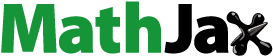
Abstract
Long-term assessment using historical satellite imagery is critical for gaining meta-information and identifying trends in land cover change. Informed policymaking about this assessment ensures forest sustainability and mitigates climate change to consider the identified trends in land cover changes and historical disturbances. Therefore, this study aimed to describe the trend of land cover change and historical disturbances in Mutis Timau Nature Reserve (CA Mutis Timau), Indonesia using the LandTrendr platform. The land cover classification is based on SNI 7645-1:2014, with a 1:50,000 scale. The data was processed using Google Earth Engine’s LandTrendr platform, which covered the vegetation index, specifically the normalized burn ratio (NBR). The results showed that the trend of land cover change in CA Mutis Timau remained stable from 1986 to 2023. These disturbance locations have low slopes and are located near neighborhoods. In specifics, the Eucalyptus forest land cover experienced the largest land cover change between 1986 and 2023, losing 945.68 ha of vegetation, or 8% of the total area of CA Mutis Timau. Over a duration of 38 years, from 1986 to 2023, the Eucalyptus forest had a cover change that turned into marble stone, barren land, grassland, and shrubs. Forest management policies, such as establishing a nature reserve area on Mount Mutis areas, had an impact on the long-term sustainability of vegetation land cover.
Introduction
Forest land cover is crucial in reducing carbon dioxide (CO2) emissions through storage (Marimpan et al. Citation2022; Zheng and Zheng Citation2023) and the increase contributes to the essential function of reducing the present climate change. Furthermore, forest land cover constitutes 54.1% of total carbon stock, more than other land cover (Baccini et al. Citation2012). Disturbances such as degradation, have an impact on the loss of forest land cover (Shimizu et al. Citation2023). Meanwhile, degradation changes the structure, vegetation composition, and productivity of forests, which leads to reduced carbon stores, biodiversity, and environmental service functions (Adinugroho et al. Citation2019; Vásquez-Grandón et al. Citation2018). This disturbance, which tends to be uncontrollable and complex, can also lead to the reduction or loss of biological and economic productivity of land (Kwakwa et al. Citation2022; Talakua and Osok Citation2019; Peprah Citation2015).
Land degradation can be prevented through assessment initiatives used as data for forest policies of management. However, policymakers have to consider factors that affect changes in the extent and condition of forest cover, such as land use change and statistical-based models using spatial variables (Pujiono et al. Citation2023). Before 1970, the decrease in forest area was comparatively reduced, but between 1970 and 1990, timber sector operations developed and became the primary driver of deforestation. Illegal and unsustainable harvesting practices from the 1990s to 2015 increased the rate of deforestation. Forestry policies implemented, such as tree planting promoted since 2011, can minimize the rate (Tsujino et al. Citation2016). The trend has decreased drastically, with the average area of forest degradation between 1990 and 2012 being 0.51 million ha per year (Adinugroho et al. Citation2019).
The areas have a high conservation value due to ability to provide habitat and ecosystem services (Graham et al. Citation2021). Mutis Timau Nature Reserve (CA Mutis Timau) is a forest group in Mutis Timau (BKSDA Citation2018a), which is a conservation area on Timor Island to protect the endemic species Eucalyptus urophylla. CA Mutis Timau has tremendous natural resources and advances the environment (Nau and Buku Citation2020). In addition, the high biodiversity of flora and fauna has the potential to improve the ecology, economy, and life of the community (Dako et al. Citation2018). CA Mutis Timau, a dry land area, is important to the long-term trend of CO2 sink (Wang et al. Citation2022), specifically 627.65 MgCha-1 (Marimpan et al. Citation2022; Sadono et al. Citation2023). The crucial role in carbon absorption is one of the mitigation initiatives for climate change. Therefore, biodiversity needs to be protected, particularly the existence of flora. The CA Mutis Timau area is disturbed by anthropogenic activities that serve the community’s interests. The Ministry of Environment and Forestry intended to transform the CA Mutis Timau area into other conservation areas in 2018 (BKSDA Citation2018b). Previous studies also showed that CA Mutis Timau degraded at a yearly rate of roughly 1% or 3.649 ha between 1987 and 2017 (Pujiono et al. Citation2019a). According to Pujiono et al. (Citation2023), degradation situations are induced by anthropogenic activities of the society as well as cultural and institutional factors.
