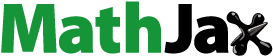
Abstract
Correct estimation of tree biomass is important when calculating uptake or emission of CO2 in relation to land-use and land-use change. The objectives of this study were (1) to estimate the root/shoot ratio for the estimation of root biomass based on the above-ground biomass (AGB) of Acacia mearnsii, and (2) to develop allometric equations for the estimation of the above-and below-ground biomass (BGB) of Acacia mearnsii. To estimate the AGB and BGB, twenty-four trees of varying ages (3, 4, 5, and 6 years) were harvested, with six trees per age group. We measured the dry biomass for different tree parts and developed allometric models using tree height (H) and diameter at breast height (DBH) as independent variables. The results showed that the biomass of the stem accounted for 69% of the total biomass, followed by branches (14%), roots (8.1%), and leaves (7.3%). The recorded mean root/shoot ratio was 0.11. The biomass of the stem and coarse roots increased with increasing tree age, while a contrary trend was observed for the other tree components. Each component has its unique allometric model.
1. Introduction
Estimating aboveground tree biomass in forests to establish biomass C stocks and the direction of change of the biomass C pool is increasingly important in climate mitigation projects (Sebrala et al. Citation2022). Measurement, reporting and verification (MRV) protocols are utilized to assess carbon dioxide emission reduction interventions like Reducing Emissions from Deforestation and Forest Degradation (REDD+) projects (Virgulino-Júnior et al. Citation2020). They are also commonly used in compilation of regional or national carbon budgets (Henry et al. Citation2011; Yu et al. Citation2014). The development of allometric equations can enhance the accuracy of tree biomass estimations and hence improve the MRV protocols (Chave et al. Citation2014).
Direct or indirect approaches and combinations thereof can be used to measure tree biomass (Henry et al. Citation2011). The direct method (cutting, weighing and determination moisture content) is the most accurate but also the most costly and time-consuming. The most popular non-destructive technique for assessing forest biomass is the application of empirical allometric equations. However, they initially require the destruction of sample trees to establish the allometric models (Chave et al. Citation2014). The most important step in improving the accuracy of measuring forest biomass is choosing the right allometric models (Picard et al. Citation2015).
The link between biomass weight and tree morphological and physiological traits such as tree height, diameter at breast height, and wood density are used for developing allometric equations (Kachamba et al. Citation2016; Zhao et al. Citation2019). For various forests and tree species, a generalized allometric model has been created (Basuki et al. Citation2009). However, tree biomass may vary considerably between tree species due to the tree architecture and morphology. It is therefore recommended to create species-specific allometric equations to minimize biased assessments of tree biomass (Chaturvedi and Raghubanshi Citation2015).
In the Fagita-Lekoma district, Acacia mearnsii plantations were started in the early 1990s as state plantation sites on degraded hill slopes. Around the year 2000 some farmers started to plant the species on their crop land in order to produce charcoal, a practice that spread quickly due to its profitability. The rapidly expanding plantations (Wondie and Mekuria,Citation2018; Liyew et al. Citation2019) imply that the plantations might be a significant carbon sequestration. The carbon sequestrations from the plantations are not yet determined due to lack of allometric biomass functions for Acacia mearnsii. The objectives of this study were (1) to estimate the root/shoot ratio for the estimation of root biomass based on the AGB of Acacia mearnsii and (2) to develop allometric equations for the estimation of the above- and below-ground biomass of Acacia mearnsii.
2. Material and methods
2.1. Study site
The study was conducted in the Fagita-Lekoma district of Awi zone, in the Amhara region of northwestern Ethiopia (). The district is located at 36°40ˈ01˝ to 37° 50ˈ21˝E longitude and 10°57ˈ23 to 11°11ˈ21˝ N latitude within the altitudinal range from 1888 to 2915 m above sea level. The agro-ecological zone of the study area is the subtropical oceanic highland. Acrisols and Leptosols are the two main soil groups found in the area (FAO, 2014). The annual mean temperature varies between 9.4 and 25 °C. The area experiences bimodal rainfall, with the majority of the precipitation falling between June and September. The average yearly rainfall ranges from 1951 to 3424 mm (Abebe et al. Citation2020).
