ABSTRACT
The expression of two metabolic enzymes, i.e., aldehyde dehydrogenase 7 family, member A1 (ALDH7A1) and lipase C, hepatic type (LIPC) by malignant cells, has been measured by immunohistochemical methods in non-small cell lung carcinoma (NSCLC) biopsies, and has been attributed negative and positive prognostic value, respectively. Here, we demonstrate that the protein levels of ALDH7A1 and LIPC correlate with the levels of the corresponding mRNAs. Bioinformatic analyses of gene expression data from 4921 cancer patients revealed that the expression of LIPC positively correlates with abundant tumor infiltration by myeloid and lymphoid cells in NSCLC, breast carcinoma, colorectal cancer and melanoma samples. In contrast, high levels of ALDH7A1 were associated with a paucity of immune effectors within the tumor bed. These data reinforce the notion that the metabolism of cancer cells has a major impact on immune and inflammatory processes in the tumor microenvironment, pointing to hitherto unsuspected intersections between oncometabolism and immunometabolism.
Introduction
The metabolism of malignant cells is rewired towards anabolic reactions that facilitate proliferation, resistance against a hostile milieu, and immune escape.Citation1–Citation5 Although most of the literature on oncometabolism has been elaborated based on in vitro studies, in which malignant cells are usually cultured as cell lines (i.e., in the absence of additional cellular and non-cellular components of the tumor microenvironment), it has become clear that tumors function like a micro-ecosystem, in which different cell types interact to favor tumor insurgence and progression.Citation6,Citation7 Moreover, the metabolism of immune cells is largely influenced by the tumor milieu, often skewing the differentiation of myeloid and lymphoid cell types towards a pro-inflammatory and immunosuppressive phenotype.Citation8–Citation10
Hence, oncometabolism and immunometabolism are connected by a bidirectional and intimate relationship that has profound implications for targeting cancer with metabolic interventions. As a standalone example, fasting, i.e., the temporary reduction of caloric intake, can limit tumor growth in vitro, as well as in vivo, in human cancers xenografted into immunodeficient mice.Citation11 The effect of such treatments depends to some extent on the intrinsic characteristic of tumor cells including their dependency on glycolysis or glutaminolysis.Citation12–Citation14 In addition, fasting can stimulate anticancer immune responses, and this effect is likely to stem from the activation of autophagy in cancer cells, favoring their immune recognition,Citation15,Citation16 as well as from direct stimulatory effects on immune cells.Citation17,Citation18 Similarly, inhibition of glycolysis has been suggested to directly affect tumor growth, yet also stimulates anticancer immune responses.Citation19
Driven by these considerations, we decided to evaluate the impact of specific metabolic enzymes on the immune infiltrate of non-small cell lung carcinoma (NSCLC) lesions, the prognosis of which is known to be dictated by anticancer immune responses.Citation20–Citation28 Based on an admittedly cell-autonomous perspective of NSCLC, we previously reported that the expression or activity enzymes involved in (1) vitamin B6 metabolism, such as pyridoxal kinase (PDXK) and aldehyde dehydrogenase 7 family, member A1, (ALDH7A1), (2) lipid synthesis, such as lipase C, hepatic type (LIPC), or (3) poly-ADP ribosylation, such as poly(ADP-ribose) polymerase 1 (PARP1) would affect the response of NSCLC cells to chemotherapy in vitro and in vivo, and affect patient prognosis.Citation29–Citation35 However, we have not yet explored the impact of these enzymes on anticancer immune responses. Here, we investigated the possibility that such enzymes might affect natural or therapy-driven anticancer immunosurveillance using a bioinformatic approach.
Results
Correlations of mRNA and protein expression levels for ALDH7A1 and LIPC
The notion that LIPC, PARP1 and PDXK expression affects the response of NSCLCs to therapy and the prognosis of NSCLC patients has initially been acquired by studying the CHEMORES cohort (www.chemores.ki.se), followed by validation in additional patient series. In particular, high LIPC expression (as measured by immunohistochemistry), low PARP1 activity (as measured by assessing the abundance of the PARP1 product poly(ADP-ribose) using immunochemistry) and high PDXK levels (as measured by immunohistochemistry) in NSCLC cells were identified as favorable prognostic biomarkers for NSCLC patients.Citation29–Citation33,Citation35 For ALDH7A1, we did observe an effect on cisplatin responses in vitro, yet we did not find a significant impact on progression-free survival or overall survival in the CHEMORES cohort,Citation29 contrasting with a more recent report demonstrating that high ALDH7A1 expression is associated with recurrence in patients with surgically resected NSCLC.Citation36 Since mRNA expression data (as determined by transcriptomic analyses) in biopsies from cancer patients are considerably more abundant than immunohistochemical data, we reasoned that a meta-analysis of the impact of such enzymes on immune infiltration should be based on mRNA rather than protein levels. To this aim, we determined the correlation between mRNA and protein levels for a number of gene products within the CHEMORES database. Positive correlations between mRNA levels (as determined by transcriptomic analysis) and protein levels (as determined by immunohistochemistry) were observed for ALDH17A, BCL2L1 (better known as BCL-XL)Citation29 and LIPC, but not for PARP1, PARP2, pyridoxal phosphatase (PDXP) and other proteins such as ALK receptor tyrosine kinase (ALK) and WD repeat and SOCS box containing 2 (WSB2) (–). We also determined the correlation between the densities of tumor-infiltrating CD8+ cytotoxic T lymphocytes (CTLs), FOXP3+ regulatory T (TREG) cells, and DC-LAMP+ dendritic cells (DCs) as determined by immunohistochemistry, and the abundance of different immune subsets measured with the Microenvironment Cell Populations-counter (MCP-counter) method, which is based on the expression levels of signature mRNAs.Citation37 Convincing correlations were observed for CD8 and CTLs, but neither for FOXP3 and TREG cells, nor for DC-LAMP and DCs (). Driven by these results, we decided to focus our study on ALDH7A1 and LIPC in relationship to the tumor immune infiltrate.