Long-term assessment can be carried out to monitor forest management and offer temporal information on environmental changes and complex ecological systems (Negi et al. Citation2019, p. 73; Zhang et al. Citation2016). In this context, the data is important for detecting environmental changes and ecosystem responses to disturbances caused by climate change and human activity (Owari et al. Citation2020; Krebs et al. Citation2023). Remote sensing possesses the capability in forest assessment and Landsat satellite imagery proves to be the most effective tool for long-term vegetation assessment (Matas-Granadis et al. Citation2022). A long-term monitoring system (LTMS) uses long-term assessment data to predict trends in land cover change. LTMS uses satellites to provide meta-information in the form of a graphical user interface (GUI) (Mediterranean Biodiversity Protection Community Citation2019). LandTrendr is a machine learning system that uses Landsat satellite data to offer information on long-term trends of change to provide meta-information in LTMS. The LandTrendr temporal segmentation algorithm allows users to extract important features of land use/land cover transitions and forest disturbances from annual Landsat time series data (Shimizu et al. Citation2023). LandTrendr is most commonly used for detecting forest disturbances because to its efficient operation and adaptability for parallel computation on the Google Earth Engine (GEE) cloud platform (Qiu et al. Citation2023). LandTrendr has been used in several previous research to reconstruct and map forest disturbance histories with annual levels (Griffiths et al. 2012; Meigs et al. Citation2011; Fragal et al. Citation2016). Therefore, this study aimed to describe the trend of land cover change as a long-term assessment in CA Mutis Timau. The assessment results can be used to inform policymaking on climate change mitigation and the conservation of CA Mutis Timau’s biodiversity.
Materials and methods
Study area
This study was carried out at CA Mutis Timau, which had an area of 12,315.61 ha and was located in East Nusa Tenggara (NTT) Province. CA Mutis Timau is administratively divided into two regions, namely South and North Central Timor Regency (). This nature reserve is located between 124°10′ − 124°20′ E and 9°30′ − 9°40′ S. The Mutis Timau Forest features hilly to mountainous terrain, with Mount Mutis reaching an elevation of 2,427 meters above sea level. The slope conditions range from ramps to steep slopes, wavy to mountainous, and the majority of places have slopes of 60% or higher. The CA Mutis Timau area has wetter land than other locations on Timor Island, with an average annual rainfall of 2000 − 3000 mm. Wet months last from November to March, with temperatures ranging from 14 °C to 29 °C in normal conditions and reaching 9 °C in extreme conditions. The Mediterranean soil dominates CA Mutis Timau, which has a folded mountain structure. The CA Mutis Timau area is a homogeneous highland forest dominated by Eucalyptus urophylla (BKSDA Citation2018a).
Data sources
The temporal condition of the land cover was investigated using a series of Landsat satellite imagery data from 1986 to 2023 between June and September through Google Earth Engine (GEE) platform. GEE platform is also used for pre-processing and LandTrendr to save time and money. The total number of Landsat images used was 38, collected from Landsat 5 TM, Landsat 7 ETM+, Landsat 8 OLI/TRIS, and Landsat 9 OLI/TRIS (). This research adopts sensor-to-sensor harmonization following Umarhadi et al. Citation2023. The technique minimizes spectral differences (Umarhadi et al. Citation2023), re-projection, re-scaling, and re-alignment of satellite images (Nguyen et al. Citation2020).
Table 1. Band characteristics for Landsat 5 TM, landsat 7 ETM+, landsat 8 OLI/TRIS, and landsat 9 OLI/TRIS.
Calculation of the normalized burn ratio
The Normalized Burn Ratio (NBR) was used as a vegetation index to detect disturbances in the CA Mutis Timau. NBR vegetation index is more sensitive to disturbance events compared to Normalized Difference Vegetation Index (NDVI) (Liu et al. Citation2017). The NBR index consists of the near-infrared (NIR) and short-wave infrared 2 (SWIR2) bands, which are more sensitive to forest disturbance (Umarhadi et al. Citation2023). NIR and SWIR2 are sensitive to the chlorophyll composition of vegetation and water vapor, respectively. A high NBR value implies that the vegetation is healthy, as evidenced by high and low NIR and SWIR2 values. Meanwhile, a low NBR value shows soil characteristics that are apparent in disturbed pixels, as confirmed by a high SWIR2 value (Xiao et al. Citation2022). The formula for calculating NBR is used by Hislop et al. (Citation2019) (Equation 1).
