ABSTRACT
Immunotherapy with checkpoint inhibitors, such as antibodies blocking the programmed cell-death receptor-1 (PD-1), has resulted in remarkable responses in patients having traditionally refractory cancers. Although response to PD-1 inhibitors correlates with PD-1 ligand (PD-L1 or PD-L2) expression, PD-1 ligand positivity represents only a part of the predictive model necessary for selecting patients predisposed to respond to immunotherapy. We used all genomic, transcriptomic, proteomic and phenotypic data related to 8,475 pan-cancer samples available in The Cancer Genome Atlas (TCGA) and conducted a logistic regression analysis based on a large set of variables, such as microsatellite instability (MSI-H), mismatch repair (MMR) alterations, polymerase δ (POLD1) and polymerase ϵ (POLE) mutations, activation-induced/apolipoprotein-B editing cytidine deaminases (AID/APOBEC) alterations, lymphocyte markers and mutation burden estimates to determine independent factors that associate with PD-1 ligand overexpression. PD-1 ligand overexpression was independently and significantly correlated with overexpression of and mutations in APOBEC3 paralogs. Additionally, while high tumor mutation burden and overexpression of PD-L1 have been previously correlated with each other, we demonstrate that the specific mutation pattern caused by APOBEC enzymes and called kataegis—rather than overall mutation burden, MSI-H or MMR alterations—correlates independently with PD-L1/PD-L2 expression. These observations suggest that APOBEC3 alterations, APOBEC3 overexpression and kataegis play an important role in the regulation of PD-1 ligand overexpression, and thus, their relationship with immune checkpoint inhibitor response warrants exploration.
Introduction
During the last decade, various immunotherapy approaches have shown promising results in patients having cancer. By modulation of self-reactive cellular immunity (mediated by cytotoxic T-lymphocytes), these therapies permit substantial tumor regression, sometimes leading to durable clinical responses.Citation1 One of the most successful strategies in this regard consists of impeding the immune checkpoints located on the surface of T-cells, which act as “off”-switches when bound to their ligands found on antigen-presenting and tumor cells and, thus, are considered protective factors for the cancer cell.Citation2 Recently, 3 immune checkpoint inhibitors blocking the programmed cell-death 1 receptor (PD-1 or PDCD1) or its ligands—namely PD-L1 (CD274 or B7-H1) and PD-L2 (CD273 or PDCD1LG2) —have been approved by the Food and Drug Administration (FDA): nivolumab, pembrolizumab and atezolizumab; for the treatment of melanoma, Hodgkin's lymphoma, renal cell cancer, non-small cell lung cancer (NSCLC) and bladder cancer.Citation3-8
PD-1 ligands are constitutively expressed in a variety of normal tissues, such as placenta, heart, lung, liver, spleen, lymph nodes and thymus.Citation9 Expression of PD-L1 can be induced in lymphocytes, monocytes, macrophages and epithelial cells after infection by pathogens or inflammatory processes, through the bacterial lipopolysaccharides (LPS), the pro-inflammatory cytokine interferon gamma (IFNγ), and to a lesser extent by the tumor necrosis factor alpha (TNFα).Citation9 Aberrant PD-L1 expression may also be observed as a result of various genomic and post-transcriptional alterations, such as after introduction of an ectopic PD-L1 gene promoter by a translocation phenomenonCitation10 or after stabilization by truncation of the 3′ untranslated region (3′-UTR) of the PD-L1 transcript.Citation11 PD-L2 induced expression is less frequent and restricted to limited cell types.Citation9 Both PD-L1 and PD-L2 protein overexpression have been described as relevant, albeit imperfect, predictive biomarkers for the response to anti-PD-1 and/or anti-PD-L1 agents.Citation12,13 Additionally, PD-L1 and PD-L2 amplification (both genes are located on the same amplicon on the short arm of chromosome 9) has been associated with high response rates to anti-PD-1 agents in Hodgkin's lymphoma.Citation8,14
Recent evidence has established a link between the genomic instability of cancer and the response to checkpoint blockade in various tumor types. In colorectal and endometrial carcinoma, mismatch repair (MMR) deficient tumors (also described as microsatellite instability “high” or MSI-H tumors) present higher levels of PD-L1 and PD-L2 compared to MMR-proficient tumors and this association may explain, at least in part, the high clinical response rates observed in various colonic and extra-colonic MSI-H tumors after pembrolizumab treatment.Citation15,16 PD-L1 expression has also been associated with high tumor mutation burden in melanoma,Citation17 NSCLC,Citation18 and with additional mechanisms leading to hyper-mutativity, such as POLE and POLD1 aberrations in endometrial carcinomaCitation19 and APOBEC3 overexpression in urothelial carcinoma.Citation20 However, the molecular mechanisms underlying the association between PD-L1/2 overexpression, the salutary effects of immune checkpoints inhibition and the tumor mutation burden remain largely elusive.
Aggregation of a large number of mutations in a cell can be caused by exposure to exogenous mutagens (such as ultraviolet radiation or tobacco-related carcinogens) or several endogenous mutagenic processes. In particular, tumor hyper-mutation has been associated with different mechanisms impairing the DNA replication fidelity process: (i) loss of DNA damage repair function by mutation, deletion or post-transcriptional regulation of MMR proteins; (ii) alterations of the proof-reading domains of replicative polymerases ϵ and ∂ by mutation of POLE or POLD1 gene; and (iii) unleashed activity of APOBEC (apolipoprotein B mRNA editing cytidine deaminase) enzymes, which leads to a localized hyper-mutation phenomenon called kataegis.Citation21,22
APOBEC family members are nucleic acid mutators originally implicated in lipoprotein metabolism (by apolipoprotein-B mRNA editing),Citation23 antigen-driven antibody diversification (by somatic hyper-mutation, gene conversion and class-switch recombination of immunoglobulin genes)Citation24 and innate anti-retroviral immunity (by inhibition of the replication of retroviruses and retrotransposons).Citation25 Although each member of the APOBEC family (AICDA or AID, APOBEC1, APOBEC2, APOBEC3A, APOBEC3B, APOBEC3C, APOBEC3D, APOBEC3F, APOBEC3G, APOBEC3H and APOBEC4) presents distinct circumstances of expression and specific functions, their activity results almost exclusively in cytosine-to-thymine (C-to-T) transitions in their DNA/RNA substrates. Besides their natural and protective functions, APOBEC enzymes may also cause substantial off-target genotoxic damages (arising mostly during the DNA replication) and thus be implicated in tumor hyper-mutation.Citation26
In this study, we used a large set of pan-cancer tumor samples (TCGA Research Network: http://cancergenome.nih.gov/) and a series of multivariate regression models to elucidate the overall association between high levels of PD-1 ligand expression and the mutation burden mechanisms mentioned above, especially as it pertains to anomalies correlated with the APOBEC family of enzymes.
Results
Pan-cancer collection description
A total of 8,475 samples presenting a complete set of data (i.e., with complete annotation of genomic mutations, copy number variations (CNV) and mRNA expression) were included in the regression analysis. The samples corresponded to 32 different cancer types as described in Table S1.
