ABSTRACT
Most human blood γδ cells are cytolytic TCRVγ9Vδ2+ lymphocytes with antitumor activity. They are currently investigated in several clinical trials of cancer immunotherapy but so far, their tumor infiltration has not been systematically explored across human cancers. Novel algorithms allowing the deconvolution of bulk tumor transcriptomes to find the relative proportions of infiltrating leucocytes, such as CIBERSORT, should be appropriate for this aim but in practice they fail to accurately recognize γδ T lymphocytes. Here, by implementing machine learning from microarray data, we first improved the computational identification of blood-derived TCRVγ9Vδ2+ γδ lymphocytes and then applied this strategy to assess their abundance as tumor infiltrating lymphocytes (γδ TIL) in ∼10,000 cancer biopsies from 50 types of hematological and solid malignancies. We observed considerable inter-individual variation of TCRVγ9Vδ2+γδ TIL abundance both within each type and across the spectrum of cancers tested. We report their prominence in B cell-acute lymphoblastic leukemia (B-ALL), acute promyelocytic leukemia (M3-AML) and chronic myeloid leukemia (CML) as well as in inflammatory breast, prostate, esophagus, pancreas and lung carcinoma. Across all cancers, the abundance of αβ TILs and TCRVγ9Vδ2+ γδ TILs did not correlate. αβ TIL abundance paralleled the mutational load of tumors and positively correlated with inflammation, infiltration of monocytes, macrophages and dendritic cells (DC), antigen processing and presentation, and cytolytic activity, in line with an association with a favorable outcome. In contrast, the abundance of TCRVγ9Vδ2+ γδ TILs did not correlate with these hallmarks and was variably associated with outcome, suggesting that distinct contexts underlie TCRVγ9Vδ2+ γδ TIL and αβ TIL mobilizations in cancer.
Introduction
In healthy human adults, circulating T lymphocytes comprise ∼1–3% of γδ T cells, most of which express TCRVγ9Vδ2, and mediate Th1 and cytolytic activities in response to stimulation by soluble phosphoantigens (PAg) or cancer cells.Citation1-3 Hence, this subset of T lymphocytes represent promising effectors for cancer immunotherapy, but the few γδ cell-based trials performed so far have proven difficult to interpret due to a lack of biomarkers for the stratification of patients.Citation4-7 Notably, the detection of tumor infiltrating γδ T lymphocytes (γδ TIL) has been hampered by their controversial detection by immuno-histochemical (IHC) methods due to their unreliable recognition by antibodies.
Alternative options would be to enumerate γδ TIL from gene expression profiles (GEP), which is now possible due to recently developed computational methodologies that can perform cell type-specific quantifications from the transcriptomic data of bulk samples (for review, seeCitation8). For example, the “deep deconvolution” CIBERSORT algorithm uses the transcriptome profile of reference leucocyte cell types to define a reference gene signature matrix which it then uses to deduce the leucocyte composition from the transcriptome of a tissue.Citation9 Using CIBERSORT, the Alizadeh group were able to determine the relative proportions of 22 subsets of leucocytes that had infiltrated tumors from 30,000 transcriptomes representing 39 distinct malignancies, and identified γδ TILs as the most significant favorable cancer prognostic cell population.Citation10 However, we show that applying CIBERSORT to pure γδ T cell transcriptomes failed to correctly differentiate these cells from αβ T CD4+, CD8+ and natural killer (NK) cells. Here we optimized this deconvolution and applied it to enumerate γδ TIL in human cancers.
Results
Optimization of CIBERSORT deconvolution for inferring γδ T cell abundance from a transcriptome
We first applied the CIBERSORT algorithm and its LM22 gene signature to determine the fraction of γδ T cells from the GEP of 12 well-characterized samples of TCRVγ9Vδ2+ γδ lymphocytes purified from healthy donor peripheral blood mononuclear cells (PBMC) (purity >95%, assessed by staining for the TCRVγ9 and TCRVδ2 chains followed by FACS analysis).Citation11 CIBERSORT-LM22 detected 7–40% of γδ cells and overestimated the CD4+, CD8+ and NK cell content of these samples (). In addition, CIBERSORT-LM22 enumerated up to 55% of γδ T cells in transcriptomes from purified CD4+, CD8+ and NK cells (Table S1). The GEP of these related cell subsets partly overlapCitation11 and the multicollinearity of this overlap is presumably what drives CIBERSORT to wrongly identify γδ T cells as T CD4+, T CD8+ or NK cells and vice versa. Thus, these data demonstrated the limits of CIBERSORT's LM22 reference matrix for the rating of TCRVγ9Vδ2+ γδ cells. The LM22 reference gene signature matrix was developed by machine learning from Affymetrix HG-U133A microarrays (∼8500 genes) from 113 reference samples downloaded from GEO (https://www.ncbi.nlm.nih.gov/geo/) and which included only two samples annotated as “blood γδ cell” whose purity and TCR phenotype, although most likely of the TCRVγ9Vδ2+ subset, were not specified.Citation12 Furthermore, of the 58 genes in the LM22 gene signature which are aimed at identifying γδ cells, the only TCRγδ-specific gene TRDC was actually not expressed by any of the 12 purified TCRVγ9Vδ2+ γδ cell samples described above. These observations prompted us to seek to improve the deconvolution of tissue expression profiles for assessing TCRVγ9Vδ2+ lymphocytes. We undertook this by increasing the CIBERSORT learning phase and reducing the number of cell subsets to discriminate between. The group of reference data sets was increased to 265 profiles, taken only from the Affymetrix HGU133 Plus 2.0 platform (which analyzes twice more genes than HGU133A microarrays), and included the above-depicted 12 samples of purified TCRVγ9Vδ2+ γδ T lymphocytes (Table S2). To optimize the CIBERSORT output, the number of subsets to determine was reduced from 22 to 7. These included B cells (pooling naïve, memory B cells and plasma cells), CD4+ cells (pooling CD4+ resting, CD4+ activated, regulatory T cells (Treg), and follicular helper T cells (TFH), CD8+ cells (resting and activated CD8+ cells), γδ T cells (resting and activated TCRVγ9Vδ2+ γδ cells), NK cells (resting and activated NK cells), “MoMaDC” cells (pooling monocytes, macrophages and DC), and granulocytes (arbitrarily pooling mastocytes, eosinophils and neutrophils). These 265 reference data sets were curated, assembled, normalized using the robust multiarray average (RMA) method and then collapsed to construct a novel leucocyte gene signature matrix using the CIBERSORT learning algorithm.Citation9 After the removal of genes expressed by non-hematopoietic tissues the resulting matrix, called LM7 (Table S3), comprised 375 genes, 134 of which were present in LM22. This learning identified 23 genes specifically upregulated in human γδ T cells only: BCOR, BTBD5, CCR5, CREM, CXCR3, CXCR6, FAM46C, FLJ25967, GPR132, GPR171, LEPREL1, LOC653506, LOC653725, METRNL, NR4A3, PDE4B, PDE4D, PRKX, PRKY, RORA, SYTL2, SYTL3 and UPP1, while it found that the TCRγδ−encoding genes TRD@, TRDV2, TRGC2, TRGV2 and TRGV9 are strongly expressed in both γδ T, αβ T and NK cells (Table S3).
