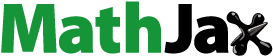
Abstract
This study was conducted to establish reasonable forest management plan by developing site index curves about Pinus densiflora stands that is major species growing in Jeolla-do in Republic of Korea. A total of 613 Pinus densiflora plots and 20m × 20m sampling plot was installed for each stand. The altitude height, slope, orientation bearing, soil type, the height and diameter at breast height (1.2 m from the ground) of a dominant tree, and the age of trees were measured. After developing site index curves using Chapman-Richards, Schumacher and Gompertz models, top three geographical factors were added to asymptote and shape parameters. In results, Gompertz model for Pinus densiflora stands was chosen for best model of height and site index model. Also, soil type, parent rock and topography were added into Gompertz model for Pinus densiflora stand as the independent variables, using the random forest analysis method. As result of adding geographical factors to asymptote and shape of Gompertz model for Pinus densiflora stand, the precision of the model has increased with decreasing MSE. As hybrid site index model including geographical factors indicated influence growth of Pinus densiflora, reasonable forest management plan is determined.
Introduction
It is difficult to manage the forest in Republic of Korea due to diverse environmental factors including steep and mountainous terrains. Therefore, it is essential to establish a reasonable forest management plan for managing a forest successfully. Moreover, the judgment of site quality is a critical decision factor for establishing a reasonable forest management plan.
Fast-growing stands need thinning mainly at the beginning of management. On the other hand, tending and fertilization are implemented at the beginning of management for slow-growing stands. Therefore, site quality is an essential element to establish the overall forest management plan and select a silvicultural system (Son et al. Citation2013). Site index is a direct evaluation method of site quality.
Site index is defined as the height of the dominant tree or co-dominant tree at the base age (Clutter et al. Citation1983; Philip Citation1994; van Laar & Akça Citation1997; Avery and Bukhart Citation2002; Husch et al. Citation2002). The most important application of site index is to evaluate the production capacity of a stand by examining the possibility of future height growth (Bailey and Clutter Citation1974; Biging Citation1985; Dyer and Bailey Citation1987; Cieszewski Citation2002).
A guide curve method and an algebraic difference equation are commonly used to estimate a site index (Son et al. Citation1997). A guide curve method is a way to estimate a site index by using the height of a dominant tree at one point in a real stand when a permanent sample point and a stem analysis are not available. An algebraic difference equation is a way to estimate a site index from measurements at fixed interval (generally 5–10 years) of a permanent sample point or at least two measurements of a stem analysis (Lee et al. Citation2001).
The growth of trees is highly affected by environmental and geographical factors. Therefore, various studies have been conducted to improve the fitness of the model by applying various environmental and geographical factors to the model. Previous studies selected geographical factors suitable for developing site index prediction model by using correlation analysis between a site index and the geographical factors.
The recent development of information power has increased the number of environmental factors and meteorological factors associated with the site index. Therefore, factors affecting the prediction of site index are increasing. It is very important to select the most influential and predictive variable among these numerous independent variables for estimating the site index. Consequently, the random forest analysis method (RFAM), which is one of the ways to determine the priority of independent variables affecting a dependent variable, has been highlighted in many fields. It is one of data mining and machine learning techniques and it reduces the prediction error by maximizing the randomness based on decision tree. Particularly, it has been shown that RFAM has high predictive power for multidimensional data, which have a large number of explanatory variables. RFAM has, recently, been used widely in the forest ecology field because it can avoid the overfitting problem of the single decision analysis using multiple decision trees (Breiman Citation2001).
This study selected geographical factors suitable for developing a site index predictive equation by applying RFAM, which is one of data mining techniques, to Pinus densiflora stands, which is major species in Jeolla-do region in Republic of Korea. Moreover, the selected geographical factors, which were selected through RFAM, were used to develop the site index model. It was judged that it would be possible to conduct an analysis that could cope with diverse conditions through this geographical factors selection and develop a more accurate site index model. This study was conducted to establish a reasonable forest management plan based on findings.
Materials and methods
Materials
This study utilized the 2016 survey data of Pinus densiflora stands growing in Jeollado in Republic of Korea. The study selected 613 Pinus densiflora plots and one 20m × 20m sampling plot was installed for each stand. Altitude height, slope, orientation bearing, soil type, the height and diameter at breast height (1.2 m from the ground) of a dominant tree, and age of trees were measured (). Ages of the low data ranged from 10–60 and 61 for the Pinus densiflora stands. Accordingly, this age limits the site index model for those species stands were developed age ranges from 10–60 years and the average age is 39.8 years. Also, height of the low data, ranged from 3.9–21.6 m, represents the average height that is 13.2 m. The extent of DBH low data is 6.6–47.4cm and it appears 25.0 cm average value.
Table 1. Status of Species stands.
Methods
Selection of priority independent variables
RFAM was used to determine the priority of independent variables affecting a site index and RFAM was conducted using R-Studio. An analysis should be carried out by dividing a variable group using a data set. The data set for RFAM is composed of two species and one variable group (11 geographical factors). The independent variables used in the analysis are shown in .
