ABSTRACT
Blockade of the PD-1 receptor has revolutionized the treatment of metastatic melanoma, with significant increases in overall survival (OS) and a dramatic improvement in patient quality of life. Despite the success of this approach, the number of benefitting patients is limited and there is a need for predictive biomarkers as well as a deeper mechanistic analysis of the cellular populations involved in clinical responses. With the aim to find predictive biomarkers for PD-1 checkpoint blockade, an in-depth immune monitoring study was conducted in 36 advanced melanoma patients receiving pembrolizumab or nivolumab treatment at Karolinska University Hospital. Blood samples were collected before treatment and before administration of the second and fourth doses. Peripheral blood mononuclear cells were isolated and stained for flow cytometric analysis within 2 h of sample collection. Overall survival and progression-free survival (PFS) were inversely correlated with CD69 expression NK cells. In the myeloid compartment, high frequencies of non-classical monocytes and low frequencies of monocytic myeloid derived suppressor cells (MoMDSCs) correlated with response rates and OS. A deeper characterization of monocytic subsets showed that PD-L1 expression in MDSCs, non-classical and intermediate monocytes was significantly increased in patients with shorter PFS in addition to correlating inversely with OS. Our results suggest that cellular populations other than T cells can be critical in the outcome of PD-1 blockade treatment. Specifically, the frequencies of activated NK cells and monocytic subsets are inversely correlated with survival and clinical benefit, suggesting that their role as predictive biomarkers should be further evaluated.
Introduction
The role of the immune system in the surveillance and elimination of several types of human cancers has been known for a long time, but not until recently has this knowledge been transformed into clinically useful cancer therapies.Citation1,Citation2 The recent surge in cancer immunotherapies is based on a better knowledge of the regulation of the interaction between cancer cells and the immune system via immunologic checkpoints. Antibody blockade of the checkpoints cytotoxic T lymphocyte-associated antigen 4 (CTLA-4) and the programmed cell death protein 1 pathway (PD-1/PD-L1) has demonstrated efficacy in several malignancies, especially in melanoma, having completely revolutionized treatment of metastatic disease. Currently, the standard of care for melanoma patients includes PD-1 blockade with either of two antibodies: pembrolizumab or nivolumab.Citation3 Response rates are higher than those observed with ipilimumab treatment and the immune-related side effects, while still present, are lower.
The significant incidence of side effects and the relatively modest number of patients benefiting from treatment are, however, limitations to this therapeutic approach. To help overcome these limitations there is an urgent need to identify biomarkers for those patients that respond to treatment. Since both the treatment and the side effects are immune mediated, there should be a high likelihood to find such biomarkers by studying the patient´s immune system.
With a meticulously designed strategy of sample collection, analysis and biobanking, we conducted a thorough immune monitoring study of patients undergoing PD-1 checkpoint blockade. This would allow us to find biomarker candidates that could predict clinical outcome and design experimental approaches to improve our understanding of the detailed mechanisms of treatment efficacy.
The PD-1 checkpoint receptor is expressed mainly on T cells, but NK cells have recently been described to express PD-1, especially in physiological conditions related to poor prognosis in different malignancies.Citation4,Citation5 Although NK cells can be crucial in tumor elimination, little is known about their role in checkpoint blockade therapy, hence their inclusion in this study.
Although they have not been reported to express PD-1 on their surface, Myeloid Derived Suppressor Cells (MDSCs) accumulate in cancer patients.Citation6–Citation8 Studies carried out in our group described the presence of increased numbers of monocytic MDSCs (MoMDSCs) in the peripheral blood of patients with stage III–IV melanoma.Citation9 These MDSCs mediate strong suppression of CD8 and CD4 T-cell proliferation and inhibit T-cell IFN-γ production.Citation9 However, their presence appears to be strongly tumor-dependent, as they rapidly disappear once the tumor is surgically removed or successfully diminished by other treatments.Citation8,Citation10 We recently described that checkpoint blockade with ipilimumab has significant effects on reducing these suppressive mechanisms and in some cases correlates with clinical benefit and overall survival.Citation2,Citation11 Furthermore, myeloid cells are an important source of host PD-L1, acting as key regulators of the immune response via the PD-1/PD-L1 pathway. It is therefore conceivable that, in patients with advanced malignant melanoma, myeloid cells in general and MDSCs in particular can have a major influence on their ability to respond to PD-1 checkpoint blockade.
The main objective of this study was to evaluate the immune system of patients undergoing treatment with the anti-PD1 blocking antibodies pembrolizumab or nivolumab, with the intent of discovering biomarker candidates that could predict progression-free survival (PFS) or could be correlated with overall survival. To do this, we analyzed cellular populations and immune-related phenotypic markers from fresh peripheral blood samples taken in patients with advanced melanoma before and during PD-1 checkpoint blockade treatment.
Results
Treatment outcome and patient evaluation
Between February 2015 and July 2018, 36 patients between 37 and 83 y were enrolled in this study (Supplementary Table 1). Thirteen (36%) patients discontinued the treatment due to complete response or durable stable disease. At the time of data cutoff, 17 (47%) patients had died. Median overall survival (MOS) was 886 d and median progression-free survival (PFS) was 264 d (Supplementary Figure 1).
Objective response rate (defined as complete response or partial response) was observed in 18 (50%) patients, out of which 10 (28%) had complete response (CR) and 8 (22%) had partial response (PR). Three patients (8%) had mixed response (MR, defined as a combination of tumor growth and tumor regression) and two (6%) had stable disease (SD). Thirteen (36%) of the treated patients did not benefit from treatment and had progressive disease (PD).
For analytical purposes, the patients were divided into those with long PFS (progression-free survival) >6 months and short PFS <6 months. Sixteen patients had short PFS and 20 patients had long PFS. Out of the 20 patients with long PFS 10 had CR, 7 PR, 1 MR and 2 SD. Out of the 16 patients with short PFS 13 had PD, 2 MR and 1 PR. The only patient with a partial response and short PFS had a late response with marked regression that started 7 weeks after discontinuing nivolumab treatment. This response lasted 10 weeks before progression and the patient showed clinical benefit during this time period. With the exception of this patient, response was correlated with short PFS. The median overall survival for the group with short PFS was 318 d and for the group with long PFS 1191 d, showing that time to progression correlates with survival (Supplementary Figure 1)
Any-grade treatment-related adverse events occurred in 21 (58%) of patients; most of these treatment-related adverse events were grades 1–2. The most common any-grade treatment-related adverse events were fatigue, muscle or joint pain and thyroiditis. Grade 3–5 treatment-related toxicities occurred in 5 (14%) of patients. Treatment-related toxicity resulted in treatment discontinuation for 6 (17%) of the treated patients. Treatment-related toxicity was generally easily managed with supportive care, withholding treatment and/or, when indicated, corticosteroids.
Multivariate analysis and preselection of biomarker candidates
A total of 55 parameters were used to build a total of 6 multivariate projection models at each of the sampling time points using two different stratification strategies (Supplementary Table 2). In the first case, the discriminant variable was progression-free survival (shorter than 6 months vs. longer than 6 months). The second stratification strategy used overall survival to stratify the cohort (OS > 18 months vs. OS < 18 months). This modeling method, Orthogonal Projections to Latent Structures, Discriminant Analysis (OPLS-DA) is based on Principal Component Analysis, and simultaneously takes all variables into account with equal importance independent of the range of values.