Figure 1. Deconvolution of microarrays from pure TCRVγ9Vδ2+ γδ T lymphocytes. (A) CIBERSORT detected only marginal rates of γδ cells when the original reference matrix LM22 was applied to deconvolution of the transcriptomes from 12 samples of purified (> 90% TCRVγ9Vδ2+)γδ T lymphocytes. (B) Using the reference matrix LM7, CIBERSORT detected respectively 92%, 76%, 92%, 93%, 87%, 71%, 94%, 84%, 95%, 85%, 81% and 85% of TCRVγ9Vδ2+ γδ cells in the same samples as above. (C) Deviation of CIBERSORT results from the rates specified in each sample annotation file. With LM7, the deconvolution results were closer to most rates specified in the annotation file of control samples of known leucocyte composition, compared with LM22. (D) Pearson correlation of results from CIBERSORT-LM22 or CIBERSORT-LM7 with FACS-based reference rates of CD4+, CD8+ and B cells in a series of follicular lymphoma biopsies (GSE 65135). (E) Results from CIBERSORT-LM22 and CIBERSORT-LM7 yielded consistent rates of αβ TILs but not of γδ TILs upon the deconvolution of ∼10,000 cancer data sets (see below). The rates of αβ TILs were taken as the sum of CD4+ and CD8+ cells. Pearson correlation values are specified; the smaller scale of the γδ TILs scatterplot reflects their lower abundance than αβ TIL in the biopsies. (F) Mean leucocyte abundance in normal tissues from a validation series of (n = 114) transcriptomes.
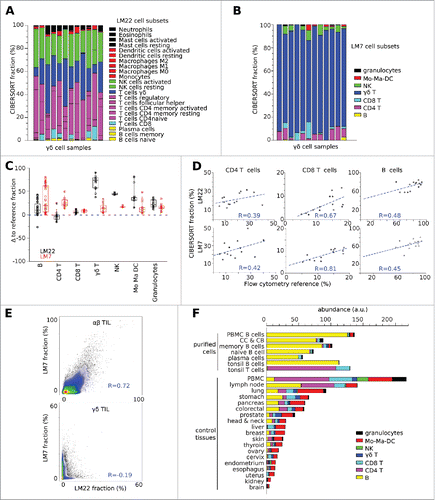
With the above reference data set (n = 265 samples), the relative leucocyte proportions predicted by CIBERSORT-LM7 and CIBERSORT-LM22 were concordant with the compositions annotated in most GEO sample record (GSM), with the exception of the above-depicted TCRVγ9Vδ2+ γδ samples whose values were still perfectible though significantly improved with LM7 (which calculated >90% γδ cells on average, versus 31% with LM22) ( and Table S1). We then downloaded from GEO and processed a validation data set of tissues (n = 114) whose composition had previously been determined (Table S4). Deconvolution of this data set using CIBERSORT-LM7 and CIBERSORT-LM22 matched the specified fractions of B cells and CD4+ cells, while the predicted levels of cytolytic (CD8+, NK, TCRVγ9Vδ2+ γδ), myeloid (monocytes, macrophages and DC) and granulocyte subsets improved with LM7 (). However, deconvolution with CIBERSORT-LM7 of (n = 6) transcriptomes from purified cord blood γδ T cells, which encompass both TCRVγ9Vδ2 and non-TCRVγ9Vδ2 γδ T cells dominated by TCRVγ8Vδ1 cells in cytomegalovirus-infected samples,Citation13 gave quite low results (detection of 24% γδ cells on average; range 11–51%), which were better than with CIBERSORT-LM22 (detection of < 5% γδ cells on average). Further, deconvolution of the transcriptomes of ∼10,000 tumor biopsies of unknown composition (section below) by CIBERSORT-LM22 and CIBERSORT-LM7 was concordant for αβ tumor-infiltrating lymphocytes (TILs) (taken as sum of CD4+ and CD8+ T cells, Pearson r = 0.72) but not for γδ TILs (Pearson r = −0.12) (), and was variably concordant for the other subsets: Mo-Ma-DC (Pearson r = 0.8), B lymphocytes (Pearson r = 0.76), NK (Pearson r = 0.3) and Granulocytes (Pearson r = 0.2) (data not shown).
Although CIBERSORT-LM7 was able to determine the leucocyte composition of tissues arrayed with Affymetrix HG-U133 Plus 2.0 microarrays, it was possible that the use of other platforms which lack some of the LM7 genes could alter its results. We estimated this bias by evaluating the LM7-generated leucocyte subset fractions from the training data set with and without the genes missing from the other platforms (Fig. S1) and found that the incomplete data introduced negligible (< 5%) deviations from the original deconvolution results (Fig. S2).
CIBERSORT determines the relative fractions of leucocytes within a sample, and not their abundance, whereas the sample enrichment score (SES) of leucocytes (SES-LM7) (see Materials and methods) reflects the abundance of leucocytes in each sampleCitation14 and parallels CD45 gene expression levels (Fig. S3). Thus, the abundance of each leucocyte subset in each sample was obtained by computing [(SES-LM7) × (CIBERSORT-LM7 fraction)] using the DeepTIL algorithm (see Materials and methods) which yields abundance results expressed as arbitrary units. The leucocyte abundance estimates determined by this method from HGU133 Plus 2.0 microarrays of normal tissues (n = 494) were consistent with the annotations of purified cell samples and the composition of normal tissues in that leucocytes were found to be most abundant in PBMC, lymph nodes and lung tissue while scarce in normal brain and kidney tissue (). This validated the DeepTIL algorithm as a useful tool for obtaining more accurate quantification of leucocyte subset abundance, particularly the blood-derived TCRVγ9Vδ2+ γδ T cells.
