Abstract
Changes in the forest landscape of Mt. Makiling Forest Reserve were evaluated given the three different time periods of remotely sensed datasets using ISOCLUST method. The classification explicitly figured out that the forest areas are persistent or still intact while spatially expanding the coverage from the area. The change analysis revealed that the spatial distribution of various land-cover categories was subjected to gain and loss based on the latest and previous remotely sensed data sets. High gains for forest areas and many losses on agroforestry areas were established at certain time spans. The land cover by category denotes a decrease of forest areas between 1993 and 2002, and eventually increases in line with the latest period. The increase in agroforestry areas was merely detected between 1993 and 2002 and subsequently reduced the possible expansion of its area coverage. A great deal of land cover can be perceived to the restoration efforts made in the study area. Applying NDVI in the segmentation process during the image classification demonstrated to be a constructive approach of classifying land cover types.
Introduction
Sustainable forest management has become the salient crosscutting theme in forestry throughout the world today. This paradigm recognizes that forests are managed for a wide variety of ecological, economic, and social benefits (Cubbage and Harou Citation2006). It is a potential means of making restoration profitable. It remains the only way to reforest large areas, while maintaining economic viability for the landowner (Pejchar and Press Citation2006). As the need for rehabilitation is often caused by unsustainable forest management, the change is crucial to facilitate advanced management systems, which aim at avoiding degradation in the future (Weiss Citation2004).
In the case of Mt. Makiling Forest Reserve, Philippines, forest landscape has been changed primarily due to man-made (e.g. shifting cultivation, and reforestation) and natural (e.g. forest fires, typhoon, and soil erosion) disturbances. The study primarily believes that the entire forest reserve is greatly sensitive to change in landscape because of the past natural catastrophes and man-made interferences, even though Mt. Makiling does not allow further utilization and it is exclusively devoted for scientific purposes only (as prescribed in Republic Act 6967 in 1990).
Efforts at rehabilitating the forest landscapes in the forest reserve through reforestation have been sustained and were considered a success. The landscapes of Mt. Makiling are now a mosaic of land uses that include patches of residual forests, plantations, and agricultural lands. However, it may also include areas of degraded lands. Hence, it is imperative to assess the patterns and transitions of changes in land use through the use of remotely sensed datasets.
The study aimed to evaluate and classify the landscape change given the different time periods. It also aimed to determine the degree of changes to forest landscape at the study area.
Methods
Description of the site
The Makiling Forest Reserve (MFR) is in the southern part of Metro Manila, Luzon Island, Philippines (). The forest reserve geographically radiates to coordinates of 14°08'14” north latitude and 121°11'33” east longitude. The MFR is only about 42.44 km2 and under the jurisdiction of the University of the Philippines at Los Baños (UPLB) by virtue of the Republic Act No. 6967 in 1990. It is also classified as a national park and is a critical watershed for power generation (Vallesteros Citation2002).
Figure 1. (Color version available online) Location of the Mt. Makiling Forest Reserve, Philippines (composite image of red, green, and blue bands).
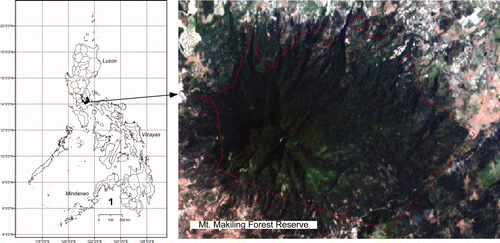
The topography of Mt. Makiling is generally rugged with elevation ranging from 40 to 1100 meters above sea level (m asl). About 70% of the total area has an elevation of more than 400 m asl. The areas at lower elevations have gentle slopes and therefore suitable for farming and settlement. The soils of Mt. Makiling belong to four series (Lipa, Macolod, Gulugod and Makiling) and are generally fertile. Clay loam is the dominant soil texture in the study site.
The favorable soil and climate makes the forest reserve a rich collection of valuable plant species. The vegetation of Mt. Makiling is a gradient from low vegetation at the base, to a typical tall forest on the lower elevations, and to a crooked, stunted mossy forest at its three peaks (Fernando et al. 2004). The forest reserve contains at least 225 families and 2,038 species of vascular plants (Pancho Citation1983) and may serve well as a representative of the Philippine mixed hardwood vegetation. The MFR is also regarded as a botanical garden, which harbors many exotic and locally introduced species of plants in the area.
