ABSTRACT
One of the mechanisms of tumor rejection in immune-modulatory treatments is antibody-dependent cell-mediated cytotoxicity (ADCC) of regulatory T cells (Tregs) that infiltrate tumors in which cells expressing activating Fcγ receptors (FcγRs) are present. Our objective was to identify, through a bioinformatics analysis, Treg marker(s) expressed at the highest levels in nine types of human cancers, in order to determine the best targets for ADCC-inducing antitumor antibodies.
We analyzed the mRNA levels of 24 surface Treg markers evaluated by the Affymetrix Human Genome U133 Plus 2.0 Array in 5728 cancer samples obtained via the Genevestigator v3 suite. Our analysis was based on overexpression of markers in tumors as compared to healthy tissues (HTs) and correlation between overexpression of the markers and the tumor suppressive microenvironment. Moreover, we evaluated tumoral infiltration of activating FcγR-expressing cells and calculated the ADCC index for each overexpressed marker, as an indicator of whether the marker was a good target for ADCC induction in tumor-infiltrating Tregs.
The results demonstrated that the ADCC strategy is unlikely to succeed in colorectal, liver, prostate and ovarian cancers. Moreover, we identified nine Treg markers that could be targeted in the other tumors: 4-1BB, CD39, galectin-9, GITR, IL-21R, LAP, neuropilin-1, TIGIT and TNFR2. GITR and TIGIT were the only markers that could be potentially useful as targets for the treatment of three cancers: non-squamous and squamous NSCLC and breast infiltrating ductal carcinoma. LAP, neuropilin-1 and CD39 presented as good targets in the treatment of renal cell carcinoma.
Our findings may have value for the development of new anti-tumor antibodies.
Introduction
Many monoclonal antibodies (mAbs) that target the immune system are under preclinical evaluation for their antitumor properties, and some of them are already used in the clinic and have shown interesting results. Their main mechanism of action is to elicit the activation of immune response to induce tumor rejection in the patient. This effect is attributable to several mechanisms, some of which occur concurrently, including direct T cell activation by either co-stimulation or inhibition of inhibitory signals (that mainly act on exhausted CD8+ T cells), direct activation of natural killer (NK) cells, indirect activation of cytotoxic CD8+ T lymphocytes (CTLs) and NK cells by modulation of antigen-presenting cells, and indirect activation of CTLs and NK cells by inhibition of regulatory T cells (Tregs). Indeed, Tregs play a pivotal role in promoting tumor growth by maintaining a suppressive microenvironment in tumors, mainly due to the expression of suppressive cytokines, such as TGF-β and IL-10.Citation1–5
Antibody-dependent cell-mediated cytotoxicity (ADCC) is the mechanism by which certain therapeutic mAbs (e.g., rituximab or trastuzumab) exert cytotoxicity against tumor cells.Citation6 ADCC requires binding of the mAb to Fcγ receptors (FcγRs) expressed by myeloid and NK cells, which leads to FcγR activation and promotes myeloid and NK cell activation. Recently, ADCC has been described as the main mechanism by which the anti-GITR DTA-1 mAb decreases the number of Tregs that infiltrate murine tumors and, thus, inhibits the suppressive microenvironment.Citation7 Further, a high percentage of treated mice recover from cancer and develop an immune memory exclusively against the cancer that they were affected with.Citation8 ADCC can be elicited by the anti-GITR Ab only if myeloid and NK cells express activating FcγR (e.g., murine FcγRIA, FcγRIII, and FcγRIV corresponding to human FcγRIA, FcγRIIA, FcγRIIC, FcγRIIIA, and FcγRIIIB).Citation7 Similar findings have been reported for the anti-tumor mAbs against CTLA-4 and OX40.Citation7,9,10 Thus, all these findings indicate that ADCC of Tregs is a powerful mechanism by which anti-tumor mAbs activate the immune system against tumor cells.
Several studies demonstrated that murine and human tumors are infiltrated by several Treg subsets including CD4+ thymus-derived Treg subsets (tTreg) and CD4+ peripherally-derived Treg subsets [pTreg, also called iTreg or adaptive Treg, e.g. T helper (Th)3 cells, T regulatory type 1 (Tr1) cells and GITR single positive cells)] that may not express FOXP3.Citation2,3,11,12 Each Treg subset is characterized by more than one Treg marker,Citation3,Citation12–20 and several Treg subsets are characterized by a tissue- and microenvironment-dependent plasticity.Citation11,21 Even though systematic studies on human tumors have not been performed, some studies indicate that the tumor-infiltrating tTreg and pTreg subsets differ across different types of tumors and that Tregs show a higher degree of heterogeneity in humans than in mice.Citation2,3,Citation22 Certain Treg markers (e.g., GITR, TNFR2, CTLA-4 and OX40) are expressed at higher levels in tumor-infiltrating Tregs than in peripheral Tregs, at least in certain types of tumorsCitation10,23–27 and some Treg subsets do not express the main transcription factor of Treg, FOXP3, or express it at low levels.Citation15,17,20 Moreover, several Treg markers and Treg-specific transcription factors are also expressed in other cell types and, in particular, in activated T cells,Citation28 and this makes the evaluation of Treg markers in tumors even more complex.
A crucial aspect of establishing an ADCC strategy is to determine which surface marker(s) of Tregs is/are expressed at the highest level in a tumor, in order to increase the number of mAbs bound per cell and the probability of Treg ADCC. Moreover, examining the differential expression of markers in tumor-infiltrating Tregs and tumor-infiltrating conventional T cells and peripheral Tregs appears crucial from the viewpoint of both treatment efficacy and safety. Tumor immune infiltrates, including Treg infiltrates, have been characterized by immunohistochemistry and flow cytometry in some tumors.Citation29,30 However, these approaches are limited by a number of factors, including the number of cell types and markers that can be assayed simultaneously, the amount of tissue required, and the cost. Moreover, technical differences between studies make it difficult to compare different tumors. Computational techniques applied to the gene expression profiles of bulk tumors can rapidly provide a wide range of data on tumor-infiltrating immune cells.Citation31–33 Thus, in the present study, we used a bioinformatics approach to characterize nine types of human cancers.
We performed a bioinformatics analysis using mRNA expression data from the Affymetrix Human Genome U133 Plus 2.0 Array on specimens from nine human cancers (breast infiltrating ductal carcinoma, colorectal carcinoma, liver carcinoma, non-squamous and squamous NSCLC, renal cell carcinoma, ovarian serous carcinoma and ovarian clear cell carcinoma, and prostate carcinoma) and tissue from healthy donors (HDs) obtained via the Genevestigator v3 suite.Citation34 In particular, we investigated which Treg markers are overexpressed in each tumor and whether they correlate with the presence of a suppressive microenvironment. In order to determine whether mAbs that promote ADCC would be effective for treatment of the tumors, the level of expression of activating FcγR was also evaluated. Finally, the probability of successful induction of ADCC by the Treg markers observed in each tumor was estimated by setting an ADCC index. In summary, we have demonstrated that (1) the level of infiltration of T cells, Tregs and cells expressing activating FcγRs differ across tumors, so the ADCC strategy may not be successful for all tumors; (2) the markers of Tregs that are expressed at the highest levels are different in different tumors; (3) within each tumor type, the variability of the expression of some Treg markers is higher than that of others, which makes certain markers potentially useful targets in a higher percentage of patients; and (4) certain Treg markers seem promising for the application of the ADCC strategy in specific tumors.
Results
T cell infiltration, CTL activity and Tregs in the tumors based on analysis of Affymetrix data
Several studies have analyzed the level of T cell infiltration in tumors using bioinformatics data from multiple datasets derived using different technologies (e.g., RNAseq and array releases).Citation29,31–33,35,36 In this study, we used the Genevestigator software to obtain data from the Gene Expression OmnibusCitation37 and European Bioinformatics InstituteCitation38 databases. Only data generated using the Affymetrix platform U133 Plus 2.0 were considered. Genevestigator does not consist of a mere collection of experiments, but it is a software that provides normalized data thus making possible the comparison within individual datasets and among datasets derived from the same tissue or different tissues (https://genevestigator.com/userdocs/manual/GENEVESTIGATOR_UserManual.pdf).Citation34
Our first aim was to compare the conclusions on T cell infiltration of tumors published in the literature with those obtained by our analysis. At the same time, we wanted to assess the presence of crucial T cell subsets in the nine tumor types that were analyzed.
First, we compared the expression of T cell genes in tumors with that in tissue samples from HDs (healthy tissues, HTs) by using the T cell infiltration score based on the expression of T cell genes proposed and validated by Bindea et al.Citation31 As reported previously by others,Citation30,33 our analysis shows that all the tumors were infiltrated by T cells (). However, the degree of infiltration was higher in some cancers than in others. In particular, the levels of T cell infiltration of in non-squamous NSCLC, squamous NSCLC, renal cell carcinoma, and breast infiltrating ductal carcinoma were significantly higher than the levels of infiltration of liver, prostate and ovarian carcinomas. Evaluating the expression of T cell genes proposed by Becht et al.Citation39 similar results were obtained (Figure S1).