Abundance of tumor infiltrating leucocytes in a spectrum of cancers
We then investigated the abundance of TCRVγ9Vδ2+ γδ T cells and other LM7-defined leucocyte subsets determined by this computational method from a compendium of ∼10,000 bulk tumor biopsies from 16 hematological and 30 solid types of cancers (listed in Table S6). This showed that the hematological malignancies had a distinct leucocyte abundance and composition dominated by the cell-of-origin lineage (COO), except for Hodgkin's lymphoma (HL) and T-cell/histiocyte rich B cell lymphoma (THRBL) whose composition reflected the tumor microenvironment.Citation9 In follicular lymphoma (FL), CIBERSORT-LM7 detected less TCRVγ9Vδ2+ γδ TILs (on average 0.22 a.u. corresponding to 0.5% of leucocytes) than previously reported (∼5% of leucocytes infiltrating FL and diffuse large B cell lymphoma (DLBCL) according toCitation10). Our result () are in agreement with earlier IHC and FACS studies showing significantly less γδ T cells in FL lymph nodes than in inflammatory adenitis lymph nodes.Citation15 Of note, deconvolution of the same FL and DLBCL transcriptomes by CIBERSORT-LM-7 detected 10-fold less γδ cells than CIBERSORT-LM22, illustrating its more stringent identification of γδ cells (). Besides a few cases with a γδ COO,Citation16,17 most peripheral T cell lymphoma (PTCL) and the other T cell malignancies did not present abundant TCRVγ9Vδ2+ γδ cell infiltrates, whereas the cohorts of B-ALL, CML and AML included in this study revealed an abundant TCRVγ9Vδ2+ γδ TIL compartment (corresponding to ∼10% of leucocytes). This result is higher than has previously been reported for AML samples,Citation9,18,19 and even for normal bone marrow (∼2.5% of leucocytes,Citation20). Among the eight subtypes of AML defined by the French–American–British (FAB) classification, acute megakaryoblastic leukemia (FAB-M7 subtype of AML) presented the greatest infiltration of total leucocytes and acute promyelocytic leukemia (FAB-M3 subtype of AML) presented the highest abundance of TCRVγ9Vδ2+ γδ TILs. Solid tumors generally presented less abundant leucocyte infiltration than blood malignancies, and half of their infiltrated content was consistently of myeloid lineage. Quantitatively, these infiltrates were found to be most important in lung, pancreas, prostate, breast, stomach, renal (papillary and clear cell subtypes), colorectal, bladder, liver, esophagus, uterus, liver, head and neck carcinoma, melanoma, thyroid, uterus, endometrium, cervix, and ovary carcinoma tissues (ranked by decreasing magnitude). The least abundant infiltrates were found in cancers carrying the lowest number of somatic mutations, such as kidney chromophobe carcinoma, Ewing sarcoma, kidney oncocytoma and indeed in central nervous system (CNS) cancers. The infiltrates of brain cancers are mostly of myeloid lineage, probably the monocyte-related microglia.Citation21 The abundance of TCRVγ9Vδ2+ γδ TILs varied across the different cancers, being quite high in prostate, esophagus, cervix, head and neck, lung, pancreas and breast carcinoma. Both CD8+ and TCRVγ9Vδ2+ γδ TILs were abundant in inflammatory breast carcinoma, whose samples generally consisted of triple negative breast cancers. In melanoma, both αβ and TCRVγ9Vδ2+ γδ TILs were also found to be more abundant in primary lesions than in metastases (). Overall, the extent of leucocyte infiltration was quite heterogeneous across cancers and the average abundance of αβ and TCRVγ9Vδ2+ γδ TILs varied likewise between cancer types (Table S5). This large inter-individual fluctuation (ranging across several orders of magnitude) was also seen in FACS analysis of TILs from small cohorts of AML and prostate cancer patients (not shown). Plotting the individual abundance of αβ TILs at the level of patients and ranking cancer types on this criterion confirmed that the abundance of immune infiltrates fluctuated strongly among patients both inside and across cancer types. The abundance of TCRVγ9Vδ2+ αβ TILs among patients was also found to be highly variable, though they were consistently less abundant than αβ TILs. Strikingly however, the individual abundance of TCRVγ9Vδ2+ γδ TILs did not appear to correlate with the abundance of the αβ TILs neither within nor across the different types of cancer ().
Figure 2. Abundance of TILs across 55 types of hematological and solid cancers. Shown are the mean leucocyte composition of each specified cancer type. Blood malignancies were found to be as “leucocyte-rich” as normal PBMC or lymph nodes, whereas solid tumors included less leucocytes. Lung carcinomas displayed the most abundant leucocyte infiltrates, whereas brain tumors had a particularly low leucocyte composition.
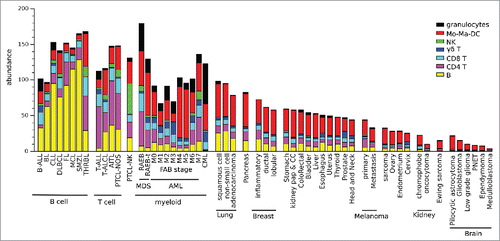
Figure 3. Individual abundances of tumor-infiltrating αβ T and TCRVγ9Vδ2+ γδ T lymphocytes across 55 types of hematological and solid cancers. Individual abundances of αβ and TCRVγ9Vδ2+ γδ TILs across cancer types. Blood and solid cancers are successively presented in order of increasing αβ TIL abundance. Each dot is a sample, red bars show the median of each cancer type, blue dotted lines show the mean abundance of the specified TILs across hematological (top) and solid (bottom) cancers. For each type of cancer, the Wilcoxon test was used to calculate the statistical significance of deviation from the mean abundance of TIL in hematological or solid cancers. The Benjamini–Hochberg (BH) correction was applied to determine the FDR for multiple comparisons, only the significant BH-corrected p-values are specified (right).
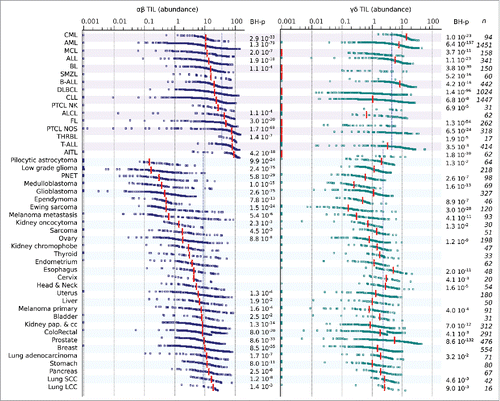
No correlation between αβ TIL and TCRVγ9Vδ2+ γδ TIL abundance across the cancer spectrum
The above results suggested that tumor infiltration by the αβ and TCRVγ9Vδ2+ γδ TILs occur in distinct immunological contexts. To characterize these contexts, we computed SESCitation14 of all the gene sets from the MSigDBCitation22 for the 10,000 cancer samples, and determined whether these correlated with the abundance of αβ and TCRVγ9Vδ2+ γδ TILs. This approach revealed significant differences between the TIL subsets. Across all cancers, the abundance of αβ TILs positively correlated with the enrichment of “TCR signaling” (Spearman r = 0.78), “Toll-like receptor signaling pathway” (Spearman r = 0.45) and related gene sets, whereas the abundance of TCRVγ9Vδ2+ γδ TILs did not correlate with these (Spearman r = −0.02 and −0.03, respectively). These distinct features were best exemplified within specific cancer types. For example, in colorectal carcinoma, the abundance of αβ TILs correlated with the enrichment of the “Response to IFNα”, “Inflammatory response”, “Antigen processing and presentation”, “T cell activation” and “Cytolytic activity” gene sets, as well as with the abundance of Mo-Ma-DC cells (). We found that all of these metrics also cross-correlated, and patients with “αβ TIL-high” tumors presented significantly higher overall survival (OS) rates than those with “αβ TIL-low” tumors (). This result is in accordance with the well-known association of αβ TIL density with the outcome of colorectal cancer patients.Citation23,24 In contrast, the abundance of TCRVγ9Vδ2+ γδ TILs did not correlate with any of these transcriptomic readouts (Spearman r < 0.12), since correlation with these reference MSIgDB gene sets was the same as with a collection of random gene sets (RGS, data not shown). Nevertheless, colorectal cancer patients with “TCRVγ9Vδ2+ γδ TIL-high” tumors presented better OS rates than those with “TCRVγ9Vδ2+ γδ TIL-low” tumors, irrespective of their abundance of αβ TIL. Hence, the presence of TCRVγ9Vδ2+ γδ TIL was like αβ TIL, associated with a favorable outcome in colorectal cancer, even though these lymphocytes are recruited through a distinct immunological context. The same conclusions were drawn from the analyses of additional cohorts of CLL, AML and prostate cancer patients (). These and the above results () indicate that the recruitment of αβ and TCRVγ9Vδ2+ γδ TILs occurs in different immunological contexts.