Eq.1
Eq.1
LandTrendr processing
Kennedy et al. (Citation2010) developed the LandTrendr algorithm, which is a temporal segmentation algorithm for the assessment of forest disturbance and recovery. The vegetation disturbance information can be obtained in the form of Landsat satellite images with a time scale of every year (Xiao et al. Citation2022). The disturbance is known from the spectral trajectories of each pixel using an annual composite. A regression-based technique and point-to-point fitting were used to generate spectral trajectories, which were represented by a series of straight-line segments (Kennedy et al. Citation2010). The P-value of the F-statistic was used to calculate the optimal model fit. This method can give a temporal representation for recognizing progressive transitions and suppress interference from clouds, shadows, snow, and haze (Abera et al. Citation2023). Several parameters were set for data processing with LandTrendr, as shown in . The greatest change scenario is used in loss and gain analysis to generate final results that represent the highest disturbance during the observation period (Umarhadi et al. Citation2023).
Table 2. Defined segmentation parameters.
Validation
The LandTrendr results were validated using the Accuracy Assessment of Thematic Maps (AcATaMa) plugin from QGIS software. Samples were selected using a stratified random sampling method based on the proportion of the area of change. Image observations from 1986 to 2023 were carried out to validate whether the sample points had changes in forest land cover at the correct point in time, according to the LandTrendr data. The overall accuracy estimate for vegetation loss and gain showed findings of 74.29% and 81.43%, respectively ( and ).
Results and discussion
LandTrendr’s data processing can detect disturbance occurrences by providing information on the year of change, magnitude, and duration. From 1986 to 2023, the vegetation land cover of CA Mutis Timau fluctuates and encounters vegetation loss and gain. Southeast and northwest edges suffered the most significant loss, while north and northwest edges projecting toward the center had the most significant gain ().
According to the NBR value, the land cover of CA Mutis Timau changed in three distinct phases between 1986 and 2023 (). Between 1986 and 2006, the trend of land cover change in CA Mutis Timau showed a removal trend, indicating that degradation was still taking place in the area. The trend of land cover change was stagnant between 2006 and 2014, indicating that land degradation had been offset by forest vegetation regeneration. Furthermore, the 2014-2023 timeframe indicates a growth trend, indicating that forest vegetation is expanding rapidly and deforestation has decreased. This trend also shows that there is a continuing disturbance in the CA Mutis Timau, causing a decrease in vegetation land cover. The spectral index depicts the trend of land cover change since the reduction in value shows that the area is experiencing vegetation loss. Conversely, increasing spectral index values imply recovery in the form of vegetation gain in the CA Mutis Timau area. This is in line with Alcaras et al. (Citation2022), who claimed that high NBR values often indicate healthy vegetation, whereas low values suggest bare land or recently burned area.
Based on long-term assessment data processing from 1986 to 2023, vegetation loss and gain are relatively stable, with an area of less than 1000 ha (). In 1987, the CA Mutis Timau area observed a total gain of 3979.37 ha and a loss of 1115.06 ha. The high gain in forest vegetation of 32.35% in 1987 is a favorable result of 1970 rehabilitation works in the forest area, which was previously damaged (Dako et al. Citation2019). However, there was a 9.06% loss of forest vegetation in 1987. Pujiono et al. (Citation2019b) found that the likelihood of deforestation between 1987 and 1999 was significantly correlated with distance to settlement, distance to savanna, elevation, population density, and management unit variables. The Minister of Forestry Decree Number 89/Kpts-II/1983, which became the forerunner of the Mutis Nature Reserve, also had an impact on the significant vegetation gain occurrence (BKSDA Citation2018a). Managers were motivated to increase vegetation cover and after 1987, land cover changes were relatively reduced. This is because human activity has decreased its designation as a natural reserve (Marimpan et al. Citation2022). Furthermore, CA Mutis Timau is a mountainous area with difficult-to-access topography, resulting in more protected forest land and less conversion (Margono Citation2014). The management is critical to preserving and growing forest vegetation cover (Pujiono et al. Citation2019a).
Table 3. Area of vegetation loss and gain in the CA Mutis Timau from 1986 to 2023.
Disturbance detection every year from 1986 to 2023
Disturbances occurred in the north, southeast, south, west, and center regions of the CA Mutis Timau area from 1986 to 2023, as shown in . The disturbed CA Mutis Timau area has a low slope. According to Pujiono et al. (Citation2023), local people prefer to take forest products on low slopes. Disturbances in the area are caused by anthropogenic activities such as forest encroachment, illegal grazing, illegal logging, and shifting agriculture (Kurniadi et al. Citation2017; Dako et al. Citation2019; Mooy Citation2021). Anthropogenic activities are one of the factors affecting land cover changes (Bai et al. Citation2022) and can reduce species diversity (Hussein et al. Citation2021; Mooy Citation2021).