Figure 1. Map of the study area in Fagita-Lekoma district, Amhara region, Ethiopia (a), and the point indicate the distribution of sampling points in each stand age with six replications for biomass estimation (b).
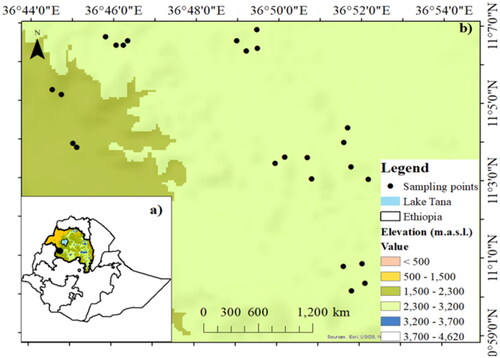
2.2. Studied species
Acacia mearnsii (black wattle) belongs to the family Fabaceae and originates from Australia. In Ethiopia, it grows well in moist and wet Weyna Dega agro-climatic zones, 1600–2500 m above sea level. The tree is a relatively short-lived species that decline in vigour after 10–15 years (Bekele-Tesemma and Tengnäs, Citation2007). It is a fast-growing and nitrogen fixing tree that reaches up to 6–12 m in height under favourable conditions. It has good potential for utilization as fuel wood, for charcoal production, and to improve soil fertility (Chanie et al. Citation2021).
2.3. Biomass sampling and data collection
Four stand ages (3–6 years) of Acacia mearnsii were chosen from farmers-managed woodlots to represent various diameter classes. Six representative sample trees were chosen at each stand age; a total of 24 sample trees were cut down close to the ground. Following the harvesting of the sample trees, the stems, branches, and leaves were separated, and the fresh weights of each component were measured in the field. Subsamples were taken from each component of the stem (in five equal parts measuring 5 cm in length), branch, and leaf. Samples of roots were taken down to a soil depth of 40 cm. After sieving, roots were separated and classified into three categories: fine (< 2 mm), medium (2–5 mm), and coarse roots (> 5 mm) based on their diameter classes. Subsamples were taken after total fresh biomass was measured. The subsamples were packed in a plastic bag, and transported to the University of Gondar for drying and weighing. The stem subsamples were oven-dried at 105 °C, while other components were oven-dried at 65 °C until they reached a constant weight. The root/shoot ratio was calculated as the ratio of the total dry weight of the roots to the dry weight of the AGB.
2.4. Statistical analysis and model development
Allometric equations may use diameter at breast height (DBH) as a single variable. Combing DBH with tree height (H) can improve the accuracy of the allometric equation (Kachamba et al. Citation2016; Sukhbaatar et al. Citation2023; Zhao et al. Citation2019). In this study, four allometric equations with the combination of DBH and H as predictor variables were developed by non-linear regression to estimate the stem, branch, leaves, above-ground biomass (AGB), below-ground biomass (BGB), and total tree biomass (TB) (). Several metrics, including the coefficient of determination (R2), mean absolute difference (MAD), root mean square error (RMSE), Akaike information criterion (AIC), and p-value, were used to assess its accuracy and efficiency (Pham Citation2019; Zeng et al. Citation2017). Models with higher values of R2 and lower scores of RMSE, MAD, and AIC are better for predicting below- and above-ground biomass (Djomo and Chimi Citation2017; Kusmana et al. Citation2018; Virgulino-Júnior et al. Citation2020). These values are acquired based on the following formulas;
(Eq. 1)
(Eq. 1)
(Eq. 2)
(Eq. 2)
(Eq. 3)
(Eq. 3)
(Eq. 4)
(Eq. 4)
where, yi = the observed biomass, ŷi = the predicted biomass, ỹi = the mean biomass of n plots, n = the number of samples, L = log-likelihood estimates, k = number of independent variables, R2 = coefficient of determination, RMSE = root mean squared error, MAD = mean absolute difference, and AIC = akaike information criteria.
Table 1. Allometric equations for predicting BGB and AGB of Acacia mearnsii.