Figure 1. (a): Spearman’s correlation coefficients between mRNA levels (as determined by transcriptomic studies) and protein score (as determined by immunohistochemistry). Significant correlations are annotated: *p < 0.1, **p < 0.05, ***< 0.01, ****p < 0.0001). (b,c): Scatter plot representations of protein scores versus mRNA levels for significant correlations from panel A (ALDH7A1, BCL2L1 and LIPC). Significant correlations are annotated: *p < 0.1, **p < 0.05, ***< 0.01, ****p < 0.0001). ((e): Spearman’s correlation coefficients between immune infiltrate estimated by microarray expression deconvolution (MCP-counter method) and protein score estimated from immunofluorescence).
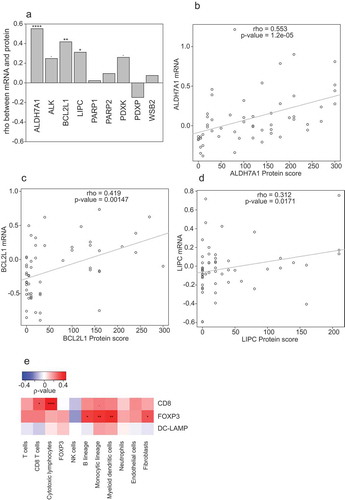
Associations between immunosurveillance and the expression of ALDH7A1, BCL2L1 and LIPC and across multiple cancers
In samples from the CHEMORES cohort, the protein levels of BCL2L1 and LIPC correlated with a prevalence of DCs as determined by the MCP counter, while ALDH7A1 levels exhibited a trend towards negative correlation with the abundance of NK cells (). These correlations were much more pronounced when only mRNA levels were analyzed. ALDH7A1 mRNA levels negatively correlated with those of multiple immune effectors (T cells, CTLs, TREG cells, B cells and monocytic cells) (). BCL2L1 mRNA levels correlated with the abundance of the FOXP3 mRNA, as well as with that of mRNAs from myeloid cell subsets (cells from the monocytic lineage, myeloid DCs, neutrophils). Similarly, the levels of LIPC mRNA positively correlated with those characterizing a number of distinct immune subset (T cells, CD8+ T cells, TREG cells, B cells, cells from the monocytic lineage, myeloid DCs and neutrophils).
Figure 2. (a,b): Spearman’s correlations coefficients between immune infiltrate estimates (MCP-counter method) and protein score (estimated from immunofluorescence, (a)) or mRNA levels (as determined by transcriptomic studies, (b)). Significant correlations are annotated: *p < 0.1, **p < 0.05, ***< 0.01, ****p < 0.0001.
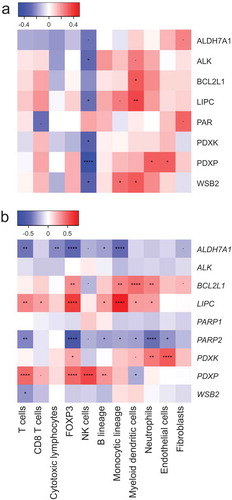
The positive correlation between LIPC levels and immune effectors could not be attributed to the preferential expression of BCL2L1 or LIPC by leukocytes. Indeed, BCL2L1 and LIPC were typically expressed by NSCLC cells, not by tumor-infiltrating immune cells (). Moreover, BCL2L1 and LIPC mRNA levels are not particularly high in leukemic cells or normal leukocytes subpopulations, as compared to a negative control mRNA (TYR, which codes for the melanoma-specific protein tyrosinase) and a positive control mRNA (CD45, encoding a common leukocyte antigen) (). Based on these considerations, it appears plausible that it is indeed the expression of LIPC and ALDH7A1 by malignant cells (as opposed to stromal elements) that exhibits a positive and negative correlation, respectively, with the immune infiltrate.
Figure 3. (a–d): Representative images for BCL2L1 (a,b) and LIPC (c,d) immunohistochemistry in NSCLC samples with high (a,c) or low (b,d) expression levels.
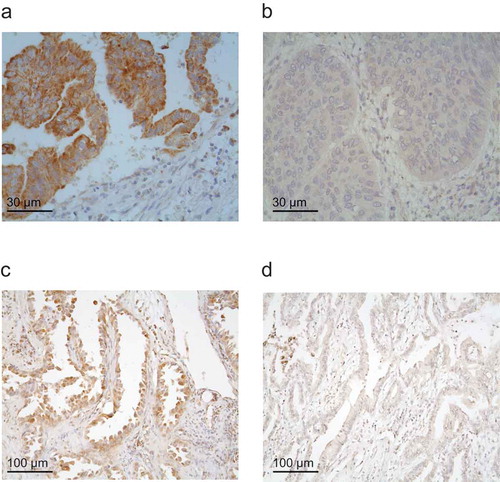
Figure 4. (a): mRNA abundance in Transcripts Per Million (TPM, as provided by The Human Protein Atlas website), for ALDH7A1, BCL2L1, CD45, LIPC and TYR in different cancer cell lines. TPM is artificially limited to an upper value of 100, for improved visualization. (b): mRNA normalized expression levels, in log10 scale, estimated by the DESQ2 package on RNA-seq data (as provided by the “Immunological Genome Project” website), for ALDH7A1, BCL2L1, CD45, LIPC and TYR in different immune cell types.
