ABSTRACT
Although immunotherapies have achieved remarkable salutary effects among subgroups of advanced cancers, most patients do not respond. We comprehensively evaluated biomarkers associated with the “cancer-immunity cycle” in the pan-cancer setting in order to understand the immune landscape of metastatic malignancies as well as anti-PD-1/PD-L1 inhibitor resistance mechanisms. Interrogation of 51 markers of the cancer-immunity cycle was performed in 101 patients with diverse malignancies using a clinical-grade RNA sequencing assay. Overall, the immune phenotypes demonstrated overexpression of multiple checkpoints including VISTA (15.8% of 101 patients), PD-L2 (10.9%), TIM3 (9.9%), LAG3 (8.9%), PD-L1 (6.9%) and CTLA4 (3.0%). Additionally, aberrant expression of macrophage-associated markers (e.g. CD68 and CSF1R; 11-23%), metabolic immune escape markers (e.g. ADORA2A and IDO1; 9-16%) and T-cell priming markers (e.g. CD40, GITR, ICOS and OX40; 4-31%) were observed. Most tumors (87.1%, 88/101) expressed distinct immune portfolios, with a median of six theoretically actionable biomarkers (pharmacologically tractable by Food and Drug Administration approved agents [on- or off-label] or with agents in clinical development). Overexpression of TIM-3, VISTA and CD68 were significantly associated with shorter progression-free survival (PFS) after anti-PD-1/PD-L1-based therapies (among 39 treated patients) (all P < .01). In conclusion, cancer-immunity cycle biomarker evaluation was feasible in diverse solid tumors. High expression of alternative checkpoints TIM-3 and VISTA and of the macrophage-associated markers CD68 were associated with significantly worse PFS after anti-PD-1/PD-L1-based therapies. Most patients had distinct and complex immune expression profiles suggesting the need for customized combinations of immunotherapy.
KEYWORDS:
Introduction
Immunotherapies, including vaccine therapy, chimeric antigen receptor (CAR) T-cell therapy and immune checkpoint inhibitors (ICIs) have evolved rapidly in recent years as a consequence of our accumulating understanding of tumor immunityCitation1 Among these, ICIs, such as monoclonal antibodies against cytotoxic T lymphocyte antigen-4 (CTLA-4) and programmed death-1 (PD-1) or programmed death ligand-1 (PD-L1), are some of the most successful examples of drug development and, consequently, immune therapy has become standard of care in diverse malignancies.Citation2 Indeed, ICIs have been reported to exhibit anti-tumor effects against a wide range of tumor types, including both solid and hematological malignancies.Citation3–Citation7
However, ICIs are effective in only a portion of patients (response rates ranging from <5% to >40%, depending on the cancer type). Further, many patients exhibit serious side effects and some may also show accelerated disease progression (known as hyperprogression).Citation8 Thus, there is an unmet need to understand the biomarkers associated with anti-tumor immune effects in individual patients. To date, several markers have been identified as predictors of response to ICIs including, but not limited to; (i) high PD-L1 expression or amplification,Citation9,Citation10 (ii) microsatellite instability)- high (MSI-H)Citation11 or deficiency in mismatch repair genes,Citation12 (iv) presence of tumor infiltrating lymphocytes (TILs)Citation13 and (v) high tumor mutation burden (TMB).Citation14 However, even in the presence of the aforementioned biomarkers, not all patients demonstrate response to ICIs.Citation15–Citation17
To further enhance the anti-cancer effect with ICIs, deeper interrogation of the immune tumor milieu is warranted. This issue is also relevant to the many clinical trials combining ICIs with other therapeutic modalities, such as chemotherapy, molecularly targeted drugs, radiotherapy, or with various immune modulators.Citation18–Citation20 Although there are signals of improved anti-tumor effect with some combination approaches,Citation21–Citation24 in large, preliminary results suggest that optimization of combinations is still needed. For example, epacadostat, an IDO1 inhibitor, showed promising results in a phase II trial; however, a pivotal phase III trial in advanced melanoma failed to demonstrate superiority with the addition of epacadostat to pembrolizumab when compared to pembrolizumab alone (ECHO-301/Keynote-252 study).Citation22 Moreover, the clinical efficacy of combinations of ipilimumab plus nivolumab when compared to nivolumab alone in patients advanced melanoma appears similar (overall survival [OS] rate at 3 years of 58% in the ipilimumab-plus-nivolumab group vs. 52% in the nivolumab group).Citation24
In the field of genomics, there are several remarkable successes with the use of targeted drugs. The most striking response rates occur when patients with specific genomic alterations are selected to receive cognate antagonists: imatinib for the treatment of chronic myelogenous leukemia harboring BCR-ABL fusion gene,Citation25 anti-EGFR therapies (e.g. gefitinib, erlotinib, osimertinib) for epidermal growth factor receptor (EGFR)-mutant lung cancer,Citation26 and crizotinib for anaplastic lymphoma kinase (ALK)-mutant lung cancer.Citation27 Hence the key to success is choosing the right patient for the right drug based on matched biomarkers.Citation28–Citation32 These results have been confirmed by meta-analyses of large datasets, including meta-analyses of 346 published phase I trials showed that a personalized (biomarker matched) strategy using genomic biomarkers was associated with significantly higher response rates compared with a non-matched approach (response rate, 42% vs. 5%; p < .001)Citation30
Although anti-CTLA-4 inhibitors and anti-PD-1 or anti-PD-L1 inhibitors already have remarkable effects in a subgroup of patients, many patients still do not respond to these drugs. To enhance patient selection, especially for combination therapies, comprehensive understanding of the cancer immunogram landscape is imperative.Citation33 Each step in the cancer-immunity cycle is regulated by various stimulatory and inhibitory factors that can potentially be targets of interest to optimize the anti-cancer immune effect (Supplemental Table 1). It is conceivable that, as is the case with matching therapy to genomic targets, matching immune modulators to the individual patient immune landscape may optimize salutary effects. Therefore, in order to facilitate rational clinical trial development, and better understand response and resistance, we comprehensively analyzed pan-cancer immunity markers.