In the model comparing baseline samples with PFS as a discriminatory variable, a separation between patients with short and long PFS () with an overall fit (R2) value of 73.5%, a predictive capacity (Q2) of 54.9% and a highly significant cross-validated ANOVA P value (p[CV-ANOVA] = 0.0001) was observed. The loadings plot () showed 13 parameters that could be correlated with PFS, although only 9 were significant considering the jack-knifed confidence intervals (BRAF status, Leukocyte counts and LDH were not significant). After the scaled loadings (p(corr)[1]) and the variable importance in the projections (VIP) were plotted (), these 13 parameters were selected for validation by univariate analysis.
Figure 1. Discriminant analysis of melanoma patients (n = 36) treated with PD-1 checkpoint inhibitors at baseline. (a) Discriminant analysis related to PFS: green squares, PFS >6 months, blue squares, PFS <6 months. The horizontal axis represents the predictive, the vertical axis represents the orthogonal component. Ellipse Hotelling’s T2 95% confidence interval limit. (b) Loadings plot with the 14 most relevant variables correlated with PFS. Error bars represent jack-knifed 95% confidence intervals. Positive correlation to long PFS means negative correlation to short PFS, and vice versa. (c) Combined plot showing the scaled loadings (p(corr)[1]) and the variable importance in the projections (VIP) according to PFS; dashed lines mark VIP values = 1.0, and |p(corr[1])| = 0.4, which were used as cutoff points for biomarker selection. Panels d–f show the discriminant analysis (d), loadings (e) and p(corr)[1]/VIP plot (f) when OS (long vs. short) was used as a discriminant binary variable.
![Figure 1. Discriminant analysis of melanoma patients (n = 36) treated with PD-1 checkpoint inhibitors at baseline. (a) Discriminant analysis related to PFS: green squares, PFS >6 months, blue squares, PFS <6 months. The horizontal axis represents the predictive, the vertical axis represents the orthogonal component. Ellipse Hotelling’s T2 95% confidence interval limit. (b) Loadings plot with the 14 most relevant variables correlated with PFS. Error bars represent jack-knifed 95% confidence intervals. Positive correlation to long PFS means negative correlation to short PFS, and vice versa. (c) Combined plot showing the scaled loadings (p(corr)[1]) and the variable importance in the projections (VIP) according to PFS; dashed lines mark VIP values = 1.0, and |p(corr[1])| = 0.4, which were used as cutoff points for biomarker selection. Panels d–f show the discriminant analysis (d), loadings (e) and p(corr)[1]/VIP plot (f) when OS (long vs. short) was used as a discriminant binary variable.](/cms/asset/a3ea48b6-5790-4d16-9ddb-189fc9649a20/koni_a_1786888_f0001_oc.jpg)
Equivalent results were observed in the baseline model when OS was used as a discriminatory parameter, where separation was also visible between long and short survivors (). This model had good overall fit (R2 = 68.8%), predictive capacity (Q2 = 43.1%) and a highly significant p[CV-ANOVA] = 0.0028. In this case, the loadings plot showed 15 candidates to be correlated with OS, 13 of which were significant after considering the jack-knifed confidence intervals (). The p(corr)[1]/VIP plot shows the parameters that were selected as candidates for further validation ().
The projection models built for timepoints 1 and 2 are shown in Supplementary Figure 2. In these cases, only the PFS model at timepoint 1 resulted in acceptable fit, predictive capacity and significant p[CV-ANOVA] values (Supplementary Table 2). The relevant parameters associated to short PFS after the first dose of anti- PD-1 therapy were BRAF status (wild type), the absence of any-grade treatment-related adverse events (AEs) and MoMDSCs.
No significant outliers were found at any of the timepoints using either of the discriminant variables (PFS or OS).
Additionally, using the presence/absence of AEs as a discriminatory variable, three projection models (one for each time point) were built. None of these models showed acceptable fit or predictive capacity and resulted in no association between the immune parameters included in this study with the onset of adverse events (data not shown).
Hematological measurements
PD-1 blockade did not cause significant changes in the absolute counts of leukocytes, lymphocytes, eosinophils, neutrophils or monocytes in the total patient cohort or when patients were separated according to their PFS (Supplementary Figure 3). However, when patients with clinical benefit were compared with those with progressive disease, we observed significant differences in several cell types (): Total leukocyte counts were significantly higher at baseline in patients with short PFS times (<6 months). Similarly, neutrophil counts were higher in the short PFS patient group. No significant differences were observed between short and long PFS patients regarding eosinophils, monocytes or the neutrophil to lymphocyte ratio (NLR) at any of the sampling timepoints (Supplementary Table 3).
Figure 2. Hematological parameters that correlate with Progression-Free Survival or Overall Survival. LDH (a–b), leukocytes (c–d), neutrophils (e–f) and NLR (g–h). Left column: Comparison between long and short PFS patients. Each dot represents an individual patient; mean ±95% confidence interval (CI) are represented. Unpaired Mann-Whitney U test: *, P < .05. The dashed line represents the cutoff point that divides each parameter into high and low as calculated using Cutoff Finder software. Right column Kaplan-Meier survival analysis after cutoff determination.
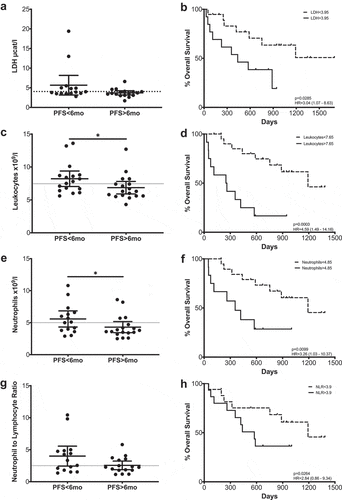
In view of these data, survival analysis was performed, comparing patients with high and low counts of each of the cell populations with the cutoff points initially being determined by the median values. If significant differences were found, the cutoff point was refined using cutoff finder. Significant differences were observed at baseline and at the first timepoint in the leukocyte counts, where, in agreement with the PFS data, higher counts correlated inversely with overall survival (OS) (p = .0003; p = .0203) (, Supplementary Figure 4). The patient group with high neutrophil counts at baseline presented shorter MOS (p = .0099). Although the differences were not statistically significant after patients received the first antibody infusion, a survival advantage was also observed at this time point (Supplementary Table 3).
The possible clinical relevance of neutrophils was further confirmed when overall survival according to NLR was analyzed (). In this case, a high ratio was always correlated with poorer survival times, with statistically significant results at baseline.
Furthermore, serum levels of lactate dehydrogenase (LDH) were measured during treatment. Although LDH levels did not change significantly during treatment, patients with elevated LDH values (>3,95 µcat/l) at baseline had a significantly shorter OS ().
NK cell activation markers correlate with clinical benefit and survival
NK cell frequencies did not change significantly in response to treatment nor were correlated with clinical outcome or survival (Supplementary Table 3). Simultaneously with relative frequencies, NK cells were also characterized according to surface marker expression (Supplementary Figure 5). No significant correlations with clinical outcome or survival were observed when HLA-DR, DNAM-1, NKG2D, CD16 or the CD56 dim/bright ratio were analyzed (Supplementary Table 3, Supplementary Figure 3).
However, the early activation marker CD69 did correlate with PFS both at baseline and at the first time point (). In this case, patients with PFS shorter than 6 months had circulating NK cells with significantly higher CD69+ frequencies. Receiver operating characteristic (ROC) curve analysis also showed that CD69 could be a potential PFS predictive biomarker with significant odds ratio (OR) values and area under the curve (AUC) values above 0.75 at both time points (Supplementary figure 6, Supplementary Table 3). CD69 expression in NK cells also correlated with survival at baseline and after the first and second treatment cycle; those patients with high frequencies of CD69+ NK cells had a significantly lower median (MOS) than patients with low frequencies. It is interesting to note that after analyzing the time course of CD69+ NK cells during PD-1 blockade treatment, significant differences were observed (). In this case, patients with long PFS times presented an increase in the frequency of CD69+ NK cells, showing statistical differences between the levels at baseline and at the second timepoint. In patients with short PFS times, this behavior was not observed.
