, Joung JK. 2014. Improving CRISPR-Cas nuclease specificity using truncated guide RNAs. Nat Biotechnol. 32(3):279–284. doi: 10.1038/nbt.2808
- Gans M, Audit C, Masson M. 1975. Isolation and characterization of sex-linked female- sterile mutants in Drosophila melanogaster. Genetics. 81(4):683–704.
- Gilbert LA, Horlbeck MA, Adamson B. 2014. Genome-scale CRISPR-mediated control of gene repression and activation. Cell. 159(3):647–661. doi: 10.1016/j.cell.2014.09.029
- Gilbert LA, Larson MH, Morsut L. 2013. CRISPR-mediated modular RNA-guided regulation of transcription in eukaryotes. Cell. 154(2):442–451. doi: 10.1016/j.cell.2013.06.044
- Groff AF, Sanchez-Gomez DB, Soruco MML. 2016. In vivo characterization of Linc-p21 reveals functional cis-regulatory DNA elements. Cell Rep. 16(8):2178–2186. doi: 10.1016/j.celrep.2016.07.050
- Guilinger JP, Thompson DB, Liu DR. 2014. Fusion of catalytically inactive Cas9 to FokI nuclease improves the specificity of genome modification. Nat Biotechnol. 32(6):577–582. doi: 10.1038/nbt.2909
- Habib N, Hsu PD, Wu X, Jiang W, Luciano A. 2013. Multiplex genome engineering using CRISPR / Cas systems. Science. 339(6121):819–823. doi: 10.1126/science.1231143
- Han K, Jeng EE, Hess GT, Bassik MC. 2017. Synergistic drug combinations for cancer identified in a CRISPR screen for pairwise genetic interactions. Nat Biotechnol. 35(5):463–474. doi: 10.1038/nbt.3834
- Hart T, Brown KR, Sircoulomb F, Moffat J. 2018. Measuring error rates in genomic perturbation screens: gold standards for human functional genomics. Mol Syst Bio. 10:733. doi: 10.15252/msb.20145216
- Hart T, Chandrashekhar M, Aregger M. 2015. High-resolution CRISPR screens reveal fitness genes and genotype-specific cancer liabilities. Cell. 163(6):1515–1526. doi: 10.1016/j.cell.2015.11.015
- Hinze L, Pfirrmann M, Karim S. 2019. Synthetic lethality of wnt pathway activation and asparaginase in drug-resistant acute leukemias. Cancer Cell. 35(4):664–676.e7. doi: 10.1016/j.ccell.2019.03.004
- Horvath P, Barrangou R. 2010. CRISPR/cas, the immune system of bacteria and archaea. Science. 327(5962):167–170. doi: 10.1126/science.1179555
- Hutchinson L. 2010. Targeted therapies: PARP inhibitor olaparib is safe and effective in patients with BRCA1 and BRCA2 mutations. Nat Rev Clin Oncol. 7(10):549. doi: 10.1038/nrclinonc.2010.143
- Jaitin DA, Weiner A, Yofe I. 2016. Dissecting immune circuits by linking crispr- pooled screens with single-cell RNA-Seq. Cell. 167(7):1883–1888.e15. doi: 10.1016/j.cell.2016.11.039
- Ji W, Lee D, Wong E. 2014. Specific gene repression by CRISPRi system transferred through bacterial conjugation. ACS Synth Biol. 3(12):929–931. doi: 10.1021/sb500036q
- Jiang W, Bikard D, Cox D, Zhang F, Marraffini LA. 2013. RNA-guided editing of bacterial genomes using CRISPR-Cas systems. Nat Biotechnol. 31(3):233–239. doi: 10.1038/nbt.2508
- Jinek M, Chylinski K, Fonfara I, Hauer M, Doudna JA, Charpentier E. 2012. Cas9-crRNA ribonucleoprotein complex mediates specific DNA cleavage for adaptive immunity in bacteria. Proc Natl Acad Sci USA. 109(39):E2579–E2586. doi: 10.1073/pnas.1208507109
- Khorashad JS, Eiring AM, Mason CC. 2015. shRNA library screening identifies nucleocytoplasmic transport as a mediator of BCR-ABL1 kinase-independent resistance. Blood. 125(11):1772–1781. doi: 10.1182/blood-2014-08-588855
- Kim D, Bae S, Park J. 2015. Digenome-seq: genome-wide profiling of CRISPR-Cas9 off-target effects in human cells. Nat Methods. 12(3):237–243. 1 p following 243. doi: 10.1038/nmeth.3284