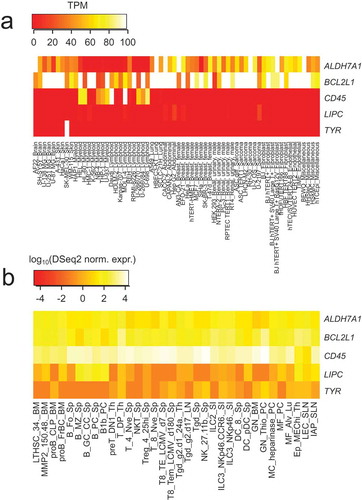
We next investigated the correlation between ALDH7A1, BCL2L1 and LIPC mRNA expression levels and the abundance of an array of immune cell subsets using the MCP-counter. To this aim, we generated a database encompassing gene expression data from 4921 patients with NSCLC, breast cancer, colorectal carcinoma or melanoma (). The correlations were calculated separately for each cohort of patients (5 cohorts with NSCLC, 6 with breast cancer, 3 with colorectal carcinoma, 5 with melanoma) and then for each cancer type together. ALDH7A1 mRNA levels were associated with a paucity of immune cells and fibroblasts, in particular amongst NSCLC and breast cancer patients (). Similarly, BCL2L1 mRNA levels tended to correlate with limited infiltration by various immune subsets, in particular CTLs and NK cells, in NSCLC, breast and colon cancer, but not in melanoma (). The most spectacular associations were found for LIPC. The levels of LIPC mRNA were associated with a poor immune infiltrate and even a scarcity of other non-malignant cell types such as endothelial cells and/or fibroblasts in all cancer types analyzed in this study (). This does not seem to be related to stem cells, as LIPC (nor ALDH7A1) did correlate with the stem cell marker PROM1 (CD133) (see sheet 1 of Supplemental Table S1). From these results, we conclude that LIPC and ALDH7A1 constitute two cancer-relevant metabolic enzymes whose expression correlates with the immune infiltrate in an opposite fashion. Notwithstanding the strong association between LIPC and ALDH7A1 mRNA levels and the immune infiltrate, there was little or no correlation between their abundance and patient survival across multiple cancer types (Suppl. Fig. S1-S4). This might reflect the possibility that ALDH7A1 and LIPC effects on cancer prognosis cancel each other because of their ambiguous impact on the immune infiltrate (which involves both positive and regulatory elements) and tumor cell-intrinsic characteristics. Moreover, neither LIPC nor ALDH7A1 correlated with the mRNA levels of PD-1, which codes for an important immune checkpoint molecule relevant to NSCLC, or LKB1, which codes for a metabolic regulator with relevance to NSCLC (sheet 2,3 of Supplemental Table S1).
Table 1. List of databases analyzed in this paper.
Figure 5. Spearman’s correlation coefficients between immune infiltrate estimates (MCP-counter method) and ALDH7A1 (A), BCL2L1 (B) and LIPC (C) mRNA levels from different public microarray datasets, of NSCLC, breast cancer, colorectal carcinoma and melanoma (). Significant correlations are annotated: *p < 0.1, **p < 0.05, ***< 0.01, ****p < 0.0001. For each pathology, a combined p value has been calculated as described in Materials and Methods.
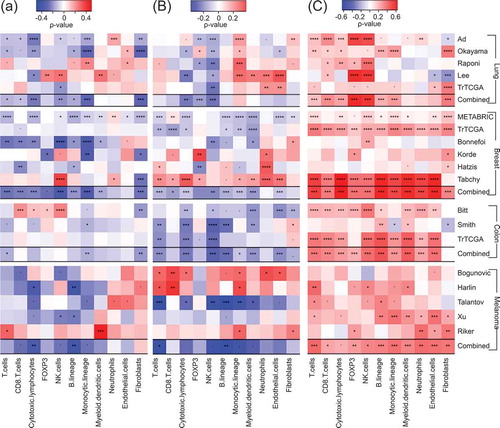
Discussion
ALDH7A1 is an aldehyde dehydrogenase with an important role in the detoxification of aldehydes generated by alcohol metabolism and lipid peroxidation. ALDH7A1 is best known for the fact that loss-of-function mutations of this gene cause pyridoxine-dependent epilepsy.Citation38 Moreover, knockdown of ALDH7A1 sensitizes NSCLC cells to the lethal effect of cisplatin.Citation29 Interesting, ALDH7A1 is overexpressed in cancer stem cellsCitation39 and is druggable.Citation40 However, to the best of our knowledge, the possibility to inhibit ALDH7A1 to obtain antineoplastic effects has not been investigated yet in preclinical models. In one study of ALDH7A1 expression in NSCLC patients subjected to surgery, low ALDH7A1 levels were accompanied by improved recurrence-free and overall survival.Citation36 Mechanistically, this may be linked to improved anticancer immunosurveillance, given that ALDH7A1high tumors are immunologically desert. However, how high levels of ALDH7A1 might condition the tumor microenvironment to cause a general paucity of immune effectors remains to be determined.
BCL2L1 is well known for its cell-autonomous apoptosis- and autophagy-inhibitory effects on cancer cells,Citation41,Citation42 and is well possible that these features indirectly impact on the tumor microenvironment. Although BCL2L1 was found to positively correlate with the myeloid immune infiltrate in our exploratory study (on the CHEMORES cohort), the correlations turned out to be mostly negative (in particular within the NK cell and T cell compartments) across several NSCLC, breast cancer and colon cancer cohorts. How BCL2L1 expression (mostly by neoplastic cells) can mediate such effects remains to be studied.
LIPC catalyzes the hydrolysis of triacylglycerides, and is mostly known for its participation in the release of free fatty acids from intermediate-density lipoproteins, thus generating low-density lipoproteins.Citation43 Genetic variants of LIPC affect dyslipidemia, for instance in the context of coronary artery disease.Citation44,Citation45 The function of LIPC in cancer is unknown. The only study dealing with the impact of LIPC on cancer prognosis demonstrated that high LIPC protein levels correlate with improved progression-free and overall survival in two distinct NSCLC patient cohortsCitation46 (and TCGA consortium). Initially, we identified LIPC as a factor involved in cisplatin-resistance in multiple different human NSCLC cell line.Citation31 This finding is apparently at odds with the fact that low LIPC expression is a poor prognostic marker, yet such stage I NSCLC patients might benefit from a platinum based chemotherapy as LIPClow patients receiving adjuvant chemotherapy exhibited an OS that was comparable to that of LIPChigh patients, irrespective of their status of therapy receiver.Citation29 The data reported here suggest yet another possibility, namely that elevated LIPC expression by malignant cells modulates the tumor microenvironment to improve anticancer immunosurveillance. However, it is not possible to establish causal relationships at this point. Moreover, the potential mechanisms through which LIPC would favor anticancer immune responses (rather than pro-carcinogenic inflammatory processes) remain entirely obscure.