Data availability
The information content of remotely sensed data is directly related to the spatial resolution of the imagery. The spatial resolution of an image is often indicated through pixel size. The 15 m × 15 m and 30 m × 30 m spatial resolution of ASTER images and Landsat Enhanced Thematic Mapper plus (ETM + ), respectively, were utilized in this study. ASTER and Landsat ETM + imageries have a relatively large coverage with a single scene covering approximately 75 km × 75 km and 225 km × 225 km, respectively. However, the region of interest was specified for the study area. provides details of datasets acquired for the study.
Table 1. List of land satellite imageries used in the study.
Image preprocessing
Atmospheric correction was not completed for the Landsat ETM + and ASTER imageries. Based on the examination of imageries, the atmospheric effects were small and images had excellent quality with no haze and only some small patches of cloud and shadow cover. Indeed, the main distinction of all images was the season of acquisition (2 April 1993, 3 April 2002, and 11 March 2008).
To locate ground features on imagery, or to compare a series of images, a geometric correction procedure was used to register each pixel to real world coordinates (Jensen Citation2000).
Imageries were already orthorectified for the study area. However, ground control points (GCPs) through the Global Positioning System were established for geometry correction since imageries have different platforms and sensors. Datasets were projected to UTM 51N coordinate system and WGS84 datum. A nearest neighbor resampling method was applied to the ASTER datasets with a pixel spacing of 30 m × 30 m to maintain the integrity of the pixel values. The orthorectified imageries were clipped to the MFR reserve boundary.
Classification
The methodology was mostly based on the approach proposed by Wulder et al. (2004) as follows: creation of a Normalized Difference Vegetation Index (NDVI) image and its segmentation into high, low and non-vegetation values; land-cover classification for each NDVI segment completed using an iterative self-organizing clustering algorithm; aggregation and labeling of classes; quality assurance of classification outputs; and production of final maps.
Segmentation of the image was based on NDVI, which is related to the vigor of vegetation. NDVI offers the advantage of reducing the impact of topographic effects visible in the image (Canadian Space Agency and Hatfield Consultant 2006). The overall aim of segmentation was to simplify the classification by focusing on areas of the image for the unsupervised clustering. NDVI imageries from the Landsat ETM + datasets were calculated using the following relationship:
In the case of ASTER imagery, the NDVI was expressed as:
The result of calculating NDVI was an index with a possible range between –1 and + 1, with values of NDVI having several significant figures. The process produced 32-bit images, as the NDVI values require a large number of decimal places. However, 32-bit images were very large in size and to reduce issues relating to file storage and manipulation, the NDVI values were simplified and scaled to 8-bit integer values (0 to 255) using the following formula:
The ISOCLUST classification was performed within the segments of the NDVI image (high, low, and non-vegetation) on the original bands of image data. Some classes such as regenerating forest (often containing a mixture of land-cover classes) were found to occur in more than one segment. Therefore, the definitive edge of the NDVI segment is not as critical as separating general groups of vegetation and non-vegetation communities using ISOCLUST. The detailed threshold NDVI values adopted in the study are shown in .
Table 2. Threshold NDVI values adopted at the study area.
ISOCLUST is an iterative self-organizing unsupervised classifier based on a concept similar to the well-known ISODATA routine of Ball and Hall (Citation1965) and cluster routines, such as the H-means and K-means procedures (Eastman Citation2006). The ISOCLUST algorithm specifies the number of iterations, the number of clusters, and the minimum sample size per class required in the training process within an iteration. The process begins with a specified number of arbitrary cluster values. It is then processed repetitively to improve the clusters. Each image was classified into 10 to 15 clusters for each NDVI segment. The final clusters were determined according to the prescribed land-cover type, which was an offshoot of direct observation or field validation for the study area.
Change in forest landscape
Land covers from three different scenes were considered for change analysis within the study site. Land-cover modeling was utilized for the change in forest landscape analysis, allowing one to generate evaluations of gain and loss, net change, persistence, and specific transitions. Three combinations with different time intervals were performed for the analysis, including 10 years (between 1993 and 2002), 16 years (between 1993 and 2008), and 7 years (between 2002 and 2008) windows. A 1993 scene was considered as a baseline period because the estimated coverage is relatively close to the frequently reported land-cover area (Bantayan Citation2001; Vallesteros Citation2002).