3. Results
3.1. Biomass distribution and proportion of the tree components
Based on the descriptive statistics of the measured variables presented in , different tree ages of selected sample trees have variations in DBH, tree height, and tree biomass variables. The mean DBH and tree height of selected trees were 7.1 ± 0.001 cm and 4.4 ± 0.26 m, respectively (). The proportion of stem biomass gradually increased with increasing tree age, while the opposite was observed for other tree components (). The largest biomass amount for the stem was 7.81 kg/tree (69%), followed by branch biomass which was 1.63 kg/tree (14%). While the BGB was 0.91 kg/tree (8.1%) and leaf biomass 0.83 kg/tree (7.3%) (). Based on the estimation of BGB and AGB, the average root/shoot ratio of the Acacia mearnsii tree was 0.11, varying from 0.15 for the three years age class to 0.08 for the six years age class. In comparison, root biomass allocation by diameter classes, the coarse root biomass (> 5 mm) increased with increasing tree age, representing 82% of the total root biomass (). Moreover, the allocation of fine roots (< 2 mm) and medium roots (2–5 mm) decreased with increasing tree age, and the fine and medium root biomass accounted for 13 and 5.5% of the overall BGB, respectively ().
Figure 2. Proportion of each biomass component in total tree biomass (a) and distribution of root size classes in total root biomass depending on each stand age (b).
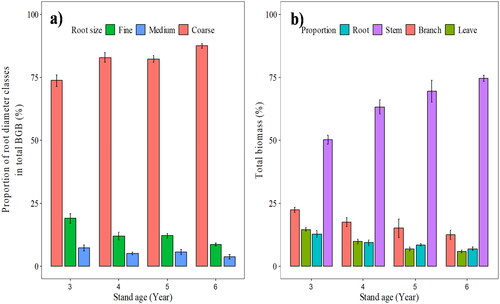
Figure 3. Comparison of predicted AGB with our best model AGB = 1.92 + 0.02(DH)1.68, predicted AGB with the equations of Brown (1997) AGB = exp(-2.134 + 2.53ln(D); predicted AGB with equations of Berhe et al. (Citation2013) AGB= 0.0163*D2H (a); predicted BGB with our best equations BGB = 0.01D2.57 H0.09; predicted BGB with equations of Usoltsev et al. (Citation2016) BGB = exp(-1.6042 + 2.5524 + ln(D)-0.803ln(H) (b).
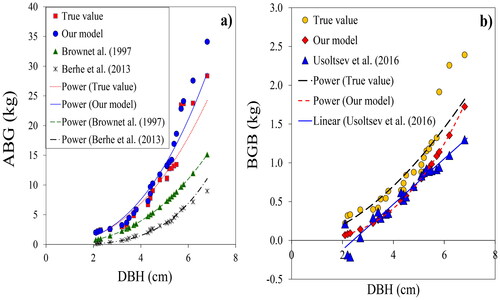
Table 2. Statistical description of tree biomass components.
3.2. Allometric models for each tree component
The parameters of four allometric models together with their statistical performance indicators are given in . From the selected allometric models, a specific model was developed for each tree component. The R2 of the best allometric equations ranged from 0.50 to 0.98. This means the biomass variations of Acacia mearnsii can be well explained (50 < R2 < 98%) by the combination of tree height and DBH (). The selected allometric equations on stem biomass show that the values of RMSE, MAD, and AIC are lowest in model 1 compared to the second selected model (). This model can explain stem biomass variations well (R2 > 98%).
Table 3. Comparison of allometric equations to estimate the AGB and BGB for Acacia mearnsii. The best performing model for each category is indicated in bold. D: diameter at breast height (cm); H: tree height (m); a, b, and c: equation parameters; R2: coefficient of determination; RMSE: root mean square error; MAD: mean absolute deviation; and AIC: akaike information criteria. The allometric equations are statistically significant at 0.05 if the p-value <0.05.
The same result as for the stem biomass was found in the above-ground biomass (AGB) and total biomass, in which model 1 performed better (it has the highest values of R2 > 97% and the lowest values of RMSE, MAD, and AIC) than the other tested models (), which indicated that this model estimate more is more accurate for the stem, AGB, and total biomass ().
The best allometric model for leaf biomass was model 4. The values AIC were lower in model 4 than in other selected models (). Although model 3 had similar values to model 4 for RMSE and MAD, the AIC was lower. Further, model 3 was not significant (p > 0.05).