The meta-analysis of different cancer types that we performed suggests that an elevated expression of LIPC or reduced ALDH7A1 levels similarly correlate with an increased presence of multiple distinct immune cell subtypes, some of which are involved in anticancer immunosurveillance (such as DCs and CTLs) while others are rather immunosuppressive (such as TREG cells and granulocytes).Citation24 In this context, it may be important to note that immunologically “cold” tumors usually lack both immunostimulatory and suppressive cell types altogether, while “hot” tumors contain a high frequency of different immune cells subtypes because of the intrinsic organization of the immune infiltrate that is particularly elaborate in breast cancer.Citation47–Citation53 Thus, LIPC and ALDH7A1 might have an influence on the overall density of the leukocyte infiltrate, while other factors may affect the proportion and functional interactions among positive and negative elements of local immune circuitries.Citation54
In sum, our work illustrates a potentially important crosstalk between oncometabolism and immunometabolism that warrants further mechanistic and preclinical evaluation.
Material and methods
Data from CHEMORES
We used 58 NSCLC biopsies from the CHEMORES cohort (http://www.chemores.ki.se), for which transcriptomic data were available and protein scores for ALDH7A1, ALK, BCL2L1, LIPC, poly(ADP)-ribose, PDXK, PDXP and WSB2 were estimated by immunohistochemistry. We were able to estimate the immune infiltration in 51 of 58 samples, using CD8, FOXP3 and DC-LAMP as immunohistochemical biomarkers.
Immunohistochemistry
Immunohistochemistry was performed as previously described,Citation29,Citation31,Citation35,Citation55 with primary antibodies specific for ALD7AH1 (rabbit monoclonal antibody IgG #AB53278, Abcam), ALK (mouse monoclonal IgG3 # M7195, Dako), BCL2L1 (mouse monoclonal IgG2a #AHO0222, Invitrogen), CD8 (monoclonal #NCL-L-CD8-295, Novocastra), DC-LAMP (rat monoclonal IgG2a #DDX0191, Dendritics), FOXP3 (mouse monoclonal IgG #ab450, Abcam), LIPC (mouse monoclonal IgG1, clone XHL1-1C, #sc-21741, Santa Cruz Biotechnology), PAR (mouse monoclonal antibody IgG #AM80, Calbiochem), PDXK (rabbit antiserum #AP7167A, Abgent), PDXP (rabbit antiserum #HPA001099, Atlas Antibodies) and WSB2 (rabbit antiserum #12124-2-AP, Proteintech, Chicago, USA). Staining intensity was quantified on a 0–3 scale, using as a reference the signal observed in fibroblasts or endothelial cells (score 2), while the percentage of positive tumor cells was scored on a 0–100% scale. These variables were integrated into a single score (ranging from 0–300) by calculating the product between staining intensity (0–3) and the percentage of tumor cells (0–100). Staining intensity of DC-LAMP was quantified as the total number of positive cells of 5 regions of interest. Staining intensity of CD8 and FOXP3 were reported as the median density (number of positive cells per µm2) of 5 regions of interest. Positive cells were automatically detected and counted with a custom written macro for ImageJ software (Rasband, W.S., ImageJ, U. S. National Institutes of Health, Bethesda, Maryland, USA, https://imagej.nih.gov/ij/, 1997–2018.).
Expression data in cell lines and immune cell subtypes
We considered two types of cell line expression: (1) expression in a general set of cell lines from The Protein AtlasCitation56 website (https://www.proteinatlas.org/), for which Transcript Per Million (TPM) data are available; (2) expression in immune cell from the Immunological Genome ProjectCitation57 (https://www.immgen.org/), for which normalized expression (based on DSeq2 packageCitation58) is available.
Public microarray data
We used several public microarray datasets for different pathologies: NSCLCCitation59–Citation61 (and TCGA consortium), breast carcinomaCitation46,Citation62–Citation65 (and TCGA consortium), colorectal carcinomaCitation66 (and TCGA consortium, http://www.intgen.org/), melanoma.Citation67–Citation71 We considered only large datasets (). R-package MCPcounter was used to estimate immune cell infiltrates from microarray data.Citation37 For correlation analysis, the Spearman’s correlation coefficient and test were employed. We used Fisher’s method on one-tail correlation p values (positive for LIPC, negative for ALDH7A1) in order to calculate “combined” p values for (for the “combined” correlation coefficient, we have chosen the one that has the smallest one-tail p value).
Survival analysis
For the analyses depicted in Supplemental Fig. S1, we used the datasets for which clinical data was available: METBARIC,Citation46 Breast TCGA (TCGA consortium) and Adenoconsortium.Citation59 We used Cox regression analysis of the R survival package.Citation72 For the analyses depicted in Supplemental Fig. S2, S3 and S4, we extracted data from the PROGgeneV2 database (dataset list is given in Supplemental Table S2).Citation73 We used the R-package metaCitation74 for the summary analysis and forest plots.
Disclosure of Potential Conflicts of Interest
No potential conflicts of interest were disclosed
Authors contribution
LG provides remunerated consulting to AstraZeneca, OmniSEQ and VL47, and is a member of the Scientific Advisory Committee of OmniSEQ. GK has been holding research contracts with Bayer Healthcare, Genentech, Glaxo Smyth Kline, Institut Mérieux, Lytix Pharma, Nucana, Oncolinx, PharmaMar, Sotio and Tioma and Vasculox. GK is on the Board of Directors of the Bristol Myers Squibb Foundation France. GK is a scientific co-founder of everImmune.