All logistic regression models were built by using single and/or combined predictors gathered by function: MMR alteration (8 markers), polymerases δ and ϵ mutation (2 markers), AID/APOBEC family alteration (36 markers), PD-1 ligand alteration (9 markers), microsatellite phenotype (1 marker), lymphocyte phenotype (11 markers) and mutation burden/genomic instability estimate (5 markers), as defined in Table S2. A minority of samples were evaluated for MSI (1,328 samples— 15.7% of the tumors) and only 3,088 (72.9%) and 3,009 (71.0%) tumors were tested by immunohistochemistry to assess lymphocyte and macrophage infiltration. For these particular markers, analysis was done by examining patients with the presence of the marker versus those without, as well as with an unknown status versus those without. For markers corresponding to specific genomic alteration (mutation, copy number variation or mRNA expression), assessment was done as yes versus no for the presence of the marker. Finally, for mutation burden markers, groups-of-interest were delimited at the median: samples with value greater than median compared with samples with value lower or equal to median (Table S2).
When considering the complete collection, median number of mutations per sample was 66.5 (ranging from 0 to 23,950) and minimal number of APOBEC-related alterations was 0 (ranging from 0 to 2,347 minimal load of APOBEC-related alterations per sample). Median number of gains, losses and CNV per sample were 3,228 (ranging from 0 to 14,686), 3,957.5 (ranging from 0 to 14,179) and 8,100 (ranging from 0 to 24,591), respectively.
Independent predictors of PD-1 ligand overexpression
A significantly high level of expression of PD-1 ligands was observed in 469 tumors (5.5% of the pan-cancer cohort), and most them (n = 406, 86.6%) presented at least one molecular alteration within MMR, polymerases ϵ or δ, APOBEC3 or PD-L1/2 factors ( and ).
Figure 1. Distribution of common molecular alterations in PD-L1/2 overexpressed tumors (part A). Molecular alterations were ordered by descending frequency in the subset studied (n = 469 tumors), except for MSI-High as too many tumors were not evaluated for this marker. Each column represents a tumor. Dark green: tumor exempt of alteration. Light green: tumor not presenting the mutation. Gray: alteration not evaluated in the tumor. Purple: total number of mutations and kataegis-related mutations observed in each tumor, represented in percentile (minimum: light purple, maximum: dark purple). Frequency of alterations in comparison to non PD-1 ligand overexpressed tumors (part B). The table presents frequencies of alterations in both PD-1 ligand overexpressed and non-overexpressed tumors, p values of the univariate analysis and p values obtained in the final model of prediction for PD-1 ligand overexpression. Median alterations counts were 66.5 total mutations and 0 kataegis-related mutations per tumor. PD-L1 3′-UTR data were extracted from Ref. Citation11. This factor is displayed for descriptive purpose only and was not included in the analysis. Abbreviations. APOBEC = apolipoprotein B editing complex; MMR = mismatch repair; MSI = microsatellite instability; MSI-high = microsatellite instability high; ns = non-significant; 3′-UTR = 3′ untranslated region.
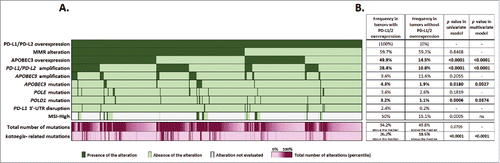
In univariate analysis, factors associated with PD-L1 or PD-L2 overexpression were: PD-1 ligand genomic locus amplification, MSI-H phenotype, POLD1 mutation, presence of kataegis, APOBEC3 mutation (APOBEC3G and APOBEG3H single factors were significant), AICDA overexpression, APOBEC3 overexpression (all 7 paralogs were significant), APOBEC4 amplification, monocytes infiltration, overexpression of immune markers (7 single factors were significant), as well as overexpression of IFNγ (Table S3).
Interdependent relationships between these factors and PD-1 ligand overexpression were assessed by a logistic regression method adapted to rare events (Firth's penalized likelihood analysis). The final models, as shown in , presented a pseudo-R2 (likelihood-ratio index of McFadden) of 25.9% and 24.2% (for models using single and combined factors, respectively), demonstrating the percentage of variability of PD-1 ligand overexpression that may be explained by the set of chosen factors.Citation27 Particularly, the model obtained with combined factors revealed a strong correlation between the presence of APOBEC alterations and the high level of expression of PD-L1 or PD-L2. APOBEC alterations were represented by the presence of any APOBEC3-member mRNA overexpression (Odds Ratio OR = 2.7, p < 0.0001), the presence of a coding mutation within any of the APOBEC3 paralogs (OR = 2.4, p = 0.0027) and the presence of a kataegis signature (OR = 1.3, p = 0.0210). Additional positively-related predictors were the presence of a PD-L1/2 amplification (OR = 3.6, p < 0.0001); overexpression of IFNγ (OR = 3.1, p < 0.0001); overexpression of T-lymphocyte, natural-killer cell, monocyte and macrophage markers (OR ranging from 1.6 to 3.2, p < 0.0135); and presence of a POLD1 mutation (OR = 2.1, p = 0.0374). All predictors described for the model presented in remained significant after application of the re-sampling method (1,000 replicates, p < 0.05).
Table 1. Multivariate analysis of associationFootnotea between all factors and PD-1 ligand mRNA overexpression, using single factors (model A) or relevant combined factors (model B).
These data confirmed the association previously observed between the PD-1 ligand expression and the presence of tumor mutagenesis processes, and to a lesser extent POLD1 alterations; but above all, allowed to highlight the critical role played by APOBEC enzymes and their dysfunction. Interestingly, kataegis-signature and APOBEC alterations appeared to be related to PD-L1/2 overexpression independently of the immune cell infiltrate and IFNγ release ().
Figure 2. Graphical model of conditional dependences between PD-1 ligand overexpression, APOBEC3 mutation, APOBEC3 overexpression, kataegis and mutational burden predictors. Arrows represent dependent relationships between factors. Each dependent variable (in red) can be modeled by the association of independent factors pointing in its direction (multivariate models using combined factors, p ≤ 0.05). Confirmed bilateral relationships between major factors are represented in red. Common microsatellite and genomic instability factors are represented in blue. Additional genomic alterations are represented in dark gray and marker of lymphocyte activation (IFNγ overexpression) is represented in green. Associations with others APOBEC members and lymphocyte markers are not shown in this diagram. Abbreviations. APOBEC = apolipoprotein B editing complex; CNV = copy number variations; MMR = mismatch repair; MSI = microsatellite instability; MSI-high = microsatellite instability high.
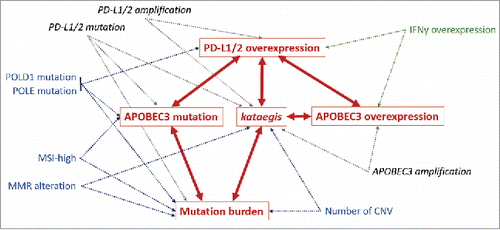
Independent predictors of tumor mutation burden
In univariate analysis, the factors positively associated with a high tumor mutation burden (i.e., total number of mutations per tumor greater than 66.5) were: MSI-H phenotype, MMR alteration (all MMR proteins were significant), POLD1 or POLE mutation, high number of CNV (gains, losses or combined factor), kataegis-signature, APOBEC3 mutation or amplification (all paralogs were significant), APOBEC3 overexpression (more specifically APOBEC3A/B/F/G overexpression), PD-1 ligand genomic mutation or amplification, PD-L1 overexpression (but not PD-L2), presence of a lymphocyte or monocyte infiltrate and IFNγ overexpression (Table S4).
