ABSTRACT
Insulin resistance is caused by the abnormal secretion of proinflammatory cytokines in adipose tissue, which is induced by an increase in lipid accumulation in adipocytes, hepatocytes, and myocytes. The inflammatory pathway involves multiple targets such as nuclear factor kappa B, inhibitor of nuclear factor κ-B kinase, and mitogen-activated protein kinase. Vitamins are micronutrients with anti-inflammatory activities that have unclear mechanisms. The present study aimed to describe the putative mechanisms of vitamins involved in the inflammatory pathway of insulin resistance. The strategy to achieve this goal was to integrate data mining and analysis, target prediction, and molecular docking simulation calculations to support our hypotheses. Our results suggest that the multitarget activity of vitamins A, B1, B2, B3, B5, B6, B7, B12, C, D3, and E inhibits nuclear factor kappa B and mitogen-activated protein kinase, in addition to vitamins A and B12 against inhibitor of nuclear factor κ-B kinase. The findings of this study highlight the pharmacological potential of using an anti-inflammatory and multitarget treatment based on vitamins and open new perspectives to evaluate the inhibitory activity of vitamins against nuclear factor kappa B, mitogen-activated protein kinase, and inhibitor of nuclear factor κ-B kinase in an insulin-resistant context.
Introduction
The obesogenic environment is a predisposing factor for the development of obesity, such as sedentary lifestyle, urbanization, rural-to-urban migration, hypercaloric food consumption, and physical inactivity. The final step of the interaction of these factors generates pathophysiological mechanism, such as insulin resistance (IR), which is commonly the starting point for the development of more complex diseases, such as type 2 diabetes mellitus, cardiovascular diseases, metabolic syndrome, and breast or colorectal cancer [Citation1]. Chronic consumption of a hypercaloric diet can lead to inflammation and lipid oxidation with excessive accumulation of lipids in adipocytes, myocytes, and hepatocytes [Citation2].
IR starts in adipose tissue with the phosphorylation of insulin receptors caused by the lipo-inflammation process, which is characterized by an increase in the size of adipocytes (excessive lipid accumulation) and metabolic dysfunction (proinflammatory cytokine secretion). Inflammatory pathways in adipocytes and macrophages begin with mitogen-activated protein kinase (MAPK), Junk kinase (JNK) and inhibitor of nuclear factor κ-B kinase (IKK) phosphorylation, followed by nuclear factor kappa B (NF-κB) activation and translocation into the nucleus [Citation3]. Then, proinflammatory cytokines such as tumour necrosis factor-α (TNF-α), interleukin-6 (IL-6), and interleukin-1β (IL-1β) are produced by adipocytes and stimulate changes in the M2 to M1 phenotype of macrophages. Macrophages M1 are characterized by the secretion of proinflammatory cytokines [Citation4,Citation5].
Several alternative treatments have been proposed to increase insulin sensitivity and decrease inflammation and oxidative stress levels in obesity models. For example, natural products are promising sources for the development of novel preventive and curative treatments [Citation6–8]. Moreover, vitamins have demonstrated the potential to reduce inflammation and oxidative stress. For example, vitamins A, B1, C, and E have antioxidant activity, and vitamin E can re-establish enzyme glutathione function [Citation9,Citation10]. Vitamins B1 [Citation11], B2 [Citation12], B3 [Citation13], B5 [Citation14], B6, B7, B12 [Citation15], C [Citation16], D3 [Citation17], and E [Citation18] have been shown to have anti-inflammatory activity. shows the structure of exemplary vitamins with anti-insulin resistance activity that have been associated with antioxidant or anti-inflammatory activities, mediated by a decrease in proinflammatory cytokines. However, the precise mechanism of action (MOA) is unclear.
Figure 1. Representative vitamins associated with anti-insulin resistance activity.
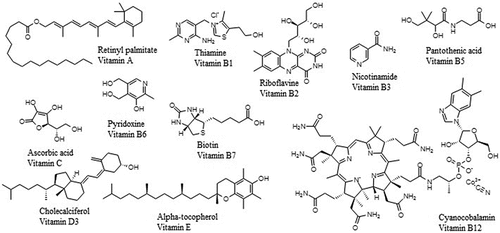
Vitamin B2 shows reduction of TNF-α and other proinflammatory cytokines associated of NF-κB inhibition [Citation12], in the same way, vitamin C administered with vitamin B5 decreased C-reactive protein serum levels [Citation14]. Vitamin C also decreases TNF-α serum levels due to its inhibitory activity on TNF-α mRNA transcription [Citation16], and vitamin E suppresses proinflammatory signalling such as NF-κB and transcription factor STAT3/6 (STAT3/6) [Citation19]. Finally, vitamin D and its receptor (VDR) regulate inflammation by upregulating of MAPK and inhibiting the NF-κB signalling pathway [Citation20]. Therefore, more studies are needed to elucidate the specific MOA of vitamins in the inflammatory pathway. Thus, these molecules represent promising candidates for the treatment or prevention of IR, especially if it is administered a multivitamin (i.e., based on a polypharmacy approach or multitarget therapy).
In silico studies help to identify promising compounds and molecular targets that represent future solutions for different types of diseases [Citation21–23]. Target prediction using machine learning models, molecular docking calculations, and network pharmacology are examples of the current methodologies used to discover and develop novel drugs [Citation24]. Overall, the rational use of these and other computational techniques saves time, assesses cost-effective drugs, and reduces data gaps [Citation25–27]. Thus, computational methods are powerful for establishing a solid hypothesis for the MOA of the proposed vitamins (A, B1, B2, B3, B5, B6, B7, B12, C, D3, and E) in the inflammatory pathway, which is an important component of IR. Similarly, vitamin D3 was evaluated for its inhibitory activity on mitogen-activated protein kinase kinase 1 (MAPK1) and MAP2K1 by GO and KEGG enrichment analyses. Suggesting that vitamin D3 plays a key role in the prevention of colorectal cancer (CRC) through core targets, the phosphatidylinositol 3-kinase- protein kinase B (PI3K-AKT), hypoxia-inducible factor 1 (HIF-1), and forkhead box protein (FoxO) pathways [Citation28]. In addition, vitamin D3 plays an important role in the inhibition of the NF-κB pathway; therefore, it has been proposed as a treatment for rheumatoid arthritis [Citation29].