Supplemental Material
Download Zip (106.1 KB)Acknowledgments
The authors would like to thank Kariman Chaba and Philippe Girard (Gustave Roussy Cancer Campus; Villejuif, France) for their technical support. LG is supported by a startup grant from the Department of Radiation Oncology of Weill Cornell Medical College (New York, NY, USA), by industrial grants from Lytix (Oslo, Norway) and Phosplatin (New York, NY, USA), and by donations from Sotio (Prague, Czech Republic), Phosplatin (New York, NY, USA) and the Luke Heller TECPR2 Foundation (Boston, MA, USA). GK is supported by the Ligue contre le Cancer (équipe labelisée); Agence National de la Recherche (ANR) – Projets blancs; ANR under the frame of E-Rare-2, the ERA-Net for Research on Rare Diseases; Association pour la recherche sur le cancer (ARC); Cancéropôle Ile-de-France; Chancelerie des universités de Paris (Legs Poix), Fondation pour la Recherche Médicale (FRM); the European Commission (ArtForce); the European Research Council (ERC); Fondation Carrefour; Institut National du Cancer (INCa); Inserm (HTE); Institut Universitaire de France; LeDucq Foundation; the LabEx Immuno-Oncology; the RHU Torino Lumière, the Seerave Foundation; the SIRIC Stratified Oncology Cell DNA Repair and Tumor Immune Elimination (SOCRATE); the SIRIC Cancer Research and Personalized Medicine (CARPEM); and the Paris Alliance of Cancer Research Institutes (PACRI).
Supplementary Material
Supplemental data for this article can be accessed on the publisher’s website.
References
- Kroemer G, Pouyssegur J. Tumor cell metabolism: cancer’s Achilles’ heel. Cancer Cell. 2008;13:472–482. doi:10.1016/j.ccr.2008.05.005.
- Galluzzi L, Kepp O, Vander Heiden MG, Kroemer G. Metabolic targets for cancer therapy. Nat Rev Drug Discov. 2013;12:829–846. doi:10.1038/nrd4145.
- Hanahan D, Weinberg RA. Hallmarks of cancer: the next generation. Cell. 2011;144:646–674. doi:10.1016/j.cell.2011.02.013.
- Thompson CB, Palm W. Reexamining how cancer cells exploit the body’s metabolic resources. Cold Spring Harb Symp Quant Biol. 2016;81:67–72. doi:10.1101/sqb.2016.81.030734.
- Muir A, Danai LV, Vander Heiden MG. Microenvironmental regulation of cancer cell metabolism: implications for experimental design and translational studies. Dis Model Mech. 2018;11(8). doi:10.1242/dmm.035758.
- Yang L, Achreja A, Yeung TL, Mangala LS, Jiang D, Han C, Baddour J, Marini JC, Ni J, Nakahara R, et al. Targeting stromal glutamine synthetase in tumors disrupts tumor microenvironment-regulated cancer cell growth. Cell Metab. 2016;24:685–700. doi:10.1016/j.cmet.2016.10.011.
- Gouirand V, Guillaumond F, Vasseur S. Influence of the tumor microenvironment on cancer cells metabolic reprogramming. Front Oncol. 2018;8:117. doi:10.3389/fonc.2018.00117.
- Costa A, Kieffer Y, Scholer-Dahirel A, Pelon F, Bourachot B, Cardon M, Sirven P, Magagna I, Fuhrmann L, Bernard C, et al. Fibroblast heterogeneity and immunosuppressive environment in human breast cancer. Cancer Cell. 2018;33:463–79.e10. doi:10.1016/j.ccell.2018.01.011.
- Galluzzi L, Baehrecke EH, Ballabio A, Boya P, Bravo-San Pedro JM, Cecconi F, Choi AM, Chu CT, Codogno P, Colombo MI, et al. Molecular definitions of autophagy and related processes. Embo J. 2017;36:1811–1836. doi:10.15252/embj.201796697.
- Bantug GR, Galluzzi L, Kroemer G, Hess C. The spectrum of T cell metabolism in health and disease. Nat Rev Immunol. 2018;18:19–34. doi:10.1038/nri.2017.99.
- Lee C, Raffaghello L, Brandhorst S, Safdie FM, Bianchi G, Martin-Montalvo A, Pistoia V, Wei M, Hwang S, Merlino A, et al. Fasting cycles retard growth of tumors and sensitize a range of cancer cell types to chemotherapy. Sci Transl Med. 2012;4:124ra27. doi:10.1126/scitranslmed.3003293.
- Birsoy K, Possemato R, Lorbeer FK, Bayraktar EC, Thiru P, Yucel B, Wang T, Chen WW, Clish CB, Sabatini DM. Metabolic determinants of cancer cell sensitivity to glucose limitation and biguanides. Nature. 2014;508:108–112. doi:10.1038/nature13110.
- Green DR, Galluzzi L, Kroemer G. Cell biology. Metabolic control of cell death. Science. 2014;345:1250256.
- Obrist F, Michels J, Durand S, Chery A, Pol J, Levesque S, Joseph A, Astesana V, Pietrocola F, Wu GS, Castedo M, Kroemer G. Metabolic vulnerability of cisplatin-resistant cancers. EMBO J. 2018;37(14). doi:10.15252/embj.201798597.
- Ma Y, Galluzzi L, Zitvogel L, Kroemer G. Autophagy and cellular immune responses. Immunity. 2013;39:211–227. doi:10.1016/j.immuni.2013.07.017.
- Pietrocola F, Pol J, Vacchelli E, Rao S, Enot DP, Baracco EE, Levesque S, Castoldi F, Jacquelot N, Yamazaki T, et al. Caloric restriction mimetics enhance anticancer immunosurveillance. Cancer Cell. 2016;30:147–160. doi:10.1016/j.ccell.2016.05.016.
- Zitvogel L, Pietrocola F, Kroemer G. Nutrition, inflammation and cancer. Nat Immunol. 2017;18:843–850. doi:10.1038/ni.3754.