Results
Patient characteristics
Among 101 patients with diverse malignancies, the median age was 57.1 years (range: 24.6–87.1 years), and 61.4% (62/101) were women. The most common diagnosis was gastrointestinal cancers (non-colorectal, 30.7% [31/101] and colorectal cancers, 20.8% [21/101]), followed by gynecologic cancers (15.8% [16/101]) (). Among 101 patients, 39 patients received anti-PD-1/PD-L1 based regimens.
Table 1. Patient characteristics (N = 101)
A variety of patterns of RNA expression level for immune markers were seen in patients with cancer (N = 101)
A variety of immune response markers were evaluated (N = 51 markers) and RNA expression was ranked on a scale of 1 to 100 and stratified into “Very high” (95–100), “High” (85–94), “Moderate” (50–84), “Low” (20–49), and “Very Low” (0–19) based on an internal reference populationCitation34 Among checkpoint markers, high or very high RNA expression were most commonly observed in VISTA (15.8% [16/101 of patients]) followed by PD-L2 (10.9% [11/101]), TIM3 (9.9% [10/101]), LAG3 (8.9% [9/101]) and PD-L1 (6.9% [7/101]). High/very high RNA expression in macrophage-associated markers (some of which are myeloid suppressors) were most commonly seen in CSF1R (22.8% [23/101]), CCR2 (13.9% [14/101]) and CD163 (12.9% [13/101]). Among metabolic immune escape markers, RNA was highly expressed in CD39 (15.8% [16/101]), IDO1 (12.9% [13/101]) and ADORA2A (8.9% [9/101]). T-cell primed markers were most commonly expressed among OX40 ligand (30.7% [31/101]) followed by ICOS ligand (25.7% [26/101]), CD86 (18.8% [19/101]), CD80 (12.9% [13/101]), GZMB (12.9% [13/101]) and GITR (11.9% [12/101]). Several pro-inflammatory response markers were highly expressed in IL1B (34.7% [35/101]), DDX58 (32.7% [33/101]) and MX1 (24.8% [25/101]). Lastly, among tumor infiltrating lymphocyte markers, high/very high RNA expression were seen among CD4 (18.8% [19/101]), FOXP3 (17.8% [18/101]) and KLRD1 (11.9% [12/101]) (, Supplemental Table 2 and Supplemental Figure 1).
Most patients had potentially actionable cancer-immunity markers (N = 101)
Overall, 52.5% (N = 53) of the 101 patients had at least one cancer-immunity cycle associated biomarker which was theoretically actionable by an FDA-approved agent (on- or off-label). Additionally, 46.5% (N = 47) had at least one cancer-immunity biomarker which was potentially targetable with an agent that is in clinical investigation ( and Supplemental Table 1). Altogether, 99.0% (N = 100) of the patients had at least one theoretically actionable biomarker either with agents that are approved by the Food and Drug Administration (FDA) (on- or off-label) or with agents that are in clinical trials. The median number of actionable biomarkers that were associated with the cancer-immunity cycle was 6 (per patient) (range, 0–16).
Most patients had distinct expression patterns of cancer-immunity markers (N = 101)
Among the 101 patients, most patients (87.1% [88/101]) had different expression patterns of cancer-immunity markers (). Four patients had high/very high RNA expression only in IL1B; three had high/very high RNA expression in both DDX58 and MX1; and six did not have highly expressed RNA in any of the cancer-immunity markers evaluated ().
Patients with colorectal cancer had higher expression of IL-1B (inflammatory cytokine) than non-colorectal cancer
We examined the expression patterns of cancer-immunity markers between colorectal cancer (N = 21) and non-colorectal cancers (N = 80) and found that CCR2 (receptor for the CCL2; mediates signaling for chemotaxis) and GATA3 (highly expressed in helper T cells) were highly expressed among patients with non-colorectal cancers (high expression of CCR2 and GATA3 in colorectal vs. non-colorectal: 0% [0/21] vs. 17.5% [14/80], P = .038) (Supplemental Table 3). In contrast, high expression of IL1B was more commonly seen among patients with colorectal cancer (57.1% [12/21] vs. 28.7% [23/80], P = .021) (Supplemental Table 3). Moreover, patients with gynecological cancers appear to have numerically higher frequencies of certain cancer-immunity markers when compared to other disease types including high expression of LAG3 (25%), GZMB (25%), IDO1 (31.3%), IL10 (43.8%) and OX40 ligand (62.5%) (though this did not reach statistical significance) (Supplemental Table 3).