The best allometric models for BGB and branch biomass were model 3. For BGB, model 3 can explain the biomass variations (R2 > 90%), and it had lower MAD values (, ). Although model 2 had lower values of AIC, the model was not significant (p > 0.05). For leaf biomass, no selected allometric model could explain the biomass variations well (R2 < 60%). Model 4 produced better accuracy for biomass estimation (lower values of RMSE, MAD, and AIC), and it explained 50% of biomass variation ().
4. Discussion
4.1. Biomass allocation and root/shoot ratio
In this study, the highest proportion of biomass is found in stem biomass, and the second largest biomass component is branch biomass across stand age. This is consistent with other research findings (Yang et al. Citation2019; Zhang et al. Citation2014). The variation in the allocation of biomass at different stand ages, in the early ages of the stand root and leaves, is critical for the acquisition of scarce water and nutrient resources from the ground (Yang et al. Citation2019).
The calculation of the BGB and AGB of trees is crucial for understanding the dynamics of carbon stocks (Sukhbaatar et al. Citation2023). According to Agathokleous et al. (Citation2019), the root/shoot ratio revealed the trees’ potential for acquiring water and nutrients in comparison to their development potential. Although it changes depending on the size and species of the tree, it is a useful tool for quickly estimating the BGB based on the AGB for carbon assessment (Sukhbaatar et al. Citation2023; Zhao et al. Citation2019). In this study, the mean root/shoot ratio was 0.11. The root/shoot ratio of Acacia mearnsii gradually decreased with increasing stand age. This is less than the root/shoot ratios of Sonneratia species 0.23 (Kusmana et al. Citation2018) and Larix sibirica 0.25 (Sukhbaatar et al. Citation2023).
4.2. Selected models for biomass prediction
In this study, the allometric model for Acacia mearnsii was developed by using a combination of DBH and tree height. Measurements of DBH and tree height are easy in the field. Hence, researchers chose these variables in combination when creating allometric models (Chave et al. Citation2014; Getnet and Negash Citation2021; Gurmessa et al. Citation2016; Mugasha et al. Citation2013; Zhao et al. Citation2019). As per several studies (Bi et al. Citation2015; Kenzo et al. Citation2009; McCormack et al. Citation2015; Sukhbaatar et al. Citation2023; Zhao et al. Citation2019), the biomass allometric model that is created by combining DBH and H is generally more accurate than using DBH alone.
The best allometric models for each tree component of Acacia mearnsii differed in accuracy from one another, with R2 values ranging from 50.6 to 98.6%. The stem biomass had the highest value, while the branch biomass had the lowest value. The stem, AGB, and total biomass all had the best allometric models with 98.6, 97.5, and 97.8%, respectively. This study have higher R2 values than those of Sitoe et al. (Citation2014) on the AGB of mangrove forests (R2 = 89%), Zhao et al. (Citation2019) on the AGB and total biomass of sub-tropical forests in southern China (R2 = 90%), Kenzo et al. (Citation2020) on the stem biomass and AGB of Tectona grandis (R2 = 99 and 98%), and Kangkuso et al. (Citation2016) on the stem biomass of Lumnitzera racemosa tree (R2 = 98.9%). Additionally, the BGB’s R2 value is similar to that of Kachamba et al. (Citation2016) on the BGB of Miombo woodlands (R2 = 94%) and Sukhbaatar et al. (Citation2023) on the BGB of Larix sibirica trees (R2 = 98%). Nevertheless, the biomass of the branches and leaves has lower R2 values than the other tree components.
5. Conclusion
The biomass distribution of Acacia mearnsii increased with increasing tree ages. The largest biomass was recorded in stems (69%), followed by branches (14%), roots (8.1%), and leaves (7.3%). A comparison of root biomass allocation by diameter fractions showed that the coarse roots made up 82% of the total root biomass, and the average root/shoot ratio was 0.11. The biomass equations that use the combination of DBH and H are more precise than equations that are solely based on DBH. Consequently, the best-fit allometric models for estimating AGB and BGB are 1.92 + 0.02(DH)1.68 and 0.01D2.57H0.09, respectively. The selected allometric equations can be used to estimate the biomass and carbon stock of Acacia mearnsii.