The main goal of this work was to describe the putative mechanisms of 11 vitamins with anti-inflammatory activity, inhibiting pathways such as nuclear factor kappa B (NF-κB), inhibitor of nuclear factor κ-B kinase (IKK), and mitogen-activated protein kinase (MAPK), involved in the prevention or treatment of IR. Using bibliographic data analysis and in silico methods such as target prediction and molecular docking calculations. In silico methods used in this study were validated using both positive and negative controls.
Results
Using a knowledge-based drug design approach, we explored in silico the putative affinity of vitamins A, B1, B2, B3, B5, B6, B7, B12, C, D3, and E with targets involved in the anti-inflammatory potential of the nuclear factor kappa B (NF-κB) pathway. illustrates the general protocol used. In vitro and in vivo data supported the computational predictions.
Figure 2. General workflow used in this work.
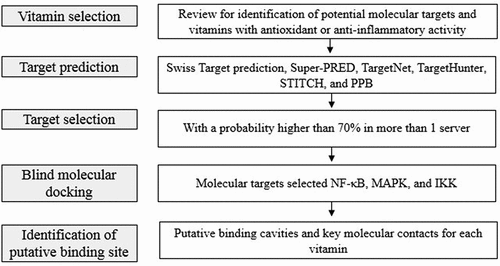
Target prediction
Target prediction is a commonly used technique in drug discovery [Citation30]. The most common target prediction servers allow the identification of potential targets of small molecules based on their structural and/or interactome similarity with each target inhibitor contained in the target prediction servers databases. In this study, we used target prediction servers based on similarities and interactome similarities to identify potential targets for each studied vitamin.
and Table S1 in the Supporting Information summarize the interactions of vitamins A, B1, B2, B3, B5, B6, B7, B12, C, D3, and E with NF-κB, MAPK, and IKK using seven target predictor servers (Swiss Target prediction [Citation31], Super-PRED [Citation32], TargetNet, TargetHunter [Citation33], STITCH [Citation34] and PPB [Citation35]). We selected the prediction with a higher than 70% confidentiality and selected for a deep study of the targets predicted in almost two different servers. The benchmark was selected according to the report by Gallo [Citation36], which remarks on the structural diversity of inhibitors of different kinds of targets. Interestingly, approximately 10% of the consensus predictions have been documented in previous studies (), which indicates the utility of these servers.
Table 1. Vitamins target prediction using different servers.
Molecular docking
Molecular docking has been used for drug discovery and optimization [Citation50]. Docking helps to identify putative binding sites, potential inhibitors, and/or identifying new molecular targets, including targets related to IR like inflammatory pathway (NF-κB, MAPK, and IKK) that lead to the proinflammatory cytokine’s translation, and oxidative stress pathway, involved in the phosphorylation of ISR [Citation51,Citation52]. In this case, we used a blind docking approximation to identify the putative binding site (and hypothetical affinity) of each vitamin against targets related to the NF-KB pathway (). We used positive controls for NF-κB (i.e., oxidized hTRX), MAPK (i.e., SRC-SM1-71-R), and IKK (i.e., Cmpd1/2), and established the molecular docking conditions to reproduce their crystallographic reports with an RMSD value lower than 2 Angtroms (that is, the traditional protocol to validate blind docking protocols). The results of that study established a robust pharmacological hypothesis of protein-vitamin interactions associated with their polypharmacological activity or selectivity against targets related to IR, as illustrated in . The polypharmacological activity and selectivity against targets related to IR are illustrated in .
Figure 3. Putative ligand efficiency of vitamins against selected targets related to IR.
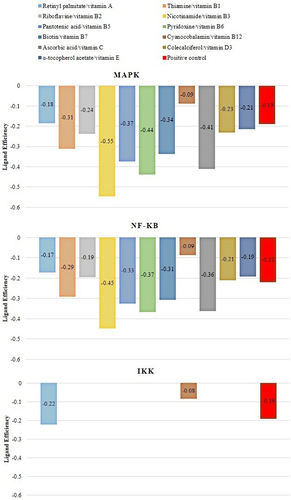
Figure 4. Putative binding sites and molecular interaction predicted by blind molecular docking of vitamins against MAPK.
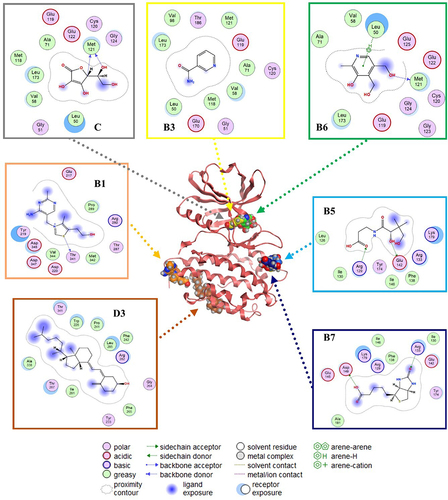
Figure 5. Prediction of binding sites and molecular interactions between vitamins and NF-kB using blind molecular docking.
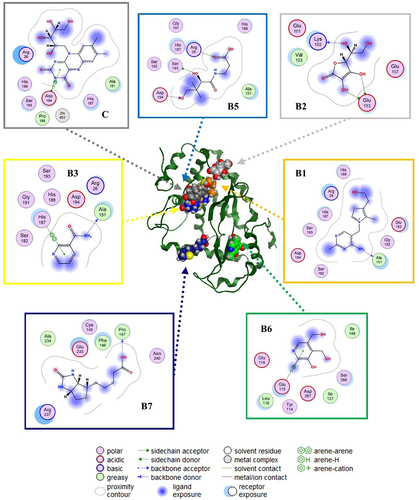
In this study, we employed molecular docking calculations to provide insights into the putative affinity of vitamins (A, B1, B2, B3, B5, B6, B7, B12, C, D3, and E for), MAPK and IKK at the structural level.