Table 2. Independent variables used in analysis.
It can be hard to interpret the results of RFAM since it cannot derive an intuitive graph, like the decision tree. Therefore, other indices such as variable of importance index and partial dependence plots are provided to understand the importance (influence) of an explanatory variable to a response variable in number or graphs (Yoo Citation2015). This study also used these indices. This study extracted the importance of independent variables affecting the dependent variables (site index) in Pinus densiflora stands using RFAM. It was to select geographical factors used as independent variables and develop a hybrid type site index curve with a high degree of fitness. X1 (altitude height), X2 (slope), and X3 (orientation bearing), among geographical factors, have a great influence on the extraction of variable importance and they are already known as important variables. They were excluded from the analysis and sued as default variables. The importance of variables from X4 to X11 was analyzed.
Dependent variable
This study used Chapman-Richards, Schumacher, Gompertz models based on the assumption that the stem density does not influence the height growth (). Moreover, 30-year was used as a base age, which is an important factor for estimating a site index. Forest stands in Republic of Korea were mostly immature so 20-year was used as a base age. However, currently IV age class is the most dominant class in Republic of Korea (Korea forest service Citation2016). Therefore, this study estimated a site index using 30-year, which is the currently used base age in Republic of Korea.
Table 3. General forms of projection models applied to data.
Statistical method of analysis
Mean square errors
The Mean square errors (MSE) is the sum of squared errors (MSE) divided by the degree of freedom. It is calculated by considering the estimation offset and the degree of the model.
Patterns of residuals
Residuals should be distributed randomly on the X-axis when the developed regression model is valid. Therefore, this study examined if residuals’ distribution meat the homoscedasticity assumption.
Results and discussion
Selection of priority independent variables
Analysis result
The importance of each geographical factors affecting the site index (the mean height of dominant trees at the age of 30 years) was analyzed. The results showed that soil type (26.1801) had the highest influence, followed by parent rock (11.0121) and topography (10.0496) ().
Conclusion of regression analysis to analyze the relatively important geographical factors included in the site index prediction models for Pinus densiflora stand living in the central temperate zone. It showed that factors are parent rocks, soil drainage, soil type, B soil depth and dry/wet condition of A soil, which were similar with the results of this study (Shin et al. Citation2007).
Developing site index
The analysis results showed that the MSE of Chapman-Richards, that of Schumacher and that of Gompertz were 7.1576, 7.1565 and 7.1624, respectively. The MSE of Schumacher model was the smallest. However, it was analyzed that Chapman-Richards and Schumacher models were not statistically reasonable because the 95% confidence limits interval of parameter estimates included “0” range. Therefore, this study developed the site index model by using Gompertz model, which had the MSE value of 7.1624 and the 95% confidence limits interval did not include “0” (). The developed site index curve agreed with the height of the dominant tree in the corresponding the site index. Moreover, it has the same shape with different magnitudes for the site index ranging between 6 and 18 ().
Table 4. Non-linear squares summary statistics for 3 models fitted to site index of Pinus densiflora stand.
Developing site index including geographical factors
This study applied the top three geographical factors identified from RFAM to the developed site index model for developing a site index model considering geographical factors. The model was divided into the asymptote part and the shape part and the coefficient of each part was controlled. Adding order 1, adding order 1 and 2, adding order 1, 2 and 3 models were divided per asymptote and shape parts and 6 additional models were developed for each species.
Adjusting asymptote of Gompertz model
Statistical analyses were conducted by applying geographical factors to the asymptote of the developed Gompertz model. The results showed that the MSE of the basic model was 7.1624, while that with the adding order 1 model, that with the adding order 1 and 2 model, and that with the adding order 1, 2 and 3 model were 6.1633, 6.0373 and 5.9319 (). The results indicated that more additional geographical factors decreased MSE value, indicating an improved precision. Moreover, the decrease rate of the error of sum squares compared to the basic equation was 14.0921%, 15.9850% and 17.5871% for the adding order 1 model, that with the adding order 1 and 2 model, and that with the adding order 1, 2 and 3 model, respectively. Additionally, the decrease rate of mean square error was 13.9492%, 15.7084% and 17.1800% for the adding order 1 model, that with the adding order 1 and 2 model, and that with the adding order 1, 2 and 3 model, respectively. The analysis results revealed that it was a good model with high precision because the 95% confidence limits interval of the parameter estimates did not include “0” ().
Table 5. Adjusting asymptote of dominant height model for Pinus densiflora stand considering geographical factors.
Table 6. Reduction summary statisics for adjusting asymptote of dominant height model for Pinus densiflora stand considering geographical factors.
Consequently, the results indicated that the asymptote of the site index changed according to the soil type and parent rock. Moreover, tree height growth of a dominant tree varied by the topography (flat land, gentle hill, mountain land). Therefore, the asymptote of the height growth of a dominant tree changed in the model with soil type, parent rock and topography and it was determined that geographical factors affected the precise site index developing.