Figure 4. Different correlates of αβ and TCRVγ9Vδ2+ γδ TIL abundance. (A) In the specified groups of cancer patients, heatmaps of spearman correlation between abundance of αβ TILs (top) or TCRVγ9Vδ2+ γδ TILs (bottom) with SES for the gene sets indicated. The abundance of αβ TILs never shows the same correlation profile as the abundance of TCRVγ9Vδ2+ γδ TILs. (B) Kaplan–Meier plots for the overall survival of colorectal carcinoma, CLL and AML patients or for the biochemical relapse (BCR) of prostate cancer patients, according to the abundance of the αβ TILs only (left), the TCRVγ9Vδ2+ γδ TILs superimposed on the αβ TILs-low only (middle) and the TCRVγ9Vδ2+ γδ TILs on αβ TILs-high only (right). Abundance of both subsets of TILs is associated to favorable outcome in these cohorts of cancer patients.
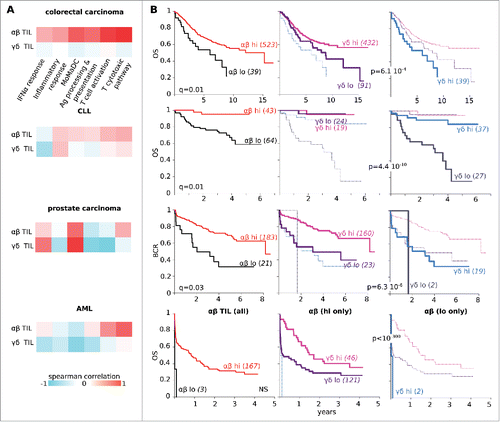
Discussion
We report here the application of an emerging method of machine learning for microarray deconvolution to assess the abundance of leucocyte infiltration in tumor biopsies. Since its first application in the deconvolution of blood transcriptomes from patients with systemic lupus erythematosus through iterative linear least-squares fitting,Citation25 this field has rapidly expanded and has progressively validated the use of computational deconvolution to identify the individual component cells from RNA mixtures. The current in silico approaches for characterizing cellular heterogeneity from GEP encompass either the analysis of cell type-specific genes and gene set enrichments, or the direct algorithmic deconvolution of transcriptomes to deduce cell-type compositions (reviewed inCitation8). These both now yield results for the rapid profiling of TILs that match those achieved by conventional flow cytometry (FACS) and IHC techniques, but without the biases due to the mechanical dissociation of samples or the antibodies used for reliable detection of γδ TILs by IHC of formalin-fixed paraffin-embedded tumor biopsies. Optimizing the discrimination of ontologically related cell types is not trivial, however, as it requires the multicollinearity of their highly similar transcriptomes be addressed. Several techniques of automated deconvolution have recently been developed toward this aim (reviewed inCitation26), among which the most robust and performant is currently the machine learning algorithm CIBERSORT.Citation9 CIBERSORT applies nu-support vector regression to enumerate 22 leucocyte subsets from bulk tumor biopsies, and has allowed the identification of genes and leucocyte predictors of survival in a meta-analysis of thousands of cancers.Citation10 More recently, CIBERSORT also enabled the successful leucocyte enumeration by deconvolution of microarray data sets from PBMC,Citation27 glioma,Citation28 lung carcinomaCitation29 and triple negative breast cancers.Citation30 Here, however, we found that CIBERSORT-LM22 does not discriminate between γδ T cells and the closely related T CD4+, T CD8+ and NK lymphoid subsets due to insufficient training from only two γδ Τ cell transcriptomes assessed on first-generation microarrays. The improved learning from more than 260 second-generation microarrays (encompassing ∼21,000 genes) and the reduced output of only 7 instead of 22 subtypes of leucocytes was expected to reduce the multicollinearity of their individual gene profiles, and thereby improve output reliability. Our report shows that CIBERSORT-LM7 detects TCRVγ9Vδ2+ γδ T lymphocytes more accurately than CIBERSORT-LM22, but it has learnt this from 12 microarrays of purified, resting and activated polyclonal γδ T cells expressing only TCRVγ9Vδ2, raising the question of whether it detects as efficiently the other (non-TCRVγ9Vδ2) subsets of γδ T lymphocytes. So far, the scarce publicly available microarrays for such purified γδ T cells do not allow to address this point. Nevertheless, the less performant deconvolution of transcriptomes for γδ cell bulks highly purified from fetal blood,Citation13 although far better with LM7 than LM22, indicates that the current learning process enabled CIBERSORT-LM7 to reliably identify as γδ cells those cells sharing the gene signature of blood TCRVγ9Vδ2 γδ T cells. Future integration of other γδ T cell subsets purified from normal tissues or tumor samples and profiled on microarray or RNA Seq will enlarge the learning of CIBERSORT-LM7 to empower its identification capacities. However, since learning from more than one hundred CD4+ and CD8+ microarrays does not empower CIBERSORT to discriminate between the various TCR-defined subsets of αβ T cells, the learning from smaller sampling data sets might set limits for performing TCR-defined γδ T cell identification.