Major land-cover types in the forest reserve were mainly classified as forest, agroforestry, and special uses. The land-cover types category for the MFR was patterned to the description and classification made by Bantayan (Citation1996). For instance, forest areas are represented by second growth dipterocarps and plantations, including the tropical rain-forest park and botanic gardens. Agroforestry areas are those where annual crops and fruit trees along with woody species are grown. Brush lands, grasslands and coconut plantations areas are also categorized as part of the agroforestry areas. Special use areas include the campus, research institutes, built-up areas, and outdoor recreation resorts located inside the reserve.
Results and discussion
NDVI segmentation
The entire forest reserve constantly marked high NDVI values ranging from 0.52 to 0.89 in three scenes. The 1993 NDVI imagery provided a crude estimate of vegetation health with an average value of 0.55. The 2002 scene depicted NDVI maximum values of 0.52 with a mean of 0.35. In 2008, about 0.72 vegetative index was estimated with mean value of 0.51. Kidwell (Citation1997) indicated the NDVI values, such as low values for lightly vegetated areas (0.1–0.2), moderate values for shrub and grassland areas (0.2–0.3), and higher values (0.4–0.7) for dense vegetation areas. Meanwhile, the negative values are represented by those non-vegetative structures like water, clouds, and shadows.
Results implied that a large portion of the area is highly vegetative. The dense and existing forest conditions were likely concentrated at the middle of the study area. Equally, patches and fragmented low-vegetated indices were usually found along the boundary and put down in the southeast portion. Clouds and shadows were also evident in the 2008 scene which is represented by the grayish palette.
Essentially, estimated high NDVI values from the area can be considered as an indicator of relative biomass and greenness (Chen and Brutsaert Citation1998). NDVI is a representative of plant assimilation condition and of its photosynthetic apparatus capacity and biomass concentration (Groten Citation1993). In the secondary forest of the reserve, a high amount of litter production in tons ha−1 yr−1 was accounted by Lee (Citation2006), which included the litterfall components, such as leaf (8.17 ± 0), non-leaf (2.65 ± 0.25), and reproductive part (0.84 ± 0.26).
Image classification
The high-vegetation segment revealed clusters related to primary and secondary forests, regenerating and plantation. The low-vegetation segment revealed clusters related to regenerating, non-forest/agriculture, and plantation. The non-vegetation segment revealed clusters associated with bare ground, water, and human settlement. Class allocation was performed based on data collected and through direct observation in the field. The land cover maps in three scenes are shown in .
Figure 3. (Color version available online) Land cover classification based on (a) 1993, (b) 2002, and (c) 2008 scenes at the study area.
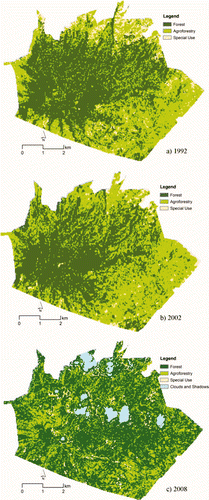
Based on 1993 land-cover classification, about 2357 ha (54%) were classified as forest areas, 1747 ha (40%) were estimated as agroforestry areas, and 242 ha (6%) were designated for special-use purposes as seen in . In particular, forest areas were really concentrated on high elevations. Patches of forest areas were also found in the southeast part of the forest reserve. Agroforestry areas were mostly evolving in the southeast portion while often scattered on the other part with lower elevation. In the case of the 2002 classified land cover, a high area coverage was estimated for forest areas of about 2241 ha (51.6%), followed by agroforestry of about 1999 ha (46%), and special-use areas which remained low to approximately 106 ha (2.4%). The distribution of forest areas was described as being concentrated on high elevation but there was an indication of fragmentation on the lower elevation of the forest reserve. Surprisingly, agroforestry areas were relatively increasing in the southeast location and fragmented on the low to middle elevation.
Table 3. Summary of spatial coverage from the classified land cover at the study area.
Seven years later, the distribution of the land-cover types was seemingly different in appearance. About 2909 ha (66.9%) of the forest reserve were classified as forest areas and 25.5% (1105 ha) were designated for agroforestry areas. Remaining areas were designated for special uses (3.2%) and clouds and shadows influence (4.4%). About 191 ha were affected by clouds and shadows, of which almost 52% of coverage has the potential to be agroforestry areas located at the northern part of the forest reserve. Clouds and shadows positioned near the central part of the forest reserve were obviously forest areas. Outcomes of the classification clearly indicated that the forest areas were closely intact and extended the coverage to the previously classified agroforestry areas. These areas looked scattered, and apparently expanded in the southern and western sides of the forest reserve.