- Esteban-Martinez L, Sierra-Filardi E, McGreal RS, Salazar-Roa M, Marino G, Seco E, Durand S, Enot D, Graña O, Malumbres M, et al. Programmed mitophagy is essential for the glycolytic switch during cell differentiation. Embo J. 2017;36:1688–1706. doi:10.15252/embj.201695916.
- Beneteau M, Zunino B, Jacquin MA, Meynet O, Chiche J, Pradelli LA, Marchetti S, Cornille A, Carles M, Ricci J-E. Combination of glycolysis inhibition with chemotherapy results in an antitumor immune response. Proc Natl Acad Sci U S A. 2012;109:20071–20076. doi:10.1073/pnas.1206360109.
- Liu H, Zhang T, Ye J, Li H, Huang J, Li X, Wu B, Huang X, Hou J. Tumor-infiltrating lymphocytes predict response to chemotherapy in patients with advance non-small cell lung cancer. Cancer Immunol Immunother. 2012;61:1849–1856. doi:10.1007/s00262-012-1231-7.
- Remark R, Becker C, Gomez JE, Damotte D, Dieu-Nosjean MC, Sautes-Fridman C, Fridman W-H, Powell CA, Altorki NK, Merad M, et al. The non-small cell lung cancer immune contexture. A major determinant of tumor characteristics and patient outcome. Am J Respir Crit Care Med. 2015;191:377–390. doi:10.1164/rccm.201409-1671PP.
- Fucikova J, Becht E, Iribarren K, Goc J, Remark R, Damotte D, Alifano M, Devi P, Biton J, Germain C, et al. Calreticulin expression in human non-small cell lung cancers correlates with increased accumulation of antitumor immune cells and favorable prognosis. Cancer Res. 2016;76:1746–1756. doi:10.1158/0008-5472.CAN-15-1142.
- Stoll G, Iribarren K, Michels J, Leary A, Zitvogel L, Cremer I, Kroemer G. Calreticulin expression: interaction with the immune infiltrate and impact on survival in patients with ovarian and non-small cell lung cancer. Oncoimmunology. 2016;5:e1177692. doi:10.1080/2162402X.2016.1177692.
- Fridman WH, Zitvogel L, Sautes-Fridman C, Kroemer G. The immune contexture in cancer prognosis and treatment. Nat Rev Clin Oncol. 2017;14:717–734. doi:10.1038/nrclinonc.2017.101.
- Forde PM, Chaft JE, Smith KN, Anagnostou V, Cottrell TR, Hellmann MD, Zahurak M, Yang SC, Jones DR, Broderick S, et al. Neoadjuvant PD-1 blockade in resectable lung cancer. N Engl J Med. 2018;378:1976–1986. doi:10.1056/NEJMoa1716078.
- Socinski MA, Jotte RM, Cappuzzo F, Orlandi F, Stroyakovskiy D, Nogami N, Rodríguez-Abreu D, Moro-Sibilot D, Thomas CA, Barlesi F, et al. Atezolizumab for first-line treatment of metastatic nonsquamous NSCLC. N Engl J Med. 2018;378:2288–2301. doi:10.1056/NEJMoa1716948.
- Reck M, Rodriguez-Abreu D, Robinson AG, Hui R, Csoszi T, Fulop A, Gottfried M, Peled N, Tafreshi A, Cuffe S, et al. Pembrolizumab versus chemotherapy for PD-L1-positive non-Small-Cell Lung Cancer. N Engl J Med. 2016;375:1823–1833. doi:10.1056/NEJMoa1606774.
- Gandhi L, Rodriguez-Abreu D, Gadgeel S, Esteban E, Felip E, De Angelis F, Domine M, Clingan P, Hochmair MJ, Powell SF, et al. Pembrolizumab plus chemotherapy in metastatic non-small-cell lung cancer. N Engl J Med. 2018;378:2078–2092. doi:10.1056/NEJMoa1801005.
- Galluzzi L, Vitale I, Senovilla L, Olaussen KA, Pinna G, Eisenberg T, Goubar A, Martins I, Michels J, Kratassiouk G, et al. Prognostic impact of vitamin B6 metabolism in lung cancer. Cell Rep. 2012;2:257–269. doi:10.1016/j.celrep.2012.06.017.
- Galluzzi L, Vacchelli E, Michels J, Garcia P, Kepp O, Senovilla L, Vitale I, Kroemer G. Effects of vitamin B6 metabolism on oncogenesis, tumor progression and therapeutic responses. Oncogene. 2013;32:4995–5004. doi:10.1038/onc.2012.623.
- Galluzzi L, Goubar A, Olaussen KA, Vitale I, Senovilla L, Michels J, Robin A, Dorvault N, Besse B, Validire P, et al. Prognostic value of LIPC in non-small cell lung carcinoma. Cell Cycle. 2013;12:647–654. doi:10.4161/cc.23517.
- Michels J, Vitale I, Galluzzi L, Adam J, Olaussen KA, Kepp O, Senovilla L, Talhaoui I, Guegan J, Enot DP, et al. Cisplatin resistance associated with PARP hyperactivation. Cancer Res. 2013;73:2271–2280. doi:10.1158/0008-5472.CAN-12-3000.
- Michels J, Vitale I, Saparbaev M, Castedo M, Kroemer G. Predictive biomarkers for cancer therapy with PARP inhibitors. Oncogene. 2014;33:3894–3907. doi:10.1038/onc.2013.352.
- Michels J, Obrist F, Castedo M, Vitale I, Kroemer G. PARP and other prospective targets for poisoning cancer cell metabolism. Biochem Pharmacol. 2014;92:164–171. doi:10.1016/j.bcp.2014.08.026.
- Michels J, Adam J, Goubar A, Obrist F, Damotte D, Robin A, Alifano M, Vitale I, Olaussen KA, Girard P, et al. Negative prognostic value of high levels of intracellular poly(ADP-ribose) in non-small cell lung cancer. Ann Oncol. 2015;26:2470–2477. doi:10.1093/annonc/mdv393.