In multivariate analysis, the overall tumor mutation burden was modeled with a pseudo-R2 of 30.3% (). Factors considered independent predictors for the presence of a high number of point mutations in a tumor included MSI-H status (OR = 54.7, p < 0.0001), MMR alteration (OR = 1.6, p < 0.0001), polymerases δ or ϵ catalytic subunits mutation (OR = 4.0, p = 0.0002 and OR = 5.7, p<0.0001, respectively), as well as kataegis-signature (OR = 14.1, p < 0.0001), AID/APOBEC family members mutation or amplification (OR ranging from 1.2 to 13.3, p < 0.0129) and a high number of CNV (more than 8,100 CNV per sample, OR = 2.0, p < 0.0001). Factors related to the immune modulation, like PD-1 ligand mutation (OR = 5.9, p = 0.0222), lymphocyte infiltration (OR = 1.4, p = 0.0005) or T-lymphocyte CD8A marker overexpression (OR = 1.4, p = 0.0214), were also linked to the tumor mutation burden (). Remarkably, neither APOBEC3 nor PD-L1 overexpression were associated with the total number of mutations in the multivariate model (p = 0.1226 and p = 0.2668, respectively). Similar results were obtained after application of the re-sampling method: all factors described above remained significant (1,000 replicates, p < 0.05), with the exception of the MSI-H phenotype and the presence of a lymphocyte infiltrate assessed by immunohistochemistry, for which the high number of missing values may induce a lack of reproducibility.
Table 2. Multivariate analysis of associationFootnotea between all factors and tumor mutational burden (model A) or kataegis-signature (model B).
Thus, this final model endorsed the widely known functions implicated in the tumor mutagenesis process, such as DNA mismatch repair, replicative polymerases and endogenous deamination, and confirmed the absence of independent correlation between PD-1 ligand overexpression and the overall tumor mutation burden ().
Independent predictors of kataegis (localized hyper-mutation caused by APOBEC)
The presence of APOBEC-related alterations (point mutations due to kataegis) was found in 1,604 tumors (18.9%). In this pan-cancer collection, the kataegis-signature was positively associated with MMR alteration, POLE mutation, overall mutation burden, total number of CNV, APOBEC3 or APOBEC4 mutation, AID/APOBEC amplification (of all 11 paralogs), APOBEC3 overexpression, PD-1 ligand alteration (mutation, amplification or overexpression), lymphocyte and monocyte infiltrate and overexpression of CD3G and MS4A1. In this case, MSI-H, APOBEC1/2/4 overexpression as well as NCAM1 (natural-killer cell marker) and IL3RA (dendritic cell marker) overexpression were negatively correlated with the presence of a kataegis-signature (Table S5).
Similarly to the tumor mutation burden, the presence of kataegis was modeled by multivariate analysis using a set of pre-defined combined factors, with a pseudo-R2 of 30.0%. The predictors of kataegis-signature were the presence of a MMR alteration (OR = 1.2, p = 0.0133), the overall mutation burden (OR = 14.1, p < 0.0001), a high number of CNV (OR = 1.4, p = 0.0005), APOBEC3 or APOBEC4 amplification (OR = 1.6, p < 0.0001 and OR = 1.4, p < 0.0001), APOBEC3 overexpression (OR = 1.6, p = 0.0016), the presence of a PD-1 ligand altered by mutation (OR = 2.8, p = 0.0124), amplification (OR = 1.3, p = 0.0070) or overexpression (OR = 1.4, p = 0.0099), and the presence of a lymphocyte infiltrate (OR = 1.2, p < 0.0001) (). All factors, except the correlation between the lymphocyte infiltrate and the kataegis-signature, were confirmed by the internal validation procedure (1,000 replicates, p < 0.05).
Such analysis allowed to confirm the existence of a bi-lateral relationship between PD-L1/2 expression and the presence of kataegis, along with a bi-directional relationship between kataegis and the overall tumor burden, as represented in the probabilistic graphical model in .
Independent predictors of APOBEC3 mRNA overexpression
A significant overexpression of APOBEC3 (including APOBEC3 isoforms A, B, C, D, F, G and H) was observed in 1,394 (16.4%) of the tumors. The factors associated with APOBEC3 overexpression in univariate analysis were: MMR altered (particularly MLH3 and MSH3), the number of genes lost and/or gained, the number of mutations as well as the number of kataegis-related mutations, APOBEC3C mutation, AICDA, APOBEC1 and APOBEC3 amplification, AICDA overexpression, PD-L1/2 amplification and overexpression, presence of a lymphocyte infiltrate with overexpression of lymphocyte markers CD3G, CD4+, CD8A, MS4A1, IL3RA, CD14 and CD33 and IFNγ overexpression (Table S6).
Independent factors significantly associated with APOBEC3 overexpression in multivariate analysis (pseudo-R2 = 14.0%) were the total number of APOBEC-related alterations (OR = 1.2, p = 0.0048), the overexpression of PD-1 ligand (OR = 2.6, p < 0.0001), amplification of APOBEC3 genes (OR = 2.3, p < 0.0001) or overexpression of AICDA (OR = 2.2, p < 0.0001), as well as expression of IFNγ (OR = 3.7, p < 0.0001) and lymphocyte markers (all excepted NCAM1 and CD14, OR = 1.5–3.5, p < 0.0131) (). All predictors for the model were confirmed by internal validation (1,000 replicates, p < 0.05), and bidirectional dependences between APOBEC3 overexpression and kataegis, plus APOBEC3 overexpression and PD-1 ligand overexpression, were inferred in the graphical model in .
Table 3. Multivariate analysis of associationFootnotea between all factors and APOBEC3 members (APOBEC3A/B/C/D/F/G/H) mRNA overexpression (model A) or mutation (model B).
Independent predictors of APOBEC3 mutation
Non-silent mutations of APOBEC3 were observed in 171 (2%) of the tumors. At the univariate level, the presence of a mutation in one of the 7 APOBEC3 paralogs was related to MSI-H phenotype, the presence of MMR alteration (MLH3, MSH2, MSH6 or PMS2 proteins), presence of mutagenesis process (overall mutation burden and kataegis), the presence of a non-silent mutation within POLD1, POLE, AICDA, APOBEC1, APOBEC2, APOBEC4 and PD-1 ligand genes, APOBEC4 amplification, PD-L1/2 overexpression and CD14 overexpression (Table S7).