High RNA expression in checkpoint markers TIM3 and VISTA as well as macrophage-associated markers CD68 as potential resistant markers for anti-PD-1/PD-L1 based regimens (N = 39)
Among 101 patients analyzed in this study, 39 received anti-PD-1/PD-L1 based regimens and were evaluated for progression-free survival (PFS) (). Among diverse cancer-immunity markers, high expression of TIM3 (P = .007), VISTA (P = .001), and CD68 (P = .009) were significantly associated with shorter PFS (median 1.7 versus 5.9 months in each case) ( and Supplemental Table 4) and were retained after the Bonferroni correction for multiple comparisons.
Table 2. Characteristics of patients who were treated with anti-PD-1/PD-L1 based immunotherapy (N = 39)
Table 3. Association between immune markers and progression-free survival among patients who received anti-PD-1/PD-L1 based regimens (N = 39)
Among 39 patients who received anti-PD-1/PD-L1 based immunotherapy, 32 patients were evaluable for response. Clinical benefit was defined as achieving stable disease (SD) ≥ 6 months, partial response (PR) or complete response (CR). High expression of TIM3 and VISTA showed a trend toward lower rates of SD ≥6 months/PR/CR (0 versus 52% in each case; p = .052) as did CD68 (albeit weaker; 0 versus 50%; p = .113) (). The small number of patients evaluable for response may have limited the analysis.
Table 4. Correlation between immune markers and clinical benefit (SD≥6m/PR/CR) from anti-PD-1/PD-L1 based immunotherapy (N = 32)
Discussion
Although immunotherapies, especially checkpoint inhibitors, have achieved some remarkable salutary anti-cancer effects among patients with numerous advanced malignancies, only a subgroup of patients respond to immunotherapies. Several biomarkers have been demonstrated to correlate with responsiveness.Citation8–Citation12,Citation14 However, as new immunotherapy agents have entered the clinical arena, a broader and more robust spectrum of predictive markers is needed, especially for combination treatments.Citation20,Citation35 Herein, we comprehensively evaluated biomarkers associated with the “cancer-immunity cycle” among patients with diverse advanced solid tumors.
Patients appeared to have a wide variety of immune markers ( and Supplemental Table 2). Along with the well-known checkpoint marker PD-L1, some tumors had high expression of other checkpoint markers (such as CTLA4 [3.0% of 101 patients had high expression], TIM3 [9.9%] and VISTA [15.8%]) as well as other factors involved in the cancer-immunity cycle including high expression of macrophage-associated markers (such as CD68 [11.9%], CCR2 [13.9%] and CSF1R [22.8%]), metabolic immune escape markers (such as ADORA2A [8.9%] and IDO1 [12.9%]), and anti-inflammatory immune markers (such as IL10 [20.8%]) (, Supplemental Table 2 and Supplemental Figure 1). Theoretically, patients harboring high expression of immune-suppressive markers may benefit by targeting the specific inhibitory markers (e.g. to selectively administer IDO1 inhibitor among patients found to have high expression of IDO1) (Supplemental Table 1). On the other hand, expression of certain immune-stimulatory factors was low among patients with diverse cancers (e.g., very low/low expression was seen in ICOS ligand [38.6% of 101 patients]), CD40 ligand [53.5%] and GITR [53.5%]). For those patients with low expression of immune-stimulatory factors, it is conceivable that interventions that enhance the stimulatory factor may have a higher chance of demonstrating anti-cancer immune effects when compared to giving such stimulators to patients who may already have high endogenous expression of the molecule (Supplemental Table 1).
Figure 2. Overview of mRNA expression level of multiple immune markers for each individual cases (N = 101)
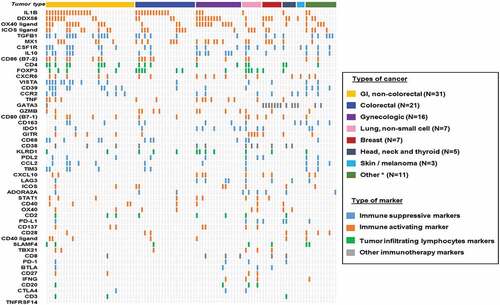
Especially pertinent for the development of various immunotherapy agents was the observation that the immune environment differed from patient to patient. Indeed, most patients (87.1%, 88/101) had distinct expression patterns of cancer-immunity markers. This observation is comparable to that reported in the cancer genomic field where the landscape of genomic alterations is complex and differs between patients even when they harbor tumors of the same histologic diagnosisCitation36 Admittedly, there are some pattern similarities within histologies (in both the genomic portfolios patients carry and, per our study, in the immune landscape of tumors). For instance, patients with colorectal cancer appeared to have higher expression of the inflammatory cytokine IL1B when compared to non-colorectal cancer patients (Supplemental Table 3). The high IL1B could conceivably be due to the fact that KRAS alterations (common in colorectal cancer) have been previously shown to stimulate signal transduction pathways that activate the IL1B promoterCitation37 Still, high IL1B was not exclusive to colorectal cancer, and an immunoprint of our patients () illustrates the diversity of immune portfolios within and between histologies. Additionally, most patients (99% [100/101]) had potentially targetable cancer-immunity markers that were pharmacologically tractable with either an FDA-approved agent (on- or off-label) or with an agent that is in clinical investigation (median number of potentially actionable immune biomarkers per patient: 6) ( and Supplemental Table 1). Taken together, these findings suggest that the current standard of one-size-fits-all approach for immunotherapy may not be ideal for optimizing responsiveness and that obtaining an individualized immunogram Citation38 and treating on that basis warrants exploration.