The most representative binding positions of vitamins B1, B3, B5, B6, B7, C, and D3 against MAPK are illustrated in , which shows the putative binding cavities and key molecular contacts. For example, as mentioned previously, vitamins C and D3 can inhibit MAPK [Citation53,Citation54]. Interestingly, the molecular docking results suggest that vitamins B3 and B6 could interact in a similar binding site to vitamin C with similar affinity (), highlighting the importance of key interactions with LEU 50 and MET 121, which form part of the protein kinase domain. Vitamin B1 shows a binding site similar to that of vitamin D3, highlighting the importance of THR 341, which forms part of the p38 mitogen-activated protein kinase (p38MAPK)-binding site [Citation55]. However, most of the vitamin B1 and D3 structures do not directly interact with MAPK (solvent ligand exposure), suggesting that their MOA could be generated by promoting allosteric changes in MAPK. In the case of vitamins B5 and B7, their putative binding mechanism could be completely different from that of vitamins C or D3, but in both cases, the positively charged amino acids (e.g., ARG, GLU, or ASP) could explain their affinities.
illustrates the predicted binding of the vitamins to NF-κB. As previously mentioned, vitamins B2, B5, and B6 can inhibit NF-κB [Citation15]. The key interactions observed between B2 and B5 against NF-κB highlight the importance of positively charged amino acids (e.g., ARG 26, LYS 102, GLU 153, and ARG 194) in their putative binding site, which forms part of the RHD domain involved in the molecular recognition of IKK [Citation56,Citation57]. Similar key interactions with B2 and B5 were predicted for vitamins B1, B3, and C. In contrast, blind molecular docking results suggested that vitamins B7 and B6 interact with different binding sites of the RHD domain in NF-κB.
Discussion
IR is an uncontrolled pandemic, principally associated with lifestyle. For example, unhealthy alimentary habits promote IR, which are associated with the development of type 2 diabetes mellitus, metabolic syndrome, and cardiovascular diseases. IR is generated initially by inflammation caused by lipid accumulation in the adipose tissue, liver, and muscle, inducing phosphorylation of insulin receptors, followed by insulin resistance. Previous studies have shown that vitamins can prevent IR due to their anti-inflammatory activity and decreased levels of TNF-α, IL-6, IL-1, and IL-1β in the serum [Citation10,Citation12,Citation14,Citation16,Citation19,Citation20]. In the present work, we used an in silico workflow () to formulate mechanistic hypotheses at the molecular level regarding the putative anti-inflammatory activity of vitamins against targets related to IR (e.g., NF-κB, MAPK, and IKK).
MAPK is the first step in the inflammatory pathway ending in NF-κB activation; this process has been implicated in chronic diseases, such as metabolic diseases [Citation58–60]. Therefore, we considered three important points: NF-κB, MAPK, and IKK. A previous study in vitro of vitamin C in HeLa cells showed its inhibitory effects on IKK [Citation61]. A similar effect has been reported for vitamin D [Citation62]. IKK is also inhibited by vitamin E [Citation19].
Interestingly, previous studies have reported the inhibitory activity of vitamins C and D against MAPK, and their indirect inhibition of NF-κB consequently stops the transcription of proinflammatory cytokines [Citation16,Citation20]. In addition, vitamins B2, B5, and B6 inhibit the p105 subunit (of NF-κB), thereby decreasing the levels of proinflammatory cytokines [Citation12,Citation14,Citation63]. These findings were consistent with our blind molecular docking results (). However, our results highlight the potential inhibitory activity of additional vitamins (e.g., B1, B3, B5, B6, and B7) against MAPK or (e.g., B1, B3, B7, and C) NF-κB.
IKK, another target of insulin resistance, is associated with vitamin inhibition. For example, vitamins E [Citation19], C [Citation61], and D3 [Citation62] inhibit inflammation and decrease the intracellular proinflammatory state. However, our results did not reproduce these reported interactions. In contrast, our findings highlight the putative interaction between vitamin A and B12 and IKK, and the molecular models that represent these molecular interactions are available in Table S1 in the supplementary material section.
In summary, several studies show that vitamins administered individually (e.g. B2 [Citation12], B3 [Citation13], B5 [Citation14], B6, B7, B12 [Citation15], C [Citation64], D [Citation65], E [Citation66]) or co-administrated using multivitamins formulations [Citation67–69] can decrease IR, guided by is the regulation of oxidative stress pathways [Citation70].
Additionally, vitamins show have demonstrated an anti-inflammatory effect by decreasing serum levels of TNF-α, IL-6, IL-1, and IL-1β [Citation10,Citation12,Citation14,Citation16,Citation19,Citation20]. Nevertheless, vitamins C [Citation15], D [Citation65] have been associated with the non-genomic pathway MAPK, vitamins C [Citation61], D3 [Citation62] and E [Citation36] can inhibit IKK and B2, B5, and B6 inhibit the p105 subunit of NF-κB [Citation12,Citation14,Citation63]. In line manner, our main results suggest the potential inhibitory activity of vitamins B1, B3, B5, B6, and B7 against MAPK or B1, B3, B7, and C the potential inhibitory of NF-κB. In contrast, our results did not reproduce inhibitory activity of IKK with previous reports.
In addition, the authors remarked on the limitations of this study. For example, targets with a probability of 70% (in target predictor servers) could be inhibited by vitamins, but this was not considered in this study. In addition, we performed molecular docking with the structures of the vitamins in their pure state and not with their respective metabolites, as we expected to find them in in vivo models. Additionally, there is insufficient experimental data to confirm the putative binding site predicted in this study. For this reason, the authors remark on the importance of generating new crystallography, in vitro, and in vivo studies to complement this gap in information, allowing the generation of new perspectives and hypotheses. For example, the following section provides an integrative overview of in silico, in vitro, and in vivo achievements that explain the complex context of IR in more detail, which could lead to the rationalization of a novel polypharmacology therapy to combat it.