- Kim HS, Lee K, Bae S. 2017. CRISPR / Cas9-mediated gene knockout screens and target identification via whole-genome sequencing uncover host genes required for picornavirus infection. J Biol Chem. 292(25):10664–10671. doi: 10.1074/jbc.M117.782425
- Konermann S, Brigham MD, Trevino AE. 2015. Genome-scale transcriptional activation by an engineered CRISPR-Cas9 complex. Nature. 517(7536):583–588. doi: 10.1038/nature14136
- Labun K, Montague TG, Gagnon JA, Thyme SB, Valen E. 2016. CHOPCHOP v2: a web tool for the next generation of CRISPR genome engineering. Nucleic Acids Res. 44(W1):W272–W276. doi: 10.1093/nar/gkw398
- Li W, Xu H, Xiao T. 2014. MAGeCK enables robust identification of essential genes from genome-scale CRISPR/Cas9 knockout screens. Genome Biol. 15(12):554. doi: 10.1186/s13059-014-0554-4
- Lin Y, Cradick TJ, Brown MT. 2014. CRISPR/cas9 systems have off-target activity with insertions or deletions between target DNA and guide RNA sequences. Nucleic Acids Res. 42(11):7473–7485. doi: 10.1093/nar/gku402
- Liu SJ, Horlbeck MA, Cho SW. 2017. CRISPRi-based genome-scale identification of functional long non-coding RNA loci in human cells. Science. 355:6320. doi: 10.1126/science.aah4712
- Liu R, Paxton WA, Choe S. 1996. Homozygous defect in HIV-1 coreceptor accounts for resistance of some multiply-exposed individuals to HIV-1 infection. Cell. 86(3):367–377. doi: 10.1016/S0092-8674(00)80110-5
- Marceau CD, Puschnik AS, Majzoub K. 2017. Genetic dissection of Flaviviridae host factors through genome- scale CRISPR screens. Nature. 535(7610):159–163. doi: 10.1038/nature18631
- Nüsslein-volhard C, Wieschaus E. 1980. Mutations affecting segment number and polarity in Drosophila. Nature. 287(5785):795–801. doi: 10.1038/287795a0
- Pagliarini R, Shao W, Sellers WR. 2015. Oncogene addiction: pathways of therapeutic response, resistance, and road maps toward a cure. EMBO Rep. 16(3):280–296. doi: 10.15252/embr.201439949
- Paralkar VR, Taborda CC, Huang P. 2016. Unlinking an lncRNA from its associated cis element. Mol Cell. 62(1):104–110. doi: 10.1016/j.molcel.2016.02.029
- Pattanayak V, Lin S, Guilinger JP, Ma E, Doudna JA, Liu DR. 2013. High-throughput profiling of off-target DNA cleavage reveals RNA-programmed Cas9 nuclease specificity. Nat Biotechnol. 31(9):839–843. doi: 10.1038/nbt.2673
- Ran FA, Hsu PD, Lin CY. 2013. Double nicking by RNA-guided CRISPR Cas9 for enhanced genome editing specificity. Cell. 154(6):1380–1389. doi: 10.1016/j.cell.2013.08.021
- Rédei GP. 2007. The connectivity map: a new tool for biomedical research. Nat Rev Cancer. 7(1):54–60. doi: 10.1038/nrc2044
- Ren X, Yang Z, Xu J. 2014. Manuscript A. Enhanced specificity and efficiency of the CRISPR/Cas9 system with optimized sgRNA parameters in Drosophila. Cell Rep. 9(3):1151–1162. doi: 10.1016/j.celrep.2014.09.044
- Rosenbluh J, Xu H, Harrington W. 2017. Complementary information derived from CRISPR Cas9 mediated gene deletion and suppression. Nat Commun. 8:15403. doi: 10.1038/ncomms15403
- Sanson KR, Hanna RE, Hegde M, Donovan KF, Strand C, Sullender ME, Doench JG. 2018. Optimized libraries for CRISPR-Cas9 genetic screens with multiple modalities. Nat Commun. 9(1):5416. doi: 10.1038/s41467-018-07901-8
- Shalem O, Sanjana NE, Hartenian E, Zhang F. 2014. Genome-scale CRISPR-Cas9 knockout screening in human cells science. Science. 343(6166):84–87. doi: 10.1126/science.1247005
- Shen JP, Zhao D, Sasik R. 2017. Combinatorial CRISPR-Cas9 screens for de novo mapping of genetic interactions. Nat Methods. 14(6):573–576. doi: 10.1038/nmeth.4225
- Shi J, Wang E, Milazzo JP, Wang Z, Kinney JB, Vakoc CR. 2015. Discovery of cancer drug targets by CRISPR-Cas9 screening of protein domains. Nat Biotechnol. 33(6):661–667. doi: 10.1038/nbt.3235