The overall output of CIBERSORT-LM7 matches that of CIBERSORT-LM22 but with a better reliability for the detection of γδ T cells, which were previously mistakenly identified as CD4+ (31%) or NK cells (23%), while 8% of CD8+ T cells were mistakenly classified as γδ T lymphocytes (Table S4). As a consequence, the association of γδ cells with a favorable outcome across a large number of cancer patients should be taken with caution.Citation10 Our study reports TCRVγ9Vδ2+ γδ TILs levels that are fully consistent with the body of literature about various types of cancers (31-34 and see below). For B-ALL, however, this result could reflect either the actual recruitment of γδ TILs or the bi-phenotypic profile of such leukemia, which frequently co-express rearranged Ig and γδ TCR genes.Citation35,36 In CML, bone marrow-infiltrating TCRVγ9Vδ2+ γδ T lymphocytes have not been reported, but the reported average of 8% CD3, 0% CD4+ and <4% CD8+ T cells in such tissuesCitation37 is compatible with our results. In AML, the few studies which have quantified TCRVγ9Vδ2+ γδ TILs in bone marrow biopsies have found them to be at similar levels as in the blood, but these figures might differ across patients and FAB subtypes.Citation19,38 In solid tumors, independent studies have mentioned an abundance of γδ TILs in cancers of the pancreas,Citation34 breast,Citation31 head and neck,Citation33 colorectalCitation39 and lung,Citation40 but there have been no reports for prostate, cervix and esophagus cancers. Moreover, beside of the pioneering CIBERSORT studies which sub-optimally detect γδ cells,Citation9,10 there are currently no published meta-analyses of TCRVγ9Vδ2+ γδ TIL across different human cancers.
The results presented here detected highly heterogeneous amounts of TCRVγ9Vδ2+ γδ TILs in cancers, and their relative abundance in the above solid cancers as well as in prostate and esophagus carcinoma. The high level of the TCRγ gene expression by prostatic epithelial cellsCitation41 might set pitfalls to identify γδ TILs in prostate cancer by conventional methods, but not to the deconvolution depicted here which takes in account all the LM7 genes for this aim. So far however, the abundance of TCRVγ9Vδ2γδ TILs in prostate cancer was not reported, so this study brings further rationale to harness these cells for immunotherapeutic purposes.Citation42-45 This work also reports abundant TCRVγ9Vδ2 γδ TILs in breast carcinoma, a finding in line with earlier studies demonstrating that these γδ cells are abundant but associated with immunosuppressive activity, tumor progression, lymph node metastases and correlate with poor outcome.Citation31 Likewise, the stroma of ductal adenocarcinoma was reported to comprise abundant γδ TIL with unfavorable role,Citation34 supporting our result for this disease. Actually, a detrimental role is reportedly associated to γδ TILs in breast, colorectal and pancreas cancer,Citation31,34,39 raising the question of whether their abundance generally predicts a favorable or unfavorable outcome. The results presented here illustrate an association of TCRVγ9Vδ2+ γδ TILswith favorable clinical evolution in four cohorts of patients with different types of cancer (). This seemingly contrasts with an earlier study depicting promotion of colorectal cancer in patients with abundant Th17-producing γδ TILs.Citation39 Nevertheless, the clinical significance of γδ TILs abundance in cancer most probably depends on the TCR subsets of γδ cells,Citation7 their maturation and cytokine profileCitation46 and the tumor contexts as demonstrated in AML,Citation47 breast,Citation31 colorectalCitation39 and pancreaticCitation34 cancers.
The abundance of αβ and TCRVγ9Vδ2+ γδ TIL across the spectrum of cancers tested varied considerably. The ordering of cancers according to their abundance of αβ TILs presented a similar pattern as that seen when they are ordered by mutational load.Citation48,49 This was illustrated by children's cancers, which typically have few mutations and here presented the least αβ TILs whereas cancers associated with durable exposure to carcinogens had the greatest number of these cells (). This is in agreement with the finding that the diversity and amount of αβ TIL clonotypes increases with the amount of non-synonymous mutations within tumors.Citation50 Nevertheless, we detected a different distribution of TCRVγ9Vδ2+ γδ TILs, suggesting that their incentive for infiltrating tumors is not mutational as for αβ TILs. The lack of identification of transcriptomic correlates for the abundance of TCRVγ9Vδ2+ γδ TILs across the cancer spectrum could be due to several diverse reasons: the screen for correlates involved only MSIgDB-predefined human gene sets not unknown ones, this human transcriptome-based strategy cannot detect associations with most viralCitation51 or microbiotal contexts,Citation52,53 this strategy might be intrinsically unable to detect the non-transcriptional hallmarks of cancers such as specific somatic mutations or the accumulation of endogenous (onco) metabolites. Due to the metabolic and microbial origin of the PAg that activate most human blood TCRVγ9Vδ2+ γδ lymphocytes,Citation2 such hypotheses deserve further investigation. Future research exploiting the increasing body of RNASeq and metabolomics data sets from human cancers will certainly help in addressing these issues.
Materials and methods
Signature matrix
Public raw data of (n = 265) transcriptomes from purified cell types obtained using Affymetrix HGU133 Plus 2.0 microarrays were downloaded from https://www.ncbi.nlm.nih.gov/gds, normalized together and collapsed to HUGO gene symbols using chipset definition files available from the NCBI GEO. These are referred to as reference data (Table S2). As a filtration step, genes with enriched expression (mean log2 (expression level) >7) in all the normal non-hematopoietic tissues from the Human Body Index (study GSE7307) were removed. We then defined seven leucocyte subsets (B cells, CD4+ T cells, CD8+ T cells, γδ T cells, Mo-Ma-DC cells and granulocytes) which were imported into the CIBERSORT software (https://cibersort.stanford.edu/)Citation9 to build the LM7 matrix (Table S3). For validation, CIBERSORT-LM7 was applied to a variety of external normalized public data sets (Table S4) containing purified leucocyte cells subsets including non-TCRVγ9Vδ2 γδ T cells isolated from human cord bloodCitation54 or mixed populations of known composition.
Abundance calculations
Abundances were calculated from the CIBERSORT results and the SES, as depicted in the text. The SESCitation14 were computed by applying the open source software AutoCompare-SES (https://sites.google.com/site/fredsoftwares/products/autocompare_ses) with normalized settings. Then, the open source software DeepTIL (https://sites.google.com/site/fredsoftwares/products/deeptil) was used to automatically compute the abundance of the seven leucocyte subsets in the samples. DeepTIL was written using the cross-platform Julia programming languageCitation55; its typical computation time is 3–5 sec per sample. A parallel version of DeepTIL is also available for computing data tables simultaneously. From CIBERSORT and SES calculations, DeepTIL computes the following steps: (1) selection of LM7 genes with a mean log2 (expression level) >11.5 in each leucocyte type to constitute its defining gene set. In each sample, the SES of the seven leucocyte defining gene sets are computed against the testing alternative “greater than”.Citation14 The SES of each type of leucocyte is also computed for each of the seven pure types of leucocyte to determine the seven corresponding correcting factors. (2) a linear combination of the seven leucocyte type SESs weighted by their respective correcting factors is computed for each sample. (3) for each sample, CIBERSORT fractions are finally multiplied by the linear combination of SES to produce the abundance of each leucocyte subset. The mean abundance of each leucocyte subset in ∼10,000 samples of human cancers is summarized (Table S6).