The presence of a mutation in any of the APOBEC3 genes was modeled with a correlational pseudo-R2 of 20.1% by several positive independent predictors including MSI-H (OR = 5.4, p < 0.0001), high number of non-silent mutations (total number of mutations greater than 66.5, OR = 6.7, p < 0.0246), mutations within POLE, APOBEC1, APOBEC2 and PD-L1/2 (OR ranging from 3.0 to 6.4, p < 0.0001), and overexpression of PD-1 ligands (OR = 2.0, p = 0.0067) (). These predictors, with the exception of MSI-H status, were confirmed by the bootstrapping method (1,000 replicates, p < 0.05) and added to the graphical model in .
Graphical model of prediction of PD-1 ligand overexpression
In fine, observed data-based inferences were implemented in a directed graphical model, representing the conceptual framework of conditional relationships between all factors-of-interest ().Citation28 In such representation, each factor constitutes a node, and each node may or may not be connected to each other, in regards of the results obtained in the series of logistic regression models defined above. Reciprocals effects (also called bivariate correlations)—observed between PD-1 ligand overexpression, kataegis signature, APOBEC3 mutation, APOBEC3 overexpression and tumor mutation burden—were symbolized by bidirectional plain arrows. Others dependence relationships, not validated for their reciprocity (e.g., predictive relationship observed between IFNγ overexpression and PD-1 ligand overexpression) were symbolized by dotted-line arrows.
Discussion
PD-L1 immunohistochemistry positive staining of tumor cells has emerged as a significant, though imperfect, predictive feature for response to immune checkpoint blockade mediated by agents, such as nivolumab, pembrolizumab or atezolizumab.Citation12,13,29 Recently, several publications have reported a correlation between the presence of a large number of mutations (also called tumor mutation burden) and the expression of PD- L1.Citation17,19 The aim of this study was to clarify, using a large collection of samples, the molecular and cellular factors associated with the overexpression of PD-1 ligands in tumor cells, especially as it pertains to the mechanisms leading to an accumulation of mutations in such cells, and this, independently of the cancer subtype.
While an association between mutation burden and increased PD-L1 or PD-L2 expression was confirmed in our pan-cancer dataset in univariate analysis, we show that, in multivariate analysis, PD-L1 and PD-L2 overexpression appears to be rather highly and independently correlated with kataegis—a specific localized hyper-mutational process caused by the AID/APOBEC family of enzymes—as well as with overexpression of APOBEC3 members ( and ). These results are consistent with the observation recently described in urothelial carcinoma, where the expression of APOBEC3F was associated with the expression of PD-L1 in tumor cells, while the expression of APOBEC3A, APOBEC3D and APOBEC3H was associated with the expression of PD-L1 in tumor infiltrating mononuclear cells.Citation20
The mechanisms by which APOBEC3 and PD-L1/2 overexpression are correlated may be explainable by several hypotheses (). First, a viral infection may result in both overexpression of APOBEC3 and PD-1 ligands (). The pathogen entry induces the secretion of cytokines (such as IFNγ) by immune cells in virally infected hosts, which in turn can increase both APOBEC3 and PD-L1 expression via transcriptional regulation.Citation9,30 De facto, in our study, a strong positive association was found between IFNγ and PD-1 ligand expression (OR = 2.6, p < 0.0001), as well as between IFNγ and APOBEC3 expression (OR = 3.7, p < 0.0001). However, if the induction of APOBEC3 enzymes in response to a pathogen appears to be a mechanism of host defense (by interfering with proper replication of retroviruses), the induction of PD-1 ligand seems rather counter-intuitive as favoring immune tolerance and viral persistence. Indeed, the paradoxical overexpression of immune checkpoints has been described as a trigger of T-lymphocyte exhaustion after chronic pathogen exposureCitation31 and a mechanism of tissue protection against early T-cell mediated damaging inflammatory events in various human non-infectious diseases and animal models.Citation32,33 Also, one may think that upregulation of PD-L1 represents a second-step in the viral invasion mechanism, producing conditions that allow the virus natural reservoir's persistence and continuous replication, and therefore, evading the host immune response. Indeed, it has been recently shown that viruses themselves can disrupt the 3′ region of the PD-L1 gene resulting in a marked elevation of PD-L1 transcripts.Citation11 Another hypothesis to explain the concurrent overexpression of PD-1 ligand and APOBEC3 enzymes could relate to the existence of a common signaling cascade, distinct from infectious processes (). Initially, APOBEC3 members were called “phorbolins,” as their expression could be induced by the plant-derived compounds called phorbols.Citation34 Interestingly, phorbol esters (e.g., phorbol-12-myristate-13-acetate (PMA), 12-O-Tetradecanoylphorbol-13-acetate (TPA)), are potent activators of the protein kinase C (PKC) used in biomedical research to promote carcinogenesis or stimulate T-cell activation, and have also been described as inducers of PD-1 ligand expression.Citation35 Phorbols are tumor promoters that induce malignant transformation when associated with other carcinogens. They are considered as indirect DNA damaging agents by formation of superoxide radicalsCitation36 and thus may amplify the hyper-mutation signature observed in certain tumors. A third hypothesis that would explain the association observed between overexpression of APOBEC3 and PD-1 ligands could be based on a selection concept (). If cells exhibiting high levels of mutation burden fail to upregulate PD-L1, they may be eliminated and hence not observed. As APOBEC enzymes and PD-1 ligands share the same pattern of expression, the latter offers the possibility for tumor cells harboring localized hyper-mutation patterns to escape the immune system. Of interest in this regard, recent data suggests that the relationship between prognosis and genomic instability as reflected by copy number alterations and mutation clonality in tumor cells is an inverted U shape—those individuals harboring tumors with the lowest and highest genomic instability do better than those with intermediate levels.Citation37 The latter could be due to the fact that, when a cell presents a high genomic instability—regardless of the mechanism of mutagenesis— this highly modified genotype generates multiple neo-antigens, which leads to the activation of the immune system and the subsequent elimination of the cell. The only cells persisting in this setting are those producing factors such as PD-L1, allowing them to successfully evade immune elimination and thus form a tumor.Citation38,39
Figure 3. Causal hypothesis of mutation burden and immune tolerance co-occurrence in human tumors. Pathogen concept (Part A). Both PD-1 ligand and APOBEC enzymes are induced after viral infection, via interferon gamma (IFNγ) secretion. The defensive anti-viral function of APOBEC3 results in off-target alterations within the host genome, which can be repaired by DNA repair processes, unless alteration of these functions. Carcinogen concept (Part B). Both PD-1 ligand and APOBEC enzymes are regulated by the same factors, like exogenous activators. PKC has been linked to APOBEC3 and PD-L1 overexpression in distinct studies.Citation35 PKC can be activated by exogenous substances such as phorbol, which acts as a co-carcinogen and T-lymphocyte activator.Citation34 Clonal selection concept (Part C). Co-occurrence of kataegis-mutation process and expression of immune checkpoints such as PD-L1/2 leads to the accumulation of mutations and the tumor progression. In the presence of high level of mutation burden, only tumors that overexpress PD-L1 (and hence evade the immune system) survive. Other mechanisms of immune escape, not studied herein, could also be surgical. Abbreviations. APOBEC = apolipoprotein B editing complex; MMR = mismatch repair.