Although durable clinical response can be seen with ICIs in a portion of patients, most patients do not demonstrate response or unfortunately have a short duration of clinical responses. Thus it is essential to understand the underlying biomarkers that may predict both response and resistance. In addition to known response markers (e.g. high TMB, MSI-high, deficiency in mismatch repair genes and highPD-L1 expression or amplification),Citation9–Citation12,Citation14 several resistance markers for immune therapy have also been reported: PTEN, STK11/LKB1 alterations and activation of WNT/beta catenin for innate resistance markers, alterations in JAK2 or beta-2-microglobulin as an acquired resistance, and EGFR and MDM2 alterations as potential markers for hyperprogression.Citation8,Citation39–Citation42
In this regard, we examined immune markers that might correlate with attenuated anti-PD-1/PD-L1 responsiveness ( and ). Importantly, patients with high expression of alternative checkpoints such as TIM-3 and VISTA, as well as the macrophage-associated markers CD68, had significantly shorter PFS (). TIM-3, also known as Hepatitis A Virus Cellular Receptor 2 (HAVCR2), is a cell surface receptor expressed on activated T cells. Binding of C-type lectin galectin-9 (ligand of TIM-3) to TIM-3 leads to an immune inhibitory signal.Citation43 Further, TIM-3 upregulation is associated with resistance to anti-PD-1 therapies in preclinical modelsCitation44 Additionally, VISTA, also known as V-Set Immunoregulatory Receptor (VSIR), is an immunoregulatory receptor that inhibits the T-cell activationCitation45 Among patients with prostate cancer treated with ipilimumab (anti-CTLA-4 antibody), upregulation of VISTA was observed, suggesting a compensatory inhibitor pathway as a resistance mechanism after ipilimumab therapyCitation46 Moreover, increased expression of VISTA was seen among melanoma patients who progressed on anti-PD-1 inhibitor therapy, also suggesting a role for VISTA as an immune checkpointCitation47 Since TIM-3 and VISTA were both implicated as resistant markers for anti-PD-1/PD-L1 based immunotherapy in our dataset, targeting of TIM-3 and VISTA may be required to achieve a better clinical outcome in selected patients. In the current study, high expression of CD68 was also significantly associated with poor PFS after anti-PD-1/PD-L1 based immunotherapy (). CD68 is a tumor-associated macrophage (TAM) marker that can have pro-tumoral and immunosuppressive functions. High TAM correlates with poor prognosis in breast cancer and myxoid liposarcoma patients.Citation48,Citation49 In the preclinical setting, TAM has been reported to play a key role in resistance to anti-PD-1 therapy,Citation50 which is in line with our current clinical observations.
There were several limitations to our study. First, the sample size was relatively small and was heterogeneous. Thus, further validation in a larger dataset is needed. This is especially pertinent for the clinical data, which requires a larger sample size that would permit a multivariate analysis. Second, since the number of patients for each cancer diagnosis was based on the number of tests requested for cancer-immunity cycle markers by the treating physician, there is a potential of sample size bias. Third, though the cancer immune landscape data was collected prospectively, the analysis of correlation with immunotherapy treatment outcome was evaluated retrospectively. Fourth, mRNA expression does not always correlate the protein expression. Moreover, immune markers can be expressed on both immune and tumor cells, which may be differentiated by immunohistochemistry testing, and requires further investigation. Fifth, dynamic changes in cancer-immunity markers can be observed, especially with anti-cancer therapies. In-depth analysis of the association between therapy and immunity markers is required. Despite these limitations, the current report provides a comprehensive analysis of cancer-immunity cycle markers and clinical correlates in the pan-cancer setting.
In conclusion, we have investigated 101 patients with diverse cancers and demonstrated that most patients (87.1%, 88/101) had distinct (and complex) patterns of cancer-immunity markers. The majority of patients (99% [100/101]) had multiple potentially targetable cancer-immunity markers (with either an FDA-approved agent [on- or off-label] or with an agent that is in clinical investigation). Therapeutically, high expression of the checkpoints TIM-3 and VISTA as well as the macrophage-associated marker CD68 were associated with significantly worse PFS after anti-PD-1/PD-L1 based immunotherapies. Our observations suggest that upregulation of alternative checkpoints or myeloid suppression (via macrophage-associated markers) may diminish responsiveness to anti-PD-1/PD-L1 agents. Furthermore, individualizing immunotherapy agents to each patient’s tumor immune portfolio merits investigation for optimized outcomes.