Figure 1. Infiltration of tumors by T cells and T cell subsets. (A) The T cell infiltration score was calculated by evaluating the mRNA expression of genes included in the T cell signature proposed by Bindea et al.Citation31 However, the genes TRA and TRAC are not included in the Affymetrix platform and therefore were not considered. The ratio of the expression in the patient's tumor sample and the mean expression in the HTs was calculated for each gene. Then, the mean ratio of the genes in each patient was expressed as log(2). The mean normalized expression of genes included in the T cell signature in tumors T1, T2, T3 and T4 was found to be significantly higher as compared to T6, T7, T8 and T9 (####, p<0.0001). (B) The CTL activity score was calculated by evaluating the mRNA expression of genes included in the CTL signatureCitation31 normalized to the expression of CD8α. The ratio of the expression in the patient's tumor sample and the mean expression in the PBMCs of HDs was calculated for each gene. Then, the mean ratio of the genes in each patient was expressed as log(2). The mean normalized expression of genes included in the CTL signature in each tumor was found to be significantly lower (##, p<0.01; ###, p<0.001; ####, p<0.0001) or not significantly different (n.s.) as compared to the mean normalized expression in PBMCs of HDs. (C) The suppressive score was calculated by evaluating FOXP3, IL-10 and TGFβ mRNA expression normalized to the expression of CD4. The ratio of the expression in the patient's tumor sample and the mean expression in the PBMCs of HDs was calculated for each gene. Then, the mean ratio of the genes in each patient was expressed as log(2). The mean normalized expression of FOXP3, IL-10 and TGFβ in each tumor was found to be significantly higher as compared to the mean normalized expression in PBMCs of HDs (####, p<0.0001). (D) Correlation of TGFβ mRNA expression and CD4 mRNA expression in patients with non-squamous NSCLC. The Spearman coefficient (ρ) and p value are reported. (E) Correlation of IL-10 mRNA expression and CD4 mRNA expression in patients with renal cell carcinoma. Spearman coefficient (ρ) and p value are reported. All box-and-whisker plots (A-C) represent values within the interquartile range (boxes), and whiskers represent the highest and the lowest values.
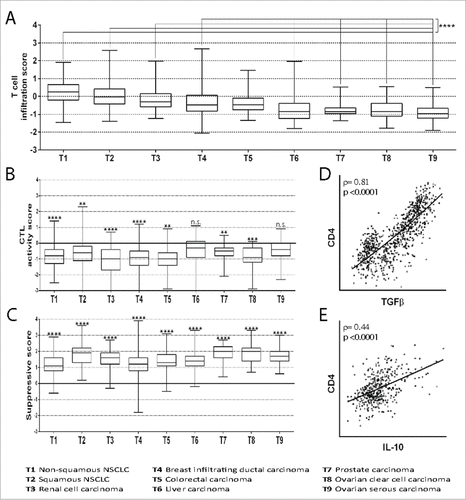
Level of infiltrating CD4+ and CD8+ T cells correlated significantly with T cell infiltration in patients who had the same cancer (Table S1), which indicates that the tumors were infiltrated by both CD4+ and CD8+ T cells.
We next investigated the CD8α and CD4 mRNA expression in tumors and peripheral blood mononuclear cells (PBMCs) from HDs (), and we found that the CD8α/CD4 mRNA ratio is significantly lower in all the tumors than HTs, which is in accordance with previous reports.Citation40,41 Moreover, the percentage of CTLs (i.e. activated CD8+ T cells) in CD8+ T cells is significantly lower in all the tumors than in PBMCs from HDs (), with the exception of liver and ovarian serous carcinomas that are similar to PBMCs from HDs. Other bioinformatics studies on single tumor types have reached similar conclusions.Citation42,43
Table 1. Decrease in the CD8+/CD4+ ratio in cancer specimens as compared to PBMCs from HDs.
It is generally believed that tumors are characterized by a suppressive microenvironment.Citation1,40,42,44,45 To investigate whether this was demonstrated by our bioinformatics approach, too, we set up a suppressive score based on the expression of FOXP3, the transcription factors expressed in all subsets of tTregs and in Th3 cells, and the suppressive cytokines TGFβ and IL-10 that are expressed by several pTreg and tTreg subsets and other immune cells.Citation46–48 The expression of suppressive genes was normalized to that of the CD4 gene in tumors and PBMCs from HDs, and the suppressive score was determined by calculating the tumor/PBMC ratio. shows that in all tumor types, the proportion of cells favoring a suppressive microenvironment is much higher than that in PBMCs (p<0.0001 in all tumors), with some differences between different types of tumors. To further investigate the suppressive microenvironment, we examined whether TGFβ and IL-10 expression was correlated with CD4+ T cell infiltration in patients who had the same cancer. and and show that TGFβ expression is correlated with CD4+ T cell infiltration in patients with all carcinomas, although the degree of correlation varies, and that IL-10 expression is correlated with T cell infiltration in non-squamous NSCLC, kidney, liver and ovarian serous cancers. Our findings are in agreement with other bioinformatics studies performed on some tumor types.Citation30,31,35,36 Moreover, the suppressive microenvironment appears to be specific to each tumor, as indicated by the difference in the degree of correlation between T cell infiltration and TGFβ and IL-10 expression.
Table 2. Correlation between mRNA expression of the regulatory cytokines and the mRNA expression of CD4 in cancer specimens.
We next investigated whether T cell infiltration correlated with the expression of genes related to CTL activity in patients who had the same cancer (). Interestingly, CTL activity was inversely correlated with T cell infiltration in all tumors with a p≤0.0019 and a Spearman correlation coefficients ranging between −0.33 and −0.70. This finding confirms that tumor-infiltrating T cells (likely CD4+ T cells, as suggested by and S1) inhibit CTL activity due to the expression of suppressive cytokines (as suggested by ).
Table 3. Correlation between the mean mRNA expression of genes representing T cells (see Figure S1) and the mean mRNA expression of genes representing CTL, normalized by CD8α expression, in cancer specimens.
In conclusion, data obtained using the Genevestigator software depict the immune landscape of tumors with regard to T cell infiltration and CTL activity, in a way that is similar to other bioinformatics analyses. As expected, high levels of T cell infiltration correlate positively with high levels of suppressive cytokines, suggesting that tumor-infiltrating CD4+ cells are biased toward a regulatory phenotype. Indeed, the higher is the level of infiltrating T cells, the lower is the CTL activity, confirming that the reduced CTL activity in tumors depends on the Treg-dependent suppressive microenvironment.
Overexpression of specific extracellular markers of Tregs in certain tumors
Murine Tregs are known to be heterogeneous with regard to both their phenotype and function.Citation3,Citation11–19 The heterogeneity of Tregs appears to be even more striking in humans.Citation1–3,22 Intratumoral Tregs have certain features that distinguish them from peripheral Tregs, including the expression of proteins related to their recruitment within tumors, their expansion and their activity.Citation1 Several studies have evaluated the features of Tregs in specific tumors, but none of them have investigated whether tumor-infiltrating Tregs differ across tumors. Therefore, in this investigation, we analyzed the expression, in tumor samples, of genes that are considered to code Treg markers or proteins that, at least in some contexts, are overexpressed by Tregs (tTregs, pTregs or both). Based on the hypothesis that ADCC of Tregs induces tumor rejection, our objective was to identify the best Treg marker(s) for each tumor, so that Tregs can be targeted by ADCC-inducing antibodies directed against those specific markers. Therefore, we focused only on the expression of markers found on the surface of the cells. However, we also considered two proteins secreted by Tregs: galectin-9Citation49 and LAP.Citation28,50 Galectin-9 binds extracellular markers of Tregs, such as Tim-3,Citation51 4-1BB,Citation52 and CD44,Citation53 and can be detected by antibodies on the Treg surface.Citation52 LAP is synthesized from the TGFB1 gene as a pro-TGF-β precursor that is cleaved to form mature TGF-β and LAP, which are non-covalently assembled (latent TGF-beta binding protein), secreted and anchored on the surface of Tregs trough GARP.Citation54 The other 22 genes were chosen because they have been reported to be expressed at high levels in at least one Treg subset (see Table S2 of the supplemental material), even if they were expressed at lower levels by other cells, including activated T cells. Even though we strived to include all the relevant surface Treg markers, it is possible that some less characterized surface Treg markers were not included in our study.
The expression levels of the Treg markers in tumor tissues were compared with the expression levels of the same markers in HTs. The comparison was performed in two ways: the mean expression of a marker in a tumor was compared with (1) its mean expression in the corresponding HT (the tissue from which the tumor was derived), and (2) its mean expression in all the HTs. In particular, markers were selected for further analysis when they were significantly overexpressed (p<0.05) and had an overexpression: (A) higher than two-fold in the tumor than in the corresponding HT or (B) higher than 1.5-fold in the tumor than in the corresponding HT and higher than 1.5-fold in the tumor than the mean expression in all HT samples.
and Table S3 show that several (but not all) Treg markers have significantly higher expression in some (but not all) tumors: 8 and 5 Treg markers were overexpressed in non-squamous and squamous NSCLC, respectively; 9 Treg markers were overexpressed in renal cell carcinoma; 10 Treg markers were overexpressed in breast infiltrating ductal carcinoma; 2 Treg markers were overexpressed in colorectal carcinoma; 1 Treg marker was overexpressed in liver carcinoma; no Treg markers were overexpressed in prostate carcinoma; 2 and 2 Treg markers were overexpressed in ovarian serous cysto-adenocarcinoma and clear cell adenocarcinoma, respectively. To evaluate whether certain markers were useful for the treatment of prostate carcinoma, as a rescue strategy, we selected the previously excluded markers for which the ratio of expression in the tumor to expression in the corresponding HT was between 1.5 and 2.0 (p<0.05) (dotted boxed markers in ). This condition was satisfied only by CD103.
Figure 2. Expression of Treg markers in tumors vs. HTs. The ratio of expression of the marker in the tumor to its expression in HTs is shown on a log(2) scale. Markers with higher than two-fold expression in the tumor compared to the corresponding HTs (#, p<0.05) or higher than 1.5-fold expression in the tumor compared to both the corresponding HTs (#, p<0.05, as evaluated by Student's t-test) and the mean expression in HTs (#, p<0.05) were selected for further analysis (markers within grey boxes). As a rescue strategy (prostate carcinoma), markers showing higher than 1.5-fold expression in the tumor as compared to the corresponding HTs (#, p<0.05) were selected for further analysis (marker within grey dotted boxes). Markers that met the expression conditions but were not selected because the difference between tumors and HTs was not significant (n.s.) are shown within white boxes.