The existing land-cover classification and assessment at three different scenes were comparable to the Bantayan (Citation1996), Bantayan and Bishop (Citation1998) and Vallesteros (Citation2002) investigations. Bantayan (Citation2001) has accounted forest areas of around 2387 ha in 1992, 2126 ha in 1997, and 2305 in 1999. Agroforestry areas were estimated as approximately 1795 ha, 2055 ha, and 1874 ha for the periods of 1992, 1997, and 1999, respectively. Such figures were closely associated to the land-cover classification made for the study area at different times. This is probably due to the nearness of the scene involved in the image classification and the similarity of imageries source used even though there were different techniques for extracting remotely sensed data. It must be noted that the classification based on previous scenes purely relied on the available and selected satellite imageries. The latest imageries have different resolutions and sensor types which could be a source for the modest discrepancy of estimates.
Overall, an accuracy assessment was completed for the land-cover classification based on a standard quantitative method using field survey data taken in 2008. A total of 73 samples were used for validation and entered into a matrix to generate statistics (). The overall accuracy level was 89% while the computed overall kappa index was 0.82, which indicated good accuracy assessment. Misclassification errors were mostly observed along the transitional areas between forest and agroforestry areas, and between forest and special use areas.
Table 4. Land cover classification accuracy assessment.
Changes in forest landscape
Results of the change analysis showed that the spatial distribution of various land-cover categories were subjected to gain and loss depending on the period involved as shown in . Between 1993 and 2002, the agroforestry areas gained 933 ha which was mainly derived from the change of forest areas (773 ha) to agroforestry areas while the remaining areas were for special-use contribution. It was very evident that the loss of agroforestry areas (682 ha) was in fact almost the gains of forest areas (681 ha). The results simply implied that there was a simultaneous and remarkable change linking agroforestry and forest areas and vice versa.
Figure 4. Gains and losses in area coverage between 1993 and 2002, 1993 and 2008, and 2002 and 2008.
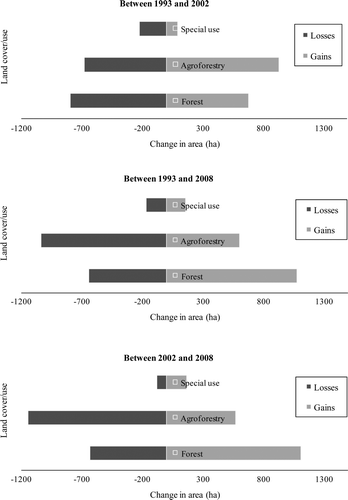
Prior to this study, Bantayan (Citation2001) made a comparative analysis of forest and agroforestry areas in the Mt. Makiling Forest Reserve which revealed that there had been a steady decrease of forest cover (from 2505 to 2126 ha) and a corresponding increase in agroforestry areas (1339–2055 ha) from 1979 until 1997. However, estimated areas in 1999 period were closely related to the 1992 classification where forest areas (2305 ha) slightly increased while agroforestry areas (1874 ha) decreased a little.
In this study, similar observation was found in which a very minimal decrease (about 2%) was estimated for forest areas while there was around a 6% increase for the agroforestry areas in the involved periods. Hence, the pattern between 1993 and 2002 land-cover change indicated a slight decrease in forest areas and increase in agroforestry areas. Conversely, a different pattern and trend was noticed considering the 16 years' time window from the change analysis. High gain between 1993 and 2008 for forest areas (1080 ha) was found while significant loss on agroforestry areas (606 ha) was established during this particular time span. The condition denotes that large agroforestry areas would likely be restored or re-grown to a secondary forest.
A possible land-use transition sequence in the Philippines was described by Verburg and Veldkamp (Citation2004). This particular transition sequence in restoring forest growth is most likely complemented in the case of Mt. Makiling Forest Reserve. Basically, the secondary forest can develop from grassland or fallow land as long as burning and grazing are restricted. The conversion of secondary forest to primary forest is possible through regrowth. In contrast, new areas of shifting cultivation are most likely to develop through the clearance of secondary or primary forest. In particular, Vallesteros (Citation2002) also revealed that the widespread incidence of re-cleaning of farm lots was an indication that forest re-growth was happening in the study area. Abandoned farms would regain forest cover naturally especially those located at the edge of the forested area. In the initial stage, brush vegetation would occur before big trees take over (Oliver and Larson Citation1996). The dynamics of change from forest to cultivated area and the reverse process is seen in the land-cover change. The situation indicates that the changes are more likely to happen along the boundary of forest and agroforestry areas.
