ABSTRACT
This study estimates the benefits of beach quality improvements, using travel costs as an implicit and entrance fee as an explicit payment vehicle in two otherwise identical labelled discrete choice site selection models. Including entrance fee as an explicit payment vehicle in addition to implicit travel costs is expected to affect beach visitors’ preferences and willingness to pay (WTP) since travel costs only are not expected to measure maximum WTP. Convergent validity of preference parameters and WTP derived from the two identical discrete choice experiments (DCEs) is tested using a split-sample approach and specifying a mixed logit choice model. Both preferences and scale parameters are significantly different between the two samples. As expected, mean WTP values are higher when an explicit entrance fee is included in the DCE. Our results suggest that implicit payment vehicles in choice experiments underestimate welfare changes. Beach visitors’ positive WTP holds promise for the introduction of economic instruments such as entrance fees to support the financial sustainability of improved beach management.
1. Introduction
Coastal urbanisation, industrialisation and population expansion generate waste and water pollution that degrade coastal resources such as beaches, estuaries, coral reefs and mangroves around the world. Although coastal resources play an important role in local and national economies by providing recreational benefits to visitors, their economic value is often hard to quantify due to their public good characteristics. Beaches experiencing poor coastal water quality, littering and over-crowding may lead to substantial welfare losses for residents and visitors. To avoid such welfare losses and improve the economic gains associated with recreational beach benefits, policy makers and coastal resource managers often look for information about the social value of coastal waters and other environmental quality improvements at beaches, so that they can manage these resources in an economically efficient and sustainable manner.
Beach site selection discrete choice experiments (DCE's) may provide information to policy makers and resource managers about visitors’ beach site choice behaviour. In such DCEs visitors are asked to choose a beach out of multiple alternative sites, keeping in mind the sites’ attributes and their varying levels across choice sets. This method allows researchers to assess visitors’ marginal willingness to pay (WTP) for (future) changes in the sites’ attributes if a payment vehicle is included (Carlsson, Frykblom, and Lagerkvist Citation2007). As these site selection DCEs include alternative beach locations, each visitor has her or his own individual-specific travel cost, which has traditionally been used as an implicit payment vehicle for estimating WTP values. For estimating these WTP values, the inclusion of distance and travel time as a proxy for visitors’ travel costs in DCEs is often considered adequate (e.g. Adamowicz et al. Citation1994; Hanley, Wright, and Koop Citation2002; Christie, Hanley, and Hynes Citation2007). However, from a financial management perspective, relying on this implicit payment vehicle only may be insufficient as resource managers may need additional funding sources for managing coastal resources, especially in developing countries. Moreover, and more importantly from the perspective of measuring welfare changes, travel costs likely do not (or only by accident) measure individual maximum WTP of individuals, implying underestimation of welfare changes. By design, adding an explicit price to a DCE gets us closer to maximum WTP than what is possible using travel costs only, implying that adding such an explicit price to the choice experiment design may affect choice behaviour and increase WTP estimates. This would imply that DCE studies using implicit payment vehicles underestimate welfare changes.
Our study addresses and tests this issue explicitly. A split-sample approach is used, implementing two identical labelled DCEs with and without the explicit entrance fee to investigate how preferences and WTP estimates are affected when the number of payment vehicles and total price increases. The first choice model (SP1) estimates the impact of the calculated future travel cost increase as a monetary control variable on choice behaviour and the second model (SP2) combines the hypothetical entrance fee with the future travel cost increase. Differences in preferences between the two models and the derived WTP welfare measures are empirically tested using convergent validity and non-parametric combinatorial tests. This is to our knowledge the first study to investigate the separate and combined effect of implicit and explicit payments on public preferences and their acceptability of these payments in terms of WTP values using labelled site selection DCEs and a split sample approach.
In the next sections, we discuss the limited number of previous studies on this issue, describe how our study differs from existing studies, and argue how our study design provides better insight into the issue at hand. The remainder of this paper is organized as follows. Section 2 describes the general research methodology and presents our research hypotheses, while Section 3 presents the case study area. This is followed by a discussion of the survey and DCE design in Section 4 and the site selection modelling framework in Section 5. The results are reported in Section 6, and aggregate welfare effects are presented and discussed in Section 7. Section 8 concludes.
2. Existing literature
Several empirical studies have been conducted around the world to estimate the non-market value of clean coastal waters and other beach attributes under a random utility modelling (RUM) framework, using either actual choices in revealed preferences (RP) methods, such as the travel cost method (TCM) (e.g. Hilger and Hanemann Citation2006), or hypothetical choices in stated preferences (SP) methods, such as contingent valuation method (e.g. Ferrini, Schaafsma, and Bateman Citation2014), contingent behaviour (e.g. Whitehead et al. Citation2010) or DCEs (e.g. Eggert and Olsson Citation2004; Brouwer et al. Citation2017). Some research combines RP and SP data and tests convergent validity of visitors’ preferences for site quality improvements (e.g. Adamowicz, Louviere, and Williams Citation1994, Citation1997; Hanley, Wright, and Koop Citation2002).
In the DCE literature, usually one single payment vehicle is included in the experimental design. However, different payment vehicles may be available when estimating the same recreational services provided by beach resources. For example, Smith, Zhang, and Palmquist (Citation1997) and Brouwer et al. (Citation2017) use combinations of entrance fees and local taxes in the US, Netherlands, Greece and Bulgaria. Blakemore and Williams (Citation2008) and Priyapada and Wang (Citation2014) use an entrance fee for beaches in Turkey and Thailand, while Loomis and Santiago (Citation2013) do not specify the payment vehicle, but instead refer to an increase in travel costs. The use of more than one payment vehicle for the purpose of testing convergent validity of preferences and WTP values is a more recent phenomenon in the environmental valuation literature, where studies investigate the influence of multiple payment vehicles on preferences and WTP values in a variety of different ways, pertaining to their (i) inclusion or exclusion (e.g. Carlsson, Frykblom, and Lagerkvist Citation2007; Van Zanten, Koetse, and Verburg Citation2016), (ii) types (e.g. Rai and Scarborough Citation2013; Gibson et al. Citation2016), and (iii) levels, ranges and directions (e.g. Carlsson, Frykblom, and Lagerkvist Citation2007; Aravena, Peter, and Scarpa Citation2014; Koetse and Brouwer Citation2016).