Overview and perspectives
Inflammation is a key endpoint of IR, and vitamins playing a significant role in IR protection [Citation8]. For example, several vitamins (e.g., B1, B2, B3, B5, C, D3, and E) have the potential to restore the inflammatory environment in metabolic diseases [Citation64–66] and mediate the regulation of targets related to inflammatory pathways (e.g., MAPK, IKK, JNK, and NF-κB; ).
Figure 6. Summary of vitamins and targets validated on in vivo or in vitro models and putative inhibitory activities (predicted with in silico tools in this work).
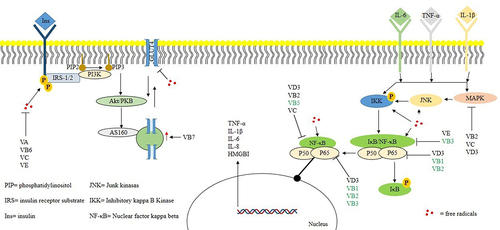
Several researchers have reported that the administration of a multivitamin complex can prevent IR [Citation67,Citation68,Citation71–73]. Multivitamin treatment significantly decreased the serum levels of TNF-α, IL-6, IL-1, and IL-1β in murine models. However, anti-inflammatory related targets (such as NF-κB, MAPK, and IKK) have not been quantified, generating an information gap in the complete understanding of the anti-inflammatory mechanisms [Citation20]. This information limitation justifies our work, which improves our knowledge about the anti-inflammatory effects of vitamins.
Polypharmacology offers new perspectives that can resolve different issues related to single-target drug design [Citation26,Citation74]. Simultaneously, polypharmacology presents the possibility of generating novel and refined approaches to address complex diseases such as metabolic diseases (e.g., IR) [Citation75,Citation76]. In this context, the generation and optimization of polypharmacological formulations represent a new challenge in drug design. Which is imperative for the study of their interaction with secondary targets. Furthermore, the experimental validation (using in vitro models) of multiple target interactions does not guarantee their clinical relevance; in these cases, the clinical impact must be confirmed using in vivo models.
This study provides an overview of vitamins with potential applications in IR treatment and prevention. Vitamins were selected based on previously reported knowledge. Furthermore, this study was limited by the selected targets (). However, this is a key point in the design of new chemical entities against IR and a discussion point regarding its nutraceutical role.
Moreover, this bibliographic and in silico study (using validated methods) uncovers the possibility of identifying vitamins that act at different molecular levels (i.e., changing the signalling, gene expression, epigenetic modifications or physiological effects) on IR, and opens new hypotheses that must be corroborated by in vitro or in vivo models. In fact, the parallel modulation of different endpoints could contribute to reducing the necessary doses to generate therapeutic effects that simultaneously contribute to reducing the associated side effects in in vivo models and the excessive economic costs derived from it. In addition, it can be used as an alternative to animal models. Finally, understanding the polyactivities of vitamins could improve preventive medicine [Citation77–79].
Conclusions
The role of vitamins in the regulation of the NF-κB signalling pathway during IR, and this regulation was associated with MAPK, IKK, and NF-κB, without neglecting free radicals, such as important mechanisms involved in insulin receptor oxidation and indirect activation of the NF-κB pathway.
Our main results indicated that vitamins C and D3 interact with the protein kinase domain and p38 MAPK-binding site of MAPK. Additionally, the results suggest that vitamins B2, B5, and B6 interact with the rel homology domain (RHD) domain of NF-κB, which explains their inhibitory activity against MAPK and NF-κB. Finally, the molecular docking results suggested that vitamins A and B12 protect against IKK. The present work clearly opens new perspectives to study targets such as NF-κB, MAPK, and IKK involved in the inflammatory context of IR and in the development of multivitamin treatments. A follow-up study could discuss the regulation of the NF-κB signalling pathway, MAPK, and IKK in the prevention or treatment of IR, which must be investigated in vitro and in vivo based on drug discovery.
Methodology
The methodology includes diverse in silico tools to improve our understanding of the putative polypharmacological activity of different vitamins (A, B1, B2, B3, B5, B6, B7, B12, C, D3, and E) against possible targets. The first part of the methodology describes the use of predictive servers based on molecular similarity and biological interactome similarity methods to predict the targets of the different vitamins evaluated in this study. The second part of the methodology describes the molecular docking protocol to describe the putative binding interactions between vitamins and the main targets (NF-κB, MAPK, and IKK) predicted in the first methodological section.
Target prediction
Each vitamin was represented using the canonical SMILES obtained from the ChEMBL database, version 33 [Citation80]. Each SMILE code was used to predict putative interactions of each vitamin with different targets. We used seven target-predictor servers (Swiss Target prediction [Citation31], Super-PRED [Citation32], TargetNet, TargetHunter [Citation33], STITCH [Citation34] and PPB [Citation35]) based on structure similarity and biological interactome similarity respect to reporter inhibitors. Targets related to IR with in vitro and in vivo validation, which additionally have been predicted by (at least) two different servers, were studied using molecular docking (vide infra).
Molecular docking
Protein and ligand preparation
The crystallographic structures of MAPK, NF-κB, and IKK (PDB IDs: 3FHR, 4Q3J, and 4E3C, respectively) were retrieved from the Protein Data Bank (available online: https://www.rcsb.org/ (accessed on 12 March 2023) [Citation81]. The ligands were built and energy-minimized in the Molecular Operating Environment (MOE) software v. 2023 using the MMFF94× force field [Citation82]. Protomers that were more stable at physiological pH were identified.
Molecular Operating Environment
The MOE software was used to generate the docking conformation of the protein-ligand complexes. For MAPK, the grid was centred in the inhibitor cavity, with a size of 27 Å3. For NF-κB and IKK, a grid was constructed around the complete proteins, that is blind docking [Citation27]. Using the ‘Triangle Matcher’ method, the binding compounds were subjected to 50 search steps (poses) and the default values for the other parameters. Clusters with RMSD < 2 Å were visually explored and considered representative and potential interactions against the studied targets [Citation83]. During docking simulations, the receptor was considered rigid, and the ligands were flexible. Conformations with the lowest binding energy were selected for additional and exhaustive visualization. For each target, the molecular docking protocol was validated, and the binding pose of crystallographically reported inhibitors was reproduced using this protocol. For example, for NF-κB, MAPK, and IKK, we used oxidized hTRX, SRC-SM1-71-R, and Cmpd1/2 as positive controls. Crystallographic poses were reproduced with an RMSD value lower than 2 angstroms [Citation84–86].