Pujiono et al. (Citation2023) found that the Timorese people’s slash-and-burn tradition for clearing agricultural land has an impact on land cover changes. Slash-and-burn may increase forest degradation and deforestation, leading to a loss of land productivity and biodiversity (Tang and Yap Citation2020; Kukla et al. Citation2019; Styger et al. Citation2007). Meanwhile, forest fires are triggered by environmental conditions in CA Mutis Timau, which is classed as a dryland forest type. Dryland environments with minimal rainfall and high temperatures can cause forest fires (Rumpel Citation2022). Fires affect the ecosystem, resulting in decreased or lost vegetation land cover. Meanwhile, vegetation is the key component in dryland ecosystems in water storage, which can influence the risk of forest fires (Du et al. Citation2017; Tian et al. Citation2019).
In 2020, the CA Mutis Timau area had a significant land cover change. We compared this to fire hotspots acquired from https://hotspot.brin.go.id/, which indicate a high level of fire. This observation is further supported by the findings of Pujiono et al. (Citation2023), who reported that the Timorese people’s slash-and-burn method of clearing agricultural land has an impact on land cover changes. Furthermore, according to the findings of Duan (Citation2022), the number of tourists visiting CA Mutis Timau increased in 2020. One of the main reasons for the high number of tourists is the main road access that connects Fatumnasi, Nenas, Mutis, and Nuapin Villages through the midst of the CA Mutis Timau area, allowing anyone to enter the CA Mutis Timau without permission from management. This visitor activity disrupts forest land cover (Boori et al. Citation2015).
Land cover change
The NBR results were then categorized into five land cover class: marble stone, barren land, grassland, shrubs, and Eucalyptus forest. The land cover class classification is based on the classification used by Sadono et al. (Citation2023) in the CA Mutis Timau area. The Eucalyptus forest land cover class experienced the largest land cover change from 1986 to 1991, with a vegetation loss of 913.01 hectares, or 7% (). The loss of vegetation in the Eucalyptus forest land cover caused changes in land cover to marble stone, barren land, grassland, and shrubs. This is inversely proportionate to the 1991–1996 period, when Eucalyptus forest land cover gained 938.91 ha, or 8% (). Vegetation gain in Eucalyptus forest land cover is caused by land cover transitions from marble stone, grassland, and shrubs to Eucalyptus forest land cover.
Table 4. Land cover change analysis table for various time periods: (a) 1986–1991, (b) 1991–1996, (c) 1996–2001, (d) 2001–2006, (e) 2006–2011, (f) 2011–2016, (g) 2016–2021, (h) 2021–2023, (i) 1986–2023.
Furthermore, the Eucalyptus forest land cover class experienced the largest vegetation loss between 1996 and 2001, with an 847.40 ha decrease, or 7% (). The loss of vegetation was caused by changes in land cover from Eucalyptus forest to marble stone, barren land, grassland, and shrubs. Between 2001 and 2006, the area covered by marble stone, barren land, grassland, and shrubs decreased. At the same time, Eucalyptus forest land cover experienced the largest vegetation gain, amounting to 1880.17 hectares, or almost 15% of the total area of CA Mutis Timau (). The research findings from 2006–2011 are inversely proportionate to the previous period. The land cover of marble stone, barren land, grassland, and shrubs increased between 2006 and 2011. In 2011, vegetation loss in Eucalyptus forest land cover reached 392.50 ha, or around 3% ().
Land cover changes in each land cover class were relatively low between 2011 and 2016, in comparison to previous periods. The land cover of Eucalyptus forest gained 394.95 hectares of vegetation, accounting for 3% of the total area (). The conversion of shrubs to Eucalyptus forest resulted in the largest vegetation gain. Between 2016 and 2021, the area of land covered by marble stone, barren land, grassland, and shrubs decreased. While Eucalyptus forest land cover gained 553.22 hectares, or 4% of its entire area (). Furthermore, the land cover change of the Eucalyptus forest in the 2021–2023 period showed the largest loss of vegetation compared to previous periods, with 2560.99 hectares lost, or almost 21% (). Overall, the analysis of land cover change from 1986 to 2023 revealed that Eucalyptus forest land cover experienced relatively low vegetation loss, amounting to 945.68 hectares, or approximately 8% of the total area of CA Mutis Timau (). Vegetation loss in Eucalyptus forest land cover occurred between 1986 and 2023 as a result of changes to marble stone, barren land, grassland, and shrubs.