In the SP literature, considerable attention has been paid to the inclusion of travel costs as an implicit payment vehicle due to the fact that it is expected to help avoid protest against the way respondents are asked to pay for public goods, which are typically provided free of charge. However, this approach has only been applied using unlabelled DCEs, and including in most cases only one single implicit payment vehicle or price vector, associated with either distance as a proxy for travel cost (e.g. Christie, Hanley, and Hynes Citation2007) or an (undefined) additional travel cost (e.g. Hynes, Hanley, and Tinch Citation2013), not including the opportunity costs of travel time and without consideration of any explicit payment vehicle, such as an entrance fee and its impact on preferences and WTP values. Originally inspired by the Mackenzie (Citation1993) hunting valuation study, which included hunting trip costs as an implicit and a license fee as an explicit payment vehicle, Campos, Caparro's, and Oviedo (Citation2007) use a contingent valuation study to show that different payment vehicles have different welfare consequences. Similarly, Oviedo et al. (Citation2016) use an unlabelled DCE and simultaneously include and test the effect of two alternative payment vehicles, i.e. an increase in trip expenditures as a result of an increase in fuel prices (without including the opportunity cost of travel time) and an entrance fee to access two public forests in Spain. They also find substantial differences in welfare estimations associated with the different payment vehicles.
Although the experimental designs used by Campos, Caparro's, and Oviedo (Citation2007) and Oviedo et al. (Citation2016) provide useful insights, they do not (or only to a limited extent) allow for testing whether an implicit payment vehicle elicits maximum WTP, which is the central hypothesis tested in this paper. Our research design is different in that it examines whether beach visitors are sensitive to the inclusion of an additional explicit payment vehicle, i.e. an entrance fee, in addition to the inclusion of a future travel cost increase as an implicit payment vehicle. For this, we ask one sample of respondents to consider travel costs only as an implicit monetary variable, while in another sample respondents face the same travel costs combined with the entrance fee attribute.Footnote1 Since different payment vehicles affect public preferences differently (Carlsson, Frykblom, and Lagerkvist Citation2007; Van Zanten, Koetse, and Verburg Citation2016), including an explicit payment vehicle in addition to the implicit payment vehicle is expected to affect preferences and WTP estimates. Specifically, implicit prices obtained from travel costs may not cover maximum WTP of individuals, in which case adding an explicit price should increase WTP estimates.
3. Case study
Despite public and policy concerns in Pakistan, so far no valuation studies have been conducted that provide coastal resource managers and policy makers with insights into economic values of coastal resources and the social costs of their pollution and degradation. Therefore, this study aims at estimating local resident WTP for improvements in coastal water quality, beach cleanliness, overcrowding and recreational and sanitary facilities on the beaches in Karachi, Pakistan. Besides assessing the impact of including an explicit payment vehicle on public WTP, we also examine public acceptance of the introduction of an entrance fee to support more sustainable coastal policy development and implementation, in particular with the aim of financing coastal resources management in the future.
Karachi, the capital city of Sindh province, is a major port in Pakistan. It is the most urbanised and industrialised city of Pakistan with a population of over 16 million (KSDP: Karachi Strategic Development Plan Citation2007). The economy of the city accounts for 20 per cent of Pakistan's GDP (FBR: Federal Board of Revenue Citation2006-07). Karachi's coastline is around 70 km long, stretching from the Mubarak Village beach in the west to Korangi creek near the Indus Delta in the east (see ). This coastline provides a wealth of recreational and other opportunities to the residents of the city, including fisheries, mangrove forest products, seaside agriculture, coastal wildlife, aquatic resources and shoreline stabilisation services (Khalil Citation1999). Due to their public good nature, the economic importance of many if not most of these services, such as beach recreation, coastal water quality and sea life, goes unaccounted for in policy and decision-making. Coastal resource managers and policy makers consequently do not have adequate information to design and implement sustainable coastal zone development strategies, especially when it comes to the potential welfare losses associated with the degradation of recreational use values of beaches due to growing population pressure and industrial development.
Karachi has several beaches, which provide a variety of water and beach-related recreational opportunities (e.g. swimming, boating and diving) to the local population. Eight of these beaches are included in our study and presented in (from east to west): Clifton/Sea View, Manora Island, Sandspit, Hawke's Bay, French, Paradise Point, Cape Mount, and Mubarak Village beach. These beaches are visited frequently. During the surveys we estimated the total number of annual visits to each beach using beach frequency counts during peak hours on both weekdays and weekends. The number of estimated visits to all eight beaches is presented in . The aggregate estimated number of visits to all eight beaches is nearly 5.7 million per year. Clifton/Sea View beach is closest to Karachi and is visited most with 1.5 million visits per year, while Mubarak Village beach is farthest away from Karachi and visited least with 0.3 million visits per year (see also ). Three beaches are excluded. The first beach excluded is Russian beach, which is not a sea beach, but a remote beach located within Korangi creek in the Indus Delta that is only occasionally visited by a limited number of visitors. The second beach that was excluded is Nathiagali beach, which is managed by the Pakistan Navy and is only accessible to the naval forces. The third beachs, Sunehra beach, is used for fishing only. As beach activities, such as swimming, boating, diving, playing sports and walking, are restricted at Nathiagali beach, commercially regulated due to fishing at Sunehra beach, and limited at Russian beach due to its relatively small width, remoteness and location within Korangi creek, the site improvements in water quality, cleanliness, crowding and facilities pertaining to these three excluded beaches were not considered relevant, because beach visitors interviewed during the pre-tests, which initially included these three beaches, believed that these three beaches provide no real substitutes to the other eight beaches included in the choice set, and suggested to exclude them.
To assess the level of complexity of our labelled choice tasks, we also pre-tested choice tasks with less alternatives, e.g. tasks that included 4 or 5 beaches in each choice situation, during the focus group meetings. However, the majority of the group meeting participants agreed that the 8 beaches constitute the main set of alternatives among which beachgoers would choose and should hence be included in each choice situation. The labelled choice set we use in the choice experiment is therefore considered to accurately represent the choice set facing most Karachi beach visitors. If certain beaches were considered literally to lie outside of a person's choice set, he/she could always ignore that option, thereby reducing choice complexity.
Social and economic activities in the city, such as residential building, fisheries, ports and shipping, power plants and industry, recreation and tourism, have expanded enormously over the years and increased the pressure on coastal resources, specifically on beaches and coastal waters. There are above 6,000 industrial units in the metropolitan area of Karachi, discharging effluents directly or indirectly through the Layari and Malir rivers into the coastal waters. Similarly, the coastal power plants and some industries are important sources of thermal pollution, collectively discharging about 1,500 million cubic meters of warm water annually into the Arabian Sea, thus raising the levels of coastal water temperatures that threaten sea life. About 330 million gallons of municipal sewage water make their way to the coastal waters every day through these rivers, drainage and other waterways. Less than 30 percent of the urban sewage water is treated and the rest is dumped into the sea as raw sewage and untreated industrial waste Citation(KSDP: Karachi Strategic Development Plan 2007). Also, coastal agricultural pollutants, including fertilizers, pesticides and herbicides, as well as waste from construction sites and poultry holdings, make their way into the coastal waters (Mansoor and Mirza Citation2007).