The results of the present assessment also recognized the large patch of persistent forest areas between 1993 and 2008. Persistence of forest areas was estimated to about 1222 ha in a 16-year period. It confirmed that high area coverage remained unaffected despite any change from other land-cover types. It also describes the persistence of the agroforestry areas which turned out into decreasing area coverage. These conditions are greatly anticipated in agroforestry areas because of regular cultivation, planting, and harvesting. In addition, classifications through remotely sensed data are very sensitive to the spectral response from the canopy closure. Hence, a great amount of agroforestry areas would be transformed as a good vegetative cover.
Findings were most likely attributed to various factors, such as reforestation, protection against forest fire and shifting cultivation, and interventions made by the different stakeholders for the study area. In particular, Torres and Aparejado (1999) described the increased environmental awareness in the early 1990s that heightened stakeholders' participation in efforts to rehabilitate the degraded areas within the Mt. Makiling Reserve. It was considered more or less a decade of activity where private sector participation in forest restoration efforts became a significant mode of partnership among them. The situation was further elaborated by Abraham (Citation2007) who reported that the area was extremely burned over in April 1990 where local stakeholders protected it from fire and the private sector carried out reforestation efforts from 1993 to 1997. Eventually, additional planting, maintenance and exclusion of forest fire continued through private sector volunteer-based approach with 80% survival rate. Hence, the spatial coverage of forest areas under all these circumstances would certainly increase for the Mt. Makiling Forest Reserve.
In a much shorter span, between 2002 and 2008, the change was also notable showing higher gain of forest areas (1114 ha) than the agroforestry areas (572 ha). Similarly, higher loss of agroforestry areas (1143 ha) was estimated compared with forest areas (633 ha). The change was spatially influenced by the alteration of agroforestry areas to forest areas. Different factors were associated, as mentioned above, to the possible restoration and transition in the area. A high persistence of forest areas (1523 ha) indicates stability even though fragmented forest patterned to other parts. Apparently, about 510 ha of agroforestry areas were largely persistent in the southwest portion of the MFR.
Overall, summarizes the net change (% of area) experienced by each category. Results denote a gain of agroforestry areas (1.84%) between 1993 and 2002, and eventually considerable loss of about 8.58% in the later period. The considerable increase in forest areas was detected between periods of 1993 and 2008 (6.61%) and 2002–2008 (8.58%). In this assessment, it must be noted that MFR is under the jurisdiction of the UPLB which is subjected to restrictions and policies being implemented in the area to various stakeholders. However, the effectiveness and other indicators for the evaluation are out of the scope. Hence, a great land cover can be perceived through the restoration efforts made in the study area.
Table 5. Net change (land cover change matrix) experienced between categories.
Conclusion
Applying NDVI in the segmentation process during the image classification demonstrated a constructive approach of classifying land-cover types and eventually forest landscape change analysis at the study area. The classification clearly figured out that the forest areas are persistent or still intact while spatially expanding coverage from the area. The forest landscape revealed that the spatial distribution of three land-cover categories was subjected to gain and loss mainly due to associated man-made and natural disturbances. In particular, high gain for forest areas was an evolving pattern, while loss on agroforestry areas was changed at certain time spans. Hence, the condition persists that large agroforestry areas can likely be restored or re-grown to a secondary forest.
The competence and motivation of the stakeholders involved to protect forest resources is very important. One critical role is that of the university. The administration must be capable of capitalizing on existing policies to augur well for their forest management initiatives. The administration should also harness a participatory approach in managing forest resources. Defining the best type of incentives depending on the need and motivation of local stakeholders is an effective tool in gaining interest in various protection strategies implemented in the forest reserve.
Acknowledgments
This study was the initiative of the Center for Restoration of Forest Ecosystem Functions on Different Forest Zones (CERES) project at Seoul National University with the support of Forest Science and Technology Projects (Project No. S210608L0101704C) provided by the Korea Forest Service.