The CA Mutis Timau area had losses and gains in various locations between 1986 and 2023. According to analysis observations from 1986 to 2023, the area has seen vegetation gain every year, as shown in . This increase in vegetation gain is a consequence of Mutis Timau Forest being designated as a nature reserve area, which limits community activities. Furthermore, forest rehabilitation initiatives contribute to increased vegetation gain in CA Mutis Timau. According to Dako et al. (Citation2018), the area experienced forest rehabilitation from 1970 to 2007, with plants such as ampupu (Eucalyptus alba), cassowary (Casuarina equisetifolia), Eucalyptus (Melaleuca leucadendron), tamarind (Tamarindus indica), kabesak (Acacia leucophloea), mahogany (Swietenia macrophylla), and gmelina (Gmelina arborea). From 2011 to 2016, the regional government implemented a program known as kebun bibit rakyat (KBR). The rehabilitation and KBR initiatives improve vegetative land cover in the CA Mutis Timau area. In contrast, the area had gains and losses in specific locations between 1986 and 2023. According to the data processing results, vegetation loss in the area decreased between 1986 and 2023, showing a reduction in deforestation (). This supports the findings of Pujiono et al. (Citation2019b), where deforestation decreased from 1987 to 2017. Pujiono et al. (Citation2019b) also predicted the land cover in 2030 using business-as-usual (BAU) and reducing emissions from deforestation and forest degradation (REDD) scenarios. The results show that deforestation will decrease again in 2030 with BAU and REDD scenarios.
Conclusion
CA Mutis Timau played a key role in absorbing CO2 to mitigate climate change and ensure the sustainability of forest services. The long-term assessment provided meta-information on vegetation loss and gain. This meta-information could be used as an LTMS database to predict future land cover changes. The trend of land cover change in CA Mutis Timau was stable from 1986 to 2023. The largest area of vegetation loss and gain occurred in 1987 at 1115.06 ha and 3979.37 ha, respectively. From 1986 to 2023, the majority of vegetation loss occurred in the west and center regions of the CA Mutis Timau.
The findings indicate that locations with losing vegetation are found close to neighborhoods, increasing the likelihood of anthropogenic disturbances. The largest change between 1987 and 2023 was mostly the loss of vegetation in the Eucalyptus forest, which covered 945.68 ha, or 8% of CA Mutis Timau’s total area. The transition from Eucalyptus forest to marble stone, barren land, grassland, and shrubs was the primary cause of the loss of vegetation. Reducing the loss of vegetation in Eucalyptus forests needs continuous monitoring in areas susceptible to degradation and deforestation. Aside from that, rehabilitation activities are required in order to increase the area of Eucalyptus forest.
Authors’ contributions
All authors had significant roles and contributed significantly to the paper’s conceptualization, collecting and analyzing data, interpretation of results, and writing the paper.
Acknowledgement
The authors are grateful to the Forest Management Unit for providing permission for the research at the Mutis Timau nature reserve.
Disclosure statement
No potential conflicts of interest were reported by the author(s).
References
- Abera T, Pellikka P, Johansson T, Mwamodenyi J, Heiskanen J. 2023. Towards tree-based systems disturbance monitoring of tropical mosaic landscape using a time series ensemble learning approach. Remote Sens Environ. 299:113876. doi: 10.1016/j.rse.2023.113876.
- Adinugroho WC, Prasetyo LB, Kusmana C, Krisnawati H. 2019. Contribution of forest degradation in Indonesia’s GHG emissions: profile and opportunity to improve its estimation accuracy. IOP Conf Ser: earth Environ Sci. 399(1):012025. doi: 10.1088/1755-1315/399/1/012025.
- Alcaras E, Costantino D, Guastaferro F, Parente C, Pepe M. 2022. Normalized burn ratio plus (NBR+): a new index for Sentinel-2 imagery. Remote Sens. 14(7):1727. doi: 10.3390/rs14071727.
- Baccini AGSJ, Goetz SJ, Walker WS, Laporte NT, Sun M, Sulla-Menashe D, Hackler J, Beck PSA, Dubayah R, Friedl MA, et al. 2012. Estimated carbon dioxide emissions from tropical deforestation improved by carbon-density maps. Nature Clim Change. 2(3):182–185. doi: 10.1038/nclimate1354.