Besides coastal water quality degradation, beach littering is another important problem, adversely affecting the recreational use benefits associated with beach recreation in the city. The Karachi Municipal Corporation (KMC) collects and disposes about 20–30 percent of the 8,000–10,000 tons of litter generated every day, whereas the remaining litter is either left in the city or dumped near the river banks and waterways, where it is ultimately blown away by the wind or washed down to the coastal waters (SACEP Citation2007). Only two urban beaches, Clifton/Sea View and Manora Island, are cleaned regularly by the authorities in Karachi. The remaining beaches get cleaned only occasionally, either by village organisations or local non-governmental organisations. Overcrowding is another important factor that contributes to beach littering due to visitors leaving behind their garbage. Overcrowding is hard to tackle because the public has free access to the beaches.
Although Pakistan is a signatory to a number of international conventions, protocols and treaties on coastal environment related issues, including MARPOL 73/78, the Convention on Marine Biological Diversity, and several others, presently there is no legislation that specifically addresses coastal water pollution, seaside littering and shoreline crowding in Pakistan. The country only has an umbrella type of Environmental Protection Act from 1997, which aims to protect the coastal and marine environment as a whole (Talpur and Jariko Citation2001). A Marine Pollution Control Board exists to deal with matters such as coastal water quality and beach litter (SACEP Citation2007), but there is no clear policy planning, coordination and implementation among responsible authorities.
Knowing that the deterioration of coastal water quality, littering and overcrowding along the beaches in Karachi bears significant social costs, and realising that the lack of financial resources may further aggravate coastal resource degradation, the public authorities are considering whether or not to introduce an entrance fee to manage site access. Introduction of an entrance fee would generate revenues for coastal management authorities who lack financial resources for environmental quality improvements, such as coastal water treatment, beach clean-up and controlling overcrowding problems.
4. Survey design and site selection choice experiment
The survey was divided into four sections. The first section refers to respondents’ past and current site choice and travel behaviour, eliciting information regarding distance from home to the different beaches they visited in the past, trip costs, trip time, on-site costs, on-site time, trip purpose, trip type, and beach activities they undertake when visiting one or more of the beaches. A city map depicting the exact locations of the eight beaches in the Karachi metropolitan area was used to assess the perceived distance from a respondent's home to each beach. The second section records respondents’ opinions regarding beach attributes, such as water quality, beach cleanliness, crowding, noise, facilities and distance from home, using a scale from 1 to 10, where 1 means ‘not important at all’ and 10 ‘most important’. This was followed by a question asking respondents to rate all the site quality conditions across all 8 beaches and state their perceived baseline quality levels for each attribute at each beach. This question aimed to get respondents starting to think about beach quality characteristics more generally before asking them to make trade-offs between these characteristics in the choice experiment. The third section contained the choice experiment, discussed in more detail below, and in the fourth and final section we collected socio-economic background information about the respondents and their households.
SP data were collected in the third section using two separate versions of the DCE: SP1 and SP2. Both versions include the eight beaches located along the Karachi coast as labelled alternatives (see ). These labelled DCEs allow modelling site choice behaviour of visitors, who are asked to choose their preferred beach out of a choice set of eight alternative beaches and an opt-out alternative (see Annex 1 for the full details of the design). All beaches are subject to environmental quality improvements in site attributes. Visitors as beneficiaries of these site improvements are confronted with two payments vehicles in the two DCE versions. In SP1 visitors are faced with a future increase in travel cost, i.e. a future increase in fuel expenditures and opportunity cost of travel time. In SP2 visitors face the same increase in future travel costs, but this version also includes an entrance fee as an explicit site access cost. Both DCEs are identical in their non-monetary site attributes, i.e. coastal water quality, beach cleanliness, crowding and site facilities, and differ only in terms of the inclusion of an entrance fee (see ).
Table 1. Site selection choice experiment design: versions, attributes and levels.
Three levels were selected for each site attribute, reflecting and depicting low, moderate and high site quality conditions. Due to the absence of coastal water regulation in Pakistan, we broadly defined poor, moderate and good levels for coastal water quality along the beaches in Karachi, similar to the categorisation of water bodies in the European Water Framework Directive (WFD), and illustrated using the US-EPA water quality ladder (Carson and Mitchell Citation1993). These water quality levels describe whether water is suitable for swimming, catching fish that are safe to eat and supporting plants, fish and other aquatic life. During the focus groups and pre-tests, it was observed that representing water quality levels based on objective physical indicators (e.g. turbidity, dissolved oxygen, etc.) was not easily understood by the general public. Hence, following Schaafsma and Brouwer (Citation2013) we developed a colour-coded water quality ladder, embodying water clarity, colour, contamination, odour, chemical status as well as its suitability for recreational uses such as swimming, bathing and wading in the water, and illustrate these levels using easy understandable pictograms (full descriptions of the attribute levels as presented to the survey participants are presented in Annex 1). The colour-coding and pictograms were applied to avoid statistical insignificance of site attributes due to lack of public understanding, as mentioned in Hanley, Wright, and Alvarez-Farizo (Citation2006), and at the same time facilitate comparison of elicited WTP values in this study with WTP values found elsewhere in the literature using similar water quality ladders (e.g. Bateman et al., Citation2011).
Likewise, we define three levels (very littered, moderately littered and a clean beach with no litter) for the attribute beach cleanliness by adopting an approach first implemented by Smith, Zhang, and Palmquist (Citation1997) to estimate the non-market benefits of reduced beach littering. Due to the absence of legislation related to coastal litter control in Pakistan, we adopt the definition applied in the EU Marine Strategy Framework Directive (MSFD) for reductions in coastal or marine litter, specifically in terms of visibility (e.g. 25 units of litter per 50 meters of beach length). However, during the focus groups and pre-tests, it was observed that the general public found it easier to assess the impacts of beach littering in terms of human health hazards and beach suitability for recreational uses such as walking, playing beach sports, playing in the sand and other recreational activities.
The attribute crowding or congestion has limited application in the DCE literature compared to RP-based site choice models (e.g. Hanley, Wright, and Koop Citation2002; Taylor and Longo Citation2010). When valuing recreational site quality improvements, crowding often is an important attribute (Boxall, Rollins, and Englin Citation2003) in view of the fact that an increase in the number of visitors may negatively affect the recreational experience of visitors in terms of reduced movement, privacy and noise (Bakhtiari, Jacobsen, and Jensen Citation2014). However, crowding may affect users’ experiences also positively (Schuhmann and Schwabe Citation2004; Taylor and Longo Citation2010). Crowding is mostly incorporated in recreational site choice models as a dummy variable (e.g. Lee, Hosking, and Du Preez Citation2015; Hanley, Wright, and Koop Citation2002). Here, we treat it instead as a categorical variable to test for possible non-linear effects (e.g. Mackenzie Citation1993; Boxall, Rollins, and Englin Citation2003; Taylor and Longo Citation2010), and added a feature of noise to it too (Brouwer Citation1999). Due to these considerations, three levels were selected (sparsely crowded, moderately crowded and very crowded). Pictograms were again used to visualize crowding, measured as beach visitors’ density.