Figure 3. CD69+ NK cells predict MOS and PFS. (a–c) Frequencies of CD69+ NK cells in patients with long and short PFS at baseline (a), timepoint 1 (b) and timepoint 2 (c). Each dot represents an individual patient, the dashed line represents the cutoff point that divides each parameter into high and low as calculated using Cutoff Finder software; mean ±95% CI are represented. Unpaired Mann-Whitney U test: *, P < .05; **, P < .01 (d-f) Kaplan-Meier survival analysis baseline (d), timepoint 1 (e) and timepoint 2 (f) after cutoff determination.
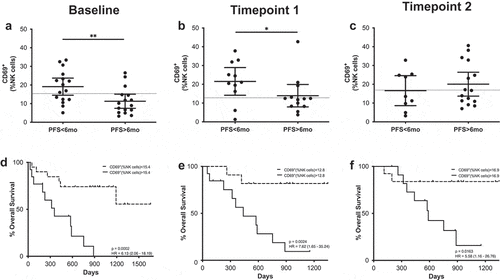
Figure 4. CD69+ NK cells increase during treatment in patients with long PFS. Frequencies of CD69+ NK cells in patients with long (a) and short PFS (b) at the different sampling timepoints. Each dot represents an individual patient, mean ±95% CI are represented. Kruskal-Wallis test followed by Dunn’s multiple comparisons test: *, P < .05.
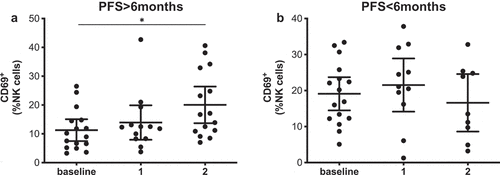
CD69 expression was further analyzed in the different NK cell subsets according to CD56 expression. At all three timepoints, a higher frequency of CD69+ cells was observed in the CD56bright subset (Supplementary figure 7). When PFS or OS were evaluated in this subset, no differences related to PFS or OS were observed (Supplementary figure 8). On the other hand, significant differences were observed in CD56dim NK cells (Supplementary figure 9): Patients with short PFS had significantly higher frequencies of CD69+ cells within this subset at baseline and after the first cycle of anti-PD-1 treatment and CD69 expression was inversely correlated with survival at all three timepoints. In the same manner as was observed for the total NK cell population, CD69 expression in the CD56dim subset was increased during treatment only in the patients with long PFS. Furthermore, ROC analysis consolidated these findings resulting in significant OR at baseline and at the first timepoint.
Additionally, a double staining for the activating receptors NKp30 and NKp46 was performed. Although no difference in the expression of these markers was found between the long and short PFS groups, a survival advantage was observed (Supplementary figure 10). In this case, patients with lower frequencies of NKp30/46+ NK cells at baseline had a significantly better MOS.
HLA-DR expression in CD14+ cells, clinical outcome and survival
CD14+ cells were gated from the myeloid population and were further classified according to their HLA-DR expression: CD14+HLA-DR+ (activated monocytes) and CD14+HLA-DRlo/- which have been extensively described as monocytic MDSCs (MoMDSCs) (Supplementary Figure 11).
At baseline, patients with PFS longer than 6 months had frequencies of circulating activated monocytes significantly higher than those patients with shorter PFS times (), and ROC analysis confirmed these cells as a possible PFS predictive marker (OR 1.1.23; p = .0245; ROC AUC = 0.76; 85.7% specificity; 58.8% sensitivity) (Supplementary figure 6). When survival according to the frequency of CD14+ HLA-DR+ cells was analyzed, patients with high frequencies of these cells presented longer survival times, although differences were not statistically significant.
Figure 5. CD14+HLA-DR+ cells predict MOS and PFS. (a–c) Frequencies of CD14+HLA-DR+ cells in patients with long and short PFS at baseline (a), timepoint 1 (b) and timepoint 2 (c). Each dot represents an individual patient, the dashed line represents the cutoff point that divides each parameter into high and low as calculated using Cutoff Finder software; mean ±95% CI are represented. Unpaired Mann-Whitney U test: ***, P < .001. (d–f) Kaplan-Meier survival analysis baseline (d), timepoint 1 (e) and timepoint 2 (f) after cutoff determination.
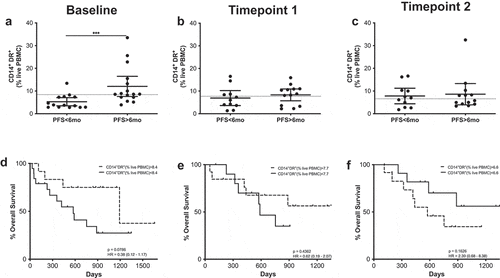
MoMDSCs were classified as CD14+HLA-DRlow/- cells and their overall frequencies did not change in response to treatment. When the patient cohort was divided according to PFS, significant differences were observed at both baseline and at the first timepoint (). At these timepoints, patients with long PFS presented significantly lower frequencies of MoMDSCs. Survival analysis further confirmed these data, showing that at these timepoints, patients with high frequencies of MoMDSCs had significantly shorter OS at baseline and after the first treatment cycle ().
Figure 6. MoMDSCs predict MOS and PFS. (a-c) Frequencies of CD14+HLA-DR− cells in patients with long and short PFS at baseline (a), timepoint 1 (b) and timepoint 2 (c). Each dot represents an individual patient, the dashed line represents the cutoff point that divides each parameter into high and low as calculated using Cutoff Finder software; mean ±95% CI are represented. Unpaired t-test: *, P < .05. (d-f) Kaplan-Meier survival analysis baseline (d), timepoint 1 (e) and timepoint 2 (f) after cutoff determination.
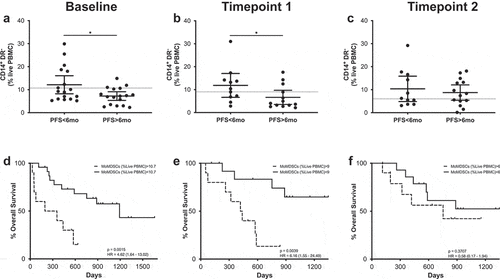
PD-L1 expression in the myeloid compartment
As a further characterization of circulating myeloid cells, PD-L1 expression was assessed as an indicator of both their activation status as well as their potential suppressive capacity. This analysis was carried out within different subsets of monocytes as is shown in Supplementary Figure 11. Surface expression of PD-L1 was measured in monocytes which were defined as myeloid (according to their forward and side scatter characteristics), CD3− CD14low/+ HLA-DRlow/+ cells. Monocytes were then subdivided into classical, intermediate and non-classical according to their CD14 and CD16 expressionCitation12 (Supplementary Figure 11). Classical monocytes are CD14+ CD16−, intermediate monocytes are CD14+ CD16− and non-classical monocytes are defined as CD14lo/- CD16+. PD-L1 expression was additionally assessed in these subpopulations of monocytes.
Regardless of their PD-L1 expression, non-classical monocytes were significantly higher at baseline in patients with long PFS and the patients with high frequencies of these cells had significantly better MOS (Supplementary Figure 12). No differences regarding PFS or OS at the different timepoints were observed in the other monocytic subsets (Supplementary Table 3, Supplementary figure 3).