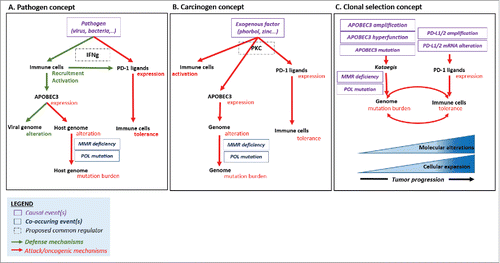
Interestingly, mutations within APOBEC3 genes appeared to be independently associated with PD-1 ligand overexpression along with, but not directly correlated with, APOBEC3 overexpression (). The exact mechanism linking the presence of mutations within APOBEC genes and the high expression level of PD-1 ligand remains to be elucidated, but suggests either a cause-and-effect relationship between the APOBEC enzyme functions and the expression of PD-1 ligands, and/or supports the hypothesis that the observation of tumor cells concurrently harboring DNA damaging mutations and immune tolerance markers may reflect positive selection of tumor cells, as proposed by the clonal-evolution model (). While a recent study reported an enrichment of kataegis-related mutation pattern in tumors presenting common APOBEC3 variants,Citation40 our analysis shown that APOBEC3 mutations were linked to the total number of mutations in the tumor (), and the latter and kataegis were associated with each other (). APOBEC3 mutations were also independently correlated with MSI, MMR alterations and POLE mutations (). All these independent mutagenesis mechanisms contribute to the accumulation of genomic alterations in tolerated tumor cells and may explain, albeit perhaps not independently, the association observed between the high mutation burden and PD-L1 positive tumors.
As mentioned above, although tumor mutation burden correlated with PD-L1/2 ligand overexpression in univariate analysis, the overall mutation burden factor was not retained in our final multivariate model. Previous reports have described an independent relationship between PD-L1 expression and the number of mutations presented by a tumor,Citation15,16,19 but APOBEC expression was not evaluated in these studies. Our results suggest that the factors that most significantly and independently correlated with PD-L1/2 overexpression relate to APOBEC dysregulation and the resulting kataegis mutational signature; and these factors are in turn independently linked to the overall mutation burden (). In this study, an additional factor independently related to PD-1 ligand overexpression was the presence of a mutation within the POLD1 gene, encoding the catalytic subunit of polymerase δ and likewise involved in tumor genomic instability.Citation41 However, POLD1 mutations represent a rare tumor event (1.2% of all tumors) and the related POLE mutations were not associated with PD-L1 and/or PD-L2 overexpression in the multivariate analysis.
All predictive factors defined in our study have been extracted from a large pan-cancer cohort, regardless of the tumor histology. One might suggest evaluation of those markers among different diagnoses, as the genomic backgrounds and mutagenesis process exposures may differ between them (e.g., effect of UV radiation in melanoma, tobacco-related carcinogens in lung cancer, human papillomavirus in cervical cancer). Also, the rather low frequency of some of our variables (e.g., PD-1 ligand overexpression and APOBEC3 mutation are present in 5.5% and 2% of the tumors, respectively) may legitimately raise concerns regarding the goodness-of-fit of the described regression models. We specifically used statistical methods adapted to rare events, such as the Firth's penalized-likelihood regression and internally evaluated the performance of our predictive models by using a re-sampling method, generating regression estimates for 1,000 replicates of n = 8,475 sample-size sets. Although the main conclusions of the study require external validation on an independent cohort, internal validation of our logistic models confirmed the key relationships existing between PD-1 ligands expression and all factors entered. Three factors, however, did not show a significant correlation when assessed by the bootstrapping method, despite their initial description in the primary models. These factors, namely the MSI phenotype, lymphocyte and macrophage infiltrate estimators, were evaluated only in specific tumor types and, therefore, exhibited a high rate of missing values (84.3%, 27.1% and 29%, respectively).
In conclusion, our study confirms the strong association between the expression of immune checkpoints and the presence of a high level of DNA instability, represented not only by the overall number of mutations, but more importantly by the unleashed activity of APOBEC enzymes. The observation of APOBEC3 variants (and not just APOBEC3 mRNA overexpression) independently associated with the overexpression of PD-1 ligands constitutes an argument in favor of a causative relationship. The concurrent observation of APOBEC function dysregulation and immune checkpoint expression may also be evidence for a positive selection process, by which late-stage tumors that have accumulated a high load of kataegis-related mutations are able to evade the immune system. It is already known that high tumor mutation burden, as well as PD-L1 overexpression, can correlate with better response to inhibitors of the PD1/PD-L1 axis.Citation18 But the causative origin of such mutation burden, the relationship between these 2 factors, as well as the evaluation of AID/APOBEC markers by assessment of activity, genomic mutation and/or mRNA expression in human tumors merit a deeper investigation. Finally, the search for biomarkers that predict response is a central focus of the checkpoint inhibitor field.Citation42 Once activated, it is crucial to know precisely, which neo-antigens are recognized by the immune system. Tumor mutational burden has emerged as an important predictor of response to immunotherapy, but mainly because a high number of mutations increases the likelihood of generating a neo-antigen which elicits immune reaction. However, it is clear that not all patients with high tumor mutational burden respond, and it is, therefore, crucial to determine the specific subset of mutations (and hence specific subset of neo-antigens) that elicit an immune reaction. As a kataegis-signature is found in more than 18.9% of the TCGA pan-cancer cohort, APOBEC alterations and the related kataegis-specific mutational load merits investigation in this regard, especially because of their association with high PD-L1 expression and viral infections.
Material and methods
Data retrieval and processing
Mutation, copy number variation, mRNA expression, protein expression, molecular phenotype (MSI and mutation burden estimates) data were downloaded from the community resource project TCGA, using the Broad GDAC Firehose website (https://gdac.broadinstitute.org/—standardized data run release 2016_01_28). A total of 11,245 samples corresponding to unique patients and related to 33 distinct tumor types were curated. All samples were published and available without restriction of use on the date of May, 1st 2016. All data used in this study respected the TCGA's Human Subjects Protection and Data Access Policies (https://cancergenome.nih.gov/abouttcga/policies/tcga-human-subjects-data-policies).
Whole-genome level 2 somatic mutation data were available for 9,103 tumor samples (all cohorts except mesothelioma), and lists of significant variants were generated using the MutSig2CV algorithm (http://www.broadinstitute.org/cancer/cga/mutsig), taking into account the somatic background mutation rate for each gene as well as neighbor genes.Citation43 All non-synonymous missense, nonsense, non-stop, del/insertions, frameshift or splicing site mutations within the genes of interest (described in Table S2) were kept for the analysis.
Focal CNV corresponding to level 3 genome-wide single nucleotide polymorphism (SNP) array data were normalized and assessed at the gene-level using the GISTIC2 protocolCitation44 (http://www.broadinstitute.org/tcga/about), where a “deep loss” was documented by the value (−2), a “single-copy loss” by the value (−1), a “low-level gain” by the value (+1) and an “amplification” by the value (+2). Both levels of losses or gains were integrated without distinction in the further analysis. A total of 10,845 tumor samples related to 33 cohorts were thereby implemented.