Site facilities were chosen as the fourth non-monetary site attribute based on feedback from focus groups and pre-tests. The levels for this attribute are cumulative, starting with parking, then parking and food stores, and finally parking, food stores and washrooms.
Finally, for calculating marginal WTP values, which exhibit the rates at which beach visitors are willing to trade off site attribute levels with income (Louviere, Hensher, and Swait Citation2000), we require a monetary attribute. The first version of the DCE only includes future increases in travel costs as an implicit payment vehicle (SP1), while the second version includes an additional monetary entrance fee as an explicit payment vehicle (SP2). For recreation purposes, visitors usually travel from their homes to the chosen beach by implicitly bearing travel costs. To calculate these travel costs, potential beach visitors were asked to state the estimated distance and travel time to each of the eight selected beaches along the Karachi coast. We subsequently used these self-reported distances to each beach to calculate fuel or fare costs per beach, and used the self-reported travel times to each beach to calculate the opportunity costs of time per beach (see also Hanley, Wright, and Koop Citation2002). Opportunity costs of time are calculated using the furthermore reported respondent-specific information on their daily wage rate and number of working hours. For further details of the travel costs calculation procedure, we refer to Annex 2.
A monetary entrance fee is used as an explicit payment vehicle in SP2. This means that potential future beach visitors will bear an increase in travel costs and an additional entrance fee. In Karachi the public pays entrance fees ranging from PKR 20 to PKR 100 when visiting recreational attractions such as the zoo, parks and museums. Currently, the public enjoys free access to all the beaches in Karachi. During the focus group interviews, we asked participants about the range of entrance fees they were typically charged to access the public and private recreational attractions mentioned above, and which range they thought would be considered comparable for introducing a beach entrance fee. Based on this discussion, the entrance fees were set to PKR 30, PKR 70 and PKR 120, where PKR 100–120 was generally considered the range containing the choke prices.Footnote2
Pictograms were used to visualize all the choice attributes in the DCEs. An example of a choice card is presented in . Each respondent was presented with six choice cards and asked to choose his or her preferred beach to visit in the next 12 months under different site conditions from the set of eight alternative beaches, or to choose the opt-out alternative, labelled as ‘None of these, I prefer to stay at home or do other non-beach activities’.
We used orthogonal designs for two separate pilot samples for the two versions of the DCE in order to generate prior parameter values (N = 48 for both the SP1 pilot and the SP2 pilot). We estimated separate conditional logit models based on the two pilot datasets. For both models all parameters had the expected signs (e.g. a negative sign for distance from a respondent's home) and orders of magnitudes (e.g. higher levels being valued more than lower levels), and were statistically significant at the 1% and 5% levels, except for the moderately crowded coefficient, which was insignificant in both DCE pilot versions. Based on these prior values, two separate D-efficient designs of 36 choice tasks were generated, using the Ngene software (version 1.1.1; Choice Metrics Citation2014). In order to reduce the respondents’ cognitive burden during the interview, these 36 choice tasks were distributed across six versions of six choice tasks each. Each respondent was randomly assigned one of the six versions.
In-person interviews were conducted with off-site Karachi residents based on a random cluster sampling approach. The off-site residential survey was preferred to an on-site survey to ensure high response rates from both (i) visitors and non-visitors to avoid endogenous stratification (Mangan et al. Citation2013), and (ii) men and women. Hence, only Karachi residents were interviewed, not non-residents, who might visit the beaches only occasionally from nearby cities. The survey was implemented by selecting different clusters of municipal neighbourhoods in Karachi city. Within each cluster, residential homes were selected for in-person interviews through a simple random sampling approach. Ultimately, we obtained completed surveys from 152 respondents for SP1 and 156 respondents for SP2. Sample characteristics for the two samples are presented in . The sample composition is very similar on most accounts, except for marital status and education level, which appear to be higher in SP1. We control for these two variables in our modelling framework, along with the number of children in each household, to ensure that these differences in sample composition do not affect our test results and main findings.
Table 2. Socio-economic characteristics of beach visitors.
5. Modelling framework and hypotheses testing
The main objective of our study is to investigate the impact of including an explicit payment vehicle (entrance fee) in addition to an implicit payment vehicle (future increase in travel costs) on current and future beach visitors’ preferences, choice behaviour and WTP values. Choices are modelled here within a nowadays standard discrete choice modelling framework. Since we have collected data from randomly selected individual respondents, who are not further divided into particular segments, we apply a mixed logit (ML) model to capture unobserved preference heterogeneity through random variations in preference parameters across the sampled individuals (Hensher, Rose, and Greene Citation2005). The ML model also relaxes the independence of irrelevant alternatives (IIA) property and accounts for potential correlation between repeated choices for each individual (Train Citation2003).
Assuming that beach visitor i makes a single-day trip to beach alternative j out k = 1…K beach alternatives along the Karachi coast on a given day, his/her indirect utility U can be expressed as a linear function of the vector of beach characteristics (or attributes) X:(1) where
is the deterministic component of the indirect utility function, and where
represents the error term which is assumed to follow an independently identically distributed (IID) Gumbel extreme value type 1 distribution. The deterministic component comprises a set of alternative-specific constants
, the vector of site attributes
, and the vector of associated preference parameters
.
To correct for preference heterogeneity across respondents we allow the vector of preference parameters to vary over individuals by specifying a mixing distribution f(β)dβ. Train (Citation2003) shows that the preference parameters can be estimated in closed form through simulation, maximizing the simulated log-likelihood function, for which R draws are taken for each individual parameter from the distribution of parameters
. Then, for each draw, the simulated logit probability is calculated. By doing so, the average of these simulated logit probabilities generates unbiased estimators of choice probability
as expressed in (Equation2
(2) ):
(2) Using expression (Equation3
(3) ), we estimate preference parameters in both the SP1 and SP2 versions. Using the monetary parameters, travel costs only as the implicit payment vehicle in SP1 and the travel costs and entrance fee in SP2, we can estimate marginal WTP values. We calculate WTP standard errors and 95% confidence intervals applying the Krinsky and Robb (Citation1986) bootstrapping method.
In this study, we test the following two hypotheses based on the choice model estimation procedure outlined above for the two split samples:
Hypothesis 1:Preferences for beach site attributes and the estimated choice models’ error variance change if an explicit payment vehicle is included in the DCE in addition to travel costs as an implicit payment vehicle.