After paired analysis, the intermediate monocyte subset showed a significantly higher frequency of PD-L1+ cells than the classical or non-classical monocyte subsets (). PD-L1 expression was also evaluated in CD14+HLA-DRlow/- MoMDSCs and CD14+HLA-DR+, showing that the former population is comprised of a significantly lower frequency of PD-L1+ cells than the latter ().
Figure 7. PD-L1 expression in the myeloid compartment as a predictive marker. Frequencies of PD-L1+ cells in monocytes classified according to their CD14/CD16 (a) and HLA-DR (b) expression. (c–h) PFS and Kaplan-Meier survival analysis of the frequencies of PD-L1+ cells in intermediate monocytes (c-d), non-classical monocytes (e–f) and MoMDSCs (g–h). Each dot represents an individual patient, dashed lines represent the cutoff point that divides each parameter into high and low as calculated using Cutoff Finder software; mean ±95% CI are represented. (a) Friedman test followed by Dunn’s multiple comparison. (b) Wilcoxon matched-pairs signed rank test. (c and g) Unpaired t-test. (e) Unpaired Mann-Whitney U test *, P < .05; **, P < . 01; ****, P < .0001.
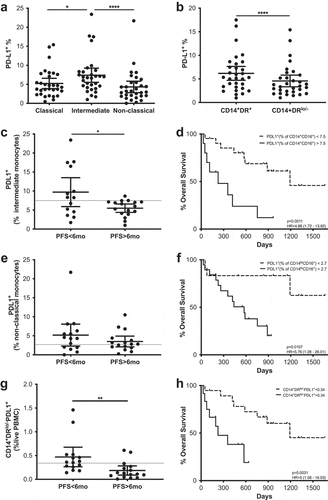
In addition to comparing PD-L1 expression levels between different subsets, we also analyzed the possible correlations with PFS and MOS considering two different approaches (). On one hand, the relevance of a given PD-L1+ subset was assessed as the frequency of the total live PBMC population. On the other hand, the frequency of PD-L1+ cells was evaluated within each subset. A significant survival advantage was correlated with lower frequencies of PD-L1+ cells within the intermediate and non-classical monocyte populations at baseline (). Likewise, PD-L1+ frequency in intermediate monocytes was significantly higher in patients with short PFS when compared to long PFS patients (), showing statistically significant predictive value (OR 0.79; p = .0181; ROC AUC = 0.66; 88.2% specificity; 57.1% sensitivity) (Supplementary figure 6, Supplementary table 3). Similar results were observed when the frequency of PD-L1+ MoMDSCs within the total PBMC population was evaluated: Patients with low frequencies of PD-L1+MoMDSCs had significantly higher MOS, and patients with long PFS have significantly lower frequencies of these potentially suppressive cells (). ROC analysis also confirmed the capacity of this cellular population to predict PFS (OR 0.0026; p = .0011; ROC AUC = 0.83; 71.4% specificity; 88.2% sensitivity) (Supplementary figure 6, Supplementary table 3).
CD69+ NK cells and PD-L1+ intermediate monocytes as predictive biomarkers
To ascertain if any of the previously mentioned relevant cellular populations were correlated with each other at baseline, a Spearman rank correlation matrix analysis was carried out (Supplementary figure 13). CD69+NK cells showed strong correlations (|rs| >0.5) with PD-L1+ frequencies of intermediate monocytes (p = .0012) (). In order to evaluate the correlation with PFS and MOS, patients were grouped in two categories according to the cutoff points previously established: patients who had low frequencies of CD69+NK cells and low frequencies of PD-L1+ intermediate monocytes and patients who had high frequencies of any of the cell types included in the analysis (). A significant survival advantage was associated with the low CD69+ and low PD-L1+ groups. Similar results were observed when correlation between CD69+NK cells and low frequencies of PD-L1+ non-classical monocytes was analyzed (). Interestingly, only the combination of CD69+NK cells and PD-L1+ intermediate monocytes presented a significant predictive value for PFS (OR 0.0833; p = .0038; 80% specificity; 75% sensitivity), whilst the combination of CD69+NK cells and PD-L1+ non-classical monocytes did not show predictive value (OR 0.4286; p = .4578; 44% specificity; 75% sensitivity).
Figure 8. CD69+ NK cells and PD-L1+ as a combined predictive biomarker. (a,c) Spearman correlation between CD69+ NK cells and PD-L1+ intermediate monocytes (a) or PD-L1+ non-classical monocytes (c). Each dot represents an individual patient, dashed lines represent the cutoff point that divides each parameter into high and low as calculated using Cutoff Finder software. (b,d) Kaplan-Meier survival analysis of the combined populations: CD69+ NK cells and PD-L1+ intermediate monocytes (b); CD69+ NK cells and PD-L1+ non-classical monocytes (d).
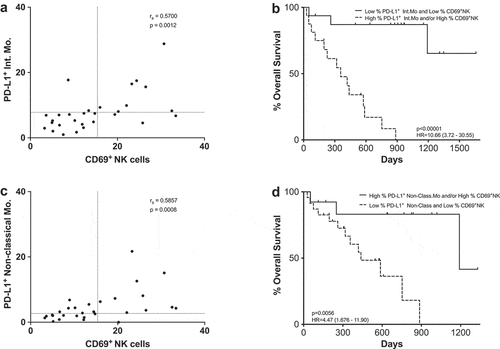
When these correlations were analyzed taking into account the frequency of CD69+ cells within the CD56dimNK cell subset similar results were observed (Supplementary Figure 14).
Discussion
In this exploratory study, we carried out extensive immune monitoring in advanced melanoma patients before and during treatment with the PD-1 blocking antibodies pembrolizumab or nivolumab. Median overall survival (886 d), objective response rate (50%) and incidence of adverse events are in agreement with previously reported data.Citation3 In order to provide a reference for outcome evaluation, patients were subdivided into two groups, according to their PFS. This classification proved to be strongly correlated with overall response rate and clinical benefit, since the group that included patients with PFS times longer than 6 months has a significantly longer MOS and included 17 of the 18 patients with an objective response, 1 patient classified as mixed response and 2 stable disease patients.
Routine hematological evaluation is one of the ideal sources for predictive and prognostic biomarker candidates due to its lack of intrusiveness, low cost and basic infrastructural requirements. When we evaluated the routine bloodwork in our patient cohort, four parameters at baseline were correlated with OS: LDH activity, leukocyte counts, neutrophil counts and neutrophil-to-lymphocyte ratio.
LDH activity has already been described as a predictor of survival in melanoma and correlated with tumor burden, OS and responses to ipilimumab and pembrolizumab.Citation2,Citation13,Citation14 In the present study, LDH levels were correlated with overall survival, but were not found to be significantly higher in patients with short PFS times.