Materials and methods
Patients
The cancer-immunity markers among 101 eligible consecutive patients with diverse solid cancers seen at the University of California San Diego Moores Cancer Center for Personalized Therapy were examined at a Clinical Laboratory Improvement Amendments (CLIA)-licensed and College of American Pathologist (CAP)-accredited clinical laboratory, OmniSeq (https://www.omniseq.com/). We used the electronic medical record to curate the clinical characteristics of these patients. All investigations followed the guidelines of the UCSD Institutional Review Board for data collection (Profile Related Evidence Determining Individualized Cancer Therapy, NCT02478931) and for any investigational therapies for which the patients consented. Among patients who had anti-PD-1/PD-L1 based therapy (N = 39), all samples were collected prior to the checkpoint inhibitor based therapy.
Tissue samples and analysis of cancer-immunity markers
Tumors were provided as formalin-fixed, paraffin-embedded (FFPE) samples and evaluated with RNA sequencing by OmniSeq laboratory. Briefly, total RNA was extracted from FFPE by means of the truXTRAC FFPE extraction kit (Covaris, Inc., Woburn, MA), following the manufacturer’s instructions with some modifications. Following purification, RNA was eluted in 50 µL water and yield was determined by the Quant-iT RNA HS Assay (Thermo Fisher Scientific, Waltham, MA), as per manufacturer’s recommendation. A predefined yield of 10 ng RNA was used as acceptance criteria to ensure adequate library preparation. RNA-sequencing absolute reads were generated using Torrent Suite’s plugin immuneResponseRNA (v5.2.0.0)Citation34 The RNA library was prepared to measure RNA expression of 51 targeted immune response markers including checkpoint markers (N = 9 markers including PD-L1, PD-L2, CTLA-4 and LAG3), macrophage-associated markers (N = 5 markers including CCR2, CD68 and CSF1R), metabolic immune escape markers (N = 3 markers including ADORA2A and IDO1), anti-inflammatory response markers (N = 2 markers, IL10 and TGFB1), T-cell primed markers (N = 15 markers including CD40, GITR, ICOS and OX40), pro-inflammatory response markers (N = 7 markers including IL1B and TNF), tumor infiltrating lymphocytes markers (N = 8 markers including CD4, CD8 and FOXP3) and other immunotherapy markers (N = 2) (Supplemental Table 1). Transcript abundance was normalized and compared to an internal reference population (N = 735 patients with diverse cancers) which were used to rank RNA expression in test samplesCitation34 Rank values were set on a scale of 1 to 100 and stratified into “Very high” (95–100), “High” (85–94), “Moderate” (50–84), “Low” (20–49), and “Very Low” (0–19). Tissue samples were obtained via biopsies in 54 patients (53.5%) and via surgically resected samples in 47 patients (46.5%). Moreover, in 50 patients (49.5%), the biopsies were from the primary tumor and in 49 patients (48.5%) they were from metastatic sites (N = 2 with carcinoma of unknown primary).
Endpoints and statistical methods
Patient characteristics and the pattern of cancer-immunity markers were summarized by descriptive statistics. The Fisher’s exact test and the Bonferroni correction were used for categorical variables. Response to anti-PD-1/PD-L1 based immunotherapy were assessed by imaging (e.g. computed tomography and/or magnetic resonance imaging) and categorized into progressive disease, SD, PR and CR according to the treating physician’s evaluation (immunotherapy response is evaluated by iRECIST criteria). PFS was defined as time interval between the start of therapy and the date of disease progression. Patients with ongoing therapy without progression at the last follow up date were censored for PFS at that date. Log-rank test and Bonferroni correction were used to compare subgroups of patients. All tests were 2-sided and P-values ≤ 0.05 were considered significant. Statistical analyses were performed with assistance from coauthor RO using SPSS version 24.0 (Chicago, IL, USA).
Disclosure of Potential Conflicts of Interest
Shumei Kato serves as a consultant for Foundation Medicine. Razelle Kurzrock receives research funding from Genentech, Incyte, Merck, Serono, Pfizer, Sequenom, Foundation Medicine, Grifols, and Guardant, as well as consultant fees from Loxo, X Biotech, NeoMed, and Actuate Therapeutics, speaker fees from Roche, and has an equity interest in IDby DNA and Curematch Inc.
Supplemental Material
Download ()Supplementary Material
Supplemental data for this article can be accessed on the publisher’s website.
Additional information
Funding
References
- Yang Y. Cancer immunotherapy: harnessing the immune system to battle cancer. J Clin Invest. 2015;125:3335–9.doi:10.1172/JCI83871.
- Chen L, Han X. Anti–PD-1/PD-L1 therapy of human cancer: past, present, and future. J Clin Invest. 2015;125:3384–3391. doi:10.1172/JCI80011.