Acknowledgements
The authors express their gratitude to Amhara Agricultural Research Institute (ARARI) and Swedish Research Council (VR) for funding this study, as well as to the local farmers and agricultural experts who granted permission to harvest the trees and participated in the data collection.
Conflict of interest
There is no conflict of interest declared by the authors.
Additional information
Funding
References
- Abebe, G., T. Atsushi, N. Haregeweyn, T. Taniguchi, M. Wondie, E. Adgo, M. Tsugiyuki, T. Mitsuru, K. Ebabu & M. Liyew, 2020. Effects of land use and topographic position on soil organic carbon and total nitrogen stocks in different agro-ecosystems of the upper blue Nile Basin. Sustainability 12(6):2425 doi: 10.3390/su12062425.
- Addo-Danso, S. D., C. E. Prescott & A. R. Smith, 2016. Methods for estimating root biomass and production in forest and woodland ecosystem carbon studies: A review. Forest Ecology and Management journal 359:332–351 doi: 10.1016/j.foreco.2015.08.015.
- Agathokleous, E., R. G. Belz, M. Kitao, T. Koike & E. J. Calabrese, 2019. Does the root to shoot ratio show a hormetic response to stress? An ecological and environmental perspective. Journal of Forestry Research 30(5):1569–1580 doi: 10.1007/s11676-018-0863-7.
- Basuki, T., P. Van Laake, A. Skidmore & Y. Hussin, 2009. Allometric equations for estimating the above-ground biomass in tropical lowland Dipterocarp forests. Forest ecology management 257(8):1684–1694.
- Bekele-Tesemma, A. & B. Tengnäs, 2007. Useful trees and shrubs of Ethiopia: identification, propagation, and management for 17 agroclimatic zones. RELMA in ICRAF Project, World Agroforestry Centre, Eastern Africa Region Nirobi.
- Berhe, L., G. Assefa & T. Teklay, 2013. Models for estimation of carbon sequestered by Cupressus lusitanica plantation stands at Wondo Genet, Ethiopia. Southern Forests: a Journal of Forest Science 75(3):113–122 doi: 10.2989/20702620.2013.805511.
- Beshir, M., F. Yimer, N. Brüggemann & M. Tadesse, 2022. Soil Properties of a Tef-Acacia decurrens-Charcoal Production Rotation System in Northwestern Ethiopia. soil systems 6(2):44 doi: 10.3390/soilsystems6020044.
- Bi, H., S. Murphy, L. Volkova, C. Weston, T. Fairman, Y. Li, R. Law, J. Norris, X. Lei & G. Caccamo, 2015. Additive biomass equations based on complete weighing of sample trees for open eucalypt forest species in south-eastern Australia. Forest Ecology and Management 349(1):106–121 doi: 10.1016/j.foreco.2015.03.007.
- Bijak, S., M. Zasada, A. Bronisz, K. Bronisz, M. Czajkowski, Ł. Ludwisiak, R. Tomusiak & R. Wojtan, 2013. Estimating coarse roots biomass in young silver birch stands on post-agricultural lands in central Poland. Silva Fennica 47(2):1–14. doi: 10.14214/sf.963.
- Chanie, Y. & A. Abewa, 2021. Expansion of Acacia decurrens plantation on the acidic highlands of Awi zone, Ethiopia, and its socio-economic benefits. Cogent Food and Agriculture 7(1):1917150 doi: 10.1080/23311932.2021.1917150.
- Chaturvedi, R. K. & A. Raghubanshi, 2015. Allometric models for accurate estimation of aboveground biomass of teak in tropical dry forests of India. Forest Science 61(5):938–949. doi: 10.5849/forsci.14-190.
- Chave, J., M. Réjou‐Méchain, A. Búrquez, E. Chidumayo, M. S. Colgan, W. B. Delitti, A. Duque, T. Eid, P. M. Fearnside & R. C. Goodman, 2014. Improved allometric models to estimate the aboveground biomass of tropical trees. Global change biology 20(10):3177–3190 doi: 10.1111/gcb.12629.