Ligand efficiency calculation
Ligand efficiency (LE) is used to improve the interpretation of docking scores because it affects the size of the ligand, which can have a marked influence on the binding sites [Citation87]. The LE was calculated from the docking score of each control and ligand (i.e., vitamins) using Equation 1:
(1) Ligand Efficiency = Docking Score/Heavy Atom Count
The Heavy Atom Count for each ligand was calculated using DataWarrior software V. 5.5.0 [Citation88].
Abbreviation list
Author contributions
The authors confirm contribution to the paper as follows: study conception and design: JAPJ, ELL, JLMF and ISR; data collection: JAPJ and ELL; analysis and interpretation of results: JAPJ, ELL, JLMF, OMR and ISR; draft manuscript preparation: JAPJ, ELL, JLMF, OMR and ISR. All authors reviewed the results and approved the final version of the manuscript.
Acknowledgments
E.L.-L. is grateful to Consejo Nacional de Humanidades Ciencias y Tecnología (CONAHCyT), Mexico, for the Ph.D. scholarship number, 762342 (No. CVU: 894234).
Disclosure statement
No potential conflict of interest was reported by the author(s).
Data availability statement
The data that support the findings of this study are openly available in figshare at http://doi.org/10.6084/m9.figshare.24547936, reference number [Citation89].
Additional information
Funding
References
- Endalifer ML, Diress G. Epidemiology, predisposing factors, biomarkers, and prevention mechanism of obesity: A systematic review. J Obes. 2020;2020:1–13. doi: 10.1155/2020/6134362
- Lee SH, Park SY, Choi CS. Insulin resistance: from mechanisms to therapeutic strategies. Diabetes Metab J. 2022;46(1):15–37. doi: 10.4093/dmj.2021.0280
- Yeung YT, Aziz F, Guerrero-Castilla A, et al. Signaling pathways in inflammation and anti-inflammatory therapies. Curr Pharm Des. 2018;24(14):1449–1484. doi: 10.2174/1381612824666180327165604
- Kawai T, Autieri MV, Scalia R. Adipose tissue inflammation and metabolic dysfunction in obesity. Am J Physiol Cell Physiol. 2021;320(3):C375–C391. doi: 10.1152/ajpcell.00379.2020
- Ren Y, Zhao H, Yin C, et al. Adipokines, hepatokines and myokines: focus on their role and molecular mechanisms in adipose tissue inflammation. Front Endocrinol. 2022;13:873699. doi: 10.3389/fendo.2022.873699
- Pivari F, Mingione A, Brasacchio C, et al. Curcumin and type 2 diabetes mellitus: prevention and treatment. Nutrients. 2019;11(8):1837. doi: 10.3390/nu11081837
- Tran N, Pham B, Le L. Bioactive compounds in anti-diabetic plants: From herbal medicine to modern drug discovery. Biology (Basel). 2020;9(9):252. doi: 10.3390/biology9090252
- Gasmi A, Noor S, Menzel A, et al. Obesity and insulin resistance: associations with chronic inflammation, genetic and epigenetic factors. Curr Med Chem. 2020;28(4):800–826. doi: 10.2174/0929867327666200824112056
- Avignon A, Hokayem M, Bisbal C, et al. Dietary antioxidants: do they have a role to play in the ongoing fight against abnormal glucose metabolism? Nutrition. 2012;28(7–8):715–721. doi: 10.1016/j.nut.2012.01.001
- Selvam S, Ramaian Santhaseela A, Ganesan D, et al. Autophagy inhibition by biotin elicits endoplasmic reticulum stress to differentially regulate adipocyte lipid and protein synthesis. Cell Stress Chaperones. 2019;24(2):343–350. doi: 10.1007/s12192-018-00967-9
- Mahdavifard S, Nakhjavani M. Thiamine pyrophosphate improved vascular complications of diabetes in rats with type 2 diabetes by reducing glycation, oxidative stress, and inflammation markers. Med J Islam Repub Iran. 2020;34(1):47. doi: 10.47176/mjiri.34.47
- Mazur-Bialy AI, Pocheć E. Riboflavin reduces pro-inflammatory activation of adipocyte-macrophage co-culture. Potential application of vitamin B2 enrichment for attenuation of insulin resistance and metabolic syndrome development. Molecules. 2016;21(12):1724. doi: 10.3390/molecules21121724
- Walocko FM, Eber AE, Keri JE, et al. The role of nicotinamide in acne treatment. Dermatol Ther. 2017;30(5). doi: 10.1111/dth.12481
- Jung S, Kim MK, Choi BY. The long-term relationship between dietary pantothenic acid (vitamin B5) intake and C-reactive protein concentration in adults aged 40 years and older. Nutr Metab Cardiovasc Dis. [2017 Sep 1];27(9):806–816. doi: 10.1016/j.numecd.2017.05.008
- Nemazannikova N, Mikkelsen K, Stojanovska L, et al. Is there a link between vitamin B and multiple sclerosis? Med Chem. 2018;14(2):170–180. doi: 10.2174/1573406413666170906123857
- Canali R, Natarelli L, Leoni G, et al. Vitamin C supplementation modulates gene expression in peripheral blood mononuclear cells specifically upon an inflammatory stimulus: a pilot study in healthy subjects. Genes Nutr. 2014;9(3):390. doi: 10.1007/s12263-014-0390-x
- Krisnamurti DGB, Louisa M, Poerwaningsih EH, et al. Vitamin D supplementation alleviates insulin resistance in prediabetic rats by modifying IRS-1 and PPARγ/NF-κB expressions. Front Endocrinol. 2023;14:1089298. doi: 10.3389/fendo.2023.1089298