Multivariate analysis models showed that patients in our cohort with V600E mutated BRAF could be correlated with better PFS and OS, when the p(corr)[1]/VIP plots were considered, although significance was not observed in the loadings plot 95% CI. Association of BRAF mutations with responses to PD-1 blockade is still a controversial issue, with contradictory results in different studies.Citation15–Citation17 Another marker associated with long PFS was the occurrence of any-grade treatment-related adverse events, confirming the results of previous studies.Citation18–Citation20
In the circulating cell populations, inverse correlations with OS were observed with regards to leukocytes and neutrophils which were also inversely correlated with PFS. The predictive value of neutrophil counts with regards to outcome was further confirmed by the high specificity and statistically significant odds ratio observed after ROC analysis. It is interesting to note that the cutoff points as determined by the cutoff analysis software are reasonably close to the reference values used in clinical routine at Karolinska University Hospital (Supplementary Table 4). An additional hematological value that is currently being considered as a potential biomarker for CTLA-4 and PD-1 checkpoint blockade in melanoma as well as in other malignancies is the neutrophil to lymphocyte ratio (NLR).Citation21–Citation26 In the discovery cohort pertaining to this study, we observed a correlation between high neutrophil to lymphocyte ratio (NLR) and survival, with similar cutoff points as those described previously.Citation27
Flow cytometric analysis revealed that, in this patient cohort, activated NK cells inversely correlate with OS and, in the case of the early activation marker CD69, may also hold predictive value for PFS. While initially unexpected, the explanation for these results may lie within the possible immunosurveillance role that has been attributed to NK cells in controlling metastatic disease.Citation28 In this scenario, higher frequencies of activated NK cells in circulation would be a consequence of high disease burden. An alternative explanation to these results could rely on the fact that excessive activation may indicate exhaustion or impair the functional potential of circulating NK cells. These hypotheses should be further explored. Interestingly, a recent study has shown that anti-PD-1 responding melanoma patients had higher frequencies of CD69+NK cells after in vitro stimulation with PMA-ionomycin.Citation29 There are two matters to take into consideration when assessing these data: the use of cryopreserved PBMCs and the fact that an in vitro stimulation was carried out. Previous studies carried out by our group and others have shown that freeze-thawing affects the viability and the expression of different surface markers on myeloid and lymphoid cells.Citation2,Citation30-Citation34 Additional studies have suggested not only that cryopreservation may affect CD69 expression in NK cells,Citation35 but also that additives present in freezing media like DMSO decrease CD69 expression in CD4+ T cells.Citation36 On the other hand, the fact that responders showed an increased frequency of CD69+ NK cells after stimulation does not necessarily disagree with our results since an increase in the frequency of CD69+ NK cells was observed after treatment in patients with long PFS. In the patient cohort included in this study, this increase in CD69+ NK cells may probably be a result of the immune stimulation in response to the PD-1 blockade treatment.
In order to ascertain additional functional implications of CD69 expression in NK cells, the different CD56 dim and bright NK cell subsets were analyzed. CD56dim NK cells are the more abundant subset in circulation and are considered to be the ones responsible for cytotoxic activity.Citation37 At all timepoints the frequency of CD69 expression in this subset was inversely correlated with survival, indicating that it is these cytotoxic, circulating CD69+ NK cells that are correlated with response and overall survival.
Our results contradict those reported by Fregui et al.,Citation35 where high frequencies of NKp46+ NK cells were directly correlated with survival in patients with stage IV melanoma. In line with our data, a recent study reported that NKp46+NK cells were inversely correlated with survival in NSCLC.Citation38 Adotévi and collaborators highlight the possible regulatory role that soluble factors produced by NKp46+NK cells may have in the expansion and activation of T cells, as has been previously suggested.Citation39,Citation40
Besides NK cells, different subsets within the myeloid compartment were observed to be correlated with responses to PD-1 blockade. The fact that frequencies of MoMDSCs were lower in patients with longer PFS and were indicators of better OS is not surprising. MoMDSCs in melanoma have been consistently associated with high tumor burden, bad prognosis, and lower response rates after ipilimumab treatment,Citation2,Citation41-Citation43 but they had not yet been associated, to our knowledge, as prognostic markers for survival after monotherapy with pembrolizumab or nivolumab in patients with advanced melanoma. A more interesting conclusion in this population was the fact that if the frequency of PD-L1+ MoMDSCs was used in survival analysis, significance was increased as well as the differences between the short and long PFS subgroups. The prognostic potential of this cellular population was consolidated after ROC analysis and suggests that the expression of PD-L1 could be essential in the suppressive potential of MoMDSCs. Coupled with the MoMDSC data, equivalent results were observed in the CD14+HLA-DR+ subset, since these cells were positively correlated with treatment outcome while the frequency of PD-L1+ cells within them was found to have a negative prognostic value. It is interesting to note that PD-L1 expression levels were significantly higher in CD14+HLA-DR+ cells than in CD14+HLA-DRlo/- MoMDSCs. Although initially these results might seem counterintuitive, they are not unexpected, since both HLA-DR and PD-L1 are upregulated via partially overlapping pathways that are related to an inflammatory response mediated, among others, by IFN-γ.Citation44–Citation47
Non-classical monocytes have been well characterized during inflammatory processesCitation48,Citation49 and are known to secrete TNF-α after stimulation with LPS.Citation49 Since they are considered immune-stimulating mature monocytes with ADCC capabilitiesCitation50,Citation51 their direct correlation with PFS and OS in this patient cohort is easily understood. Furthermore, PD-L1 expression on their surface could potentially hamper their immune-activating function and therefore explain their correlation with shorter survival times.
In the present patient cohort, PD-L1 expression was also significantly higher in the intermediate monocyte subset. These cells are known to express high levels of HLA-DR, DP, DQ and DM,Citation52 and are active antigen presenters, leading to the conclusion that high frequencies of PD-L1+ intermediate monocytes may actively suppress T cell activation during antigen presentation. Additionally, intermediate monocytes have been described as anti-inflammatory monocytes given that they produce IL-10 after stimulation with LPS.Citation50 The observation that their PD-L1 expression levels are strongly correlated with PFS and OS indicates that intermediate monocytes may play a decisive role in the modulation of the anti-melanoma immune response after PD-1 checkpoint blockade. This possibility is further consolidated when the correlation between CD69+ NK cells and PD-L1+ intermediate monocytes was analyzed. MOS in the patient group with low frequencies of both populations was undefined, resulting in the highest hazard ratio and lowest p values in our study. These results are in agreement with a recent report where tumor infiltrating NK cells upregulate CD73+ and undergo transcriptional reprogramming which leads to an increased IL-10 and TGF-β production.Citation53 Understanding the relationship between NK cell activation status, these immunosuppressive cytokines, and the expansion of MoMDSCs could be a key component in antitumoral immunity.
Our results suggest that, although T cells are the main target of PD-1 blockade therapy, the innate component of the immune system contains cellular populations that are possible predictive biomarkers. Analyzing non-cryopreserved samples has allowed us to measure certain surface markers in a more precise manner, resulting in our finding of different subsets of NK cells and monocytes as predictors of PFS and OS in patients treated with anti-PD-1 antibodies. Both NK cells and monocytes can be critical for a successful therapeutic outcome, and their detailed roles should be further evaluated.
Materials and methods
Patients
Patients with metastasized malignant melanoma receiving anti-PD1 treatment at the Department of Oncology, Karolinska University Hospital, Stockholm, Sweden were eligible to participate in this study. The protocol was approved by the local Ethics Committee and the Institutional Review Board and all patients provided written informed consent in accordance with the Declaration of Helsinki.
All patients had stage IV malignant melanoma (cutaneous or unknown primary), the most frequent metastatic category was M1 c (defined according to American Joint Committee on Cancer, AJCC), Eastern Cooperative Oncology Group (ECOG) performance status score of 0–1 and were previously treatment naïve or had received up to two previous systemic treatments. Detailed patient information can be found in Supplementary Table 1.
Ten patients were treated with pembrolizumab every 3 weeks, 24 patients with nivolumab every other week and 2 patients were treated with nivolumab every 4 weeks, all three regimens were given according to clinical routine.