The sequencing-based mRNA expression (RNAseqV2, level 3) was available for 10,261 tumor samples (33 cohorts). Expression signals were integrated and normalized for each gene, per sample, using the protocol RSEM (RNA-Sequencing by Expectation Maximization).Citation45 The standard score (z-score) for each gene per sample was calculated using the mean and standard deviation of values found in all similar tumors (same tumor type) that are diploid for the said gene. A z-score ≥2 standard deviations was used as threshold of overexpression, whereas a threshold ≤(−2) standard deviations was used to qualify underexpressed genes.
Microsatellite stability status was available for 1,909 samples, distributed in 7 cohorts (colon and rectal carcinoma, esophageal carcinoma, pancreatic adenocarcinoma, stomach adenocarcinoma, uterine corpus endometrial carcinoma and uterine carcinosarcoma). Tumors were designated as MSI-H, if there was instability in at least 3 of 7 markers (mononucleotides microsatellites BAT25, BAT26, BAT40, TGFBR2 and dinucleotides microsatellites D2S123, D5S346, D17S250), as reflected by fragment analysis of tumor compared with normal tissue.
The total number of CNV (estimated in number of genes lost and number of genes gained), overall number of mutations and number of mutations related to APOBEC (i.e., respecting the TpCpW APOBEC mutation pattern and not due to a random mutagenesis) were retrieved from the Broad GDAC Firehose website and correspond to analyses performed in August 2015 with the P-MACD (Pattern of Mutagenesis by APOBEC Cytidine Deaminases) calculation process.Citation21,46
MLH1, MSH1, MSH6 and PMS2 protein immunostaining as well as lymphocyte and macrophage infiltration estimates obtained by hematoxylin and eosin staining (H&E) were downloaded from the NCI TCGA Data Portal interface (https://tcga-data.nci.nih.gov/tcga/tcgaHome2.jsp), and were available for 479; 7,774 and 7,514 samples, respectively.
Study design
A pan-cancer cohort including all unique samples presenting complete mutations, CNV and mRNA expression data description as processed above was used for the analysis (n = 8,475). Missing values for microsatellite status and lymphocyte/macrophage infiltrate variables were categorized as “unknown” and included in the statistics. Factors of interest were sub-grouped by biological function, as shown in Table S2.
Statistical analysis
Association of all factors with PD-1 ligand overexpression (modeling the overexpression of either PD-L1 or PD-L2), the overall tumor mutation burden (modeling the presence of a high number of mutations, independently from their mechanism of origin), kataegis estimate (modeling the specific mutational activity of APOBEC members), APOBEC3 paralogs overexpression (modeling the overexpression of members APOBEC3A/B/C/D/F/G/H), or APOBEC3 paralogs mutations (modeling the presence of a mutation within the genes APOBEC3A/B/C/D/F/G/H) was assessed by univariate analysis using a Fisher's exact test. A logistic regression based on all factors presenting a two-tailed p value ≤0.25 in the univariate analysis was run to assess the best set of predictors for each dependent factor. The Firth's penalized-likelihood methodCitation47 (addressing issues of quasi-complete separation of data and low frequency events, as observed in this cohort) was used for model fitting, using both single factors and combined sets of co-variates. A backward elimination selection procedure Citation48 was run until all significant factors were found; a p value ≤0.05 was considered statistically significant for final conclusions. The goodness-of-fit was assessed for each model by the McFadden's pseudo-R2 (representing the percentage of outcome's variability that is accounted for by the particular set of variables), re-scaled from 0% to 100% for ease of interpretation.Citation27 Internal validation of the logistic regression models was conducted after bootstrapping of the initial cohort (random re-sampling with replacement method, generating 1,000 replicates of 8,475 sample-size sets).Citation49 A graphical model expressing conditional dependences between variables was built using the series of regression models obtained.Citation28
All statistical analyses were conducted by AB using SAS® University Edition software (http://support.sas.com/software/products/university-edition/).
Disclosure of potential conflicts of interest
RK has research funding from Genentech, Merck Serono, Pfizer, Sequenom, Foundation Medicine, and Guardant, as well as consultant fees from Sequenom and Actuate Therapeutics and an ownership interest in Novena, Inc. and CureMatch, Inc. IT has an ownership interest in CureMatch, Inc.
Author contributions
Conception and design: AB, RK, IT. Development of methodology: AB, RK, IT. Acquisition of data: AB. Analysis and interpretation of data: AB, RK. Writing, review and revision of the manuscript: AB, RK. Editing and approval of final version: AB, IT, RK.
Data and materials availability
All the data used in this article were downloaded from the community resource project TCGA (https://gdac.broadinstitute.org/—standardized data run release 2016_01_28) and were available without restriction of use on the date of May 1, 2016. All analysis results are shown in Supplementary Materials.
KONI_A_1284719_Supplemental_Tables1-7.docx
Download MS Word (143.6 KB)Funding
This study was funded in part by National Cancer Institute grant P30CA016672 and the Joan and Irwin Jacobs Philanthropic Fund.
References
- Dudley ME, Wunderlich JR, Robbins PF, Yang JC, Hwu P, Schwartzentruber DJ, Topalian SL, Sherry R, Restifo NP, Hubicki AM et al. Cancer regression and autoimmunity in patients after clonal repopulation with antitumor lymphocytes. Science 2002; 298:850-4; PMID:12242449; http://dx.doi.org/10.1126/science.1076514
- Pardoll DM. The blockade of immune checkpoints in cancer immunotherapy. Nat Rev Cancer 2012; 12:252-64; PMID:22437870; http://dx.doi.org/10.1038/nrc3239
- Topalian SL, Sznol M, McDermott DF, Kluger HM, Carvajal RD, Sharfman WH, Brahmer JR, Lawrence DP, Atkins MB, Powderly JD, et al. Survival, durable tumor remission, and long-term safety in patients with advanced melanoma receiving nivolumab. J Clin Oncol 2014; 32:1020-30; PMID:24590637; http://dx.doi.org/10.1200/JCO.2013.53.0105
- Ansell SM, Lesokhin AM, Borrello I, Halwani A, Scott EC, Gutierrez M, Schuster SJ, Millenson MM, Cattry D, Freeman GJ et al. PD-1 blockade with nivolumab in relapsed or refractory Hodgkin's lymphoma. N Engl J Med 2015; 372:311-9; PMID:25482239; http://dx.doi.org/10.1056/NEJMoa1411087