- Djomo, A. N. & C. D. Chimi, 2017. Tree allometric equations for estimation of above, below and total biomass in a tropical moist forest: Case study with application to remote sensing. Forest Ecology and Management 391:184–193 doi: 10.1016/j.foreco.2017.02.022.
- Getnet, D. & M. Negash, 2021. Allometric equations for estimating aboveground biomass of khat (Catha edulis)-stimulate grown in agroforestry of Raya Valley, Northern Ethiopia. Heliyon 7(1). doi: 10.1016/j.heliyon.2020.e05839.
- Gurmessa, F., T. Gemechu, T. Soromessa & E. Kelbessa, 2016. Allometric equations to estimate the biomass of Oxytenanthera abyssinica (A. Rich.) Munro.(Ethiopian lowland bamboo) in dicho Forest, Oromia region, Western Ethiopia. International Journal of Research Studies in Biosciences 4(12):33–48. doi: 10.20431/2349-0365.0412005.
- Henry, M., N. Picard, C. Trotta, R. Manlay, R. Valentini, M. Bernoux & L. Saint André, 2011. Estimating tree biomass of sub-Saharan African forests: a review of available allometric equations. Silva Fennica 48(3B):477–569. doi: 10.14214/sf.38.
- Kachamba, D. J., T. Eid & T. Gobakken, 2016. Above-and belowground biomass models for trees in the miombo woodlands of Malawi. Forests 7(2):38 doi: 10.3390/f7020038.
- Kangkuso, A., J. Jamili, A. Septiana, R. Raya, I. Sahidin, U. Rianse, S. Rahim, A. Alfirman, S. Sharma & K. Nadaoka, 2016. Allometric models and aboveground biomass of Lumnitzera racemosa Willd. forest in Rawa Aopa Watumohai National Park, Southeast Sulawesi, Indonesia. Forest science and technology 12(1):43–50. doi: 10.1080/21580103.2015.1034191.
- Kenzo, T., W. Himmapan, R. Yoneda, N. Tedsorn, T. Vacharangkura, G. Hitsuma & I. Noda, 2020. General estimation models for above-and below-ground biomass of teak (Tectona grandis) plantations in Thailand. Forest Ecology and Management 457:117701. doi: 10.1016/j.foreco.2019.117701.
- Kenzo, T., T. Ichie, D. Hattori, T. Itioka, C. Handa, T. Ohkubo, J. J. Kendawang, M. Nakamura, M. Sakaguchi & N. Takahashi, 2009. Development of allometric relationships for accurate estimation of above-and below-ground biomass in tropical secondary forests in Sarawak, Malaysia. Journal of Tropical Ecology 25(4):371–386 doi: 10.1017/S0266467409006129.
- Kusmana, C., T. Hidayat, T. Tiryana & O. Rusdiana, 2018. Allometric models for above-and below-ground biomass of Sonneratia spp. Global ecology and conservation 15:e00417 doi: 10.1016/j.gecco.2018.e00417.
- Liyew, B. M., A. Tsunekawa, N. Haregeweyn, D. T. Meshesha, E. Adgo, M. Tsubo, T. Masunaga, A. A. Fenta, D. Sultan & M. Yibeltal, 2019. Exploring land use/land cover changes, drivers and their implications in contrasting agro-ecological environments of Ethiopia. Land use policy 87:104052 doi: 10.1016/j.landusepol.2019.104052.
- Marziliano, P. A., R. Lafortezza, U. Medicamento, L. Lorusso, V. Giannico, G. Colangelo & G. Sanesi, 2015. Estimating belowground biomass and root/shoot ratio of Phillyrea latifolia L. in the Mediterranean forest landscapes. Annals of forest science 72:585–593. doi: 10.1007/s13595-015-0486-5.
- McCormack, M. L., I. A. Dickie, D. M. Eissenstat, T. J. Fahey, C. W. Fernandez, D. Guo, H. S. Helmisaari, E. A. Hobbie, C. M. Iversen & R. B. Jackson, 2015. Redefining fine roots improves understanding of below‐ground contributions to terrestrial biosphere processes. New Phytologist 207(3):505–518 doi: 10.1111/nph.13363.