Statistical analysis
Assessments of leucocyte fractions from the specified transcriptomes were performed using CIBERSORT (https://cibersort.stanford.edu/)Citation9 with 500 Monte Carlo iterations and the matrix indicated in the text. Comparisons of TIL (αβ and γδ) abundances across cancers () were performed by Wilcoxon tests, assessing the abundance of TIL in the specified cancer type comparatively to the same variable across the whole set of hematological or solid cancers. The Benjamini–Hochberg (BH) correctionCitation56 was applied for multiple comparisons. The functionally defined collection of gene sets was downloaded from MSIgDB (http://software.broadinstitute.org/gsea/msigdb),Citation22 while for control experiments, we generated a collection of 500 random gene sets of the same size as MSIgDB, referred to as RGS. For the transcriptome samples specified in the text, the SES of each gene set was computed as depictedCitation14 and their respective correlations (Pearson and Spearman) with the abundance of the specified cell types were automatically computed using the “Correlations by lines in list” software (https://sites.google.com/site/fredsoftwares/products/correlation-by-lines-in-list). For Kaplan–Meier plots, optimal cutoffs were determined with the survival R packageCitation57 and Log-Rank p-values were corrected using the BH method.Citation56
Disclosure of potential conflicts of interest
No potential conflicts of interest were disclosed.
KONI_A_1284723_supplementary_materials.pdf
Download PDF (2.5 MB)Acknowledgments
We acknowledge JJF's team members for their stimulating comments and Kelly Thornber from Scientific Scripts for English editing of the text.
Funding
This work was funded by the Institut National de la Santé et de la Recherche Médicale (INSERM), the Université Toulouse III: Paul Sabatier, the Center National de la Recherche Scientifique (CNRS), the Laboratoire d'Excellence Toulouse Cancer (TOUCAN) (contract ANR11-LABX) and the Program Hospitalo-Universitaire en Cancérologie CAPTOR (contract ANR11-PHUC0001).
References
- Fisch P, Malkovsky M, Braakman E, Sturm E, Bolhuis RL, Prieve A, Sosman JA, Lam VA, Sondel PM. Gamma/delta T cell clones and natural killer cell clones mediate distinct patterns of non-major histocompatibility complex-restricted cytolysis. J Exp Med 1990; 171:1567–79; PMID:2185329; http://dx.doi.org/10.1084/jem.171.5.1567
- Fournie JJ, Bonneville M. Stimulation of gamma delta T cells by phosphoantigens. Res Immunol 1996; 147:338–47; PMID:8876063; http://dx.doi.org/10.1016/0923-2494(96)89648-9
- Poccia F, Malkovsky M, Gougeon ML, Bonneville M, Lopez-Botet M, Fournie JJ, Colizzi V. Gammadelta T cell activation or anergy during infections: the role of nonpeptidic TCR ligands and HLA class I molecules. J Leukoc Biol 1997; 62:287–91; PMID:9307066
- Fournie JJ, Sicard H, Poupot M, Bezombes C, Blanc A, Romagne F, Ysebaert L, Laurent G. What lessons can be learned from gamma delta T cell-based cancer immunotherapy trials? Cell Mol Immunol 2013; 10:35–41; http://dx.doi.org/10.1038/cmi.2012.39
- Meraviglia S, Lo Presti E, Dieli F, Stassi G. gammadelta T cell-based anticancer immunotherapy: progress and possibilities. Immunotherapy 2015; 7:949–51; PMID:26569071; http://dx.doi.org/10.2217/imt.15.68
- Kobayashi H, Tanaka Y. gammadelta T Cell Immunotherapy-A Review. Pharmaceuticals (Basel) 2015; 8:40–61; PMID:25686210; http://dx.doi.org/10.3390/ph8010040
- Silva-Santos B, Serre K, Norell H. gammadelta T cells in cancer. Nat Rev Immunol 2015; 15:683–91; PMID:26449179; http://dx.doi.org/10.1038/nri3904
- Shen-Orr SS, Gaujoux R. Computational deconvolution: extracting cell type-specific information from heterogeneous samples. Curr Opin Immunol 2013; 25:571–8; PMID:24148234; http://dx.doi.org/10.1016/j.coi.2013.09.015
- Newman AM, Liu CL, Green MR, Gentles AJ, Feng W, Xu Y, Hoang CD, Diehn M, Alizadeh AA. Robust enumeration of cell subsets from tissue expression profiles. Nat Methods 2015; 12:453–7; PMID:25822800; http://dx.doi.org/10.1038/nmeth.3337
- Gentles AJ, Newman AM, Liu CL, Bratman SV, Feng W, Kim D, Nair VS, Xu Y, Khuong A, Hoang CD et al. The prognostic landscape of genes and infiltrating immune cells across human cancers. Nat Med 2015; 21:938–45; PMID:26193342; http://dx.doi.org/10.1038/nm.3909
- Pont F, Familiades J, Dejean S, Fruchon S, Cendron D, Poupot M, Poupot R, L'faqihi-Olive F, Prade N, Ycart B et al. The gene expression profile of phosphoantigen-specific human gammadelta T lymphocytes is a blend of alphabeta T-cell and NK-cell signatures. Eur J Immunol 2012; 42:228–40; PMID:21968650; http://dx.doi.org/10.1002/eji.201141870
- Chtanova T, Newton R, Liu SM, Weininger L, Young TR, Silva DG, Bertoni F, Rinaldi A, Chappaz S, Sallusto F et al. Identification of T cell-restricted genes, and signatures for different T cell responses, using a comprehensive collection of microarray datasets. J Immunol 2005; 175:7837–47; PMID:16339519; http://dx.doi.org/10.4049/jimmunol.175.12.7837
- Vermijlen D, Brouwer M, Donner C, Liesnard C, Tackoen M, Van Rysselberge M, Twité N, Goldman M, Marchant A, Willems F et al. Human cytomegalovirus elicits fetal gammadelta T cell responses in utero. J Exp Med 2010; 207:807–21; PMID:20368575; http://dx.doi.org/10.1084/jem.20090348
- Tosolini M, Algans C, Pont F, Ycart B, Fournie JJ. Large scale microarray profiling reveals four stages of immune escape in non-Hodgkin's lymphomas. Oncoimmunology 2016; 5(7):e1188246: in press; PMID:27622044; http://dx.doi.org/10.1080/2162402X.2016.1188246