- Ansell SM, Lesokhin AM, Borrello I, Halwani A, Scott EC, Gutierrez M, Schuster SJ, Millenson MM, Cattry D, Freeman GJ, et al. PD-1 blockade with nivolumab in relapsed or refractory Hodgkin’s lymphoma. N Engl J Med. 2015;372:311–319. doi:10.1056/NEJMoa1411087.
- Antonia SJ, Villegas A, Daniel D, Vicente D, Murakami S, Hui R, Yokoi T, Chiappori A, Lee KH, de Wit M, et al. Durvalumab after chemoradiotherapy in stage III non–small-cell lung cancer. N Engl J Med. 2017;377:1919–1929. doi:10.1056/NEJMoa1709937.
- Hodi FS, O’day SJ, McDermott DF, Weber RW, Sosman JA, Haanen JB, Gonzalez R, Robert C, Schadendorf D, Hassel JC et al. Improved survival with ipilimumab in patients with metastatic melanoma. N Engl J Med. 2010;363:711–723. doi:10.1056/NEJMoa1003466.
- Powles T, O’donnell PH, Massard C, Arkenau H-T, Friedlander TW, Hoimes CJ, Lee JL, Ong M, Sridhar SS, Vogelzang NJ, et al. Efficacy and safety of durvalumab in locally advanced or metastatic urothelial carcinoma: updated results from a phase 1/2 open-label study. JAMA Oncol. 2017;3:e172411–e172411. doi:10.1001/jamaoncol.2017.2411.
- Topalian SL, Hodi FS, Brahmer JR, Gettinger SN, Smith DC, McDermott DF, Powderly JD, Carvajal RD, Sosman JA, Atkins MB, et al. Safety, activity, and immune correlates of anti–PD-1 antibody in cancer. N Engl J Med. 2012;366:2443–2454. doi:10.1056/NEJMoa1200690.
- Kato S, Goodman A, Walavalkar V, Barkauskas DA, Sharabi A, Kurzrock R. Hyperprogressors after immunotherapy: analysis of genomic alterations associated with accelerated growth rate Clin Cancer Res. 2017;23:4242–4250. doi:10.1158/1078-0432.CCR-16-3133.
- Goodman AM, Piccioni D, Kato S, Boichard A, Wang H-Y, Frampton G, Lippman SM, Connelly C, Fabrizio D, Miller V, et al. Prevalence of PDL1 amplification and preliminary response to immune checkpoint blockade in solid tumors. JAMA Oncol. 2018;4:1237 doi:10.1001/jamaoncol.2018.1701.
- Patel SP, Kurzrock R. PD-L1 expression as a predictive biomarker in cancer immunotherapy. Mol Cancer Ther. 2015;0983:2014.
- Vanderwalde A, Spetzler D, Xiao N, Gatalica Z, Marshall J. Microsatellite instability status determined by next‐generation sequencing and compared with PD‐L1 and tumor mutational burden in 11,348 patients. Cancer Med. 2018;7:746–756.doi:10.1002/cam4.2018.7.issue-3.
- Le DT, Durham JN, Smith KN, Wang H, Bartlett BR, Aulakh LK, Lu S, Kemberling H, Wilt C, Luber BS, et al. Mismatch repair deficiency predicts response of solid tumors to PD-1 blockade. Science. 2017;357:409–413. doi:10.1126/science.aan6733.
- Chen P-L, Roh W, Reuben A, Cooper ZA, Spencer CN, Prieto PA, Miller JP, Bassett RL, Gopalakrishnan V, Wani K, et al. Analysis of immune signatures in longitudinal tumor samples yields insight into biomarkers of response and mechanisms of resistance to immune checkpoint blockade. Cancer Discov. 2016;6:827–837. doi:10.1158/2159-8290.CD-15-1545.
- Goodman AM, Kato S, Bazhenova L, Patel SP, Frampton GM, Miller V, Stephens PJ, Daniels GA, Kurzrock R. Tumor mutational burden as an independent predictor of response to immunotherapy in diverse cancers. Mol Cancer Ther. 2017;16:2598–2608.
- Dijkstra KK, Voabil P, Schumacher TN, Voest EE. Genomics-and transcriptomics-based patient selection for cancer treatment with immune checkpoint inhibitors: a review. JAMA Oncol. 2016;2:1490–1495. doi:10.1001/jamaoncol.2016.2214.
- Mushtaq MU, Papadas A, Pagenkopf A, Flietner E, Morrow Z, Chaudhary SG, Asimakopoulos F. Tumor matrix remodeling and novel immunotherapies: the promise of matrix-derived immune biomarkers. J ImmunoTher Cancer. 2018;6:65.doi:10.1186/s40425-018-0376-0.
- Zhu Y, Zhao F, Li Z, Yu J. Current landscape and future directions of biomarkers for predicting responses to immune checkpoint inhibitors. Cancer Manag Res. 2018;10:2475.
- Chen DS, Hurwitz H. Combinations of bevacizumab with cancer immunotherapy. Cancer J. 2018;24:193–204. doi:10.1097/PPO.0000000000000327.