- Mugasha, W. A., T. Eid, O. M. Bollandsås, R. E. Malimbwi, S. A. O. Chamshama, E. Zahabu & J. Z. Katani, 2013. Allometric models for prediction of above-and belowground biomass of trees in the miombo woodlands of Tanzania. Forest Ecology and Management 310:87–101 doi: 10.1016/j.foreco.2013.08.003.
- Pham, H., 2019. A new criterion for model selection. Mathematics 7(12):1215 doi: 10.3390/math7121215.
- Picard, N., F. Boyemba Bosela & V. Rossi, 2015. Reducing the error in biomass estimates strongly depends on model selection. Annals of forest Science 72:811–823. doi: 10.1007/s13595-014-0434-9.
- Sadono, R., W. Wahyu, P. Y. A. P. Wirabuana & F. Idris, 2021. Allometric equations for estimating aboveground biomass of Eucalyptus urophylla ST Blake in East Nusa Tenggara. Jurnal Manajemen Hutan Tropika 27(1):24–24 doi: 10.7226/jtfm.27.1.24.
- Sebrala, H., A. Abich, N. Mesele, Z. Asrat & L. Bohdan, 2022. Allometric Equations for Estimating Biomass and Volume of Ethiopian Forests and Establishing a Database: Review. Trees, Forests and People doi: 10.1016/j.tfp.2022.100314.
- Sitoe, A. A., M. Comissario, J. Luis & B. S. Guedes, 2014. Biomass and carbon stocks of Sofala bay mangrove forests. Forests 5(8):1967–1981. doi: 10.3390/f5081967.
- Sukhbaatar, G., D. Chimednyam, B. Nachin, B. Ganbaatar & A. Gradel, 2023. Allometric equations for the estimation of above-and below-ground biomass for Larix sibirica Ledeb. in Northern Mongolia. Forest Science and Technology 19(1):12–20 doi: 10.1080/21580103.2023.2165173.
- Usoltsev, V., V. Chasovskikh, Y. V. Noritsina & D. Noritsin, 2016. Allometric models of tree biomass for airborne laser scanning and ground inventory of carbon pool in the forests of Eurasia: comparative analysis. Journal of Forest Science (4):68–76.
- Virgulino-Júnior, P. C. C., D. N. Carneiro, W. R. NascimentoJr, M. F. Cougo & M. E. B. Fernandes, 2020. Biomass and carbon estimation for scrub mangrove forests and examination of their allometric associated uncertainties. PloS one 15(3):e0230008 doi: 10.1371/journal.pone.0230008.
- Wondie, M. & W. Mekuria, 2018. Planting of Acacia decurrens and dynamics of land cover change in Fagita Lekoma District in the Northwestern Highlands of Ethiopia. Mountain research and development 38(3):230–239 doi: 10.1659/MRD-JOURNAL-D-16-00082.1.
- Yang, B., W. Xue, S. Yu, J. Zhou & W. Zhang, 2019. Effects of stand age on biomass allocation and allometry of Quercus acutissima in the Central Loess Plateau of China. Forests 10(1):41 doi: 10.3390/f10010041.
- Yu, G., Z. Chen, S. Piao, C. Peng, P. Ciais, Q. Wang, X. Li & X. Zhu, 2014. High carbon dioxide uptake by subtropical forest ecosystems in the East Asian monsoon region. Proceedings of the National Academy of Sciences 111(13):4910–4915 doi: 10.1073/pnas.1317065111.
- Zeng, W., L. Zhang, X. Chen, Z. Cheng, K. Ma & Z. Li, 2017. Construction of compatible and additive individual-tree biomass models for Pinus tabulaeformis in China. Canadian Journal of Forest Research 47(4):467–475 doi: 10.1139/cjfr-2016-0342.
- Zhang, H., T. Song, K. Wang, H. Du, Y. Yue, G. Wang & F. Zeng, 2014. Biomass and carbon storage in an age-sequence of Cyclobalanopsis glauca plantations in southwest China. Ecological engineering 73:184–191 doi: 10.1016/j.ecoleng.2014.09.008.
- Zhao, H., Z. Li, G. Zhou, Z. Qiu & Z. Wu, 2019. Site-specific allometric models for prediction of above-and belowground biomass of subtropical forests in Guangzhou, southern China. Forests 10(10):862 doi: 10.3390/f10100862.