- Bai Z, Dong Z, Xue L, Cui Y, Shi W, Chen G, Chi Z, Zhang C. 2022. An analysis of the effects of anthropogenic factors on vegetation cover change in Guanzhong, China. Front Earth Sci. 10:831904. doi: 10.3389/feart.2022.831904.
- BKSDA. 2018a. Profil CA Mutis Timau. BKSDA NTT [accessed 2023 November 15]. http://bbksdantt.menlhk.go.id/kawasan-konservasi/ca/ca-mutis/profil-ca-mutis-timau. [Indonesia]
- BKSDA. 2018b. Evaluasi Kesesuaian Fungsi Kawasan Cagar Alam Mutis Timau. BKSDA NTT [accessed 2024 January 9]. http://bbksdantt.menlhk.go.id/14-latest-news/166-evaluasi-kesesuaian-fungsi-kawasan cagar-alam-mutis-timau. [Indonesia]
- Boori MS, Voženílek V, Choudhary K. 2015. Land use/cover disturbance due to tourism in Jeseníky Mountain, Czech Republic: a remote sensing and GIS based approach. Egypt J Remote Sens Space Sci. 18(1):17–26. doi: 10.1016/j.ejrs.2014.12.002.
- Dako FX, Purwanto RH, Faida LRW, Sumardi S. 2018. Firewood and carpentry wood contribution to the communities of Mutis Timau Protected Forest, Timor Island. jtfm. 24(3):166–174. doi: 10.7226/jtfm.24.3.166.
- Dako FX, Purwanto RH, Farida LRW, Sumardi S. 2019. Kerusakan Antropogenik Kawasan Hutan Lindung Mutis Timau dan Upaya Penanggulangannya di Pulau Timor Bagian Barat. Jurnal Pengelolaan Sumberdaya Alam Dan Lingkungan. 9(2):437–455. doi: 10.29244/jpsl.9.2.437-455.
- Du Z, Zhang X, Xu X, Zhang H, Wu Z, Pang J. 2017. Quantifying influences of physiographic factors on temperate dryland vegetation, Northwest China. Sci Rep. 7(1):40092. doi: 10.1038/srep40092.
- Duan YE. 2022. Aktivitas Masyarakat di dalam Kawasan Cagar Alam Mutis, Nusa Tenggara Timur [Doctoral dissertation]. Universitas Gadjah Mada.
- Fragal EH, Silva TSF, Novo E. 2016. Reconstructing historical forest cover change in the Lower Amazon floodplains using the LandTrendr algorithm. Acta Amaz. 46(1):13–24. doi: 10.1590/1809-4392201500835.
- Graham V, Geldmann J, Adams VM, Negret PJ, Sinovas P, Chang HC. 2021. Southeast Asian protected areas are effective in conserving forest cover and forest carbon stocks compared to unprotected areas. Sci Rep. 11(1):23760. doi: 10.1038/s41598-021-03188-w.
- Griffiths P, Kuemmerle T, Kennedy RE, Abrudan IV, Knorn J, Hostert P. 2012. Using annual time-series of Landsat images to assess the effects of forest restitution in post-socialist Romania. Remote Sens Environ. 118:199–214. doi: 10.1016/j.rse.2011.11.006.
- Hislop S, Jones S, Soto‐Berelov M, Skidmore A, Haywood A, Nguyen TH. 2019. High fire disturbance in forests leads to longer recovery, but varies by forest type. Remote Sens Ecol Conserv. 5(4):376–388. doi: 10.1002/rse2.113.
- Hussein EA, Abd El-Ghani MM, Hamdy RS, Shalabi LF. 2021. Do anthropogenic activities affect floristic diversity and vegetation structure more than natural soil properties in hyper-arid desert environments? Diversity. 13(4):157. doi: 10.3390/d13040157.
- Kennedy RE, Yang Z, Cohen WB. 2010. Detecting trends in forest disturbance and recovery using yearly Landsat Time Series: 1. LandTrendr - temporal segmentation algorithms. Remote Sens Environ. 114:2897–2910. doi: 10.1016/j.rse.2010.07.008.
- Krebs CJ, Boutin S, Boonstra R, Murray DL, Jung TS, O’Donoghue M, Gilbert BS, Kukka PM, Taylor SD, Morgan T, et al. 2023. Long-term monitoring in the boreal forest reveals high spatio-temporal variability among primary ecosystem constituents. Front Ecol Evol. 11:1187222. doi: 10.3389/fevo.2023.1187222.