- Braza MS, Caraux A, Rousset T, Lafaye de Micheaux S, Sicard H, Squiban P, Costes V, Klein B, Rossi JF. gammadelta T lymphocytes count is normal and expandable in peripheral blood of patients with follicular lymphoma, whereas it is decreased in tumor lymph nodes compared with inflammatory lymph nodes. J Immunol 2010; 184:134–40; PMID:19949101; http://dx.doi.org/10.4049/jimmunol.0901980
- Gaulard P, de Leval L. Pathology of peripheral T-cell lymphomas: where do we stand? Semin Hematol 2014; 51:5–16; PMID:24468311; http://dx.doi.org/10.1053/j.seminhematol.2013.11.003
- Przybylski GK, Wu H, Macon WR, Finan J, Leonard DG, Felgar RE, DiGiuseppe JA, Nowell PC, Swerdlow SH, Kadin ME et al. Hepatosplenic and subcutaneous panniculitis-like gamma/delta T cell lymphomas are derived from different Vdelta subsets of gamma/delta T lymphocytes. J Mol Diagn 2000; 2:11–9; PMID:11272897; http://dx.doi.org/10.1016/S1525-1578(10)60610-1
- Gertner-Dardenne J, Castellano R, Mamessier E, Garbit S, Kochbati E, Etienne A, Charbonnier A, Collette Y, Vey N, Olive D. Human Vgamma9Vdelta2 T cells specifically recognize and kill acute myeloid leukemic blasts. J Immunol 2012; 188:4701–8; PMID:22467661; http://dx.doi.org/10.4049/jimmunol.1103710
- Gertner-Dardenne J, Fauriat C, Vey N, Olive D. Immunotherapy of acute myeloid leukemia based on gammadelta T cells. Oncoimmunology 2012; 1:1614–6; PMID:23264912; http://dx.doi.org/10.4161/onci.21512
- Dean J, McCarthy D, Golden-Mason L, O'Farrelly C. Trephine biopsies are enriched for activated T/NK cells and cytotoxic T cells. Immunol Lett 2005; 99:94–102; PMID:15894117; http://dx.doi.org/10.1016/j.imlet.2005.01.008
- Jack AS, Lu JQ. Immune cell infiltrates in the central nervous system tumors. Austin Neurosurgery: Open access 2015; 2(1):30
- Subramanian A, Tamayo P, Mootha VK, Mukherjee S, Ebert BL, Gillette MA, Paulovich A, Pomeroy SL, Golub TR, Lander ES et al. Gene set enrichment analysis: a knowledge-based approach for interpreting genome-wide expression profiles. Proc Natl Acad Sci U S A 2005; 102:15545–50; PMID:16199517; http://dx.doi.org/10.1073/pnas.0506580102
- Galon J, Costes A, Sanchez-Cabo F, Kirilovsky A, Mlecnik B, Lagorce-Pages C, Tosolini M, Camus M, Berger A, Wind P et al. Type, density, and location of immune cells within human colorectal tumors predict clinical outcome. Science 2006; 313:1960–4; PMID:17008531; http://dx.doi.org/10.1126/science.1129139
- Mlecnik B, Bindea G, Angell HK, Maby P, Angelova M, Tougeron D, Church SE, Lafontaine L, Fischer M, Fredriksen T et al. Integrative analyses of colorectal cancer show immunoscore is a stronger predictor of patient survival than microsatellite instability. Immunity 2016; 44:698–711; PMID:26982367; http://dx.doi.org/10.1016/j.immuni.2016.02.025
- Abbas AR, Wolslegel K, Seshasayee D, Modrusan Z, Clark HF. Deconvolution of blood microarray data identifies cellular activation patterns in systemic lupus erythematosus. PLoS One 2009; 4:e6098; PMID:19568420; http://dx.doi.org/10.1371/journal.pone.0006098
- Newman AM, Alizadeh AA. High-throughput genomic profiling of tumor-infiltrating leukocytes. Curr Opin Immunol 2016; 41:77–84; PMID:27372732; http://dx.doi.org/10.1016/j.coi.2016.06.006
- Karpinski P, Frydecka D, Sasiadek MM, Misiak B. Reduced number of peripheral natural killer cells in schizophrenia but not in bipolar disorder. Brain Behav Immun 2016; 54:194–200; PMID:26872421; http://dx.doi.org/10.1016/j.bbi.2016.02.005
- Wang QH, Muller F, Kim H, Squatrito M, Millelsen T, Scarpace L, Barthel F, Lin YH, Satani N, Martinez-Ledesma E et al. Tumor evolution of glioma intrinsic gene expression subtype associates with immunological changes in the microenvironment. bioRxiv 2016; http://dx.doi.org/https://doi.org/10.1101/052076
- Araujo JM, Prado A, Cardenas NK, Zaharia M, Dyer R, Doimi F, Bravo L, Pinillos L, Morante Z, Aguilar A et al. Repeated observation of immune gene sets enrichment in women with non-small cell lung cancer. Oncotarget 2016; 7:20282–92; PMID:26958810; http://dx.doi.org/10.18632/oncotarget.7943
- Vinayak S, Gray RJ, Adams S, Jensen KC, Manola J, Afghahi A, Goldstein LJ, Ford JM, Badve SS, Telli ML. Association of increased tumor-infiltrating lymphocytes (TILs) with immunomodulatory (IM) triple-negative breast cancer (TNBC) subtype and response to neoadjuvant platinum-based therapy in PrECOG0105. J Clin Oncol 2014; 32: suppl.abstract 1000
- Ma C, Zhang Q, Ye J, Wang F, Zhang Y, Wevers E, Schwartz T, Hunborg P, Varvares MA, Hoft DF et al. Tumor-infiltrating gammadelta T lymphocytes predict clinical outcome in human breast cancer. J Immunol 2012; 189:5029–36; PMID:23034170; http://dx.doi.org/10.4049/jimmunol.1201892
- Kowalczyk D, Skorupski W, Kwias Z, Nowak J. Flow cytometric analysis of tumour-infiltrating lymphocytes in patients with renal cell carcinoma. Br J Urol 1997; 80:543–7; PMID:9352689; http://dx.doi.org/10.1046/j.1464-410X.1997.00408.x
- Bas M, Bier H, Schirlau K, Friebe-Hoffmann U, Scheckenbach K, Balz V, Whiteside TL, Hoffmann TK. Gamma-delta T-cells in patients with squamous cell carcinoma of the head and neck. Oral Oncol 2006; 42:691–7; PMID:16527515; http://dx.doi.org/10.1016/j.oraloncology.2005.11.008
- Daley D, Zambirinis CP, Seifert L, Akkad N, Mohan N, Werba G, Barilla R, Torres-Hernandez A, Hundeyin M, Mani VR et al. gammadelta T cells support pancreatic oncogenesis by restraining alphabeta T cell activation. Cell 2016; 166:1485-99 e15; http://dx.doi.org/10.1016/j.cell.2016.07.046