- Motzer RJ, Rini BI, McDermott DF, Redman BG, Kuzel TM, Harrison MR, Vaishampayan UN, Drabkin HA, George S, Logan TF et al. Nivolumab for metastatic renal cell carcinoma: results of a randomized phase II trial. J Clin Oncol 2015; 33:1430-7; PMID:25452452; http://dx.doi.org/10.1200/JCO.2014.59.0703
- Brahmer J, Reckamp KL, Baas P, Crinò L, Eberhardt WEE, Poddubskaya E, Antonia S, Pluzanski A, Vokes EE, Holgado E et al. Nivolumab versus Docetaxel in Advanced Squamous-Cell Non-Small-Cell Lung Cancer. N Engl J Med 2015; 373:123-35; PMID:26028407; http://dx.doi.org/10.1056/NEJMoa1504627
- Rosenberg JE, Hoffman-Censits J, Powles T, van der Heijden MS, Balar AV, Necchi A, Dawson N, O'Donnell PH, Balmanoukian A,Loriot Y et al. Atezolizumab in patients with locally advanced and metastatic urothelial carcinoma who have progressed following treatment with platinum-based chemotherapy: a single-arm, multicentre, phase 2 trial. Lancet 2016; 387:1909-20; PMID:26952546; http://dx.doi.org/10.1016/S0140-6736(16)00561-4
- Goodman A, Patel SP, Kurzrock R. PD-1-PD-L1 immune-checkpoint blockade in B-cell lymphomas. Nat Rev Clin Oncol 2016; advance online publication; PMID:27805626; http://dx.doi.org/10.1038/nrclinonc.2016.168
- Ritprajak P, Azuma M. Intrinsic and extrinsic control of expression of the immunoregulatory molecule PD-L1 in epithelial cells and squamous cell carcinoma. Oral Oncol 2015; 51:221-8; PMID:25500094; http://dx.doi.org/10.1016/j.oraloncology.2014.11.014
- Twa DDW, Chan FC, Ben-Neriah S, Woolcock BW, Mottok A, Tan KL, Slack GW, Gunawardana J, Lim RS, McPherson AW et al. Genomic rearrangements involving programmed death ligands are recurrent in primary mediastinal large B-cell lymphoma. Blood 2014; 123:2062-5; PMID:24497532; http://dx.doi.org/10.1182/blood-2013-10-535443
- Kataoka K, Shiraishi Y, Takeda Y, Sakata S, Matsumoto M, Nagano S, Maeda T, Nagata Y, Kitanaka A, Mizuno S et al. Aberrant PD-L1 expression through 3′-UTR disruption in multiple cancers. Nature 2016; 534:402-6; PMID:27281199; http://dx.doi.org/10.1038/nature18294
- Patel SP, Kurzrock R. PD-L1 expression as a predictive biomarker in cancer immunotherapy. Mol Cancer Ther 2015; 14:847-56; PMID:25695955; http://dx.doi.org/10.1158/1535-7163.MCT-14-0983
- Taube JM, Klein A, Brahmer JR, Xu H, Pan X, Kim JH, Chen L, Pardoll DM, Topalian SL, Anders RA. Association of PD-1, PD-1 ligands, and other features of the tumor immune microenvironment with response to anti-PD-1 therapy. Clin Cancer Res 2014; 20:5064-74; PMID:24714771; http://dx.doi.org/10.11581078-0432.CCR-13-3271
- Roemer MGM, Advani RH, Ligon AH, Natkunam Y, Redd RA, Homer H, Connelly CF, Sun HH, Daadi SE, Freeman GJ et al. PD-L1and PD-L2 genetic alterations define classical hodgkin lymphoma and predict outcome. J Clin Oncol 2016; 34:2690-7; PMID:27069084; http://dx.doi.org/10.1200/JCO.2016.66.4482
- Le DT, Uram JN, Wang H, Bartlett BR, Kemberling H, Eyring AD, Skora AD, Luber BS, Azad NS, Laheru D et al. PD-1 blockade in tumors with mismatch-repair deficiency. N Engl J Med 2015; 372:2509-20; PMID:26028255; http://dx.doi.org/10.1056/NEJMoa1500596
- Lal N, Beggs AD, Willcox BE, Middleton GW. An immunogenomic stratification of colorectal cancer: implications for development of targeted immunotherapy. Oncoimmunology 2015; 4(3):e976052; PMID:25949894; http://dx.doi.org/10.4161/2162402X.2014.976052
- Madore J, Strbenac D, Vilain R, Menzies AM, Yang JYH, Thompson JF, Long GV, Mann GJ, Scolyer RA, Wilmott JS. PD-L1 negative status is associated with lower mutation burden, differential expression of immune-related genes, and worse survival in stage III melanoma. Clin Cancer Res 2016; 22:3915-23; PMID:26960397; http://dx.doi.org/10.1158/1078-0432.CCR-15-1714
- Rizvi NA, Hellmann MD, Snyder A, Kvistborg P, Makarov V, Havel JJ, Lee W, Yuan J, Wong P, Ho TS et al. Cancer immunology. Mutational landscape determines sensitivity to PD-1 blockade in non-small cell lung cancer. Science 2015; 348:124-8; PMID:25765070; http://dx.doi.org/10.1126/science.aaa1348
- Howitt BE, Shukla SA, Sholl LM, Ritterhouse LL, Watkins JC, Rodig S, Stover E, Strickland KC, D'Andrea AD, Wu CJ et al. Association of polymerase e-mutated and microsatellite-instable endometrial cancers with neoantigen load, number of tumor-infiltrating lymphocytes, and expression of PD-1 and PD-L1. JAMA Oncol 2015; 1:1319-23; PMID:26181000; http://dx.doi.org/10.1001/jamaoncol.2015.2151
- Mullane SA, Werner L, Rosenberg J, Signoretti S, Callea M, Choueiri TK, Freeman GJ, Bellmunt J. Correlation of Apobec Mrna expression with overall survival and pd-l1 pxpression in urothelial carcinoma. Sci Rep 2016; 6:27702; PMID:27283319; http://dx.doi.org/10.1038/srep27702
- Alexandrov LB, Nik-Zainal S, Wedge DC, Aparicio SAJR, Behjati S, Biankin AV, Bignell GR, Bolli N, Borg A, Børresen-Dale A-L et al. Signatures of mutational processes in human cancer. Nature 2013; 500:415-21; PMID:23945592; http://dx.doi.org/10.1038/nature12477
- Burns MB, Temiz NA, Harris RS. Evidence for APOBEC3B mutagenesis in multiple human cancers. Nat Genet 2013; 45:977-83; PMID:23852168; http://dx.doi.org/10.1038/ng.2701
- Chen SH, Habib G, Yang CY, Gu ZW, Lee BR, Weng SA, Silberman SR, Cai SJ, Deslypere JP, Rosseneu M et al. Apolipoprotein B-48 is the product of a messenger RNA with an organ-specific in-frame stop codon. Science 1987; 238:363-6; PMID:3659919; http://dx.doi.org/10.1126/science.3659919
- Ramiro A, San-Martin BR, McBride K, Jankovic M, Barreto V, Nussenzweig A, Nussenzweig MC. The role of activation-induced deaminase in antibody diversification and chromosome translocations. Adv Immunol. 2007; 94:75-107; PMID:17560272; http://dx.doi.org/10.1016/S0065-2776(06)94003-6
- Cullen BR. Role and mechanism of action of the APOBEC3 family of antiretroviral resistance factors. J Virol 2006; 80:1067-76; PMID:16414984; http://dx.doi.org/10.1128/JVI.80.3.1067-1076.2006
- Smith HC, Bennett RP, Kizilyer A, McDougall WM, Prohaska KM. Functions and regulation of the APOBEC family of proteins. Semin Cell Dev Biol 2012; 23:258-68; PMID:22001110; http://dx.doi.org/10.1016/j.semcdb.2011.10.004
- McFadden D, others. Conditional logit analysis of qualitative choice behavior. 1973 [ cited 2016 Sep 3]; Available from: https://elsa.berkeley.edu/reprints/mcfadden/zarembka.pdf
- Koller D, Friedman N. Probabilistic Graphical Models: Principles and Techniques. Cambridge, MA: MIT Press; 2009.