- Kukla J, Whitfeld T, Cajthaml T, Baldrian P, Veselá‐Šimáčková H, Novotný V, Frouz J. 2019. The effect of traditional slash‐and‐burn agriculture on soil organic matter, nutrient content, and microbiota in tropical ecosystems of Papua New Guinea. Land Degrad Dev. 30(2):166–177. doi: 10.1002/ldr.3203.
- Kurniadi R, Purnomo H, Wijayanto N, Fuah AM. 2017. Model pengelolaan ternak di sekitar Hutan Gunung Mutis dan dampaknya terhadap kelestarian hutan. Jurnal Ilmu Kehutanan. 11(2):156–172. doi: 10.22146/jik.28281.
- Kwakwa PA, Alhassan H, Adzawla W. 2022. Environmental degradation effect on agricultural development: an aggregate and a sectoral evidence of carbon dioxide emissions from Ghana. JBSED. 2(1):82–96. doi: 10.1108/JBSED-10-2021-0136.
- Liu S, Wei X, Li D, Lu D. 2017. Examining forest disturbance and recovery in the subtropical forest region of Zhejiang province using landsat time-series data. Rem Sens. 9(5):479. doi: 10.3390/rs9050479.
- Margono BA. 2014. Advancing Indonesian forest resource monitoring using multi-source remotely sensed imagery [dissertation]. Maryland: University of Maryland.
- Marimpan LS, Purwanto RH, Wardhana W, Sumardi S. 2022. Carbon storage potential of Eucalyptus urophylla at several density levels and forest management types in dry land ecosystems. Biodiversitas. 23(6):2830–2837. doi: 10.13057/biodiv/d230607.
- Matas-Granados L, Pizarro M, Cayuela L, Domingo D, Gómez D, García MB. 2022. Long-term monitoring of NDVI changes by remote sensing to assess the vulnerability of threatened plants. Biol Conserv. 265:109428. doi: 10.1016/j.biocon.2021.109428.
- Mediterranean Biodiversity Protection Community. 2019. Mediterranean biodiversity protection tools catalogue. Spanyol: European Topic Centre, University of Malaga. https://biodiversity.uma.es/mbpctoolscatalogue/.
- Meigs GW, Kennedy RE, Cohen WB. 2011. A Landsat time series approach to characterize bark beetle and defoliator impacts on tree mortality and surface fuels in conifer forests. Rem Sens Environ. 115(12):3707–3718. doi: 10.1016/j.rse.2011.09.009.
- Mooy BZ. 2021. Kajian pola migrasi lebah hutan Timor (Apis Dorsata) di Kawasan Cagar Alam Gunung Mutis-Timor Tengah Selatan, Provinsi Nusa Tenggara Timur. J Bestari. 2(1):23–34. [Indonesia]
- Nau GW, Buku MNI. 2020. Inventory of woody plants in the forest area of mount Mutis Nature Preserve in East Nusa Tenggara, Indonesia. Asian J Conserv Biol. 9(2):214–220.
- Negi VS, Pathak R, Rawal RS, Bhatt ID, Sharma S. 2019. Long-term ecological monitoring on forest ecosystems in Indian Himalayan Region: criteria and indicator approach. Ecol Indic. 102:374–381. doi: 10.1016/j.ecolind.2019.02.035.
- Nguyen MD, Baez-Villanueva OM, Bui DD, Nguyen PT, Ribbe L. 2020. Harmonization of landsat and sentinel 2 for crop monitoring in drought prone areas: case studies of Ninh Thuan (Vietnam) and Bekaa (Lebanon). Remote Sens. 12(2):281. doi: 10.3390/rs12020281.
- Owari T, Im S, Guan BT. 2020. Special feature Long-term monitoring and research in Asian university forests: towards further understanding of environmental changes and ecosystem responses. J Forest Res. 25(3):134–135. doi: 10.1080/13416979.2020.1767269.
- Peprah K. 2015. Land degradation is indicative: proxies of forest land degradation in Ghana. J Degrade Min Land Manage. 3(1):477–489. doi: 10.15243/jdmlm.2015.031.477.
- Pujiono E, Sadono R, Hartono H, Imron MA. 2019b. Assessment of causes and future deforestation in the mountainous tropical forest of Timor Island, Indonesia. J Mt Sci. 16(10):2215–2231. doi: 10.1007/s11629-019-5480-1.