- Giacalone NJ, Den RB, Eisenberg R, Chen H, Olson SJ, Massion PP, Carbone DP, Lu B. ALDH7A1 expression is associated with recurrence in patients with surgically resected non-small-cell lung carcinoma. Future Oncol. 2013;9:737–745. doi:10.2217/fon.13.19.
- Becht E, Giraldo NA, Lacroix L, Buttard B, Elarouci N, Petitprez F, Selves J, Laurent-Puig P, Sautès-Fridman C, Fridman WH, et al. Estimating the population abundance of tissue-infiltrating immune and stromal cell populations using gene expression. Genome Biol. 2016;17:218. doi:10.1186/s13059-016-1070-5.
- van Karnebeek CD, Tiebout SA, Niermeijer J, Poll-The BT, Ghani A, Coughlin CR 2nd, Van Hove JLK, Richter JW, Christen HJ, Gallagher R, et al. Pyridoxine-dependent epilepsy: an expanding clinical spectrum. Pediatr Neurol. 2016;59:6–12. doi:10.1016/j.pediatrneurol.2015.12.013.
- Ma I, Allan AL. The role of human aldehyde dehydrogenase in normal and cancer stem cells. Stem Cell Rev. 2011;7:292–306. doi:10.1007/s12015-010-9208-4.
- Luo M, Gates KS, Henzl MT, Tanner JJ. Diethylaminobenzaldehyde is a covalent, irreversible inactivator of ALDH7A1. ACS Chem Biol. 2015;10:693–697. doi:10.1021/cb500977q.
- Green DR, Kroemer G. The pathophysiology of mitochondrial cell death. Science. 2004;305:626–629. doi:10.1126/science.1099320.
- Maiuri MC, Le Toumelin G, Criollo A, Rain JC, Gautier F, Juin P, Tasdemir E, Pierron G, Troulinaki K, Tavernarakis N, et al. Functional and physical interaction between Bcl-X(L) and a BH3-like domain in Beclin-1. Embo J. 2007;26:2527–2539. doi:10.1038/sj.emboj.7601689.
- Chatterjee C, Sparks DL. Hepatic lipase, high density lipoproteins, and hypertriglyceridemia. Am J Pathol. 2011;178:1429–1433. doi:10.1016/j.ajpath.2010.12.050.
- Surakka I, Horikoshi M, Magi R, Sarin AP, Mahajan A, Lagou V, Marullo L, Ferreira T, Miraglio B, Timonen S, et al. The impact of low-frequency and rare variants on lipid levels. Nat Genet. 2015;47:589–597. doi:10.1038/ng.3300.
- Helgadottir A, Gretarsdottir S, Thorleifsson G, Hjartarson E, Sigurdsson A, Magnusdottir A, Jonasdottir A, Kristjansson H, Sulem P, Oddsson A, et al. Variants with large effects on blood lipids and the role of cholesterol and triglycerides in coronary disease. Nat Genet. 2016;48:634–639. doi:10.1038/ng.3561.
- Curtis C, Shah SP, Chin SF, Turashvili G, Rueda OM, Dunning MJ, Speed D, Lynch AG, Samarajiwa S, Yuan Y, et al. The genomic and transcriptomic architecture of 2,000 breast tumours reveals novel subgroups. Nature. 2012;486:346–352. doi:10.1038/nature10983.
- Stoll G, Bindea G, Mlecnik B, Galon J, Zitvogel L, Kroemer G. Meta-analysis of organ-specific differences in the structure of the immune infiltrate in major malignancies. Oncotarget. 2015;6:11894–11909. doi:10.18632/oncotarget.4180.
- Weinstein AM, Storkus WJ. Therapeutic lymphoid organogenesis in the tumor microenvironment. Adv Cancer Res. 2015;128:197–233. doi:10.1016/bs.acr.2015.04.003.
- Stoll G, Zitvogel L, Kroemer G. Immune infiltrate in cancer. Aging. 2015;7:358–359. doi:10.18632/aging.100770.
- Kroemer G, Senovilla L, Galluzzi L, Andre F, Zitvogel L. Natural and therapy-induced immunosurveillance in breast cancer. Nat Med. 2015;21:1128–1138. doi:10.1038/nm.3944.
- Stoll G, Zitvogel L, Kroemer G. Differences in the composition of the immune infiltrate in breast cancer, colorectal carcinoma, melanoma and non-small cell lung cancer: A microarray-based meta-analysis. Oncoimmunology. 2016;5:e1067746. doi:10.1080/2162402X.2015.1067746.
- Semeraro M, Adam J, Stoll G, Louvet E, Chaba K, Poirier-Colame V, Sauvat A, Senovilla L, Vacchelli E, Bloy N, et al. The ratio of CD8+/FOXP3 T lymphocytes infiltrating breast tissues predicts the relapse of ductal carcinoma in situ. Oncoimmunology. 2016;5:e1218106. doi:10.1080/2162402X.2016.1218106.
- Solinas C, Garaud S, De Silva P, Boisson A, Van Den Eynden G, de Wind A, Risso P, Rodrigues Vitória J, Richard F, Migliori E, et al. Immune checkpoint molecules on tumor-infiltrating lymphocytes and their association with tertiary lymphoid structures in human breast cancer. Front Immunol. 2017;8:1412. doi:10.3389/fimmu.2017.01412.
- Galluzzi L, Chan TA, Kroemer G, Wolchok JD, Lopez-Soto A. The hallmarks of successful anticancer immunotherapy. Sci Transl Med. 2018;10(459). doi:10.1126/scitranslmed.aat7807.
- Semeraro M, Adam J, Stoll G, Louvet E, Chaba K, Poirier-Colame V, Sauvat A, Senovilla L, Vacchelli E, Bloy N, et al. The ratio of CD8(+)/FOXP3 T lymphocytes infiltrating breast tissues predicts the relapse of ductal carcinoma in situ. Oncoimmunology. 2016;5:e1218106. doi:10.1080/2162402X.2016.1218106.