- Hoffman-Censits J, Wilde L.Combination immunotherapy in genitourinary malignancies. Curr Opin Urol. 2016;26:523–528. doi:10.1097/MOU.0000000000000336.
- Tang J, Shalabi A, Hubbard-Lucey VM. Comprehensive analysis of the clinical immuno-oncology landscape. Ann Oncol 2018;29:84–91. doi:10.1093/annonc/mdx755.
- Gandhi L, Rodríguez-Abreu D, Gadgeel S, Esteban E, Felip E, De Angelis F, Domine M, Clingan P, Hochmair MJ, Powell SF, et al. Pembrolizumab plus chemotherapy in metastatic non–small-cell lung cancer. N Engl J Med. 2018;378:2078–2092. doi:10.1056/NEJMoa1801005.
- Long GV, Dummer R, Hamid O, Gajewski T, Caglevic C, Dalle S, Arance A, Carlino MS, Grob -J-J, Kim TM, et al. Epacadostat (E) plus pembrolizumab (P) versus pembrolizumab alone in patients (pts) with unresectable or metastatic melanoma: results of the phase 3 ECHO-301/KEYNOTE-252 study, Am Soc Clin Oncol. 2018;36:108. doi:10.1200/JCO.2018.36.15_suppl.108.
- Nikanjam M, Patel H, Kurzrock R. Dosing immunotherapy combinations: analysis of 3,526 patients for toxicity and response patterns. Oncoimmunology 2017;6:e1338997. doi:10.1080/2162402X.2017.1338997.
- Wolchok JD, Chiarion-Sileni V, Gonzalez R, Rutkowski P, Grob J-J, Cowey CL, Lao CD, Wagstaff J, Schadendorf D, Ferrucci PF, et al. Overall survival with combined nivolumab and ipilimumab in advanced melanoma. N Engl J Med. 2017;377:1345–1356. doi:10.1056/NEJMoa1709684.
- Kurzrock R, Gutterman JU, Talpaz M. The molecular genetics of Philadelphia chromosome-positive leukemias. N Engl J Med. 1988;319:990–998. doi:10.1056/NEJM198810133191506.
- Soria J-C, Ohe Y, Vansteenkiste J, Reungwetwattana T, Chewaskulyong B, Lee KH, Dechaphunkul A, Imamura F, Nogami N, Kurata T, et al. Osimertinib in untreated EGFR-mutated advanced non-small-cell lung cancer. N Engl J Med. 2018;378:113–125. doi:10.1056/NEJMoa1713137.
- Shaw AT, Kim D-W, Nakagawa K, Seto T, Crinó L, Ahn M-J, De Pas T, Besse B, Solomon BJ, Blackhall F, et al. Crizotinib versus chemotherapy in advanced ALK-positive lung cancer. N Engl J Med 2013;368:2385–2394. doi:10.1056/NEJMoa1214886.
- Fontes Jardim DL, Schwaederle M, Wei C, Lee JJ, Hong DS, Eggermont AM, Schilsky RL, Mendelsohn J, Lazar V, Kurzrock R, et al. Impact of a biomarker-based strategy on oncology drug development: a meta-analysis of clinical trials leading to FDA approval. JNCI. 2015;107. doi:10.1093/jnci/djv253.
- Schwaederle M, Zhao M, Lee JJ, Eggermont AM, Schilsky RL, Mendelsohn J, Lazar V, Kurzrock R. Impact of precision medicine in diverse cancers: a meta-analysis of phase ii clinical trials. J Clin Oncol. 2015;33:3817–3825. doi:10.1200/JCO.2015.61.5997.
- Schwaederle M, Zhao M, Lee JJ, Lazar V, Leyland-Jones B, Schilsky RL, Mendelsohn J, Kurzrock R. Association of biomarker-based treatment strategies with response rates and progression-free survival in refractory malignant neoplasms: a meta-analysis. JAMA Oncol. 2016;2:1452–1459. doi:10.1001/jamaoncol.2016.2129.
- Sicklick JK, Kato S, Okamura R, Schwaederle M, Hahn ME, Williams CB, De P, Krie A, Piccioni DE, Miller VA, et al. Molecular profiling of cancer patients enables personalized combination therapy: the I-PREDICT study. Nat Med. 2019;25:744–750. doi:10.1038/s41591-019-0407-5.
- Wheler JJ, Janku F, Naing A, Li Y, Stephen B, Zinner R, Subbiah V, Fu S, Karp D, Falchook GS, et al. Cancer therapy directed by comprehensive genomic profiling: a single center study. Cancer Res. 2016;76:3690–3701. doi:10.1158/0008-5472.CAN-15-3043.
- Chen DS, Mellman I. Oncology meets immunology: the cancer-immunity cycle. Immunity 2013;39:1–10. doi:10.1016/j.immuni.2013.07.012.