References
- Abraham , E RG . 2007 . Conservation relay: private sector participation in restoration of degraded forest landscapes in Mt. Makiling, Philippines. In: Proceedings of the IUFRO Conference on Forest Restoration . Seoul, Korea , 14–19 May 2007
- Ball , G H and Hall , D J . 1965 . A novel method of data analysis and pattern classification , Menlo Park, CA : Stanford Research Institute .
- Bantayan , N C . 1996 . Participatory decision support systems: The case of the Makiling Forest Reserve, Philippines PhD Dissertation, University of Melbourne, Australia. pp. 63–88
- Bantayan , N C . Geomatics-assisted impact assessment of land use change on the biodiversity of Mt. Makiling, Philippines . Proceedings of the 22nd Asian Conference on Remote Sensing . Singapore. 5–9 November 2001
- Bantayan , N C and Bishop , I D . 1998 . Linking objective and subjective modeling for land use decision-making . Landscape Urban Plan , 43 : 35 – 48 .
- Canadian Space Agency and Hatfield Consultant . 2006 . Earth observation support for traditional ecological mapping and biodiversity conservation in Viet Nam (EO-STEM) Project . Work Package , : 2
- Chen , D and Brutsaert , W . 1998 . Satellite-sensed distribution and spatial patterns of vegetation parameters over a tall grass prairie . J Atmosph Sci , 55 ( 7 ) : 1225 – 1238 .
- Cubbage , F P and Harou , P . 2006 . Policy instruments to enhance multi-functional forest management . For Policy Econ , 9 ( 3 ) : 261 – 273 .
- Eastman , J R . 2006 . IDRISI Andes: Guide to GIS and image processing , Worcester, MA : Clark University .
- Fernando , E S , Sun , B Y , Suh , M H , Kong , H Y and Koh , K S . 2004 . Flowering plants and ferns of Mt Makiling , Seoul : ASEAN-Korea Environmental Cooperation Unit, Seoul National University .
- Groten , S ME . 1993 . NDVI-crop monitoring and early yield assessment of Burkina Faso . Int J Remote Sens , 8 : 1495 – 1515 .
- Jensen , J R . 2000 . Remote sensing of the environment: an earth resources perspective , Upper Saddle River, NJ : Prentice-Hall .
- Kidwell , K B . 1997 . NOAA Global vegetation index user's guide , Silver Spring, MD : NOAA .
- Lee , Y K . 2006 . Ecosystem structure and functions in relation to restoration of degraded tropical forests in the La Mesa watershed and Mt. Makiling, Philippines PhD Dissertation, Seoul National University, Seoul, Korea
- Oliver , C D and Larson , B C . 1996 . Forest stand dynamics , Hoboken, NJ : John Wiley & Sons .
- Pancho , J V . 1983 . Vascular flora of Mt. Makiling and vicinity (Luzon: Philippines) Part I . Kalikasan, Philippine J Biol ,
- Pejchar , L and Press , D M . 2006 . Achieving conservation objectives through production forestry: The case of Acacia koa on Hawaii Island . Environ Sci Policy , 9 ( 5 ) : 439 – 447 .
- Republic Act No. 6967 . 1990 . An act to vest control, jurisdiction and administration of the forest reserve in Mount Makiling in the University of the Philippines in Los Baños , Metro Manila : Congress of the Philippines .
- Torres , C S and Aparejado , L A . 1999 . “ Partnership modes in reforestation and rehabilitation of the Mt. Makiling Forest Reserve ” . In The Mt. Makiling Forest Reserve: Development initiatives and management experiences , Edited by: Torres , C S and Tan , E M . 103 – 113 . Quezon City : Makiling Center for Mountain Ecosystems .
- Vallesteros , A P . 2002 . GIS-based determination of socio-economic variables affecting land-use change in Mt. Makiling Forest Reserve, Philippines . MS Thesis, UPLB, College, Lag. Phil ,
- Verburg , P H and Veldkamp , A . 2004 . Projecting land use transitions at forest fringes in the Philippines at two spatial scales . Landscape Ecol , 19 : 77 – 98 .
- Weiss , G . 2004 . The political practice of mountain forest restoration – comparing restoration concepts in four European countries . For Ecol Manage , 195 ( 1–2 ) : 1 – 13 .
- Wulder , M A , Franklin , S E , White , J C , Cranny , M M and Dechka , J A . 2004 . Inclusion of topographic variables in an unsupervised classification of satellite imagery . Can J Remote Sens , 30 ( 2 ) : 137 – 149 .