- Sigoillot FD, Lyman S, Huckins JF. 2012. A bioinformatics method identifies prominent off-targeted transcripts in RNAi screens. Nat Methods. 9(4):363–366. doi: 10.1038/nmeth.1898
- Simeonov DR, Gowen BG, Boontanrart M. 2017. Discovery of stimulation-responsive immune enhancers with CRISPR activation. Nature. 549(7670):111–115. doi: 10.1038/nature23875
- Smith I, Greenside PG, Natoli T. 2017a. Evaluation of RNAi and CRISPR technologies by large-scale gene expression profiling in the connectivity map. PLoS Biol. 15(11):e2003213. doi: 10.1371/journal.pbio.2003213
- Smith TS, Heger A, Sudbery I. 2017b. UMI-tools: modeling sequencing errors in Unique Molecular Identifiers to improve quantification accuracy. Genome Res. 27(3):491–499. doi: 10.1101/gr.209601.116
- Tebas P, Stein D, Tang WW. 2014. Gene editing of CCR5 in autologous CD4 T cells of persons infected with HIV. N Engl J Med. 370(10):901–910. doi: 10.1056/NEJMoa1300662
- Tsai SQ, Zheng Z, Nguyen NT. 2015. GUIDE-seq enables genome-wide profiling of off-target cleavage by CRISPR-Cas nucleases. Nat Biotechnol. 33(2):187–197. doi: 10.1038/nbt.3117
- Tzelepis K, Koike-Yusa H, De Braekeleer E. 2016. A CRISPR dropout screen identifies genetic vulnerabilities and therapeutic targets in acute myeloid leukemia. Cell Rep. 17(4):1193–1205. doi: 10.1016/j.celrep.2016.09.079
- Wang B, Wang M, Zhang W. 2019. Integrative analysis of pooled CRISPR genetic screens using MAGeCKFlute. Nat Protoc. 14(3):756–780. doi: 10.1038/s41596-018-0113-7
- Wang T, Wei JJ, Sabatini DM, Lander ES. 2014. Genetic screens in human cells using the CRISPR/Cas9 system. Science. 343(6166):80–84. doi: 10.1126/science.1246981
- Wang H, Yang H, Shivalila CS. 2013. One-step generation of mice carrying mutations in multiple genes by CRISPR/Cas-mediated genome engineering. Cell. 153(4):910–918. doi: 10.1016/j.cell.2013.04.025
- Wang T, Yu H, Hughes NW. 2017. Gene essentiality profiling reveals gene networks and synthetic lethal interactions with oncogenic ras. Cell. 168(5):890–903.e15. doi: 10.1016/j.cell.2017.01.013
- Wiedenheft B, Sternberg SH, Doudna JA. 2012. RNA-guided genetic silencing systems in bacteria and archaea. Nature. 482(7385):331–338. doi: 10.1038/nature10886
- Wong AS, Choi GC, Cui CH. 2016. Multiplexed barcoded CRISPR-Cas9 screening enabled by CombiGEM. Proc Natl Acad Sci USA. 113(9):2544–2549. doi: 10.1073/pnas.1517883113
- Xie S, Duan J, Li B, Zhou P, Hon GC. 2017. Multiplexed engineering and analysis of combinatorial enhancer activity in single cells. Mol Cell. 66(2):285–299.e5. doi: 10.1016/j.molcel.2017.03.007
- Yau EH, Kummetha IR, Lichinchi G, Rana TM. 2017. Genome-wide CRISPR screen for essential cell growth mediators in mutant KRAS colorectal cancers. Cancer Res. 77(22):6330–6339. doi: 10.1158/0008-5472.CAN-17-2043
- Yin Y, Yan P, Lu J. 2015. Opposing roles for the lncRNA haunt and its genomic locus in regulating HOXA gene activation during embryonic stem cell differentiation. Cell Stem Cell. 16(5):504–516. doi: 10.1016/j.stem.2015.03.007
- Zheng GX, Terry JM, Belgrader P. 2017. Massively parallel digital transcriptional profiling of single cells. Nat Commun. 8:1–12. doi: 10.1038/s41467-016-0009-6
- Zhu S, Li W, Liu J. 2016. Genome-scale deletion screening of human long non-coding RNAs using a paired-guide RNA CRISPR-Cas9 library. Nat Biotechnol. 34(12):1279–1286. doi: 10.1038/nbt.3715
- Ziegenhain C, Vieth B, Parekh S. 2017. Comparative analysis of single-cell RNA sequencing methods. Mol Cell. 65(4):631–643.e4. doi: 10.1016/j.molcel.2017.01.023