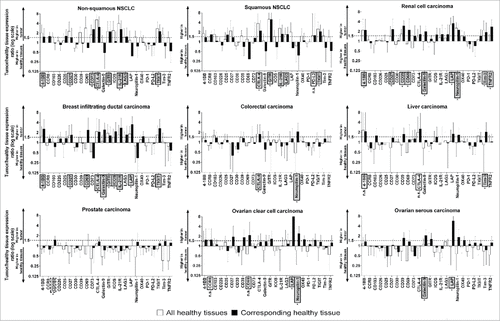
To estimate the number of patients in whom the overexpressed markers could be targeted by an ADCC-inducing mAb, we determined the percentage of patient-derived samples in which the expression of the identified Treg marker was higher than the mean+2SD value in the corresponding HTs. In , markers that met the overexpression criterion (>mean+2SD expression) in at least 40% of the patients are reported. In supplemental Figures S2-S7, the expression levels of the markers that met the criterion are shown. shows the marker that was overexpressed in the highest number of patient-derived specimens for each tumor type. No marker satisfied the overexpression criterion in colorectal, liver and prostate carcinoma (). Thus, the strategy of targeting Tregs to induce ADCC in these cancers is unlikely to be effective.
Table 4. Overexpression of Treg markers in specific tumors.
Figure 3. mRNA expression of the best Treg marker in tumor samples and the corresponding HTs. Mean±SD values of expression of the Treg marker in HTs and expression of the marker in each tumor sample are shown. The percentage of patients with expression greater than the mean+2SD value (dotted line) of the corresponding HTs is reported. The best Treg marker for each tumor is shown (the expression of the other selected Treg markers are shown in supplemental Figures S2−S7). Student's t-test was used for statistical analysis in the comparison between the expression level of the Treg marker mRNA in tumors vs. the corresponding HT samples.
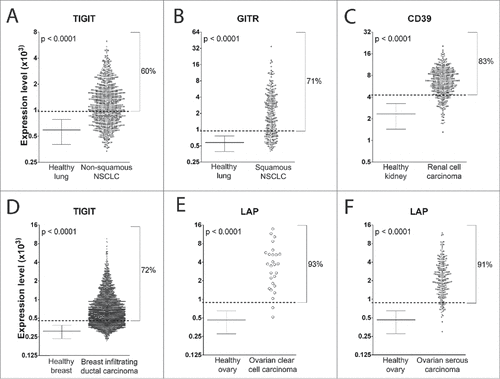
In summary, 11 markers met the overexpression criterion: 4-1BB, CD39, CD73, Galectin-9, GITR, ICOS, IL-21R, LAP, neuropilin-1, TIGIT, and TNFR2. The most interesting markers were those that were overexpressed in 70−90% of the patients with squamous NSCLC, renal cell carcinoma, breast infiltrating ductal carcinoma, ovarian serous carcinoma and clear cell carcinoma. In the case of non-squamous NSCLC, the most interesting Treg marker was the one overexpressed in 60% of the patients. Therefore, the present data demonstrate that more than half of the patients overexpressed at least one Treg marker (i.e., CD39, GITR, LAP or TIGIT). However, each of these Treg markers is overexpressed only in some tumors.
Correlation between the expression of Treg markers and regulatory cytokines
Several studies have demonstrated that the markers identified by our analysis are true Treg markers (Table S2), even though they are are also expressed in non-Treg cells, at least in some conditions.Citation1,11,19 However, the tumor microenvironment is tumor specific and differs across tumors.Citation1–3 We have shown that CD4+ T cell infiltration correlates positively with TGF-β and IL-10 expression and T cell infiltration correlates inversely with CTL activity, likely suggesting that the suppressive microenvironment within the tumor mass depends on TGF-β and IL-10 expression. Therefore, to confirm that overexpression of Treg markers is correlated with a higher number of Tregs and a suppressive microenvironment in a tumor, we examined whether the expression of Treg markers is directly correlated with the expression of the regulatory cytokines IL-10 and TGFβ in samples from patients with the same tumor type. Since all tumors had evidence of Treg infiltration (), expression of all the Treg markers was evaluated in all tumors. For each significant (p<0.05) direct correlation found between a marker and the expression of suppressive cytokines, a score of +3 (ρ>0.3) or +1 (0.3>ρ>0.1) was assigned, and for each inverse correlation, a score of −3 (ρ<−0.3) or −1 (−0.3<ρ<−0.1) was assigned. No score (0) was assigned when no correlation was found. For example, we found a direct correlation between GITR and TGFβ mRNA expression (ρ>0.3, ) and between GITR and IL-10 mRNA expression (ρ>0.3, ) in non-squamous NSCLC, so the total regulatory score of GITR in this tumor was 6. In Table S4, the scores for each Treg marker in each tumor are reported. When we found a negative score, we assumed that the marker is not a proper Treg marker in the microenvironment of that tumor (and possibly represents an activation marker). On the contrary, when we found a positive score, we assumed that the marker is a proper Treg marker in the microenvironment of that tumor. A neutral score (0) possibly indicates that the marker is not prominently expressed in Tregs.
Figure 4. Correlation between the expression of GITR and suppressive cytokines. Correlation of GITR mRNA expression with TGFβ (A) and IL-10 (B) mRNA expression in patients with non-squamous NSCLC. Spearman coefficients (ρ) and p values are reported.
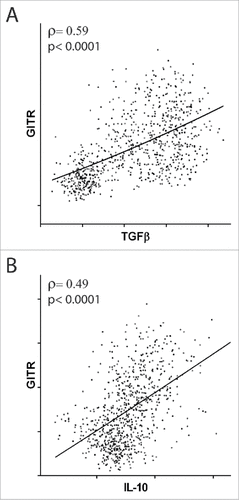
shows that the expression of nine markers (38% of the markers) was correlated with a suppressive microenvironment in all the tumors or all but one tumor; these markers were categorized as class A markers. When a marker was associated with the suppressive microenvironment in six or seven tumors, it was categorized under class B. Six such markers were identified, which represented 25% of the markers evaluated. When a marker was associated with the suppressive microenvironment in only five tumors, it was categorized under class C (five such markers were identified, which represented 21% of the markers evaluated). Four markers (17% of the total markers) were correlated with the suppressive microenvironment in less than half of the tumors and were categorized under class D.
Table 5. Correlation of the expression of Treg markers with that of the suppressive cytokines in tumor samples.
Of the 11 Treg markers that met the overexpression criterion (, grey background), 6 (55%) belong to class A and 9 (82%) belong to classes A and B. Thus, most of the Treg markers overexpressed in tumors and selected by our analysis correlate very well or well with the tumor suppressive microenvironment. Only 2 selected markers belong to class C, and none of the selected marker belong to class D.
Tumor infiltration by NK and myeloid cells expressing activating FcγRs
In murine models, anti-GITR, anti-OX40 and anti-CTLA-4 mAbs induce ADCC in Tregs when they bind to activating FcγRs expressed by NK and myeloid cells.Citation7,9,10 Thus, it is likely that this condition is necessary for all mAbs that are involved in inducing ADCC. To verify whether mAbs directed against Treg markers can induce ADCC in human tumors, we evaluated the expression of activating FcγR mRNA in tumors that overexpress Treg markers and compared it to their expression in lymph nodes, where activating FcγR-expressing cells are virtually absent.Citation7
shows that activating FcγR mRNAs are significantly (p<0.05) overexpressed in all tumors as compared to lymph nodes and, among them, FcγRIIIA mRNA shows the highest level of overexpression. When the mean level of overexpression is considered, NSCLC and renal cancer show a higher level of activating FcγR mRNA overexpression than the other cancers, and ovarian serous carcinoma shows the lowest level of activating FcγR mRNA overexpression. This means that the ADCC strategy is more likely to be effective in NSCLC and renal cancer than in ovarian serous carcinoma.
The expression of FcγRIIC is not shown in Fig. 5 because the level of the expression of this receptor in tumors was 10-fold lower than that of the other activating FcγRs (not shown), which means that it is unlikely to play a role in ADCC in cancers.
Figure 5. Expression of activating FcγR mRNA in tumors vs. lymph nodes from HDs. The ratio of the mRNA expression of the specified activating FcγR in the tumor specimen and the mean (±SD) mRNA expression in the lymph nodes of HDs is shown on a log(2) scale. In the histogram on the right, the mean of the ratios [expressed as log(2)] of the four activating FcγRs is shown. The mean expression of each FcγR in each tumor as compared to the mean expression in HD lymph nodes was found to be significantly different (#, p<0.05: ##, p<0.01: ###, p<0.001; ####, p<0.0001).
![Figure 5. Expression of activating FcγR mRNA in tumors vs. lymph nodes from HDs. The ratio of the mRNA expression of the specified activating FcγR in the tumor specimen and the mean (±SD) mRNA expression in the lymph nodes of HDs is shown on a log(2) scale. In the histogram on the right, the mean of the ratios [expressed as log(2)] of the four activating FcγRs is shown. The mean expression of each FcγR in each tumor as compared to the mean expression in HD lymph nodes was found to be significantly different (#, p<0.05: ##, p<0.01: ###, p<0.001; ####, p<0.0001).](/cms/asset/031af5d5-b9e6-4f91-b26f-e50d4646ca08/koni_a_1387705_f0005_b.gif)
The ADCC index as an indicator of the effectiveness of Treg markers for ADCC induction
The main objective of the study is to identify markers that have the highest probability of being Treg markers of tumor-infiltrating Tregs so that an antibody directed against the marker can induce Treg ADCC, provided that activating FcγRs are also present in the tumor. Therefore, we calculated the probability of each marker being an effective target in a tumor by means of the ADCC index. The ADCC index was calculated considering four parameters (): (1 and 2) the “Treg marker expression ratio A score” and “Treg marker expression ratio B score” parameters which consider the overexpression of the marker in the cancer as compared to its expression in the corresponding HT and in all HTs, respectively; (3) a tumor-related parameter which considers the level of infiltration of activating FcγR expressing cells called “Activating FcγRs expression ratio score”; (4) a parameter describing the marker as a Treg marker (score>1) or a marker of activated conventional T cells (score<1) in the tumor microenvironment, called “Treg marker suppressive score”; it derives from the multiplication of the “Treg marker suppressive score in the specific cancer” by “Treg marker suppressive score in all cancer”. The scores of the first 3 parameters range between 1 (low overexpression level) and 4 (high overexpression level). The scores of the fourth parameter range between 0.25 (marker of activated conventional T cells) and 4 (very good Treg marker). Markers with an ADCC index of 48 or higher were considered to be very interesting for the tumor for which the index was calculated. The scores, the formula combining the parameters and the 48 cut-off were chosen for the following reasons: (A) a selected marker must be expressed by Tregs; therefore, if the “Treg marker suppressive score” is equal to 0.25 or 0.5 (meaning that the marker is mainly an activation marker of conventional T cells), the ADCC index is under the cut-off (16 or 32, respectively) even if the other scores are equal to the maximum; (B) all considered parameters contribute to increase the likelihood of ADCC; therefore if two scores are equal to the minimum (1), even if the other scores are equal to the maximum (4), the ADCC index is under the cut-off (16); (C) if three scores are equal to 2, even if the other score is equal to 4, the ADCC index is under the cut-off (32); (D) if two scores are equal to 2, one score is equal to 3 and one score is equal to 4, the ADCC index is considered good (48). Obviously, the higher is the ADCC index, the higher is the probability that the marker targeted by an antibody will promote ADCC of Tregs.