- Brahmer JR, Tykodi SS, Chow LQM, Hwu W-J, Topalian SL, Hwu P, Drake CG, Camacho LH, Kauh J, Odunsi K et al. Safety and activity of anti-PD-L1 antibody in patients with advanced cancer. N Engl J Med 2012; 366:2455-65; PMID:22658128; http://dx.doi.org/10.1056/NEJMoa1200694
- Argyris EG, Acheampong E, Wang F, Huang J, Chen K, Mukhtar M, Zhang H. The interferon-induced expression of APOBEC3G in human blood-brain barrier exerts a potent intrinsic immunity to block HIV-1 entry to central nervous system. Virology 2007; 367:440-51; PMID:17631933; http://dx.doi.org/10.1016/j.virol.2007.06.010
- Hofmeyer KA, Jeon H, Zang X. The PD-1/PD-L1 (B7-H1) pathway in chronic infection-induced cytotoxic T lymphocyte exhaustion. J Biomed Biotechnol 2011; 2011:451694; PMID:21960736; http://dx.doi.org/10.1155/2011/451694
- Waeckerle-Men Y, Starke A, Wuthrich RP. PD-L1 partially protects renal tubular epithelial cells from the attack of CD8+ cytotoxic T cells. Nephrol Dial Transplant 2007; 22:1527-36; PMID:17339272; http://dx.doi.org/10.1093/ndt/gfl818
- Wang C-J, Chou F-C, Chu C-H, Wu J-C, Lin S-H, Chang D-M, Sytwu H-K. Protective role of programmed death 1 ligand 1 (PD-L1)in nonobese diabetic mice: the paradox in transgenic models. Diabetes 2008; 57:1861-9; PMID:18420489; http://dx.doi.org/10.2337/db07-1260
- Madsen P, Anant S, Rasmussen HH, Gromov P, Vorum H, Dumanski JP, Tommerup N, Collins JE, Wright CL, Dunham I et al. Psoriasis upregulated phorbolin-1 shares structural but not functional similarity to the mRNA-editing protein apobec-1. J Invest Dermatol 1999; 113:162-9; PMID:10469298; http://dx.doi.org/10.1046/j.1523-1747.1999.00682.x
- Jang B-C, Park Y-K, Choi I-H, Kim S-P, Hwang J-B, Baek W-K, Suh M-H, Mun K-C, Suh S-I. 12-O-tetradecanoyl phorbol 13-acetate induces the expression of B7-DC, -H1, -H2, and -H3 in K562 cells. Int J Oncol 2007; 31:1439-47; PMID:17982670; http://dx.doi.org/10.3892/ijo.31.6.1439
- Emerit I, Cerutti PA. Tumour promoter phorbol-12-myristate-13-acetate induces chromosomal damage via indirect action. Nature 1981; 293:144-6; PMID:7266668; http://dx.doi.org/10.1038/293144a0
- Andor N, Graham TA, Jansen M, Xia LC, Aktipis CA, Petritsch C, Ji HP, Maley CC. Pan-cancer analysis of the extent and consequences of intratumor heterogeneity. Nat Med 2016; 22:105-13; PMID:26618723; http://dx.doi.org/10.1038/nm.3984
- Greaves M, Maley CC. Clonal evolution in cancer. Nature 2012; 481:306-13; PMID:22258609; http://dx.doi.org/10.1038/nature10762
- Swanton C, McGranahan N, Starrett GJ, Harris RS. APOBEC Enzymes: mutagenic fuel for cancer evolution and heterogeneity. Cancer Discov 2015; 5:704-12; PMID:26091828; http://dx.doi.org/10.1158/2159-8290.CD-15-0344
- Middlebrooks CD, Banday AR, Matsuda K, Udquim K-I, Onabajo OO, Paquin A, Figueroa JD, Zhu B, Koutros S, Kubo M et al. Association of germline variants in the APOBEC3 region with cancer risk and enrichment with APOBEC-signature mutations in tumors. Nat Genet 2016; 48:1330-8; PMID:27643540; http://dx.doi.org/10.1038/ng.3670
- Briggs S, Tomlinson I. Germline and somatic polymerase ϵ and δ mutations define a new class of hypermutated colorectal and endometrial cancers. J Pathol 2013; 230:148-53; PMID:23447401; http://dx.doi.org/10.1002/path.4185
- Khagi Y, Kurzrock R, Patel SP. Next generation predictive biomarkers for immune checkpoint inhibition. Cancer Metastasis Rev 2016; pp 1-12; PMID:27873079; http://dx.doi.org/10.1007/s10555-016-9652-y
- Lawrence MS, Stojanov P, Polak P, Kryukov GV, Cibulskis K, Sivachenko A, Carter SL, Stewart C, Mermel CH, Roberts SA et al. Mutational heterogeneity in cancer and the search for new cancer-associated genes. Nature 2013; 499:214-8; PMID:23770567; http://dx.doi.org/10.1038/nature12213
- Beroukhim R, Getz G, Nghiemphu L, Barretina J, Hsueh T, Linhart D, Vivanco I, Lee JC, Huang JH, Alexander S et al. Assessing the significance of chromosomal aberrations in cancer: methodology and application to glioma. Proc Natl Acad Sci USA 2007; 104:20007-12; PMID:18077431; http://dx.doi.org/10.1073/pnas.0710052104
- Li B, Dewey CN. RSEM: accurate transcript quantification from RNA-Seq data with or without a reference genome. BMC Bioinform 2011; 12:323; PMID:21816040; http://dx.doi.org/10.1186/1471-2105-12-323
- Roberts SA, Lawrence MS, Klimczak LJ, Grimm SA, Fargo D, Stojanov P, Kiezun A, Kryukov GV, Carter SL, Saksena G et al. An APOBEC cytidine deaminase mutagenesis pattern is widespread in human cancers. Nat Genet 2013; 45:970-6; PMID:23852170; http://dx.doi.org/10.1038/ng.2702
- Wang X. Firth logistic regression for rare variant association tests. Front Genet 2014; 5:187; PMID:24995013; http://dx.doi.org/10.3389/fgene.2014.00187
- Heinze G, Puhr R. Bias-reduced and separation-proof conditional logistic regression with small or sparse data sets. Stat Med 2010; 29:770-7; PMID:20213709; http://dx.doi.org/10.1002/sim.3794
- Steyerberg EW, Harrell FE, Borsboom GJ, Eijkemans MJ, Vergouwe Y, Habbema JD. Internal validation of predictive models: efficiency of some procedures for logistic regression analysis. J Clin Epidemiol 2001; 54:774-81; PMID:11470385; http://dx.doi.org/10.1016/S0895-4356(01)00341-9