- Miyazawa T, Burdeos GC, Itaya M, et al. Vitamin E: regulatory redox interactions. IUBMB Life. 2019;71(4):430–441. doi: 10.1002/iub.2008
- Jiang Q. Natural forms of vitamin E: Metabolism, antioxidant, and anti-inflammatory activities and their role in disease prevention and therapy. Free Radic Biol Med. 2014;72:76–90. doi: 10.1016/j.freeradbiomed.2014.03.035
- El-Sharkawy A, Malki A. Vitamin D signaling in inflammation and cancer: Molecular mechanisms and therapeutic implications. Molecules. 2020;2514(14):3219. doi: 10.3390/molecules25143219
- Santibáñez-Morán MG, López-López E, Prieto-Martínez FD, et al. Consensus virtual screening of dark chemical matter and food chemicals uncover potential inhibitors of SARS-CoV-2 main protease. RSC Adv. 2020;10(42):25089–25099. doi: 10.1039/D0RA04922K
- Jiménez-Orozco FA, Galicia-Zapatero S, López-López E, et al. Monosubstituted coumarins inhibit epinephrine-induced platelet aggregation. Cardiovasc Hematol Agents Med Chem. 2021;20(1):43–51. doi: 10.2174/1871525719666210427132808
- Medina-Franco JL, López-López E, Martínez-Fernández LP. 7-aminoalkoxy-quinazolines from epigenetic focused libraries Are potent and selective Inhibitors of DNA Methyltransferase 1. Molecules. 2022;27(9):2892. doi: 10.3390/molecules27092892
- López-López E, Bajorath J, Medina-Franco JL. Informatics for chemistry, biology, and biomedical sciences. J Chem Inf Model. 2021;61(1):26–35. doi: 10.1021/acs.jcim.0c01301
- Ferreira LG, Dos Santos RN, Oliva G, et al. Molecular docking and structure-based drug design strategies. Molecules. 2015;20(7):13384–13421. doi: 10.3390/molecules200713384
- Garcia-Romero EM, López-López E, Soriano-Correa C, et al. Polypharmacological drug design opportunities against Parkinson’s disease. F1000Res. 2022;11:11. doi: 10.12688/f1000research.124160.1
- Prieto-Martínez FD, López-López E, Eurídice Juárez-Mercado K, et al. Computational drug design methods—current and future perspectives. In: Roy K, editor. Silico Drug Design: repurposing Techniques and Methodologies. Academic Press; 2019. p. 19–44.
- Rong K, He Q, Chen S, et al. The mechanism of vitamin D3 in preventing colorectal cancer through network pharmacology. Front Pharmacol. 2023;14:1192210. doi: 10.3389/fphar.2023.1192210
- Xu X, Luo H, Chen Q, et al. Detecting potential mechanism of vitamin D in treating rheumatoid arthritis based on network pharmacology and molecular docking. Front Pharmacol. 2022;13:1047061. doi: 10.3389/fphar.2022.1047061
- Mathai N, Kirchmair J. Similarity-based methods and machine learning approaches for target prediction in early drug discovery: performance and scope. Int J Mol Sci. 2020;21(10):3585. doi: 10.3390/ijms21103585
- Daina A, Michielin O, Zoete V. SwissTargetPrediction: updated data and new features for efficient prediction of protein targets of small molecules. Nucleic Acids Res. 2019;47(W1):W357–W364. doi: 10.1093/nar/gkz382
- Dunkel M, Günther S, Ahmed J, et al. SuperPred: drug classification and target prediction. Nucleic Acids Res. 2008;36(Web Server issue):W55–9. doi: 10.1093/nar/gkn307
- Wang L, Ma C, Wipf P, et al. Targethunter: an in silico target identification tool for predicting therapeutic potential of small organic molecules based on chemogenomic database. Aaps J. 2013;15(2):395–406. doi: 10.1208/s12248-012-9449-z
- Szklarczyk D, Santos A, Von Mering C, et al. STITCH 5: Augmenting protein-chemical interaction networks with tissue and affinity data. Nucleic Acids Res. 2016;44(D1):D380–4. doi: 10.1093/nar/gkv1277
- Awale M, Reymond JL. The polypharmacology browser: a web-based multi-fingerprint target prediction tool using ChEMBL bioactivity data. J Cheminform. 2017;9(1):11. doi: 10.1186/s13321-017-0199-x
- Gallo K, Goede A, Preissner R, et al. SuperPred 3.0: drug classification and target prediction - a machine learning approach. Nucleic Acids Res. 2022;50(W1):W726–W731. doi: 10.1093/nar/gkac297
- Bao BY, Hu YC, Ting HJ, et al. Androgen signaling is required for the vitamin D-mediated growth inhibition in human prostate cancer cells. Oncogene. 2004;23(19):3350–3360. doi: 10.1038/sj.onc.1207461
- Gyamfi M, Wan Y. The effect of ethanol, ethanol metabolizing enzyme inhibitors, and vitamin E on regulating glutathione, glutathione S-transferase, and S-adenosylmethionine in mouse primary hepatocyte. Hepatol Res. 2006;35(1):53–61. doi: 10.1016/j.hepres.2006.02.003
- Alshabrawy AK, Cui Y, Sylvester C, et al. Therapeutic Potential of a Novel Vitamin D3 Oxime Analogue, VD1-6, with CYP24A1 enzyme inhibitory activity and negligible vitamin D receptor Binding. Biomolecules. 2022;12(7):960. doi: 10.3390/biom12070960
- Shimazawa R, Suzuki T, Dodo K, et al. Design and synthesis of dysidiolide analogs from vitamin D3: novel class of Cdc25A inhibitors. Bioorg Med Chem Lett. 2004;14(12):3291–3294. doi: 10.1016/j.bmcl.2004.03.100
- Nagorna-Stasiak B, Lazuga-Adamczyk A, Kolodynska M. Alpha-amylase in the serum and tissues of rabbits and the effect of vitamin C on its activity. Acta Physiol Pol. 1987;38(1):15–21.