Figure 6. Algorithm used to calculate the ADCC index of Treg markers in a cancer. To evaluate the probability of a Treg marker being successfully used as a target to induce ADCC, we established the ADCC index. The ADCC index was calculated for each marker in each cancer as described in the material and methods. Half of the score is derived from evaluation of overexpression of the marker in the responder patients (i.e., the patients in whom the Treg marker expression level was higher than the mean+2SD value of the Treg marker expression in HTs), one-fourth from the expression of activating FcγR in the cancer sample of the responder patients, and one-fourth from the correlation of the marker expression with the suppressive microenvironment (i.e., the probability that the marker was mainly expressed in Tregs). The final score was obtained by multiplying each of these four scores. The ADCC scores of the markers in specific cancers are shown in .
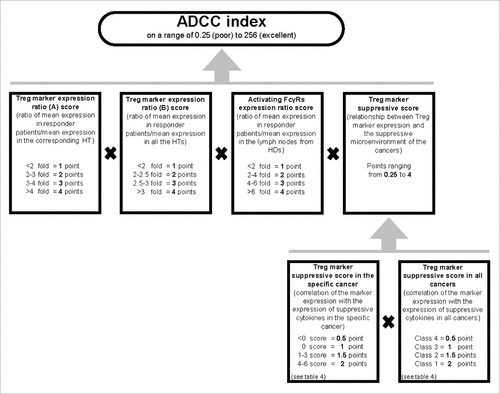
The ADCC index for the markers that met the overexpression criterion () is shown in . One marker has an ADCC index below the cut-off in the cancer where it is overexpressed, three markers have an ADCC index equal or above the cut-off in one cancer and below in others, and six markers have an ADCC index equal or above the cut-off in one or more cancers. In the case of NSCLC cancers and breast infiltrating ductal carcinoma, we identified two Treg markers for which the ADCC strategy appears to have good chances of success: GITR and TIGIT. In the case of breast infiltrating ductal carcinoma, two more markers appear to be of interest: 4-1BB and IL-21R. In the case of renal cell carcinoma, five Treg markers were found to be potentially good targets, among which LAP and neuropilin-1 have the highest ADCC index. Even though LAP is overexpressed in a large percentage of ovarian carcinomas, the infiltration of cells expressing activating FcγRs is relatively low, and therefore, the probability of inducing ADCC with an anti-LAP Ab appears to be low in the case of ovarian carcinomas.
Table 6. ADCC index of the markers overexpressed in more than 40% of the patients.
Discussion
Tumor specificity of overexpressed Treg markers
In preclinical studies, mAbs that favor the anti-tumor response by inhibiting the suppressive microenvironment have provided very interesting results,Citation55,56 and one of the mechanisms through which these antibodies function is the induction of ADCC on Tregs.Citation7,10,55-58 This study investigates the expression of Treg markers in different tumors to identify the best markers that can be targeted by anti-tumor mAbs that induce ADCC. Several studies have shown that ADCC is tumor specific (i.e., it involves tumor-infiltrating Tregs more than Tregs outside the tumor) and, thus, favors the killing of Tregs directed against tumor antigens.Citation7,9,10,57,58 ADCC is specifically targeted against tumors because it requires the expression of activating FcγRs, which are not expressed by cells in the lymph nodes or other lymphoid organs.Citation7,9,10 Therefore, the ADCC mechanism may be very effective and associated with a low rate of adverse events. However, it is crucial that the antibody targets the correct Treg marker, that is, the marker that is expressed at high levels in a large percentage of tumor-infiltrating Tregs and at much lower levels in non-Treg cells within the tumor (to avoid killing CTLs, for example) and in Treg-infiltrated HTs (to avoid adverse effects). To identify appropriate markers for the nine types of cancers that have been investigated in this study, we proposed the ADCC index as an indicator of the best potential markers of Tregs in each tumor. Among the 24 Treg markers analyzed, only 11 markers appeared to be of some interest, and out of these, 9 markers had a sufficiently high ADCC index (>48) in at least one tumor: 4-1BB, CD39, galectin-9, GITR, IL-21R, LAP, neuropilin-1, TIGIT and TNFR2.
We found that the Treg markers of interest ( and ) are not the same in all tumors. With regard to the best markers, both NSCLC cancers and breast cancers have two Treg markers in common (GITR and TIGIT) and IL-21R, with a low ADCC index in non-squamous NSCLC and a good ADCC index in breast cancer. In contrast, 4-1BB is specific to breast cancer. Further, for renal cell carcinoma, we found five interesting Treg markers. However, none of the five markers was of interest in NSCLC and breast cancers, although galectin-9 is a weaker target in breast cancer. Ovarian cancers share the markers LAP and galectin-9 with renal cell carcinoma. Thus, it is possible that different Treg subsets infiltrate different tumors, or that some markers are specifically upregulated in a tumoral microenvironment. This phenomenon has been described for GITR, TNFR2, CTLA-4 and OX40 in mouse and human tumors,Citation10,23–27 and is very interesting from a therapeutic point of view. This is because specific antibodies bind better to cells expressing the corresponding antigen at a high density, and this indicates a higher probability of ADCC within tumors. In conclusion, the use of a specific mAb eliciting ADCC of Tregs would be effective only in certain tumor types.
Effectiveness of the ADCC strategy
The effectiveness of ADCC strategy would depend on the expression of specific markers on T cells as well as the expression of FcγRs, which are required for the induction of ADCC. In the present study, in five of the nine evaluated human tumors, the strategy of treating tumors by promoting ADCC of Tregs appears unlikely to succeed. In the case of colorectal, liver and prostate carcinomas, the overexpressed markers are a few and are overexpressed in less than 40% of the patients (CD103, CTLA-4, neuropilin-1 and Tim-3) (). Among these markers, Neuropilin-1 is the only one with an interesting ADCC index (48, not shown). Thus, targeting neurolipin-1 may be effective in the few patients with colorectal carcinoma in whom it is overexpressed.
In the case of ovarian serous carcinoma, the strategy of treating tumors by Treg ADCC appears unlikely to succeed because of the low overexpression of the markers as compared to all tissues and the relatively low number of cells that express activating FcγRs. Thus, the ADCC index for LAP and galectin-9 was low (). In the case of ovarian clear cell carcinoma, the low overexpression of LAP as compared to all tissues is the main reason for the low ADCC index. However, the number of samples of ovarian clear cell carcinoma in the database was quite low (27), so the results may be biased.
Thus, based on our findings, the ADCC strategy is very likely to succeed in only four of the tumors examined: non-squamous and squamous NSCLC, breast infiltrating ductal carcinoma and renal cell carcinoma. Interestingly, these tumors are more infiltrated by T cells than the other tumors (A). However, the effectiveness of the ADCC strategy may depend on several factors, including other activity of the mAb (e.g., direct co-stimulation of conventional T cells), the direct effect of the mAb on the tumor, and the presence of other immune suppression mechanisms that may allow for tumor immune escape when Tregs are eliminated by ADCC.
Candidate markers for the ADCC-inducing strategy
Data reported by the Cancer Genome AtlasCitation36 show that GITR, a co-stimulatory molecule,Citation59 is overexpressed in lung and breast cancers (Figure S8). This is in agreement with our study findings, which show that GITR has a high probability of being a good marker in these tumors (). In the Cancer Genome Atlas, GITR appears to be overexpressed in renal and liver cancers, too, but our analysis did not reveal GITR overexpression in renal cell and liver carcinomas. It has been demonstrated that GITR is one of the best markers of activated pTregs, particularly within the tumor microenvironment,Citation11 and that the expression of GITR+ Tregs is correlated with malignancy.Citation25 Further, the anti-GITR Ab DTA-1 has been demonstrated to be effective in several tumor models,Citation55,58 and several studies demonstrate that the main mechanism by which the DTA-1 Ab exerts its antitumor activity is ADCC of tumor-infiltrating Tregs.Citation7,60–62 Our study suggests that GITR is a very promising target for antitumor treatment of non-squamous and squamous NSCLC (the ADCC indexes were among the highest observed) and breast infiltrating ductal carcinoma. Analysis of the Atlas samples indicate that GITR may also be a target for certain renal cell carcinoma subtypes or in specific stages, which might be underrepresented in our datasets.
TIGIT was initially described as a co-inhibitory molecule in conventional CD4+ T cells.Citation63 More recently, it has been demonstrated that TIGIT expression defines a functionally distinct tTreg subset with an activated phenotypeCitation64 and that TIGIT signaling in Tregs suppresses antitumor immunity via Tregs in murine tumor-infiltrating lymphocytes.Citation65 In humans, too, TIGIT is highly expressed and upregulated on Tregs after activation and in vitro expansion.Citation66 According to our analysis, TIGIT appears to be a very interesting target in the same cancer types in which GITR triggering plays a role.
LAP-expressing CD25+ cells are a subset of tTregs with great suppressive activity.Citation67 Moreover, LAP is expressed also by FOXP3− pTregs.Citation68 Interestingly, the number of LAP-expressing Tregs is significantly higher in the PBMCs of patients with cancers than in the healthy controls.Citation69,70 According to our analysis, LAP appears to be an ideal target in the treatment of renal cell carcinoma and the best target in the treatment of ovarian carcinomas.