According to local clinical routine, the patients provided blood samples including complete blood count, electrolytes, creatinine, liver status, thyroid status before every cycle and those along with the clinical general condition of the patients and any adverse events were assessed before each new cycle was administered. About 3 months after the first cycle, most patients underwent the first computed tomography (CT) scan for response evaluation. Thereafter, CT was performed about every 3 months depending on the outcome and depending on the stability of the disease response. The radiological assessments were performed by the local radiological department. The radiological and response evaluation was not made strictly according to immune-related Response Criteria, irRC. Response evaluation was made by the responsible clinician, weighing together the clinical outcome and the radiological response. Follow up time was defined as the time from first cycle to death from any cause, or to last known contact with the patient. Progression-free survival (PFS) was defined as the time from first cycle to first documented progressive disease, death from any cause, or last known contact, whichever occurred first.
The severity of adverse events was graded 0–5 according to the National Cancer Institute Common Terminology Criteria for Adverse Events version (CTCAE) 4.0.
Peripheral blood samples
Peripheral blood samples were obtained at three timepoints during treatment. The first sample (baseline) was obtained before treatment started; the second sample (timepoint 1) was obtained immediately before the second infusion of pembrolizumab or nivolumab, the third one was obtained immediately before the fourth infusion (timepoint 2). Counts of leukocytes, neutrophils, lymphocytes and eosinophils were measured as per Karolinska Hospital guidelines and are expressed as cells/l.
Peripheral blood mononuclear cells (PBMCs) were purified from the blood samples by ficoll density gradient centrifugation within 1 h of sample collection (Ficoll-Paque plus, GE Healthcare Life Sciences). Purified PBMCs were stained immediately and the remaining cells were cryopreserved in fetal calf serum with 10% DMSO.
Antibodies and flow cytometry
PBMCs were stained immediately as previously described.Citation2,Citation11 Antibody details are provided in Supplementary Table 5. Dead cells were excluded with the LIVE/DEAD® Fixable Aqua Dead Cell Stain Kit (Thermo Fisher Scientific). Cells were analyzed using an LSRII flow cytometer (BD Biosciences) and FlowJo v10.7 software (Treestar, Ashland, OR), using a non-stained control for each sample and fluorescence-minus-one (FMO) controls for critical stainings. The gating strategy used for each of the populations analyzed in this study is shown in Supplementary Figures 5 and 11. Quality control of the flow cytometer’s performance and CV values were monitored on a day-to-day basis using CS&T beads (BD Biosciences).
Multivariate statistical analysis
Multivariate analysis was carried out by applying orthogonal projections to latent structures discriminant analysis (OPLS-DA) using SIMCA version 15.02. OPLS-DA is a supervised method developed from principal component analysis to separate unrelated noise from the predictive variables of interest.Citation28,Citation54,Citation55 The discriminant binary variables used were PFS (short vs. long, cutoff at 6 months) and OS (short vs. long, cutoff at 18 months). All multivariate models were calculated using unit variance scaled data that was log10 transformed when applicable. Model quality is reported as the cumulative correlation coefficients (R2), its predictive performance is based on 7-fold cross-validation (Q2) and cross-validated ANOVA (CV-ANOVA) P values are also obtained, providing a reference for assessing the reliability of the model.
In order to choose candidate biomarkers, each variable’s modeled co-variation, calculated as model loadings ±95% confidence intervals (CI) (p[1]), its modeled correlation, calculated as scaled loadings (p(corr)[1]) and the variable importance in the projections (VIP) were used. The jack-knifed CI of the loadings plot were used as the initial criterium for parameter selection. Subsequently, biomarkers with VIP values >1.0, and |p(corr[1])|>0.4, were selected for validation by univariate analysis.Citation56
Univariate statistical analysis
Data are shown as mean with 95% confidence interval for each time point. All data sets were checked for normality (Shapiro Wilk test) and variance homogeneity (Bartlett’s test).
Two-tailed T-test with Welch’s correction and ANOVA followed by Holm-Sidak’s multiple comparison test were employed. If non-parametric analysis was deemed necessary, the tests used were the Mann-Whitney test (two-tailed) or Kruskal Wallis ANOVA followed by Dunn’s multiple comparison test. When paired analysis was possible, the Wilcoxon matched-pairs signed ranks test or the Friedman test followed by Dunn’s multiple comparison test were utilized.
Overall Survival (OS) was defined as the interval between treatment start to the time of death or last follow up. Survival probabilities were determined by Kaplan-Meier analysis and curve comparison was analyzed by log rank tests. All Kaplan-Meier curves depict overall survival.
Median values within each population were initially used as cutoff points for survival analysis. In populations of interest, cutoff points were further refined using Cutoff Finder software.Citation57 In these cases, statistical significance was calculated with Bonferroni correction according to the number of comparison tests determined by the software. Receiver Operating Characteristic (ROC) curve analysis was carried out using the cutoff points determined using Cutoff Finder software. Spearman’s non-parametric test was used for correlation analysis.
All statistical analyses were conducted using GraphPad Prism version 8.00 for Windows (GraphPad Software) and IBM SPSS Statistics v23 (IBM corporation). Differences were considered significant when p ≤ 0.05.
Study approval
The protocol was approved by the local Ethics Committee and the Institutional Review Board at Karolinska Institute and all patients provided written informed consent in accordance with the Declaration of Helsinki.
Authors’ contributions
YPC designed and performed experiments, analyzed data and wrote the paper; MW recruited patients, assisted in data analysis, provided clinical care, collected clinical information and collaborated in manuscript preparation; IHA processed patient samples, assisted in data analysis and collaborated in manuscript preparation; TN processed patient samples and collaborated in manuscript preparation; SR processed patient samples; AL collaborated in manuscript preparation; JH and GM recruited patients, provided clinical care and collaborated in manuscript preparation; RK supervised all aspects. All authors revised and commented the manuscript.
Disclosure of potential conflicts of interest
No potential conflicts of interest were disclosed.
Supplemental Material
Download ()Acknowledgments
The authors would like to thank Karl-Johan Ekdahl for his assistance in sample collection, Christian Oertlin, Ulrika Edbäck, Ernesto López, Yuan Yang, Disha Rao, Emma Houlder and Fríða B. Gunnarsdóttir for collaborating in several aspects of this work.
Supplementary material
Supplemental data for this article can be accessed on the publisher’s website.
Additional information
Funding
References
- Pico de Coaña Y, Choudhury A, Kiessling R. Checkpoint blockade for cancer therapy: revitalizing a suppressed immune system. Trends Mol Med. 2015;21(8):482–14. doi:10.1016/j.molmed.2015.05.005.
- Pico de Coaña Y, Wolodarski M, Poschke I, Yoshimoto Y, Yang Y, Nystrom M, Edback U, Brage SE, Lundqvist A, Masucci GV, et al. Ipilimumab treatment decreases monocytic MDSCs and increases CD8 effector memory T cells in long-term survivors with advanced melanoma. Oncotarget. 2017;8(13):21539–21553. doi:10.18632/oncotarget.15368.
- Weiss SA, Wolchok JD, Sznol M. Immunotherapy of melanoma: facts and hopes. Clin Cancer Res. 2019 Mar 28. doi:10.1158/1078-0432.CCR-18-1550.
- Seo H, Jeon I, Kim B-S, Park M, Bae E-A, Song B, Koh C-H, Shin K-S, Kim I-K, Choi K, et al. IL-21-mediated reversal of NK cell exhaustion facilitates anti-tumour immunity in MHC class I-deficient tumours. Nat Commun. 2017;8:15776. doi:10.1038/ncomms15776.