- Uhlen M, Fagerberg L, Hallstrom BM, Lindskog C, Oksvold P, Mardinoglu A, Sivertsson A, Kampf C, Sjostedt E, Asplund A, et al. Proteomics. Tissue-based map of the human proteome. Science. 2015;347:1260419. doi:10.1126/science.1260419.
- Shay T, Kang J. Immunological genome project and systems immunology. Trends Immunol. 2013;34:602–609. doi:10.1016/j.it.2013.03.004.
- Love MI, Huber W, Anders S. Moderated estimation of fold change and dispersion for RNA-seq data with DESeq2. Genome Biol. 2014;15:550. doi:10.1186/s13059-014-0550-8.
- Shedden K, Taylor JM, Enkemann SA, Tsao MS, Yeatman TJ, Gerald WL, Gerald WL, Eschrich S, Jurisica I, Giordano TJ, et al. Gene expression-based survival prediction in lung adenocarcinoma: a multi-site, blinded validation study. Nat Med. 2008;14:822–827. doi:10.1038/nm.1790.
- Lee ES, Son DS, Kim SH, Lee J, Jo J, Han J, Kim H, Lee HJ, Choi HY, Jung Y, et al. Prediction of recurrence-free survival in postoperative non-small cell lung cancer patients by using an integrated model of clinical information and gene expression. Clin Cancer Res. 2008;14:7397–7404. doi:10.1158/1078-0432.CCR-07-4937.
- Okayama H, Kohno T, Ishii Y, Shimada Y, Shiraishi K, Iwakawa R, Furuta K, Tsuta K, Shibata T, Yamamoto S, et al. Identification of genes upregulated in ALK-positive and EGFR/KRAS/ALK-negative lung adenocarcinomas. Cancer Res. 2012;72:100–111. doi:10.1158/0008-5472.CAN-11-1403.
- Bonnefoi H, Piccart M, Bogaerts J, Mauriac L, Fumoleau P, Brain E, Petit T, Rouanet P, Jassem J, Blot E, et al. TP53 status for prediction of sensitivity to taxane versus non-taxane neoadjuvant chemotherapy in breast cancer (EORTC 10994/BIG 1-00): a randomised phase 3 trial. Lancet Oncol. 2011;12:527–539. doi:10.1016/S1470-2045(11)70094-8.
- Korde LA, Lusa L, McShane L, Lebowitz PF, Lukes L, Camphausen K, Parker JS, Swain SM, Hunter K, Zujewski JA. Gene expression pathway analysis to predict response to neoadjuvant docetaxel and capecitabine for breast cancer. Breast Cancer Res Treat. 2010;119:685–699. doi:10.1007/s10549-009-0651-3.
- Hatzis C, Pusztai L, Valero V, Booser DJ, Esserman L, Lluch A, Vidaurre T, Holmes F, Souchon E, Wang H, et al. A genomic predictor of response and survival following taxane-anthracycline chemotherapy for invasive breast cancer. JAMA. 2011;305:1873–1881. doi:10.1001/jama.2011.593.
- Tabchy A, Valero V, Vidaurre T, Lluch A, Gomez H, Martin M, Qi Y, Barajas-Figueroa LJ, Souchon E, Coutant C, et al. Evaluation of a 30-gene paclitaxel, fluorouracil, doxorubicin, and cyclophosphamide chemotherapy response predictor in a multicenter randomized trial in breast cancer. Clin Cancer Res. 2010;16:5351–5361. doi:10.1158/1078-0432.CCR-10-1265.
- Smith JJ, Deane NG, Wu F, Merchant NB, Zhang B, Jiang A, Lu P, Johnson JC, Schmidt C, Bailey CE, et al. Experimentally derived metastasis gene expression profile predicts recurrence and death in patients with colon cancer. Gastroenterology. 2010;138:958–968. doi:10.1053/j.gastro.2009.11.005.
- Bogunovic D, O’Neill DW, Belitskaya-Levy I, Vacic V, Yu YL, Adams S, Darvishian F, Berman R, Shapiro R, Pavlick AC, et al. Immune profile and mitotic index of metastatic melanoma lesions enhance clinical staging in predicting patient survival. Proc Natl Acad Sci U S A. 2009;106:20429–20434. doi:10.1073/pnas.0905139106.
- Harlin H, Meng Y, Peterson AC, Zha Y, Tretiakova M, Slingluff C, McKee M, Gajewski TF. Chemokine expression in melanoma metastases associated with CD8+ T-cell recruitment. Cancer Res. 2009;69:3077–3085. doi:10.1158/0008-5472.CAN-08-2281.
- Talantov D, Mazumder A, Yu JX, Briggs T, Jiang Y, Backus J, Atkins D, Wang Y. Novel genes associated with malignant melanoma but not benign melanocytic lesions. Clin Cancer Res. 2005;11:7234–7242. doi:10.1158/1078-0432.CCR-05-0683.
- Xu L, Shen SS, Hoshida Y, Subramanian A, Ross K, Brunet JP, Wagner SN, Ramaswamy S, Mesirov JP, Hynes RO. Gene expression changes in an animal melanoma model correlate with aggressiveness of human melanoma metastases. Mol Cancer Res. 2008;6:760–769. doi:10.1158/1541-7786.MCR-07-0344.
- Riker AI, Enkemann SA, Fodstad O, Liu S, Ren S, Morris C, Xi Y, Howell P, Metge B, Samant RS, et al. The gene expression profiles of primary and metastatic melanoma yields a transition point of tumor progression and metastasis. BMC Med Genomics. 2008;1:13. doi:10.1186/1755-8794-1-13.
- Therneau TM A package for survival analysis in S. 2015. https://CRAN.R-project.org/package=survival.
- Goswami CP, Nakshatri H. PROGgeneV2: enhancements on the existing database. BMC Cancer. 2014;14:970. doi:10.1186/1471-2407-14-970.
- Schwarzer G. meta: {A}n {R} package for meta-analysis. R News. 2007;7:40–45.