CD39, TNFR2, and galectin-9 were found to be among the Treg markers with the best correlation with the regulatory microenvironment in all the analyzed tumors () and had a good ADCC index for renal cell carcinoma. CD39 is an ectonucleotidase that can hydrolyze ATP to 5′-AMP and adenosine, and thus mediate the suppression of T cells and NK cells.Citation71 Further, anti-CD39 Abs with potential antitumor activity have been patented.Citation72 TNFR2, along with GITR and OX40, plays a crucial role in the maturation of tTregs,Citation73 and the most suppressive Tregs can express high amounts of TNFR2 in various cancers.Citation74 Antagonistic anti-TNFR2 mAbs not only inhibit Tregs but also kill ovarian cancer cells in vitro.Citation26 Secreted galectin-9 may promote pTreg differentiation and bind to receptors expressed on Treg.Citation49,51–53 However, it can also play a role in CD8+ T cell exhaustion,Citation53 which means that it would be more appropriate to use antagonistic antibodies against galectin-9 than an ADCC-targeting strategy in tumors that overexpress galectin-9. In our study, galectin-9 was also found to be overexpressed in breast infiltrating ductal carcinoma and ovarian serous carcinoma ( and ). CD39, TNFR2 and galectin-9 are also expressed by certain tumor cells,Citation26,53,75,76 so their observed upregulation may be attributable, at least in part, to their expression by tumor cells. Therefore, targeting these markers may cause ADCC of both Tregs and tumors.
Comparison with other bioinformatics studies
Several studies have evaluated the infiltration of immune cells in tumors via a bioinformatics approach.Citation29,31–33,35,36,43,77,78 All these studies, including ours, have concluded that tumors are infiltrated by T cells to various degrees, with a high level of differences between patients, and that these lymphocytes create a suppressive microenvironment. In particular, several studies have demonstrated that Tregs are present at high levels in tumors and that the CD4+/CD8+ ratio is increased in tumorsCitation30,31,35,36,40,41; these findings were also confirmed by our study.
De Simone et al. obtained tumor-infiltrating lymphocytes from human colorectal and lung cancers and isolated T cell subsets, including Tregs, to evaluate their gene expression profile.Citation29 Interestingly, they demonstrated that tumor-infiltrating Tregs in lung cancers express several Treg markers at levels higher than HT-infiltrating Tregs, including 4-1BB, CD27, CTLA-4, LAG-3, and TIGIT, which have also been found to be overexpressed in the NSCLC samples analyzed in the present study (). On the contary, GITR was found to be overexpressed at similar levels in both tumors and healthy lung as compared to PBMC, thus to be not upregulated in NSCLC. Moreover, the Treg markers that were reported by De Simone et al. to be overexpressed in the Tregs infiltrating colorectal cancer were not found by our study, with the only exception of CTLA-4. In our opinion, the discordance between our study and the De Simone et al. study can be explained as follows: (1) The results of the De Simone et al. study did not account for differences in the number of tumor-infiltrating Tregs and tissue-infiltrating Tregs (2) De Simone et al. isolated and characterized only tumor-infiltrating tTregs (CD4+CD25+CD127− which are FOXP3+). On the contrary, our approach considered all tumor-infiltrating Treg subsets, including pTreg subsets, which may not express FOXP3 or express it at lower levels.Citation15,17,20
Plitas et al. studied tumor infiltrating tTregs from breast cancer specimens.Citation77 They demonstrated that the number of Tregs and the CD4+/CD8+ T cell ratio was higher in tumors than in breast parenchyma, which is similar to our observations. They then characterized tumor-resident Treg cells and compared them to normal tissue-resident ones and demonstrated that CCR8 is upregulated in tumor-resident Treg cells. On the contrary, we found that CCR8 was upregulated by only 1.3-fold in breast-infiltrating ductal carcinoma samples as compared to HT. However, in the Plitas et al. study, the gene signature of tumor-infiltrating pTregs was not studied. Therefore, our results are not comparable to theirs.
Limitations and strengths of the study
One of strengths of our study is that we evaluated tumor infiltration of Tregs in nine cancers by using data obtained with just one technique that provides the absolute values of gene expression and after normalization through Genevestigator suite making possible to pool data from several datasets. Pooled data for the analysis of prostate and ovarian clear cell carcinomas were obtained from three laboratories; data for analysis of squamous NSCLC, renal cell carcinoma, liver carcinoma and ovarian serous carcinoma were obtained from 5−7 laboratories; non-squamous NSCLC and colorectal carcinomas, 9−12 laboratories; and breast infiltrating ductal carcinoma, 33 laboratories (Table S5). The number of markers selected by our analysis does not correlate with the number of datasets analyzed (not shown); thus, the number of datasets does not represent a systemic bias in selecting Treg markers.
We believe that tumors are infiltrated by several Treg subsets. Therefore, we did not attempt to evaluate the signature of Tregs but only evaluated Treg markers, so that all Tregs were considered. Interestingly, CD25, a pivotal marker of tTregs, was not overexpressed in the tumors ( and Table S3) and had an overall score of 16 as far as concerns correlation with the suppressive microenvironment of tumors (ranked fourteenth among the Treg markers, ), confirming that Tregs different from tTregs play a more relevant role in tumors than that played by tTregs, as demonstrated by others.Citation3
A limitation of this study is that the level of mRNA expression of the markers did not always correlate with the level of protein expression. However, it is known that GITR, TNFR2 and CTLA-4 are overexpressed in tumor-infiltrating Tregs.Citation10,23–27 Thus, the demonstrated overexpression of these markers in tumors may correlate with their protein expression.
Another limitation of this study is that the bioinformatics analysis of mRNA levels does not establish whether the genes that are overexpressed are present only on Tregs or on other cells infiltrating the tumor as well. We have tried to overcome this limitation in two ways. First, we chose to analyze genes that are considered to code true Treg markers (see Table S2),Citation11,21,29 although we are aware that surface molecules exclusively expressed by Tregs do not exist. Second, in patients with the same tumor type, we analyzed the correlation between the level of expression of the Treg markers with the level of expression of IL-10 and TGFβ, two cytokines known to be expressed at high levels in a suppressive microenvironment. We reasoned that if the expression of a marker correlated directly with IL-10 and TGFβ expression, the marker would be expressed in tumor-infiltrating Tregs. However, even such a correlation is not a direct demonstration of exclusive expression of the marker in tumor-infiltrating Tregs. Even though some of the conclusions of our studies confirm data obtained for murine models (as discussed above), which indicates the possible success of this strategy, experimental studies on tumor-infiltrating Tregs should be performed to verify these findings. In this context, our study indicates which markers should be prioritized in future experimental studies.
Finally, we did not evaluate the carcinoma subtypes of renal cancer cells. In fact, our initial hypothesis was that the most highly expressed Treg markers are common to all tumors or, at least, are tissue specific. However, the hypothesis was verified only in part by the findings for lung and ovarian cancers.
Conclusion
In conclusion, our study findings indicate that the ADCC strategy could be successful for the treatment of certain tumors but not others. Moreover, the markers to be targeted by ADCC-inducing antibodies differ across different tumors. We have, therefore, proposed a method for the calculation of the ADCC index of a marker for a particular tumor, which may prove useful for the development of anti-tumor antibodies that elicit ADCC in tumor-infiltrating Tregs in humans.
Materials and methods
Data source, tumor types and control samples
We used the Genevestigator v3 suite,Citation34 by which the normalized absolute values of gene expression generated using the Affymetrix Human Genome U133 Plus 2.0 platform can be downloaded. The microarray data in Genevestigator are normalized at 2 levels: RMA within experiments (through the Bioconductor package “affy”Citation79 and a customized version of the package “affyExtensions”) and trimmed mean adjustament to a target for normalization between datasets. Regarding the second level, the trimmed mean consists of calculating the mean of all expression values of an experiment (across all samples) after excluding the top 5% and the bottom 5%. The combination of the 2 levels makes data highly comparable among different experiments thus making possible to pool data without further normalization. Gene expression data were obtained from datasets that are publicly available from Gene Expression OmnibusCitation37 and the European Bioinformatics Institute.Citation38
The Genevestigator database was queried in May 2017. We extracted and considered for analysis 5728 samples (i.e. all the samples relative to tumor types we wanted to study with the exclusion of metastatic tumors), which were taken from patients with cancer and belonged to different datasets, as reported in Table S5. The samples included breast infiltrating ductal carcinoma (3169 samples), colorectal carcinoma (486 samples), liver carcinoma (232 samples), non-squamous and squamous NSCLC (813 and 279 samples, respectively), renal cell carcinoma (411 samples), ovarian serous carcinoma (225 samples) and ovarian clear cell carcinoma (27 samples), and prostate carcinoma (86 samples).
Three types of samples were used as controls (Tables S3 and S5): 28 samples of PBMCs from HDs, 18 samples of lymph nodes from HDs, and 20−142 samples of seven tissues (breast, colon, liver, lung, kidney, ovarian epithelium, and prostate) from HDs (HTs).
T cell infiltration, CTL activity and suppressive scores
The T cell infiltration score was calculated for each specimen based on the expression of genes in the tumor sample and the mean expression of the same genes in HTs. The genes considered were those reported by Bindea et al.Citation31 and Becht et al.Citation39 with the only exception of TRA and TRAC (Bindea signature) and MGC40069 (Becht signature) that are not included in the Affymetrix platform. The “gene expression in tumor sample”/“mean gene expression in HT” ratio, expressed as log(2), was calculated for each gene, and the mean ratio of all the genes was considered to represent the T cell infiltration score of the tumor specimen.
The CTL activity score was calculated for each patient specimen based on the expression of genes in the tumor sample and the mean expression of genes in PBMCs of HDs, both of which were normalized to expression of the CD8α chain. The following genes were considered to represent CTLsCitation31: APBA2, APOL3, CTSW, DUSP2, GNLY, GZMA, GZMA, KLRB1, KLRD1, KLRF1, KLRK1, NKG7, RORA, RUNX3, SIGIRR, and ZBTB16. The “normalized gene expression in the tumor sample”/“normalized mean gene expression in PBMCs” ratio, expressed as log(2), was calculated for each gene, and the mean ratio of all the genes was considered to represent the CTL activity score of the tumor specimen.