- Conroy JM, Pabla S, Glenn ST, Burgher B, Nesline M, Papanicolau-Sengos A, Andreas J, Giamo V, Lenzo FL, Hyland FCL, et al. Analytical validation of a next-generation sequencing assay to monitor immune responses in solid tumors. J Mol Diagn. 2018;20:95–109. doi:10.1016/j.jmoldx.2017.10.001.
- Drake CG. Combination immunotherapy approaches. Ann Oncol. 2012;23:viii41–viii46. doi:10.1093/annonc/mds262.
- Kurzrock R, Giles FJ. Precision oncology for patients with advanced cancer: the challenges of malignant snowflakes. Cell Cycle. 2015;14:2219–2221. doi:10.1080/15384101.2015.1041695.
- Beaupre DM, Talpaz M, Marini FC 3rd, Cristiano RJ, Roth JA, Estrov Z, Albitar M, Freedman MH, Kurzrock R. Autocrine interleukin-1beta production in leukemia: evidence for the involvement of mutated RAS. Cancer Res. 1999;59:2971–2980.
- Blank CU, Haanen JB, Ribas A, Schumacher TN. CANCER IMMUNOLOGY. The “cancer immunogram”. Science. 2016;352: 658–660. doi:10.1126/science.aaf2834.
- Kato S, Ross JS, Gay L, Dayyani F, Roszik J, Subbiah V, Kurzrock R. Analysis of MDM2 amplification: next-generation sequencing of patients with diverse malignancies. JCO Precis Oncol. 2018. doi:10.1200/PO.17.00235.
- Peng W, Chen JQ, Liu C, Malu S, Creasy C, Tetzlaff MT, Xu C, McKenzie JA, Zhang C, Liang X, et al. Loss of PTEN promotes resistance to T cell-mediated immunotherapy. Cancer Discov. 2016;6:202–216. doi:10.1158/2159-8290.CD-15-0283.
- Sade-Feldman M, Jiao YJ, Chen JH, Rooney MS, Barzily-Rokni M, Eliane J-P, Bjorgaard SL, Hammond MR, Vitzthum H, Blackmon SM, et al. Resistance to checkpoint blockade therapy through inactivation of antigen presentation. Nat Commun. 2017;8:1136. doi:10.1038/s41467-017-01062-w.
- Spranger S, Bao R, Gajewski TF. Melanoma-intrinsic beta-catenin signalling prevents anti-tumour immunity. Nature. 2015;523:231–235. doi:10.1038/nature14404.
- Anderson AC. Tim-3: an emerging target in the cancer immunotherapy landscape. Cancer Immunol Res. 2014;2:393–398. doi:10.1158/2326-6066.CIR-14-0039.
- Shayan G, Srivastava R, Li J, Kane LP, Ferris RL. Adaptive resistance to anti-PD1 therapy by Tim-3 upregulation is mediated by the PI3K-Akt pathway in head and neck cancer. Oncoimmunology. 2017;6:e1261779. doi:10.1080/2162402X.2016.1261779.
- Lines JL, Pantazi E, Mak J, Sempere LF, Wang L, O’Connell S, Ceeraz S, Suriawinata AA, Yan S, Ernstoff MS, et al. VISTA is an immune checkpoint molecule for human T cells. Cancer Res. 2014;74:1924–1932. doi:10.1158/0008-5472.CAN-13-1504.
- Gao J, Ward JF, Pettaway CA, Shi LZ, Subudhi SK, Vence LM, Zhao H, Chen J, Chen H, Efstathiou E, et al. VISTA is an inhibitory immune checkpoint that is increased after ipilimumab therapy in patients with prostate cancer. Nat Med. 2017;23:551–555. doi:10.1038/nm.4308.
- Kakavand H, Jackett LA, Menzies AM, Gide TN, Carlino MS, Saw RPM, Thompson JF, Wilmott JS, Long GV, Scolyer RA, et al. Negative immune checkpoint regulation by VISTA: a mechanism of acquired resistance to anti-PD-1 therapy in metastatic melanoma patients. Mod Pathol. 2017;30:1666–1676. doi:10.1038/modpathol.2017.89.
- Mahmoud SM, Lee AH, Paish EC, Macmillan RD, Ellis IO, Green AR. Tumour-infiltrating macrophages and clinical outcome in breast cancer. J Clin Pathol. 2012;65:159–163. doi:10.1136/jclinpath-2011-200355.
- Nabeshima A, Matsumoto Y, Fukushi J, Iura K, Matsunobu T, Endo M, Fujiwara T, Iida K, Fujiwara Y, Hatano M, et al. Tumour-associated macrophages correlate with poor prognosis in myxoid liposarcoma and promote cell motility and invasion via the HB-EGF-EGFR-PI3K/Akt pathways. Br J Cancer 2015;112:547. doi:10.1038/bjc.2014.637.
- Arlauckas SP, Garris CS, Kohler RH, Kitaoka M, Cuccarese MF, Yang KS, Miller MA, Carlson JC, Freeman GJ, Anthony RM, et al. In vivo imaging reveals a tumor-associated macrophage-mediated resistance pathway in anti-PD-1 therapy. Sci Transl Med. 2017;9:eaal3604. doi:10.1126/scitranslmed.aal3604.