In order to test the first hypothesis, we use the Swait-Louviere (Citation1993) test procedure. The Swait and Louviere (Citation1993) test is a two-step procedure, which tests the equality of preference and scale parameters in the SP1 and SP2 models. The first step involves testing whether the preference parameters for the two samples are equal and can be pooled, while allowing the scale parameters to vary between subsamples:(3) A search for the best pooled model fit is performed over a range of scale parameter values. To test
, the Likelihood Ratio (LR) test statistic is applied using this best fit model:
(4) If
is rejected, then
is also rejected. In this case, the confoundedness of preference and scale parameters prevents concluding whether the observed differences are due to (a) differences in both scale and preference parameters or (b) differences in preference parameters only. If
cannot be rejected, the equality of scale parameters is tested in a second step:
(5) This second test is only meaningful if the preference parameters are equal between the analysed choice sets. Again the LR test statistic is applied, this time comparing the pooled models that assume equal and varying scale parameters for the two choice models:
(6) Hypothesis 2: WTP for improvements in non-monetary beach site attributes increase when including an additional explicit payment vehicle.
This hypothesis stems from the notion that an implicit payment vehicle likely does not cover individual maximum WTP, potentially leading to an underestimation of overall WTP. By design, including an explicit payment is expected to better approach maximum WTP at the individual level, or at least to get closer to it than when using travel costs only. Therefore including an explicit payment vehicle generally should increase WTP estimates and remove or at least mitigate the underestimation of overall WTP. In order to test hypothesis 2, we apply the Poe, Giraud, and Loomis (Citation2005) combinatorial test to assess differences in mean WTP estimates, and use the Mann-Whitney test to assess differences in WTP distributions.
Finally, with respect to the model specification, we accommodate possible non-linear threshold effects and avoid confounding effects (Louviere, Hensher, and Swait Citation2000) by applying effects coding instead of dummy coding.Footnote3 Parameters representing non-monetary site attributes are assumed to be normally distributed, while the two monetary choice attributes are fixed. Different mixing distributions were tested and the ML models with normally distributed non-monetary parameters and fixed monetary parameters were found to perform best in terms of model fit based on the pseudo R-squared and Bayesian Information Criterion (BIC).Footnote4 The models were estimated using 350 Halton draws in NLOGIT version 5.0. We estimate two separate ML models for the two samples. These two models are identical in terms of their non-monetary parameters, but differ in the monetary parameters relating to the implicit and explicit payment vehicles. The SP1 model includes the implicit future travel cost increase as its sole monetary parameter, while the SP2 model combines the explicit entrance fee with the implicit future travel cost increase to form a single monetary parameter. In order to ensure that differences in sample composition do not affect our findings, we interact marital status, education and number of children in the household with the status quo constant. This way, overall preferences are corrected for their potential effects, but beach quality attribute parameters still reflect mean beach quality preferences.
6. Results
6.1 Estimation results
ML estimation results are presented in . Significant unobserved preference heterogeneity is found at the 1% significance level for almost all choice attributes across all three models, justifying the use of the ML model. Significant observed heterogeneity across individual respondents is furthermore picked up through the inclusion of a limited number of visitor socio-demographic characteristics: higher educated respondents and respondents with more children in their household are less likely to be in favor of one of the hypothetical choice alternatives and pay for beach quality improvements, while married men are more likely to choose one of the beach alternatives and pay for specific beach quality improvements. The LR test furthermore shows that the changes in Log-Likelihood between the two models are statistically significant, with the SP2 model performing better than SP1, which makes sense since SP2 includes an additional attribute and parameter.
Table 3. Estimated mixed logit (ML) choice models.
The alternative-specific constants (ASC) capture respondent preferences for the labelled beach sites, which are not picked up by the choice attributes. The positive signs indicate that paying extra to visit one of the 8 beaches in the coming year is, ceteris paribus, preferred to the opt-out baseline alternative, i.e. irrespective of public preferences for the site quality improvements picked up by the choice attributes. Approximately half of the sites are statistically significant at the 5% level, i.e. those beach sites that are located more to the east of the city. French beach seems to be a cut-off point at the 5% level. The beaches located further west to this beach are just still significant at the 10% level in both models. Hence, there seems spatial clustering of preferred beaches to the south-east of the city.
All choice attribute coefficient estimates are statistically significant at the 1% and 5% level and have the expected signs and magnitudes reflecting sensitivity to scope, except the ‘sparsely crowded’ parameter in SP1 and the coefficients for the highest level of beach facilities in the SP2 model. All the non-monetary coefficient estimates have positive signs and exhibit higher marginal values for higher site quality improvements, while both the implicit future travel cost increase and the explicit entrance fee have the expected statistically significant negative signs. In the SP2 model the inclusion of the explicit entrance fee results in the highest level for beach facilities to become statistically insignificant (compared to the control group SP1), while sparsely crowded beaches become statistically significant. A similar effect is found for the random coefficient for the moderate crowding level, which also becomes statistically significant in the SP2 model. Moreover, the beach visitors’ characteristics, including education, married male and the number of children in the travelling group, are significant, with a negative sign for education. And this is true because during the field observations and surveys, we intensively noticed that the educated visitors travel to theses beaches less frequently.
6.2 Hypothesis testing
To test our first hypothesis, i.e. the equivalence of beach visitors’ preferences in DCE's with and without an explicit payment vehicle, the Swait and Louviere (Citation1993) test procedure is applied to the SP1 and SP2 models, which have an identical number of parameters. The LR test statistic is 83.45. With 21 degrees of freedom, the critical value of the chi-square distribution is 32.67 at the 5% significance level. Thus, our first hypothesis that preference parameters remain unchanged after including an explicit payment vehicle is rejected. This suggests that public preferences for beach quality improvements are significantly affected when an explicit payment vehicle is included in the DCE in addition to the expected increase in future travel costs. Due to the fact that equality of preference parameters is rejected in the first stage of the Swait-Louviere test procedure, the outcome of the LR test in the second stage testing equality of scale parameters is meaningless.
To test our second hypothesis regarding the increase in marginal WTP values for site quality improvements, we apply the non-parametric Poe, Giraud, and Loomis (Citation2005) combinatorial test, comparing the marginal WTP values derived from the SP1 and SP2 models. We furthermore estimate standard errors associated with these WTP values using the Krinsky and Robb (Citation1986) bootstrapping method, using 1000 random draws. Results are presented in . An important first observation is that public WTP is highest for an increase of beach water quality to the highest level for both models, followed by a reduction in beach litter. In SP1, reducing overcrowding is valued least, followed by improving beach facilities, while the latter is valued least in SP2. Secondly, inclusion of an explicit entrance fee in SP2 increases the marginal WTP for water quality, litter and crowding, but decreases marginal WTP for beach facilities. This is confirmed by the non-parametric combinatorial Poe test: marginal WTP estimates for water quality, litter and crowding are significantly higher for SP2 at 5%, while marginal WTP for the high beach facilities level is significantly lower for SP2 at 10% (the difference in WTP for medium facilities between SP1 and SP2 is not significant).
Table 4. Marginal WTP (in PKR per person per beach trip) and combinatorial test results.
The Poe test only addresses marginal WTP point estimates, and do not provide insight into the WTP distributions. Individual-specific WTP values are derived from our ML models, and WTP distributions for SP1 and SP2 are plotted in using Kernel density plots.