The suppressive score was calculated for each specimen based on the expression of genes in the tumor sample and the mean level of expression of genes in PBMCs of HDs, both of which were normalized to CD4 mRNA expression. The following genes were considered to represent Treg: FOXP3, IL10 and TGFB1. The “normalized gene expression in the tumor sample”/“normalized mean gene expression in PBMCs” ratio, expressed as log(2), was calculated for each gene, and the mean ratio of all the genes was considered to represent the suppressive score of the patient's tumor.
Expression of Treg markers in tumors vs. HT
To evaluate the expression of a Treg marker in tumors ( and Table S3), the mean expression of the Treg marker in the tumor was divided by the mean expression of the Treg marker in the corresponding HT or the mean expression of the Treg marker in all HTs (breast, colon, kidney, liver, lung, ovary and prostate). Markers with higher than two-fold expression in the tumor as compared to the corresponding HT (p<0.05) or higher than 1.5-fold expression in the tumor as compared to both the expression in the corresponding HT (p<0.05) and the mean expression in all HT samples (p<0.05) were selected for further analysis.
To determine the percentage of patients who express the Treg marker at high levels (, and Figures S2−7), we included the specimens in which the Treg marker expression level was higher than the mean+2SD value of the Treg marker expression in HT.
Correlation analysis
To evaluate whether tumor infiltration of CD4+ T cells was correlated with suppressive cytokine expression ( and , ), CD4 mRNA expression was correlated with mRNA expression of TGFB1 and IL10. To evaluate whether the expression of the Treg marker correlated with the expression of suppressive cytokines ( and Table S4), Treg marker mRNA expression was correlated with mRNA expression of TGFB1 and IL10. Correlation analyses were performed using the Spearman correlation analysis. For the Treg marker correlation score ( and Table S4), a score of +3 was assigned if p<0.05 and ρ>0.3, and a score of −3 was assigned if p<0.05 and ρ<−0.3. A score of +1 was assigned if p<0.05 and 0.1<ρ<0.3, and a score of −1 was assigned if p<0.05 and −0.1>ρ>−0.3.
ADCC index
The ADCC index was calculated for each selected marker for each tumor, as summarized in . This index was calculated by first assigning a score for (1) overexpression of the marker in the tumor as compared to the corresponding HT, (2) overexpression of the marker in the tumor as compared to all HTs, (3) overexpression of activating FcγR in the tumor as compared to lymph nodes from HDs, and (4) the correlation of the marker with the suppressive microenvironment of the specific cancer and of all cancers. For determining the ADCC index of a Treg marker, the first three scores were calculated by considering only the samples in which the marker was overexpressed (that is, the level of expression was higher than the mean±2SD expression of the corresponding HT). Therefore, the mean level of overexpression used for calculating the index was higher than that reported in , and the mean level of activating FcγRs may also differ from that reported in and that calculated for other Treg markers overexpressed by the same tumor. For the first three parameters, the scores were assigned on a range of 1 to 4 based on the expression level. In the case of the fourth parameter, an inverse correlation between a Treg marker and the suppressive microenvironment indicates that the expression of the surface molecule is higher in activated T cells than in Tregs. Therefore, only in this case, we decided to assign a score lower than 1, and so, the score for the fourth parameter ranges from 0.25 to 4. The ADCC index was obtained by the multiplying the score assigned to each of the parameters.
Based on the scoring system, the highest possible ADCC index for a Treg marker is 256. We considered markers with an ADCC index equal to or higher than 48 to be of interest. For example, the 48 score is obtained when the scores of the individual parameters (in the order in which they were described in the previous paragraph) are 4, 3, 1, and 4 respectively (as in the case of CD39, galectin-9 and TNFR2 in renal cell carcinoma). A score of 54 is obtained when the individual scores are 3, 3, 2 and 3 respectively (for example, 4-1BB and TIGIT in breast infiltrating ductal carcinoma); 3, 2, 4 and 2.25 respectively (for example, GITR in breast infiltrating ductal carcinoma); or 3, 4, 3 and 1.5 respectively (IL-21R in breast infiltrating ductal carcinoma).
Funding details
This work was supported by a grant given to Carlo Riccardi by Associazione Italiana per la Ricerca sul Cancro (AIRC, financing IG-14291) and Fondo di Ateneo per la ricerca di base 2015, fund granted to Giuseppe Nocentini
supp_data.zip
Download Zip (2.5 MB)Disclosure statement
Giuseppe Nocentini was an Amgen consultant in 2014 concerning GITR modulators in disease treatments and was supported by Ministero dell'Istruzione dell'Università e della Ricerca (MIUR) for a project titled “Modulation of T lymphocyte activity in autoimmune diseases” (2008SKTMME_003). Carlo Riccardi was supported by MIUR for a project titled “GITR-derived signals in T lymphocyte regulation” (2006052432_001) and a project titled “Identification of new pharmacological targets for treating allodynia and peripheral and central immune-inflammation.” He had also less relevant financing by Associazione Italiana per la Ricerca contro il Cancro (AIRC)(IG-10677 and IG-14291). The authors have no other relevant affiliation or financial involvement with any organization or entity with a financial interest in or financial conflict with the subject matter or material discussed in the manuscript. All this includes employment, consultancies, honoraria, stock ownership or options, expert testimony, grants or patents received or pending, and royalties.
References
- Liu C, Workman CJ, Vignali DA. Targeting regulatory T cells in tumors. FEBS J. 2016;238:2731–48.
- Whiteside TL. Induced regulatory T cells in inhibitory microenvironments created by cancer. Expert opinion on biological therapy. 2014;14:1411–25.
- Adeegbe DO, Nishikawa H. Natural and induced T regulatory cells in cancer. Front Immunol. 2013;4:190.
- Burocchi A, Colombo MP, Piconese S. Convergences and divergences of thymus- and peripherally derived regulatory T cells in cancer. Front Immunol. 2013;4:247.
- Fridman WH, Pages F, Sautes-Fridman C, Galon J. The immune contexture in human tumours: impact on clinical outcome. Nat Rev Cancer. 2012;12:298–306. doi:10.1038/nrc3245. PMID:22419253
- Clynes RA, Towers TL, Presta LG, Ravetch JV. Inhibitory Fc receptors modulate in vivo cytotoxicity against tumor targets. Nat Med. 2000;6:443–6. doi:10.1038/74704. PMID:10742152
- Bulliard Y, Jolicoeur R, Windman M, Rue SM, Ettenberg S, Knee DA, Wilson NS, Dranoff G, Brogdon JL. Activating Fc gamma receptors contribute to the antitumor activities of immunoregulatory receptor-targeting antibodies. J Exp Med. 2013;210:1685–93. doi:10.1084/jem.20130573. PMID:23897982
- Ko K, Yamazaki S, Nakamura K, Nishioka T, Hirota K, Yamaguchi T, Shimizu J, Nomura T, Chiba T, Sakaguchi S. Treatment of advanced tumors with agonistic anti-GITR mAb and its effects on tumor-infiltrating Foxp3+CD25+CD4+ regulatory T cells. J Exp Med. 2005;202:885–91. doi:10.1084/jem.20050940. PMID:16186187
- Simpson TR, Li F, Montalvo-Ortiz W, Sepulveda MA, Bergerhoff K, Arce F, Roddie C, Henry JY, Yagita H, Wolchok JD, et al. Fc-dependent depletion of tumor-infiltrating regulatory T cells co-defines the efficacy of anti-CTLA-4 therapy against melanoma. J Exp Med. 2013;210:1695–710. doi:10.1084/jem.20130579. PMID:23897981
- Bulliard Y, Jolicoeur R, Zhang J, Dranoff G, Wilson NS, Brogdon JL. OX40 engagement depletes intratumoral Tregs via activating FcgammaRs, leading to antitumor efficacy. Immunol Cell Biol. 2014;92:475–80. doi:10.1038/icb.2014.26. PMID:24732076
- Petrillo MG, Ronchetti S, Ricci E, Alunno A, Gerli R, Nocentini G, Riccardi C. GITR+ regulatory T cells in the treatment of autoimmune diseases. Autoimmun Rev. 2015;14:117–26. doi:10.1016/j.autrev.2014.10.011. PMID:25449679
- Seo N, Hayakawa S, Takigawa M, Tokura Y. Interleukin-10 expressed at early tumour sites induces subsequent generation of CD4(+) T-regulatory cells and systemic collapse of antitumour immunity. Immunology. 2001;103:449–57. doi:10.1046/j.1365-2567.2001.01279.x. PMID:11529935