- Adelakun SA, Ogunlade B, Siyanbade JA, et al. Evaluation of the ameliorative potential of nutritional dietary supplementation of vitamin B12 following chronic tramadol hydrochloride exposure induced hyperlipidemia, atherogenic indices, and hepato-renal dysfunction by downregulating redox imbalance, NO/iNOS/NF-κB response, caspase-3- activation and DNA fragmentation in rat’s model. Food Chem Adv. 2023;2:100286.
- Konze KD, Pattenden SG, Liu F, et al. A chemical tool for in vitro and in vivo precipitation of lysine methyltransferase G9a. ChemMedchem. 2014;9(3):549–553. doi: 10.1002/cmdc.201300450
- Torres-Méndez JK, Niño-Narvión J, Martinez-Santos P, et al. Nicotinamide prevents diabetic brain inflammation via NAD±Dependent deacetylation mechanisms. Nutrients. 2023;15(14):3083. doi: 10.3390/nu15143083
- Chen AC, Damian DL. Nicotinamide and the skin. Australas J Dermatol. 2014;55(3):169–175. doi: 10.1111/ajd.12163
- Gergis NK, Ashour WMR, Mohamed SE, et al. Nicotinamide role among kidney disease: review article. Egypt J Hosp Med. 2023;90(2). doi: 10.21608/ejhm.2023.292758
- Duc Nguyen H, Hee Jo W, Hong Minh Hoang N, et al. Anti-inflammatory effects of B vitamins protect against tau hyperphosphorylation and cognitive impairment induced by 1,2 diacetyl benzene: An in vitro and in silico study. Int Immunopharmacol. 2022;108:108736. doi: 10.1016/j.intimp.2022.108736
- Lindqvist Y, Schneider G, Ermler U, et al. Three-dimensional structure of transketolase, a thiamine diphosphate dependent enzyme, at 2.5Å resolution. Embo J. 1992;11(7):2373–2379. doi: 10.1002/j.1460-2075.1992.tb05301.x
- Petkovich M, Chambon P. Retinoic acid receptors at 35 years. J Mol Endocrinol. 2022;69(4):T13–T24. doi: 10.1530/JME-22-0097
- Martin RL, Heifetz A, Bodkin MJ, et al. High-throughput structure-based drug design (HT-SBDD) using drug docking, fragment molecular orbital calculations, and molecular dynamic techniques. Methods Mol Biol. 2024;2716:293–306.
- López-López E, Barrientos-Salcedo C, Prieto-Martínez FD, et al. In silico tools to study molecular targets of neglected diseases: inhibition of TcSir2rp3, an epigenetic enzyme of Trypanosoma cruzi. Adv Protein Chem Struct Biol. 2020;122:203–229.
- Hao J, Han L, Zhang Y, et al. Docking studies on potential mechanisms for decreasing insulin resistance by the tangzhiqing herbal formula. Evid Based Complemt Alternat Med. 2020;2020:1057648. doi: 10.1155/2020/1057648
- Su X, Shen Z, Yang Q, et al. Vitamin C kills thyroid cancer cells through ROS-dependent inhibition of MAPK/ERK and PI3K/AKT pathways via distinct mechanisms. Theranostics. 2019;9(15):4461–4473. doi: 10.7150/thno.35219
- Li X, Liu J, Zhao Y, et al. 1,25-dihydroxyvitamin D3 ameliorates lupus nephritis through inhibiting the NF-κB and MAPK signalling pathways in MRL/lpr mice. BMC Nephrol. 2022;23(1):243. doi: 10.1186/s12882-022-02870-z
- UniProt. 2023. Available from: https://www.uniprot.org/uniprotkb/Q16644/entry Q16644 · MAPK3_HUMAN.
- Ghosh G, Huang D, Huxford T. Molecular mimicry of the NF-κB DNA target site by a selected RNA aptamer. Curr Opin Structur Biol. 2004;14(1):21–27. doi: 10.1016/j.sbi.2004.01.004
- UniProt. 2023. p. 19838. Available from: https://www.uniprot.org/uniprotkb/P19838/entry NFKB1_HUMAN.
- Arkan MC, Hevener AL, Greten FR, et al. IKK-β links inflammation to obesity-induced insulin resistance. Nat Med. 2005;11(2):191–198. doi: 10.1038/nm1185
- Hayden MS, Ghosh S. Shared principles in NF-kappaB signaling. Cell. 2008;132(3):344–362. doi: 10.1016/j.cell.2008.01.020
- Baker RG, Hayden MS, Ghosh S. NF-κB, inflammation, and metabolic disease. Cell Metab. 2011;13(1):11–22. doi: 10.1016/j.cmet.2010.12.008
- Pedraza A, Bo O, Golde DW. Vitamin C suppresses TNF alpha-induced NF kappa B activation by inhibiting I kappa B alpha phosphorylation. Biochemistry. 2002;41(43):12995–13002. doi: 10.1021/bi0263210
- Nonn L, Peng L, Feldman D, et al. Inhibition of p38 by vitamin D reduces interleukin-6 production in normal prostate cells via mitogen-activated protein kinase phosphatase 5: implications for prostate cancer prevention by vitamin D. Cancer Res. 2006;66(8):4516–4524. doi: 10.1158/0008-5472.CAN-05-3796
- Zemel MB, Bruckbauer A. Effects of a leucine and pyridoxine-containing nutraceutical on fat oxidation, and oxidative and inflammatory stress in overweight and obese subjects. Nutrients. 2012;4(6):529–541. doi: 10.3390/nu4060529
- Chen H, Karne RJ, Hall G, et al. High-dose oral vitamin C partially replenishes vitamin C levels in patients with type 2 diabetes and low vitamin C levels but does not improve endothelial dysfunction or insulin resistance. Am J Physiol Heart Circ Physiol. 2006;290(1):H137–45. doi: 10.1152/ajpheart.00768.2005
- Szymczak-Pajor I, Drzewoski J, Śliwińska A. The molecular mechanisms by which vitamin d prevents insulin resistance and associated disorders. Int J Mol Sci. 2020;21(18):6644. doi: 10.3390/ijms21186644
- Manning PJ, Sutherland WHF, Walker RJ, et al. Effect of high-dose vitamin E on insulin resistance and associated parameters in overweight subjects. Diabetes Care. 2004;27(9):2166–2171. doi: 10.2337/diacare.27.9.2166
- Palma-Jacinto JA, Santiago-Roque I, Coutiño-Rodríguez R, et al. Effect of a multivitamin on insulin resistance, inflammation, and oxidative stress in a Wistar rat model of induced obesity. Nutrición Hospitalaria. 2023;40(6):1183–119. doi: 10.20960/nh.04621
- Sárközy M, Szucs G, Pipicz M, et al. The effect of a preparation of minerals, vitamins and trace elements on the cardiac gene expression pattern in male diabetic rats. Cardiovasc Diabetol. 2015;14(1):85. doi: 10.1186/s12933-015-0248-6
- Sárközy M, Fekete V, Szucs G, et al. Anti-diabetic effect of a preparation of vitamins, minerals and trace elements in diabetic rats: A gender difference. BMC Endocr Disord. 2014;14(1):72. doi: 10.1186/1472-6823-14-72
- Lampousi AM, Lundberg T, Löfvenborg JE, et al. Vitamins C, E, and beta-carotene and risk of type 2 diabetes: a systematic review and meta-analysis. Adv Nutr. 2024;15(5):100211. doi: 10.1016/j.advnut.2024.100211
- Deshmukh U, Katre P, Yajnik CS. Influence of maternal vitamin B12 and folate on growth and insulin resistance in the offspring. Nestle Nutr Inst Workshop Ser. 2013;74:145–154.