- Goorha R, Bunin N, Mirro J, Jr, Murphy SB, Cross AH, Behm FG, Quertermous T, Seidman J, Kitchingman GR. Provocative pattern of rearrangements of the genes for the gamma and beta chains of the T-cell receptor in human leukemias. Proc Natl Acad Sci U S A 1987; 84:4547–51; PMID:2955409; http://dx.doi.org/10.1073/pnas.84.13.4547
- Buccheri V, Matutes E, Dyer MJ, Catovsky D. Lineage commitment in biphenotypic acute leukemia. Leukemia 1993; 7:919–27; PMID:8501986
- Bachireddy P, Wu CJ. Understanding anti-leukemia responses to donor lymphocyte infusion. Oncoimmunology 2014; 3:e28187; PMID:25340010; http://dx.doi.org/10.4161/onci.28187
- Aswald JM, Wang XH, Aswald S, Lutynski A, Minden MD, Messner HA, Keating A. Flow cytometric assessment of autologous gammadelta T cells in patients with acute myeloid leukemia: potential effector cells for immunotherapy? Cytometry B Clin Cytom 2006; 70:379–90; PMID:16977635; http://dx.doi.org/10.1002/cyto.b.20115
- Wu P, Wu D, Ni C, Ye J, Chen W, Hu G, Wang Z, Wang C, Zhang Z, Xia W et al. gammadeltaT17 cells promote the accumulation and expansion of myeloid-derived suppressor cells in human colorectal cancer. Immunity 2014; 40:785–800; PMID:24816404; http://dx.doi.org/10.1016/j.immuni.2014.03.013
- Zocchi MR, Ferrarini M, Migone N, Casorati G. T-cell receptor V delta gene usage by tumour reactive gamma delta T lymphocytes infiltrating human lung cancer. Immunology 1994; 81:234–9; PMID:8157272
- Essand M, Vasmatzis G, Brinkmann U, Duray P, Lee B, Pastan I. High expression of a specific T-cell receptor gamma transcript in epithelial cells of the prostate. Proc Natl Acad Sci U S A 1999; 96:9287–92; PMID:10430935; http://dx.doi.org/10.1073/pnas.96.16.9287
- McNeel DG, Malkovsky M. Immune-based therapies for prostate cancer. Immunol Lett 2005; 96:3–9; PMID:15585302; http://dx.doi.org/10.1016/j.imlet.2004.06.009
- Dieli F, Vermijlen D, Fulfaro F, Caccamo N, Meraviglia S, Cicero G, Roberts A, Buccheri S, D'Asaro M, Gebbia N et al. Targeting human {gamma}delta} T cells with zoledronate and interleukin-2 for immunotherapy of hormone-refractory prostate cancer. Cancer Res 2007; 67:7450–7; PMID:17671215; http://dx.doi.org/10.1158/0008-5472.CAN-07-0199
- Dieli F, Caccamo N, Meraviglia S. Advances in immunotherapy of castration-resistant prostate cancer: bisphosphonates, phosphoantigens and more. Curr Opin Investig Drugs 2008; 9:1089–94; PMID:18821471
- Lang JM, Wallace M, Becker JT, Eickhoff JC, Buehring B, Binkley N, Staab MJ, Wilding G, Liu G, Malkovsky M et al. A randomized phase II trial evaluating different schedules of zoledronic acid on bone mineral density in patients with prostate cancer beginning androgen deprivation therapy. Clin Genitourin Cancer 2013; 11:407–15; PMID:23835291; http://dx.doi.org/10.1016/j.clgc.2013.04.029
- Rei M, Pennington DJ, Silva-Santos B. The emerging Protumor role of gammadelta T lymphocytes: implications for cancer immunotherapy. Cancer Res 2015; 75:798–802; PMID:25660949; http://dx.doi.org/10.1158/0008-5472.CAN-14-3228
- Jin Z, Luo Q, Lu S, Wang X, He Z, Lai J, Chen S, Yang L, Wu X, Li Y. Oligoclonal expansion of TCR Vdelta T cells may be a potential immune biomarker for clinical outcome of acute myeloid leukemia. J Hematol Oncol 2016; 9:126; PMID:27863523; http://dx.doi.org/10.1186/s13045-016-0353-3
- Alexandrov LB, Nik-Zainal S, Wedge DC, Aparicio SA, Behjati S, Biankin AV, Bignell GR, Bolli N, Borg A, Børresen-Dale AL et al. Signatures of mutational processes in human cancer. Nature 2013; 500:415–21; PMID:23945592; http://dx.doi.org/10.1038/nature12477
- Lawrence MS, Stojanov P, Polak P, Kryukov GV, Cibulskis K, Sivachenko A, Carter SL, Stewart C, Mermel CH, Roberts SA et al. Mutational heterogeneity in cancer and the search for new cancer-associated genes. Nature 2013; 499:214–8; PMID:23770567; http://dx.doi.org/10.1038/nature12213
- Li B, Li T, Pignon JC, Wang B, Wang J, Shukla SA, Dou R, Chen Q, Hodi FS, Choueiri TK et al. Landscape of tumor-infiltrating T cell repertoire of human cancers. Nat Genetics 2016; 48:725–32; PMID:27240091; http://dx.doi.org/10.1038/ng.3581
- Rooney MS, Shukla SA, Wu CJ, Getz G, Hacohen N. Molecular and genetic properties of tumors associated with local immune cytolytic activity. Cell 2015; 160:48–61; PMID:25594174; http://dx.doi.org/10.1016/j.cell.2014.12.033
- Zitvogel L, Ayyoub M, Routy B, Kroemer G. Microbiome and Anticancer Immunosurveillance. Cell 2016; 165:276–87; PMID:27058662; http://dx.doi.org/10.1016/j.cell.2016.03.001
- Zitvogel L, Galluzzi L, Viaud S, Vetizou M, Daillere R, Merad M, Kroemer G. Cancer and the gut microbiota: an unexpected link. Sci Transl Med 2015; 7:271ps1; PMC4690201; http://dx.doi.org/10.1126/scitranslmed.3010473
- Dimova T, Brouwer M, Gosselin F, Tassignon J, Leo O, Donner C, Marchant A, Vermijlen D. Effector Vgamma9Vdelta2 T cells dominate the human fetal gammadelta T-cell repertoire. Proc Natl Acad Sci U S A 2015; 112:E556–65; PMID:25617367; http://dx.doi.org/10.1073/pnas.1412058112
- Bezanson J, Karpinski S, Shah VB, Edelman A. Julia: A fast dynamic language for technical computing. eprint arXiv:12095145 2012.
- Hochberg Y, Benjamini Y. More powerful procedures for multiple significance testing. Stat Med 1990; 9:811–8; PMID:2218183; http://dx.doi.org/10.1002/sim.4780090710
- Therneau TM, Grambsch PM. Modeling survival data: Extending the cox model. New York: Springer, 2000.