Figure 3. Kernel density plots of marginal WTP values (in PKR per person per beach trip) from SP1 and SP2 mixed logit models.
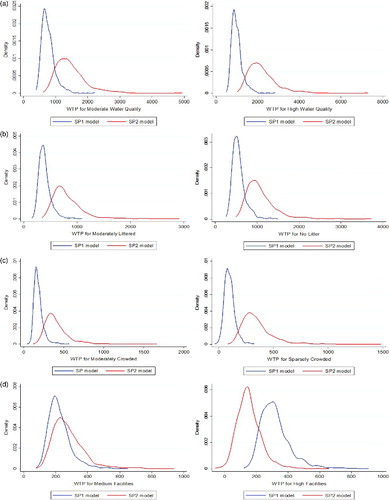
The figure confirms our findings for the WTP point estimates, but also shows that the WTP distributions for SP1 and SP2 are distinctly different. More specifically, the WTP distributions are much wider for SP2 for all attributes except medium level facilities, confirming our expectation that the explicit payment vehicle increase marginal WTP.
We perform non-parametric Mann-Whitney tests to test the differences in WTP distributions between SP1 and SP2 (). The results confirm that the differences in the median WTP values and their distributions are statistically significant for all beach attributes at the 1% significance level.
Table 5. Results from Mann-Whitney tests on differences in WTP distributions for beach attributes between SP1 and SP2.
7. Aggregate welfare effects of beach quality improvements
We calculated the number of visitors arriving at the beaches during peak hours on weekdays and weekends all year through. Using these annual visits, we estimated aggregated WTP values for improvements in water quality and cleanliness for all alternative beaches with reference to their current status water quality and cleanliness. We used a proposed status of 5 years as a short-term policy implementation aimed at achieving moderate water quality and moderate cleanliness improvements along Karachi coast, and we used a proposed status of 10 years as a long-term policy implementation aimed at attaining high water quality and clean beaches with no litter. We calculated aggregated welfare values for the SP1 and SP2 models, and for SP2 we also calculated the aggregate WTP that visitors pay in terms of entrance fee. For a sustainable beach resource management, this figure is important because it indicates potential revenues when entrance fees are charged dedicated to policies aimed at improving the quality of Karachi's beaches.
shows that, identical to marginal WTP, total aggregated values for SP2 are higher than those for SP1, because beach visitors are willing to pay an entrance fee in addition to paying for the travel costs when travelling to the selected beaches if water quality and beach cleanliness are improved. For a proposed status of 5 years, aggregated WTP values increase by around 87%, whereas for a proposed status of 10 years they increase by around 108%. These results may convince coastal resource managers in Pakistan to implement an entrance fee as an economic instrument for managing public beach access, and for improving beach quality attributes such as water quality and beach cleanliness.
Table 6. Aggregated WTP values for improvements in water quality and cleanliness.
8. Conclusions and discussion
In this paper we investigate convergent validity of beach visitors’ preferences and WTP values obtained from different versions of beach site selection choice experiments. The study was carried out on the beaches in Karachi, the largest and most populated city in Pakistan, along the Arabian Sea. Using a split-sample approach, we implemented two choice experiments, one with implicit travel costs as the payment vehicle and one with both travel costs and an explicit entrance fee as payment vehicles. Because implicit travel costs likely do not elicit maximum WTP, and by design an explicit payment vehicle does or at least gets closer it, we hypothesized that WTP increases when an explicit payment vehicle is included. We estimate separate choice models for both survey versions, and apply the Swait and Louviere (Citation1993) procedure, which rejects equality of preference parameters for the two models. This confirms our hypothesis of changing preferences and is in line with findings by Carlsson, Frykblom, and Lagerkvist (Citation2007); Aravena, Peter, and Scarpa (Citation2014) and Van Zanten, Koetse and Verburg (Citation2016). WTP point estimates are larger for the model with entrance fees for almost all choice attributes, and Poe, Giraud, and Loomis (Citation2005) combinatorial tests show that the differences between the estimated WTP values are statistically significant. We also compare WTP distributions using Kernel density plots and find that also the variance of the distributions change. Mann-Whitney tests further confirm that the differences in the mean WTP values and their distributions are statistically significant for all beach attributes. These results confirm our hypothesis that welfare estimates increase when including an explicit payment vehicle, which is in line with findings by Campos, Caparro's, and Oviedo (Citation2007) and in Oviedo et al. (Citation2016). This suggests that studies that use implicit payment vehicles, such as some choice experiment studies, underestimate welfare changes, especially those related to recreation. Adding an explicit payment vehicle to such studies can be used to remedy these effects. We therefore recommend adding stated choices to travel cost elicitation studies in order to combine the strengths of each method, whilst keeping the option open of taking a revealed preference approach to welfare.
Finally, in Pakistan, a very limited number of environmental valuation studies have been conducted so far to investigate the acceptability of entrance fees to access recreational resources, such as public parks and freshwater lakes. Using a travel cost model, Mangan et al. (Citation2013) estimated the recreational value of Keenjhar Lake, Pakistan's largest freshwater lake, in Sindh province, and find an average entrance fee of PKR 25 paid by each visitor. Khan (Citation2011) also used the travel cost method to estimate the recreational value of two public parks located in the North of Pakistan, and recommend that public park management authorities could charge entrance fees per visitor ranging between PKR 20 and PKR 87. This is substantially lower than the stated preference values found in this study. However, so far no studies have addressed the acceptability of entrance fee to access beaches. Our findings therefore add to the limited body of literature in Pakistan by showing that beach visitors in Karachi are willing to pay an entrance fee in addition to their travel costs when visiting one of the city beaches if water and beach quality are improved. These findings are also shown in terms of aggregated values, which may convince coastal policy makers in Pakistan that it is worth charging entrance fees at beaches in order to manage them sustainably based on the beneficiary pays principle, and earmark the funds for improving the quality of beaches in terms of water quality and beach cleanliness.
Acknowledgments
We are grateful to the University of Sindh, Jamshoro, and the Higher Education Commission (HEC), Islamabad, Pakistan, for their financial support. The authors are also thankful to Dr. Heman Das Lohano from the Institute of Business Administration (IBA), Karachi, Pakistan for his helpful discussions and comments, especially with regards to the effects coding used in our models. As always, the usual disclaimer applies.
Disclosure statement
No potential conflict of interest was reported by the authors.
Additional information
Funding
Notes
1. In view of the fact that our DCE design includes the beach locations as labelled alternatives, including a travel cost increase as a monetary attribute with varying levels, as Oviedo et al. (Citation2016) do in their study, is not possible due to the fixed distance from a respondent's home to the locations of alternative beach sites in our choice set.