- Imanparast F, Javaheri J, Kamankesh F, et al. The effects of chromium and vitamin D3 co-supplementation on insulin resistance and tumor necrosis factor-alpha in type 2 diabetes: a randomized placebo-controlled trial. Appl Physiol Nutr Metab. 2020;45(5):471–477. doi: 10.1139/apnm-2019-0113
- Fong C, Alesi S, Mousa A, et al. Efficacy and safety of nutrient supplements for glycaemic control and insulin resistance in type 2 diabetes: An umbrella review and hierarchical evidence synthesis. Nutrients. 2022;14(1):2295. doi: 10.3390/nu14112295
- Proschak E, Stark H, Merk D. Polypharmacology by design: a medicinal Chemist’s perspective on multitargeting compounds. J Med Chem. 2019;62(2):420–444. doi: 10.1021/acs.jmedchem.8b00760
- Wang Z, Yang B. Polypharmacology in clinical applications: Metabolic Disease Polypharmacology. In Polypharmacology. Springer, Cham; 2022. p. 199–229. https://doi.org/10.1007/978-3-031-04998-9_5
- Wright EE, Stonehouse AH, Cuddihy RM. In support of an early polypharmacy approach to the treatment of type 2 diabetes. Diab Obes Metab. 2010;12(11):929–940. doi: 10.1111/j.1463-1326.2010.01255.x
- Volpe M, Chin D, Paneni F. The challenge of polypharmacy in cardiovascular medicine. Fundam Clin Pharmacol. 2010;24(1):9–17. doi: 10.1111/j.1472-8206.2009.00757.x
- Snegarova V, Naydenova D. Vitamin D: a review of its effects on epigenetics and gene regulation. Folia Med (Plovdiv). 2020;62(4):662–667. doi: 10.3897/folmed.62.e50204
- Yang Z, Kubant R, Cho CE, et al. Micronutrients in high-fat diet modify insulin resistance and its regulatory genes in adult male mice. Mol Nutr Food Res. 2023;67(18):e2300199. doi: 10.1002/mnfr.202300199
- Mendez D, Gaulton A, Bento AP, et al. ChEMBL: towards direct deposition of bioassay data. Nucleic Acids Res. 2019;47(D1):D930–D940. doi: 10.1093/nar/gky1075
- Berman HM, Westbrook J, Feng Z, et al. The Protein Data Bank. Nucleic Acids Res. 2000;28(1):235–242. doi: 10.1093/nar/28.1.235
- Chemical Computing Group Inc. Molecular Operating Environment (MOE); 2023. Available from: https://www.chemcomp.com/
- López-López E, Cerda-García-Rojas CM, Medina-Franco JL. Consensus virtual screening protocol towards the identification of small molecules interacting with the colchicine binding site of the tubulin-microtubule system. Mol Inform. 2023;42(1):e2200166. doi: 10.1002/minf.202200166
- Qin J, Clore GM, Kennedy WP, et al. Solution structure of human thioredoxin in a mixed disulfide intermediate complex with its target peptide from the transcription factor NFκB. Structure. 1995;3(3):289–297. doi: 10.1016/S0969-2126(01)00159-9
- Rao S, Gurbani D, Du G, et al. Leveraging compound promiscuity to identify targetable cysteines within the kinome. Cell Chem Biol. 2019;26(6):818–829. doi: 10.1016/j.chembiol.2019.02.021
- Xu G, Lo YC, Li Q, et al. Crystal structure of inhibitor of κb kinase β. Nature. 2011;472(7343):325–330. doi: 10.1038/nature09853
- García-Sosa AT, Hetényi C, Maran UKO. Drug efficiency indices for improvement of molecular docking scoring functions. J Comput Chem. 2010;31(1):174–184. doi: 10.1002/jcc.21306
- López-López E, Naveja JJ, Medina-Franco JL. DataWarrior: an evaluation of the open-source drug discovery tool. Expert Opin Drug Discov. 2019;14(4):335–341. doi: 10.1080/17460441.2019.1581170
- Palma-Jacinto JA, López-López E, MEDINA-FRANCO JL, et al. Files of paper “Putative mechanism of a multivitamin treatment against insulin resistance” [Internet]. 2023 [cited 2024 Jun 7]. Available from: https://figshare.com/articles/dataset/Files_of_paper_Putative_mechanism_of_a_multivitamin_treatment_against_insulin_resistance_/24547936/1