Adjusting shape of Gompertz model
Statistical analyses were conducted by applying geographical factors to the shape of the developed Gompertz model. The results showed that MSE 7.1624, 6.2497, 6.1376 and 6.0523 for the basic model the adding order 1 model, that with the adding order 1 and 2 model, and that with the adding order 1, 2 and 3 model, respectively, indicating that MSE decreased with more geographical factors. Moreover, the decreased rate of the error of sum squares compared to the basic model was 12.8882%, 14.5888% and 15.9163% for the adding order 1 model, that with the adding order 1 and 2 model, and that with the adding order 1, 2 and 3 model, respectively. Additionally, the decrease rate of mean square error was 12.7429%, 14.3081% and 15.4990% for the adding order 1 model, that with the adding order 1 and 2 model, and that with the adding order 1, 2 and 3 model, respectively. The analysis results revealed that it was a good model with high precision because the 95% confidence limits interval of the parameter estimates did not include “0”().
Table 7. Reduction summary statistics for adjusting shape of dominant height model for Pinus densiflora stand considering geographical factors.
Consequently, the results indicated that the shape of the site index changed according to the soil type, parent rock and topography, which were high priority variables, change the shape of the model and they were geographical factors influencing the growth pattern of a dominant tree. Therefore, it was determined that the site index model reflecting the soil type, parent rock and topography would improve the precision of the site index model.
Conclusions
This study prioritized the independent variables affecting the site index using RFAM scientifically and logically. The results of the analysis showed that these variables were in the order of soil type, parent rock and topography for Pinus densiflora stands. Conclusion of regression analysis to analyze the relatively important geographical factors included in the site index prediction models for Pinus densiflora stand living in the central temperate zone showed that factors are parent rocks, soil drainage, soil type, B soil depth and dry/wet condition of A soil (Son et al. Citation2013), which were similar with the results of this study and it implied that the growth of Pinus densiflora in the central temperate zone was highly affected by soil type, parent rock and topography.
Based on these results, this study developed a site index model for Pinus densiflora stand growing in Jeolla-do and deduced that Gompertz model provide the most suitable model. Moreover, it was deduced that adding more geographical factors identified by RFAM to the asymptote and shape of the model decreased the MSE value and improved the precision of the model. As a result, it was determined that soil type, parent rock and topography, which had high priority, changed the asymptote and slope of height growth and they were variables effective for estimating the site index more precisely. The result of this study implied that the site index model with additional geographical factors could improve the precision of it for Pinus densiflora stands.
This study analyzed the effects of geographical factors on the growth of Pinus densiflora growing in Jeolla-do in Republic of Korea. It is determined that reasonable forest management plan can be established using the result of this study. Additionally, it will be necessary to improve the precision of the site index model by prioritizing the meteorological factors affecting the site index as well as geographical factors.
Disclosure statement
No potential conflict of interest was reported by the authors.
Additional information
Funding
References
- Avery TE, Bukhart HE. 2002. Forest measurements. 5th edition. Boston (MA): McGraw-Hill Inc.
- Bailey RL, Clutter JL. 1974. Base-age invariant polymorphic site curves. Forest Sci. 20(2):248–259.
- Biging GS. 1985. Improved estimates of site index curves using a varying-parameter model. Forest Sci. 31(1):248–259.
- Breiman L. 2001. Random forests. Machine Learn. 45(1):5–32.
- Cieszewski CJ. 2002. Comparing fixed- and variable-base-age site equations having single versus multiple asymptotes. Forest Sci. 48(1):303–315.
- Clutter JL, Fortson JC, Pienaar LV, Brister GH, Bailey RL. 1983. Timber management: a quantitative approach. New York: John Wiley & Sons.
- Dyer ME, Bailey RL. 1987. A test of six methods for estimating true height from stem analysis data. Forest Sci. 33(1):3–13.
- Husch B, Beers TW, Kershaw JA. 2002. Forest mensuration. 4th edition. New Jersey: John Wiley & Sons.
- Korea Forest Service. 2016. 2016 Statistical yearbook of forestry. Daejeon (Republic of Korea): Korea Forest Service.
- Lee SH, Seo BS, Park UJ. 2001. Developing growth model of diameter and height growth for Quercus variabilis in Beonsan Peninsula. Korean Forest Econ Soc. 9(2):63–68.
- Philip MS. 1994. Measuring trees and forests. 2nd edn. Wallingford (USA): CAB International.
- Shin MY, Won HK, Lee SW, Lee YY. 2007. Site index equations and estimation of productive areas for major pine species by climatic zones using environmental factors. Korean J Agric Forest Meteorol. 9(3):179–187.
- Son YM, Lee KH, Chung YG. 1997. Articles: stand growth estimation using nonlinear growth equations. Korean Soc Forest Sci. 86(2):135–145.
- Son YM, Lee KS, Yoo BO, Pyo JK. 2013. Estimation of site index for Pinus thunbergii in southern region of Korea. Korean J Agric Forest Meteorol. 47(6):119–126.
- van Laar A, Akça A. 1997. Forest mensuration. 1st edn. Go¨ttingen (Germany): Cuvillier Verlag.
- Yoo JE. 2015. Random forests, an alternative data mining technique to decision tree. J Educ Eval. 28(2):427–448.