2. In 2014 when the survey was conducted, 1 Euro was equal to approximately 132.32 Pakistani Rupee (PKR).
3. Because the sum of the effects codes is equal to 0, the sum of the attribute with three levels is also equal to zero, that is β0 + β1 + β2 = 0, which can also be expressed as β0 = - β1 - β2. For calculating WTP for the medium and the higher site quality improvements, we applied the following formulas using the above definition of effects coding: WTP medium = (β1 - β0 )/βpayment vehicle = (β1 - ( - β1 - β2))/ βpayment vehicle = (2*β1 + β2))/βpayment vehicle and WTP high = (β2 - β0 )/ βpayment vehicle = (β2 - ( - β1 - β2))/ βpayment vehicle = (2*β2 + β1))/ βpayment vehicle.
4. The ML model results using alternative distributional assumptions are available from the author upon request.
References
- Aravena, C., M. Peter, and R. Scarpa. 2014. “Does Money Talk? The Effect of Monetary Attribute on the Marginal Values in a Choice Experiment.” Energy Economics 44: 483–491.
- Adamowicz, W., J. Louviere, and M. Williams. 1994. “Combining Revealed and Stated Preference Methods for Valuing Environmental Amenities.” Journal of Environmental Economics and Management 26: 271–292.
- Adamowicz, W., J. Swait, P. Boxall, J. Louviere, and M. Williams. 1997. “Perceptions versus Objective Measures in Combined Revealed and Stated Preference Models of Environmental Valuation.” Journal of Environmental Economics and Management 32: 65–84.
- Bakhtiari, F., J.B. Jacobsen, and F.S. Jensen. 2014. “Willingness to Travel to Avoid Conflicts in Danish Forests.” Urban Forestry and Urban Greening 13: 662–671.
- Bateman, I.J., R. Brouwer, S. Ferrini, M. Schaafsma, D. N. Barton, A. Dubgaard, B. Hasler, et al. 2011. “Making Benefit Transfers Work: Deriving and Testing Principles for Value Transfers for Similar and Dissimilar Sites Using a Case Study of the Non-Market Benefits of Water Quality Improvements across Europe.” Environmental and Resource Economics 50: 365–387.
- Blakemore, F., and A. Williams. 2008. “British Tourists’ Valuation of a Turkish Beach Using Contingent Valuation and Travel Cost Methods.” Journal of Coastal Research 24: 1469–1480.
- Boxall, P., K. Rollins, and J. Englin. 2003. “Heterogeneous Preferences for Congestion During a Wilderness Experience.” Resource and Energy Economics 25: 177–195.
- Brouwer, R., D. Hadzhiyska, C. Loakeimidis, and H. Ouderdorp. 2017. “The Social Cost of Marine Litter Along European Coasts.” Ocean and Coastal Management 138: 38–49.
- Brouwer, R. 1999. “Public Right of Access, Overcrowding and the Value of Peace and Quiet: The Validity of Contingent Valuation as an Information Tool.” CSERGE Working Paper GEC 99-05, University of East Anglia, UK.
- Campos, P., A. Caparro's, and J.L. Oviedo. 2007. “Comparing Payment-Vehicle Effects in Contingent Valuation Studies for Recreational Use in Two Spanish Protected Forests.” Journal of Leisure Research 39: 60–85.
- Carlsson, F., P. Frykblom, and C.J. Lagerkvist. 2007. “Preferences with and Without Prices – Does the Price Affect Behaviour in Stated Preference Surveys.” Journal of Environmental and Resource Economics 38: 155–164.
- Carson, R.T., and R.C. Mitchell. 1993. “The Value of Clean Water: The Public's Willingness to Pay for Boatable, Fishable, and Swimmable Quality Water.” Water Resources Research 29: 2445–2454.
- Christie, M., N. Hanley, and S. Hynes. 2007. “Valuing Enhancements to Forest Recreation Using Choice Experiment and Contingent Behaviour Methods.” Journal of Forest Economics 13: 75–102.
- Choice Metrics. 2014. “Ngene Software.” www.choice-metrics.com
- Eggert, H. and B. Olsson 2004. “Heterogeneous Preferences for Marine Amenities: A Choice Experiment Applied to Water Quality.” Working Paper, Department of Economics, Göteborg University, Sweden
- FBR: Federal Board of Revenue. 2006-07. Year Book of Federal Board of Revenue. Revenue Division. Islamabad: Government of Pakistan.
- Ferrini, S., M. Schaafsma, and I.J. Bateman. 2014. “Revealed and Stated Preference Valuation and Ttransfer: A Within-Sample Comparison of Water Quality Improvement Values.” Water Resources Research 50: 4746–4759.
- Gibson, J.M., D. Rigby, D.A. Polya, and N. Russell. 2016. “Discrete Choice Experiments in Developing Countries: Willingness to Pay Versus Willingness to Work.” Environmental and Resource Economics 65: 697–721.
- Hanley, N., R. Wright, and G. Koop. 2002. “Modelling Recreation Demand Using Choice Experiments: Climbing in Scotland.” Journal of Environmental and Resource Economics 22: 449–466.
- Hanley, N., R.E. Wright, and B. Alvarez-Farizo. 2006. “Estimating the Economic Value of Improvements in River Ecology Using Choice Experiments: An Application to the Water Framework Directive.” Journal of Environmental Management 78: 183–193.
- Hensher, D.A., J.M. Rose, and W.H. Greene. 2005. Applied Choice Analysis: A Primer. Cambridge: Cambridge University Press.
- Hilger, J., and M. Hanemann. 2006. Heterogeneous Preferences for Water Quality: A Finite Mixture Model of Beach Recreation in Southern California. Berkeley, CA: University of California.
- Hynes, S., D. Tinch, and N. Hanley. 2013. “Valuing Improvements to Coastal Waters Using Choice Experiments: An Application to Revisions of the EU Bathing Waters Directive.” Marine Policy 40: 137–144.
- Khalil, S. 1999. “Economic Valuation of the Mangrove Ecosystem Along the Karachi Coastal Areas.” In The Economic Value of the Environment: Cases from South Asia, edited by J.E. Hecht. Washington, DC: IUCN.
- Khan, H. 2011. “Valuation of Recreational Amenities from Environmental Resources: The Case of Two National Parks in Northern Pakistan.” In Environmental Valuation in South Asia, edited by A.K. Enamul Haque, M.N. Murty, and P. Shyamsundar. New York: Cambridge University Press.
- Koetse, M.J., and R. Brouwer. 2016. “Reference Dependence Effects on WTA and WTP Value Functions and Their Disparity.” Environmental and Resource Economics 65: 723–745.
- Krinsky, I., and A.L. Robb. 1986. “On Approximating the Statistical Properties of Elasticities.” Review of Economics and Statistics 68: 715–719.
- KSDP: Karachi Strategic Development Plan. 2007. Karachi Strategic Development Plan-2020. Karachi, Pakistan: Master Plan Group of Offices, City District Government.
- Lee, D.E., S.G. Hosking, and M. Du Preez. 2015. “Managing Some Motorised Recreational Boating Challenges in South African Estuaries: A Case Study at the Kromme River Estuary.” South African Journal of Economics 83: 286–302.