- Park IH, Yang HN, Lee KJ, Kim T-S, Lee ES, Jung S-Y, Kwon Y, Kong S-Y, Hae Park I, Na Yang H, et al. Tumor-derived IL-18 induces PD-1 expression on immunosuppressive NK cells in triple-negative breast cancer. Oncotarget. 2017;8(20):32722–32730. doi:10.18632/oncotarget.16281.
- Poschke I, Kiessling R. On the armament and appearances of human myeloid-derived suppressor cells. Clin Immunol. 2012;144(3):250–268. doi:10.1016/j.clim.2012.06.003.
- Groth C, Hu X, Weber R, Fleming V, Altevogt P, Utikal J, Umansky V. Immunosuppression mediated by myeloid-derived suppressor cells (MDSCs) during tumour progression. Br J Cancer. 2019;120(1):16–25. doi:10.1038/s41416-018-0333-1.
- Umansky V, Adema GJGJ, Baran J, Brandau S, van Ginderachter JAJA, Hu X, Jablonska J, Mojsilovic S, Papadaki HAHA, Pico de Coaña Y, et al. Interactions among myeloid regulatory cells in cancer. Cancer Immunol Immunother. 2019;68(4):645–660. doi:10.1007/s00262-018-2200-6.
- Poschke I, Mougiakakos D, Hansson J, Masucci GV, Kiessling R. Immature immunosuppressive CD14+HLA-DR-/low cells in melanoma patients are Stat3hi and overexpress CD80, CD83, and DC-sign. Cancer Res. 2010;70(11):4335–4345. doi:10.1158/0008-5472.CAN-09-3767.
- Fleming V, Hu X, Weber R, Nagibin V, Groth C, Altevogt P, Utikal J, Umansky V. Targeting myeloid-derived suppressor cells to bypass tumor-induced immunosuppression. Front Immunol. 2018;9:398. doi:10.3389/fimmu.2018.00398.
- Pico de Coaña Y, Poschke I, Gentilcore G, Mao Y, Nystrom M, Hansson J, Masucci GV, Kiessling R. Ipilimumab treatment results in an early decrease in the frequency of circulating granulocytic myeloid-derived suppressor cells as well as their Arginase1 production. Cancer Immunol Res. 2013;1(3):158–162. doi:10.1158/2326-6066.CIR-13-0016.
- Ziegler-Heitbrock L, Ancuta P, Crowe S, Dalod M, Grau V, Hart DN, Leenen PJM, Liu YJ, MacPherson G, Randolph GJ, et al. Nomenclature of monocytes and dendritic cells in blood. Blood. 2010;116:16. doi:10.1182/blood-2010-02-258558.
- Gershenwald JE, Scolyer RA, Hess KR, Sondak VK, Long GV, Ross MI, Lazar AJ, Faries MB, Kirkwood JM, McArthur GA, et al. Melanoma staging: evidence-based changes in the American Joint Committee on Cancer eighth edition cancer staging manual. CA Cancer J Clin. 2017;67(6):472–492. doi:10.3322/caac.21409.
- Martens A, Wistuba-Hamprecht K, Foppen MG, Yuan J, Postow MA, Wong P, Romano E, Khammari A, Dreno B, Capone M, et al. Baseline peripheral blood biomarkers associated with clinical outcome of advanced melanoma patients treated with ipilimumab. Clin Cancer Res. 2016;22(12):2908–2918. doi:10.1158/1078-0432.CCR-15-2412.
- Larkin J, Lao CD, Urba WJ, McDermott DF, Horak C, Jiang J, Wolchok JD. Efficacy and safety of nivolumab in patients with BRAF V600 mutant and BRAF wild-type advanced melanoma: a pooled analysis of 4 clinical trials. JAMA Oncol. 2015;1(4):433–440. doi:10.1001/jamaoncol.2015.1184.
- Wolchok JD, Chiarion-Sileni V, Gonzalez R, Rutkowski P, Grob -J-J, Cowey CL, Lao CD, Wagstaff J, Schadendorf D, Ferrucci PF, et al. Overall survival with combined nivolumab and ipilimumab in advanced melanoma. N Engl J Med. 2017;377(14):1345–1356. doi:10.1056/NEJMoa1709684.
- Jessurun CAC, Vos JAM, Limpens J, Luiten RM. Biomarkers for response of melanoma patients to immune checkpoint inhibitors: a systematic review. Front Oncol. 2017;7(Sep). doi:10.3389/fonc.2017.00233.
- Teraoka S, Fujimoto D, Morimoto T, Kawachi H, Ito M, Sato Y, Nagata K, Nakagawa A, Otsuka K, Uehara K, et al. Early immune-related adverse events and association with outcome in advanced non–small cell lung cancer patients treated with nivolumab: a prospective cohort study. J Thorac Oncol. 2017;12(12):1798–1805. doi:10.1016/j.jtho.2017.08.022.
- Indini A, Di Guardo L, Cimminiello C, Prisciandaro M, Randon G, De Braud F, Del Vecchio M. Immune-related adverse events correlate with improved survival in patients undergoing anti-PD1 immunotherapy for metastatic melanoma. J Cancer Res Clin Oncol. 2019;145(2):511–521. doi:10.1007/s00432-018-2819-x.
- Okada N, Kawazoe H, Takechi K, Matsudate Y, Utsunomiya R, Zamami Y, Goda M, Imanishi M, Chuma M, Hidaka N, et al. Association between immune-related adverse events and clinical efficacy in patients with melanoma treated with nivolumab: a multicenter retrospective study. Clin Ther. 2019;41(1):59–67. doi:10.1016/j.clinthera.2018.11.004.
- Takahashi H, Sakakura K, Tada H, Kaira K, Oyama T, Chikamatsu K. Prognostic significance and population dynamics of peripheral monocytes in patients with oropharyngeal squamous cell carcinoma. Head & Neck. 2019;41(6):1880–1888. doi:10.1002/hed.25625
- Ascierto PA, Capone M, Grimaldi AM, Mallardo D, Simeone E, Madonna G, Roder H, Meyer K, Asmellash S, Oliveira C, et al. Proteomic test for anti-PD-1 checkpoint blockade treatment of metastatic melanoma with and without BRAF mutations. J Immunother Cancer. 2019;7(1):91. doi:10.1186/s40425-019-0569-1.
- Jiang T, Bai Y, Zhou F, Li W, Gao G, Su C, Ren S, Chen X, Zhou C. Clinical value of neutrophil-to-lymphocyte ratio in patients with non-small-cell lung cancer treated with PD-1/PD-L1 inhibitors. Lung Cancer. 2019;130:76–83. doi:10.1016/j.lungcan.2019.02.009.
- Ma J, Kuzman J, Ray A, Lawson BO, Khong B, Xuan S, Hahn AW, Khong HT. Neutrophil-to-lymphocyte ratio (NLR) as a predictor for recurrence in patients with stage III melanoma. Sci Rep. 2018;8(1):4044. doi:10.1038/s41598-018-22425-3.
- Weide B, Martens A, Zelba H, Stutz C, Derhovanessian E, Di Giacomo AM, Maio M, Sucker A, Schilling B, Schadendorf D, et al. Myeloid-derived suppressor cells predict survival of patients with advanced melanoma: comparison with regulatory T cells and NY-ESO-1- or melan-A-specific T cells. Clin Cancer Res. 2014;20(6):1601–1609. doi:10.1158/1078-0432.CCR-13-2508.
- Forget P, Khalifa C, Defour JP, Latinne D, Van Pel MC, De Kock M. What is the normal value of the neutrophil-to-lymphocyte ratio? BMC Res Notes. 2017;10(1):1–4. doi:10.1186/s13104-016-2335-5.