- Morikawa H, Sakaguchi S. Genetic and epigenetic basis of Treg cell development and function: from a FoxP3-centered view to an epigenome-defined view of natural Treg cells. Immunol Rev. 2014;259:192–205. doi:10.1111/imr.12174. PMID:24712467
- Chen W, Jin W, Hardegen N, Lei KJ, Li L, Marinos N, McGrady G, Wahl SM. Conversion of peripheral CD4+CD25- naive T cells to CD4+CD25+ regulatory T cells by TGF-beta induction of transcription factor Foxp3. J Exp Med. 2003;198:1875–86. doi:10.1084/jem.20030152. PMID:14676299
- Bianchini R, Bistoni O, Alunno A, Petrillo MG, Ronchetti S, Sportoletti P, Bocci EB, Nocentini G, Gerli R, Riccardi C. CD4(+) CD25(low) GITR(+) cells: a novel human CD4(+) T-cell population with regulatory activity. Eur J Immunol. 2011;41:2269–78. doi:10.1002/eji.201040943. PMID:21557210
- Nocentini G, Cari L, Migliorati G, Riccardi C. The role of GITR single-positive cells in immune homeostasis. Immun Inflamm Dis. 2017;5:4–6. doi:10.1002/iid3.148. PMID:28250919
- Gagliani N, Magnani CF, Huber S, Gianolini ME, Pala M, Licona-Limon P, Guo B, Herbert DR, Bulfone A, Trentini F, et al. Coexpression of CD49b and LAG-3 identifies human and mouse T regulatory type 1 cells. Nat Med. 2013;19:739–46. doi:10.1038/nm.3179. PMID:23624599
- Roncarolo MG, Gregori S, Bacchetta R, Battaglia M. Tr1 cells and the counter-regulation of immunity: natural mechanisms and therapeutic applications. Curr Top Microbiol Immunol. 2014;380:39–68. PMID:25004813
- Campbell DJ, Koch MA. Phenotypical and functional specialization of FOXP3+ regulatory T cells. Nat Rev Immunol. 2011;11:119–30. doi:10.1038/nri2916. PMID:21267013
- Rezende RM, da Cunha AP, Kuhn C, Rubino S, M'Hamdi H, Gabriely G, Vandeventer T, Liu S, Cialic R, Pinheiro-Rosa N, et al. Identification and characterization of latency-associated peptide-expressing gammadelta T cells. Nat Commun. 2015;6:8726. doi:10.1038/ncomms9726. PMID:26644347
- Shevach EM, Thornton AM. tTregs, pTregs, and iTregs: similarities and differences. Immunol Rev. 2014;259:88–102. doi:10.1111/imr.12160. PMID:24712461
- Feuerer M, Hill JA, Mathis D, Benoist C. Foxp3+ regulatory T cells: differentiation, specification, subphenotypes. Nat Immunol. 2009;10:689–95. doi:10.1038/ni.1760. PMID:19536194
- Padovani CT, Bonin CM, Tozetti IA, Ferreira AM, Fernandes CE, Costa IP. Glucocorticoid-induced tumor necrosis factor receptor expression in patients with cervical human papillomavirus infection. Rev Soc Bras Med Trop. 2013;46:288–92. doi:10.1590/0037-8682-0029-2013. PMID:23856878
- Sacchetti C, Rapini N, Magrini A, Cirelli E, Bellucci S, Mattei M, Rosato N, Bottini N, Bottini M. In vivo targeting of intratumor regulatory T cells using PEG-modified single-walled carbon nanotubes. Bioconjug Chem. 2013;24:852–8. doi:10.1021/bc400070q. PMID:23682992
- Ronchetti S, Ricci E, Petrillo MG, Cari L, Migliorati G, Nocentini G, Riccardi C. Glucocorticoid-induced tumour necrosis factor receptor-related protein: a key marker of functional regulatory T cells. J Immunol Res. 2015;2015:171520. doi:10.1155/2015/171520. PMID:25961057
- Torrey H, Butterworth J, Mera T, Okubo Y, Wang L, Baum D, Defusco A, Plager S, Warden S, Huang D, et al. Targeting TNFR2 with antagonistic antibodies inhibits proliferation of ovarian cancer cells and tumor-associated Tregs. Sci Signal. 2017;10. doi:10.1126/scisignal.aaf8608. PMID:28096513
- Selby MJ, Engelhardt JJ, Quigley M, Henning KA, Chen T, Srinivasan M, Korman AJ. Anti-CTLA-4 antibodies of IgG2 a isotype enhance antitumor activity through reduction of intratumoral regulatory T cells. Cancer Immunol Res. 2013;1:32–42. doi:10.1158/2326-6066.CIR-13-0013. PMID:24777248
- Gabriely G, da Cunha AP, Rezende RM, Kenyon B, Madi A, Vandeventer T, Skillin N, Rubino S, Garo L, Mazzola MA, et al. Targeting latency-associated peptide promotes antitumor immunity. Science Immunology. 2017;2. doi:10.1126/sciimmunol.aaj1738. PMID:28763794
- De Simone M, Arrigoni A, Rossetti G, Gruarin P, Ranzani V, Politano C, Bonnal RJ, Provasi E, Sarnicola ML, Panzeri I, et al. Transcriptional landscape of human tissue lymphocytes unveils uniqueness of tumor-infiltrating T regulatory cells. Immunity. 2016;45:1135–47. doi:10.1016/j.immuni.2016.10.021. PMID:27851914
- Plitas G, Konopacki C, Wu K, Bos PD, Morrow M, Putintseva EV, Chudakov DM, Rudensky AY. Regulatory T Cells Exhibit Distinct Features in Human Breast Cancer. Immunity. 2016;45:1122–34. doi:10.1016/j.immuni.2016.10.032. PMID:27851913
- Bindea G, Mlecnik B, Tosolini M, Kirilovsky A, Waldner M, Obenauf AC, Angell H, Fredriksen T, Lafontaine L, Berger A, et al. Spatiotemporal dynamics of intratumoral immune cells reveal the immune landscape in human cancer. Immunity. 2013;39:782–95. doi:10.1016/j.immuni.2013.10.003. PMID:24138885
- Rooney MS, Shukla SA, Wu CJ, Getz G, Hacohen N. Molecular and genetic properties of tumors associated with local immune cytolytic activity. Cell. 2015;160:48–61. doi:10.1016/j.cell.2014.12.033. PMID:25594174
- Şenbabaoğlu Y, Winer AG, Gejman RS, Liu M, Luna A, Ostrovnaya I, Weinhold N, Lee W, Kaffenberger SD, Chen YB, et al. The landscape of T cell infiltration in human cancer and its association with antigen presenting gene expression. bioRxiv. 2015.
- Hruz T, Laule O, Szabo G, Wessendorp F, Bleuler S, Oertle L, Widmayer P, Gruissem W, Zimmermann P. Genevestigator v3: a reference expression database for the meta-analysis of transcriptomes. Adv Bioinformatics. 2008;2008:420747. doi:10.1155/2008/420747. PMID:19956698
- Mandal R, Senbabaoglu Y, Desrichard A, Havel JJ, Dalin MG, Riaz N, Lee KW, Ganly I, Hakimi AA, Chan TA, et al. The head and neck cancer immune landscape and its immunotherapeutic implications. JCI Insight. 2016;1:e89829. doi:10.1172/jci.insight.89829. PMID:27777979
- Charoentong P, Finotello F, Angelova M, Mayer C, Efremova M, Rieder D, Hackl H, Trajanoski Z. Pan-cancer immunogenomic analyses reveal genotype-immunophenotype relationships and predictors of response to checkpoint blockade. Cell Rep. 2017;18:248–62. doi:10.1016/j.celrep.2016.12.019. PMID:28052254
- Barrett T, Suzek TO, Troup DB, Wilhite SE, Ngau WC, Ledoux P, Rudnev D, Lash AE, Fujibuchi W, Edgar R. NCBI GEO: mining millions of expression profiles-database and tools. Nucleic Acids Res. 2005;33:D562–6. doi:10.1093/nar/gki022. PMID:15608262
- Kolesnikov N, Hastings E, Keays M, Melnichuk O, Tang YA, Williams E, Dylag M, Kurbatova N, Brandizi M, Burdett T, et al. ArrayExpress update-simplifying data submissions. Nucleic Acids Res. 2015;43:D1113–6. doi:10.1093/nar/gku1057. PMID:25361974
- Becht E, Giraldo NA, Lacroix L, Buttard B, Elarouci N, Petitprez F, Selves J, Laurent-Puig P, Sautes-Fridman C, Fridman WH, et al. Estimating the population abundance of tissue-infiltrating immune and stromal cell populations using gene expression. Genome Biol. 2016;17:218. doi:10.1186/s13059-016-1070-5. PMID:27765066
- Vesely MD, Kershaw MH, Schreiber RD, Smyth MJ. Natural innate and adaptive immunity to cancer. Annu Rev Immunol. 2011;29:235–71. doi:10.1146/annurev-immunol-031210-101324. PMID:21219185
- Diederichsen AC, Hjelmborg J, Christensen PB, Zeuthen J, Fenger C. Prognostic value of the CD4+/CD8+ ratio of tumour infiltrating lymphocytes in colorectal cancer and HLA-DR expression on tumour cells. Cancer Immunol Immunother. 2003;52:423–8. doi:10.1007/s00262-003-0388-5. PMID:12695859
- Curiel TJ. Tregs and rethinking cancer immunotherapy. J Clin Invest. 2007;117:1167–74. doi:10.1172/JCI31202. PMID:17476346
- Clancy T, Hovig E. Profiling networks of distinct immune-cells in tumors. BMC Bioinformatics. 2016;17:263. doi:10.1186/s12859-016-1141-3. PMID:27377892
- Woo EY, Chu CS, Goletz TJ, Schlienger K, Yeh H, Coukos G, Rubin SC, Kaiser LR, June CH. Regulatory CD4(+)CD25(+) T cells in tumors from patients with early-stage non-small cell lung cancer and late-stage ovarian cancer. Cancer Res. 2001;61:4766–72. PMID:11406550
- Liyanage UK, Moore TT, Joo HG, Tanaka Y, Herrmann V, Doherty G, Drebin JA, Strasberg SM, Eberlein TJ, Goedegebuure PS, et al. Prevalence of regulatory T cells is increased in peripheral blood and tumor microenvironment of patients with pancreas or breast adenocarcinoma. J Immunol. 2002;169:2756–61. doi:10.4049/jimmunol.169.5.2756. PMID:12193750
- Massague J. TGFbeta in Cancer. Cell. 2008;134:215–30. doi:10.1016/j.cell.2008.07.001. PMID:18662538