- Louviere, J., D. Hensher, and J. Swait. 2000. Stated Choice Methods: Analysis and Applications. Cambridge, UK: Cambridge University Press.
- Loomis, J., and L. Santiago. 2013. “Economic Valuation of Beach Quality Improvements: Comparing Incremental Attribute Values Estimated from Two Stated Preference Valuation Methods.” Coastal Management 41: 75–86.
- Mackenzie, J. 1993. “A Comparison of Contingent Preference Models.” American Journal of Agricultural Economics 75: 593–603.
- Mangan, T.M., R. Brouwer, H.D. Lohano, and G.M. Nangraj. 2013. “Estimating the Recreational Value of Pakistan's Largest Freshwater Lake to Support Sustainable Tourism Management Using a Travel Cost Model.” Journal of Sustainable Tourism 21: 473–486.
- Mansoor, A., and S. Mirza. 2007. “Waste Disposal and Stream Flow Quantity and Quality of Layari River.” Indus Journal Management and Social Sciences 1: 76–89.
- Oviedo, J.L., A. Caparrós, I. Ruiz-Gauna, and P. Campos. 2016. “Testing Convergent Validity in Choice Experiments: Application to Public Recreation in Spanish Stone Pine and Cork Oak Forests.” Journal of Forest Economics 25: 130–148.
- Pakistan Bureau of Statistics. 2015. Population Statistics 2013-14. Islamabad: Government of Pakistan. www.pbs.gov.pk
- Poe, G., K. Giraud, and J. Loomis. 2005. “Simple Computational Methods for Measuring the Difference of Empirical Distributions: Application to Internal and External Scope Tests in Contingent Valuation.” American Journal of Agricultural Economics 87: 353–365.
- Priyapada, S., and E. Wang. 2014. “Modeling Willingness to Pay for Coastal Tourism Resource Protection in Ko Chang Marine National Park, Thailand.” Asia Pacific Journal of Tourism Research 20: 515–540.
- Rai, R.K., and H. Scarborough. 2013. “Economic Value of Mitigation of Plant Invaders in a Subsistence Economy: Incorporating Labour as a Mode of Payment.” Environment and Development Economics 18: 225–244.
- SACEP. 2007. “Marine Litter in the South Asian Seas Region.” A Report, September-2007, South Asia Cooperative Environment Programme (SACEP), Colombo, Sri Lanka.
- Schaafsma, M., and R. Brouwer. 2013. “Testing Geographical Framing and Substitution Effects in Spatial Choice Experiments.” Journal of Choice Modelling 8: 32–48.
- Schuhmann, P.W., and K.A. Schwabe. 2004. “An Analysis of Congestion Measures and Heterogeneous Angler Preferences in a Random Utility Model of Recreational Fishing.” Environmental and Resource Economics 27: 429–450.
- Smith, V.K., X. Zhang, and R.B. Palmquist. 1997. “Marine Debris, Beach Quality and Non-Market Values.” Environmental and Resource Economics 10: 223–247.
- Swait, J., and J. Louviere. 1993. “The Role of Scale Parameter Estimation in the Estimation and Comparison of Multinomial Logit Models.” Journal of Marketing Research 30: 304–314.
- Talpur, M.A., and G.A. Jariko. 2001. “Role of Environmental Legislation and Administration in Protecting the Environment: The Experience of Pakistan.” Biannual Research Journal of Grassroots 23: 75–84.
- Taylor, T., and A. Longo. 2010. “Valuing Algal Bloom in the Black Sea Coast of Bulgaria: A Choice Experiments Approach.” Journal of Environmental Management 91: 1963–1971.
- Train, K. 2003. Discrete Choice Methods with Simulation. New York: Cambridge University Press.
- Van Zanten, B.T., M.J. Koetse, and P.H. Verburg. 2016. “Economic Valuation at All Cost? The Role of the Price Attribute in a Landscape Preference Study.” Ecosystem Services 22B: 289–296.
- Whitehead, J.C., D.J. Phaneuf, C.F. Dumas, J. Herstine, J. Hill, and B. Buerger. 2010. “Convergent Validity of Revealed and Stated Recreation Behavior with Quality Change: A Comparison of Multiple and Single Site Demands.” Environmental and Resource Economics 45: 91–112.
Annex 1. Detailed definitions and illustrations of attribute levels
Table
Annex 2. Calculation procedure of the future travel cost increase
Since distance acts as a proxy for travel cost (Hanley, Wright, and Koop Citation2002), we converted distance into travel cost (TC) using the standard formula:(A2.1) Travel costs are measured as the sum of a visitor's round distance (fuel) cost and opportunity cost of travelling time to a beach. As people in Pakistan customarily bear the fuel cost or transport fares for their family members and relatives when travelling with them, we measured distance costs as the two-way fuel costs borne by the head of the household and, where applicable, shared by the total number of adults in the travelling group.
Given the frequency of price increases in Pakistan, it is very easy for actual and potential beach visitors to think of a price increase when visiting one of the 8 beaches in the near future. In view of this, we adjusted the distance-based fuel costs and hence increase in travel expenditures as implicit payment vehicle in the DCE by multiplying them with the expected annual average fuel-price increase in Pakistan. Fuel prices in Pakistan increase on average almost 4 times a year and fuel price data are ready available on the web-site of the Pakistan Bureau of Statistics (Citation2015). Over the last 5 years, the fuel price increased on average by 7.6 percent per month. In this way, we convert a visitor's current trip cost into a future trip expenditure increase.
The opportunity cost of time (OCT) is calculated as the foregone monetary benefits of a person spending time on something else than work, and is generally fixed at 30 percent of a person's annual income, as is common practice in the travel cost literature (e.g. Mangan et al. Citation2013). However, because working hours differ widely in both the private and public sector in Pakistan due to the lack of labour law enforcement, we included an estimate of each visitor's varying working hours (if employed) instead of a fixed number of working hours (e.g. 40 h per week) and multiplied these varying working hours with 52 weeks in order to arrive at a visitor's total annual working hours. Thus, the opportunity cost of travelling time is calculated as:(A2.2) where MI is a visitor's monthly income, EM indicates whether a visitor is employed or not, RTT is the round-trip travel time and WHrs is the varying annual number of working hours. EM equals 1 if a visitor is employed and 0 if he is unemployed. In the latter case OCT is zero (Adamowicz et al. Citation1997; Mangan et al. Citation2013) and the travel cost only depends on the distance-based transportation costs. RTT is calculated by dividing each respondent's perceived travel distance by an average travelling speed of 60 km/hour based on own observations whilst travelling to the different beaches during the survey.
The current opportunity cost of time in equation A2.2 is converted into its future (i.e. next year) value using a discount rate of 9.5 percent. In this way, we calculate the future travel cost increase as the sum of the increase in future fuel expenditures and future opportunity cost of travel time.