- Pujiono E, Sadono R, Hartono H, Imron MA. 2019a. A three decades assessment of forest cover changes in the mountainous tropical forest of Timor Island. Indonesia. Jurnal Manajemen Hutan Tropika. 25(1):51–64. doi: 10.7226/jtfm.25.1.51.
- Pujiono E, Sadono R, I MA, Wirabuana, PYAP, Hartono,. 2023. Factors contributing to forest degradation in the mountainous tropical forest: a case study of the Mutis-Timau Forest Complex, Indonesia. J Sust For. 42(7):675–694. doi: 10.1080/10549811.2022.2123349.
- Qiu D, Liang Y, Shang R, Chen JM. 2023. Improving LandTrendr forest disturbance mapping in China using multi-season observations and multispectral indices. Remote Sens. 15(9):2381. doi: 10.3390/rs15092381.
- Rumpel C. 2022. Understanding and fostering soil carbon sequestration. Cambridge: Burleigh Dodds Science Publishing Limited. doi: 10.19103/AS.2022.0106
- Sadono R, Wardhana W, Wirabuana PYAP. 2023. Estimating carbon storage of Eucalyptus urophylla vegetation in Mutis Timau Nature Reserve, East Nusa Tenggara, Indonesia using remote sensing analysis. Biodiversitas. 24(4):1946–1952. doi: 10.13057/biodiv/d240402.
- Shimizu K, Murakami W, Furuichi T, Estoque RC. 2023. Mapping land use/land cover changes and forest disturbances in Vietnam using a landsat temporal segmentation algorithm. Remote Sens. 15(3):851. doi: 10.3390/rs15030851.
- Styger E, Rakotondramasy HM, Pfeffer MJ, Fernandes EC, Bates DM. 2007. Influence of slash-and-burn farming practices on fallow succession and land degradation in the rainforest region of Madagascar. Agric Ecosyst Environ. 119(3–4):257–269. doi: 10.1016/j.agee.2006.07.012.
- Talakua SM, Osok RM. 2019. Development of a land degradation assessment model based on field indicators assessment and prediction methods in Wai Sari, Sub-Watershed Kairatu District, Western Seram Regency, Maluku Province, Indonesia. Sci Nature. 2(1):071–084. doi: 10.30598/SNvol2iss1pp071-084year2019.
- Tang KHD, Yap PS. 2020. A systematic review of slash-and-burn agriculture as an obstacle to future-proofing climate change. Proc Int Conf Clim Change. 4(1):01–19. doi: 10.17501/2513258X.2020.4101.
- Tian S, Van Dijk AI, Tregoning P, Renzullo LJ. 2019. Forecasting dryland vegetation condition months in advance through satellite data assimilation. Nat Commun. 10(1):469. doi: 10.1038/s41467-019-08403-x.
- Tsujino R, Yumoto T, Kitamura S, Djamaluddin I, Darnaedi D. 2016. History of forest loss and degradation in Indonesia. Land Use Policy. 57:335–347. doi: 10.1016/j.landusepol.2016.05.034.
- Umarhadi DA, Wardhana W, Soraya E, Senawi. 2023. Monitoring forest gain and loss based on LandTrendr algorithm and Landsat images in KTH Pati social forestry area, Indonesia.JANS. 15(3):1051–1060. doi: 10.31018/jans.v15i3.4781.
- Vásquez-Grandón A, Donoso PJ, Gerding V. 2018. Forest degradation: when is a forest degraded? Forests. 9(11):726. doi: 10.3390/f9110726.
- Wang L, Jiao W, MacBean N, Rulli MC, Manzoni S, Vico G, D’Odorico P. 2022. Dryland productivity under a changing climate. Nat Clim Chang. 12(11):981–994. doi: 10.1038/s41558-022-01499-y.
- Xiao H, Zhang X, Yan M, Zhang L, Wang H, Ma Y, Liu J. 2022. The Temporal-based forest disturbance monitoring analysis: a case study of nature reserves of Hainan Island of China From 1987 to 2020. Front Environ Sci. 10:411. doi: 10.3389/fenvs.2022.891752.
- Zhang J, Hu J, Lian J, Fan Z, Ouyang X, Ye W. 2016. Seeing the forest from drones: testing the potential of lightweight drones as a tool for long-term forest monitoring. Biol Conserv. 198:60–69. doi: 10.1016/j.biocon.2016.03.027.
- Zheng H, Zheng H. 2023. Assessment and prediction of carbon storage based on land use/land cover dynamics in the coastal area of Shandong Province. Ecol Indic. 153:110474. doi: 10.1016/j.ecolind.2023.110474.