- Alshaker HA, Matalka KZ. IFN-gamma, IL-17 and TGF-beta involvement in shaping the tumor microenvironment: The significance of modulating such cytokines in treating malignant solid tumors. Cancer Cell Int. 2011;11:33. doi:10.1186/1475-2867-11-33. PMID:21943203
- Aruga A, Aruga E, Tanigawa K, Bishop DK, Sondak VK, Chang AE. Type 1 versus type 2 cytokine release by Vbeta T cell subpopulations determines in vivo antitumor reactivity: IL-10 mediates a suppressive role. J Immunol. 1997;159:664–73. PMID:9218581
- Wu C, Thalhamer T, Franca RF, Xiao S, Wang C, Hotta C, Zhu C, Hirashima M, Anderson AC, Kuchroo VK. Galectin-9-CD44 interaction enhances stability and function of adaptive regulatory T cells. Immunity. 2014;41:270–82. doi:10.1016/j.immuni.2014.06.011. PMID:25065622
- Rifkin DB. Latent transforming growth factor-beta (TGF-beta) binding proteins: orchestrators of TGF-beta availability. J Biol Chem. 2005;280:7409–12. doi:10.1074/jbc.R400029200. PMID:15611103
- Bu M, Shen Y, Seeger WL, An S, Qi R, Sanderson JA, Cai Y. Ovarian carcinoma-infiltrating regulatory T cells were more potent suppressors of CD8(+) T cell inflammation than their peripheral counterparts, a function dependent on TIM3 expression. Tumour Biol. 2016;37:3949–56. doi:10.1007/s13277-015-4237-x. PMID:26482613
- Madireddi S, Eun SY, Lee SW, Nemcovicova I, Mehta AK, Zajonc DM, Nishi N, Niki T, Hirashima M, Croft M. Galectin-9 controls the therapeutic activity of 4-1BB-targeting antibodies. J Exp Med. 2014;211:1433–48. doi:10.1084/jem.20132687. PMID:24958847
- Kang CW, Dutta A, Chang LY, Mahalingam J, Lin YC, Chiang JM, Hsu CY, Huang CT, Su WT, Chu YY, et al. Apoptosis of tumor infiltrating effector TIM-3+CD8+ T cells in colon cancer. Sci Rep. 2015;5:15659. doi:10.1038/srep15659. PMID:26493689
- Tran DQ, Andersson J, Wang R, Ramsey H, Unutmaz D, Shevach EM. GARP (LRRC32) is essential for the surface expression of latent TGF-beta on platelets and activated FOXP3+ regulatory T cells. Proc Natl Acad Sci U S A. 2009;106:13445–50. doi:10.1073/pnas.0901944106. PMID:19651619
- Knee DA, Hewes B, Brogdon JL. Rationale for anti-GITR cancer immunotherapy. Eur J Cancer. 2016;67:1–10. doi:10.1016/j.ejca.2016.06.028. PMID:27591414
- Marabelle A, Kohrt H, Sagiv-Barfi I, Ajami B, Axtell RC, Zhou G, Rajapaksa R, Green MR, Torchia J, Brody J, et al. Depleting tumor-specific Tregs at a single site eradicates disseminated tumors. J Clin Invest. 2013;123:2447–63. doi:10.1172/JCI64859. PMID:23728179
- Nocentini G, Cari L, Ronchetti S, Riccardi C. Modulation of tumor immunity: a patent evaluation of WO2015026684A1. Expert Opin Ther Pat. 2016;26:417–25. doi:10.1517/13543776.2016.1118061. PMID:26560753
- Cohen AD, Schaer DA, Liu C, Li Y, Hirschhorn-Cymmerman D, Kim SC, Diab A, Rizzuto G, Duan F, Perales MA, et al. Agonist anti-GITR monoclonal antibody induces melanoma tumor immunity in mice by altering regulatory T cell stability and intra-tumor accumulation. PLoS One. 2010;5:e10436. doi:10.1371/journal.pone.0010436. PMID:20454651
- Nocentini G, Cari L, Riccardi C. GITR: A Modulator of Regulatory and Effector T-cell Activity, Crucial in Tumour Rejection and Autoimmune Diseses. eLS. Chichester: John Wiley & Sons Ltd, 2017. doi:10.1002/9780470015902.a0026242.
- Kim YH, Shin SM, Choi BK, Oh HS, Kim CH, Lee SJ, Kim KH, Lee DG, Park SH, Kwon BS. Authentic GITR signaling fails to induce tumor regression unless Foxp3+ Regulatory T cells are depleted. J Immunol. 2015;195:4721–9. doi:10.4049/jimmunol.1403076. PMID:26423152
- Miska J, Rashidi A, Chang AL, Muroski ME, Han Y, Zhang L, Lesniak MS. Anti-GITR therapy promotes immunity against malignant glioma in a murine model. Cancer Immunol Immunother. 2016;65:1555–67. doi:10.1007/s00262-016-1912-8. PMID:27734112
- Leyland R, Watkins A, Mulgrew K, Holoweckyj N, Bamber L, Tigue NJ, Offer E, Andrews J, Yan L, Mullins S, et al. A novel murine GITR ligand fusion protein induces antitumor activity as a monotherapy, which is further enhanced in combination with an OX40 agonist. Clin Cancer Res. 2017;23(13):3416–3427. doi:10.1158/1078-0432.CCR-16-2000. PMID:28069723
- Joller N, Hafler JP, Brynedal B, Kassam N, Spoerl S, Levin SD, Sharpe AH, Kuchroo VK. Cutting edge: TIGIT has T cell-intrinsic inhibitory functions. J Immunol. 2011;186:1338–42. doi:10.4049/jimmunol.1003081. PMID:21199897
- Joller N, Lozano E, Burkett PR, Patel B, Xiao S, Zhu C, Xia J, Tan TG, Sefik E, Yajnik V, et al. Treg cells expressing the coinhibitory molecule TIGIT selectively inhibit proinflammatory Th1 and Th17 cell responses. Immunity. 2014;40:569–81. doi:10.1016/j.immuni.2014.02.012. PMID:24745333
- Kurtulus S, Sakuishi K, Ngiow SF, Joller N, Tan DJ, Teng MW, Smyth MJ, Kuchroo VK, Anderson AC. TIGIT predominantly regulates the immune response via regulatory T cells. J Clin Invest. 2015;125:4053–62. doi:10.1172/JCI81187. PMID:26413872
- Fuhrman CA, Yeh WI, Seay HR, Saikumar Lakshmi P, Chopra G, Zhang L, Perry DJ, McClymont SA, Yadav M, Lopez MC, et al. Divergent phenotypes of human regulatory T cells expressing the receptors TIGIT and CD226. J Immunol. 2015;195:145–55. doi:10.4049/jimmunol.1402381. PMID:25994968
- Chen ML, Yan BS, Bando Y, Kuchroo VK, Weiner HL. Latency-associated peptide identifies a novel CD4+CD25+ regulatory T cell subset with TGFbeta-mediated function and enhanced suppression of experimental autoimmune encephalomyelitis. J Immunol. 2008;180:7327–37. doi:10.4049/jimmunol.180.11.7327. PMID:18490732
- Scurr M, Ladell K, Besneux M, Christian A, Hockey T, Smart K, Bridgeman H, Hargest R, Phillips S, Davies M, et al. Highly prevalent colorectal cancer-infiltrating LAP(+) Foxp3(-) T cells exhibit more potent immunosuppressive activity than Foxp3(+) regulatory T cells. Mucosal Immunol. 2014;7:428–39. doi:10.1038/mi.2013.62. PMID:24064667
- Abd Al Samid M, Chaudhary B, Khaled YS, Ammori BJ, Elkord E. Combining FoxP3 and Helios with GARP/LAP markers can identify expanded Treg subsets in cancer patients. Oncotarget. 2016;7:14083–94. doi:10.18632/oncotarget.7334. PMID:26885615
- Schuler PJ, Schilling B, Harasymczuk M, Hoffmann TK, Johnson J, Lang S, Whiteside TL. Phenotypic and functional characteristics of CD4+ CD39+ FOXP3+ and CD4+ CD39+ FOXP3neg T-cell subsets in cancer patients. Eur J Immunol. 2012;42:1876–85. doi:10.1002/eji.201142347. PMID:22585562
- Whiteside TL. Regulatory T cell subsets in human cancer: are they regulating for or against tumor progression? Cancer Immunol Immunother. 2014;63:67–72. doi:10.1007/s00262-013-1490-y. PMID:24213679
- Collin M. Immune checkpoint inhibitors: a patent review (2010-2015). Expert Opin Ther Pat. 2016;26:555–64. doi:10.1080/13543776.2016.1176150. PMID:27054314
- Mahmud SA, Manlove LS, Schmitz HM, Xing Y, Wang Y, Owen DL, Schenkel JM, Boomer JS, Green JM, Yagita H, et al. Costimulation via the tumor-necrosis factor receptor superfamily couples TCR signal strength to the thymic differentiation of regulatory T cells. Nat Immunol. 2014;15:473–81. doi:10.1038/ni.2849. PMID:24633226
- Govindaraj C, Scalzo-Inguanti K, Madondo M, Hallo J, Flanagan K, Quinn M, Plebanski M. Impaired Th1 immunity in ovarian cancer patients is mediated by TNFR2+ Tregs within the tumor microenvironment. Clin Immunol. 2013;149:97–110. doi:10.1016/j.clim.2013.07.003. PMID:23948613
- Bonnefoy N, Bastid J, Alberici G, Bensussan A, Eliaou JF. CD39: A complementary target to immune checkpoints to counteract tumor-mediated immunosuppression. Oncoimmunology. 2015;4:e1003015. doi:10.1080/2162402X.2014.1003015. PMID:26155397
- Al-Lamki RS, Sadler TJ, Wang J, Reid MJ, Warren AY, Movassagh M, Lu W, Mills IG, Neal DE, Burge J, et al. Tumor necrosis factor receptor expression and signaling in renal cell carcinoma. Am J Pathol. 2010;177:943–54. doi:10.2353/ajpath.2010.091218. PMID:20566746
- Plitas G, Rudensky AY. Regulatory T Cells: Differentiation and Function. Cancer Immunol Res. 2016;4:721–5. doi:10.1158/2326-6066.CIR-16-0193. PMID:27590281
- Robins HS, Ericson NG, Guenthoer J, O'Briant KC, Tewari M, Drescher CW, Bielas JH. Digital genomic quantification of tumor-infiltrating lymphocytes. Sci Transl Med. 2013;5:214ra169. doi:10.1126/scitranslmed.3007247. PMID:24307693
- Gentleman RC, Carey VJ, Bates DM, Bolstad B, Dettling M, Dudoit S, Ellis B, Gautier L, Ge Y, Gentry J, et al. Bioconductor: open software development for computational biology and bioinformatics. Genome Biol. 2004;5:R80. doi:10.1186/gb-2004-5-10-r80. PMID:15461798