- Nakamura Y, Tanaka R, Maruyama H, Ishitsuka Y, Okiyama N, Watanabe R, Fujimoto M, Fujisawa Y. Correlation between blood cell count and outcome of melanoma patients treated with anti-PD-1 antibodies. Jpn J Clin Oncol. 2019;49(5):431–437. doi:10.1093/jjco/hyy201.
- Tallerico R, Cristiani CM, Staaf E, Garofalo C, Sottile R, Capone M, Pico de Coaña Y, Madonna G, Palella E, Wolodarski M, et al. IL-15, TIM-3 and NK cells subsets predict responsiveness to anti-CTLA-4 treatment in melanoma patients. Oncoimmunology. 2017;6(2). doi:10.1080/2162402X.2016.1261242.
- Subrahmanyam PB, Dong Z, Gusenleitner D, Giobbie-Hurder A, Severgnini M, Zhou J, Manos M, Eastman LM, Maecker HT, Hodi FS, et al. Distinct predictive biomarker candidates for response to anti-CTLA-4 and anti-PD-1 immunotherapy in melanoma patients. J Immunother Cancer. 2018;6(1):18. doi:10.1186/s40425-018-0328-8.
- Sattui S, de la Flor C, Sanchez C, Lewis D, Lopez G, Rizo-Patron E, White AC Jr, Montes M. Cryopreservation modulates the detection of regulatory T cell markers. Cytom B Clin Cytom. 2012;82(1):54–58. doi:10.1002/cyto.b.20621.
- Florek M, Schneidawind D, Pierini A, Baker J, Armstrong R, Pan Y, Leveson-Gower D, Negrin R, Meyer E. Freeze and thaw of CD4+CD25+Foxp3+ regulatory T cells results in loss of CD62L expression and a reduced capacity to protect against graft-versus-host disease. PLoS One. 2015;10(12):e0145763. doi:10.1371/journal.pone.0145763.
- Grutzner E, Stirner R, Arenz L, Athanasoulia AP, Schrodl K, Berking C, Bogner JR, Draenert R. Kinetics of human myeloid-derived suppressor cells after blood draw. J Transl Med. 2016;14(1):2. doi:10.1186/s12967-015-0755-y.
- Kotsakis A, Harasymczuk M, Schilling B, Georgoulias V, Argiris A, Whiteside TL. Myeloid-derived suppressor cell measurements in fresh and cryopreserved blood samples. J Immunol Methods. 2012;381(1–2):14–22. doi:10.1016/j.jim.2012.04.004.
- Trellakis S, Bruderek K, Hutte J, Elian M, Hoffmann TK, Lang S, Brandau S. Granulocytic myeloid-derived suppressor cells are cryosensitive and their frequency does not correlate with serum concentrations of colony-stimulating factors in head and neck cancer. Innate Immun. 2013;19(3):328–336. doi:10.1177/1753425912463618.
- Fregni G, Messaoudene M, Fourmentraux-Neves E, Mazouz-Dorval S, Chanal J, Maubec E, Marinho E, Scheer-Senyarich I, Cremer I, Avril M-F, et al. Phenotypic and functional characteristics of blood natural killer cells from melanoma patients at different clinical stages Dieli F, editor. PLoS One. 2013;8(10):e76928. doi:10.1371/journal.pone.0076928.
- Holthaus L, Lamp D, Gavrisan A, Sharma V, Ziegler A-G, Jastroch M, Bonifacio E. CD4+ T cell activation, function, and metabolism are inhibited by low concentrations of DMSO. J Immunol Methods. 2018;463:54–60. doi:10.1016/J.JIM.2018.09.004.
- Fauriat C, Long EO, Ljunggren HG, Bryceson YT. Regulation of human NK-cell cytokine and chemokine production by target cell recognition. Blood. 2010;115(11):2167–2176. doi:10.1182/blood-2009-08-238469.
- Picard E, Godet Y, Laheurte C, Dosset M, Galaine J, Beziaud L, Loyon R, Boullerot L, Lauret Marie Joseph E, Spehner L, et al. Circulating NKp46 + natural killer cells have a potential regulatory property and predict distinct survival in non-small cell lung cancer. Oncoimmunology. 2019;8(2):1–13. doi:10.1080/2162402X.2018.1527498.
- da Silva IP, Gallois A, Jimenez-Baranda S, Khan S, Anderson AC, Kuchroo VK, Osman I, Bhardwaj N. Reversal of NK-cell exhaustion in advanced melanoma by Tim-3 blockade. Cancer Immunol Res. 2014;2(5):410–422. doi:10.1158/2326-6066.CIR-13-0171.
- Loyon R, Picard E, Mauvais O, Queiroz L, Mougey V, Pallandre J-R, Galaine J, Mercier-Letondal P, Kellerman G, Chaput N, et al. IL-21–induced MHC class II + NK cells promote the expansion of human uncommitted CD4 + central memory T cells in a macrophage migration inhibitory factor–dependent manner. J Immunol. 2016;197(1):85–96. doi:10.4049/jimmunol.1501147.
- Mao Y, Poschke I, Wennerberg E, Pico de Coaña Y, Brage SESE, Schultz I, Hansson J, Masucci G, Lundqvist A, Kiessling R, et al. Melanoma-educated CD14+ cells acquire a myeloid-derived suppressor cell phenotype through COX-2-dependent mechanisms. Cancer Res. 2013;73(13):3877–3887. doi:10.1158/0008-5472.CAN-12-4115.
- Meyer C, Cagnon L, Costa-Nunes CM, Baumgaertner P, Montandon N, Leyvraz L, Michielin O, Romano E, Speiser DE. Frequencies of circulating MDSC correlate with clinical outcome of melanoma patients treated with ipilimumab. Cancer Immunol Immunother. 2014;63(3):247–257. doi:10.1007/s00262-013-1508-5.
- Pico de Coaña Y, Masucci G, Hansson J, Kiessling R. Myeloid-derived suppressor cells and their role in CTLA-4 blockade therapy. Cancer Immunol Immunother. 2014;63(9):977–983. doi:10.1007/s00262-014-1570-7.
- Laupéze B, Fardel O, Onno M, Bertho N, Drénou B, Fauchet R, Amiot L. Differential expression of major histocompatibility complex class Ia, Ib and II molecules on monocytes and monocyte-derived dendritic and macrophagic cells. Hum Immunol. 1999;60(7):591–597. doi:10.1016/S0198-8859(99)00025-7.
- Hornell TMC, Beresford GW, Bushey A, Boss JM, Mellins ED. Regulation of the class II MHC pathway in primary human monocytes by granulocyte-macrophage colony-stimulating factor. J Immunol. 2003;171(5):2374–2383. doi:10.4049/jimmunol.171.5.2374.
- Voskamp AL, Prickett SR, Mackay F, Rolland JM, O’Hehir RE, Class MHC. II expression in human basophils: induction and lack of functional significance Ryffel B, editor. PLoS One. 2013;8(12):e81777. doi:10.1371/journal.pone.0081777.
- Chen S, Crabill GA, Pritchard TS, McMiller TL, Wei P, Pardoll DM, Pan F, Topalian SL. Mechanisms regulating PD-L1 expression on tumor and immune cells. J Immunother Cancer. 2019;7(1). doi:10.1186/s40425-019-0770-2.
- Ziegler-Heitbrock L. The CD14+ CD16+ blood monocytes: their role in infection and inflammation. J Leukoc Biol. 2007;81(3):584–592. doi:10.1189/jlb.0806510.
- Belge K-U, Dayyani F, Horelt A, Siedlar M, Frankenberger M, Frankenberger B, Espevik T, Ziegler-Heitbrock L. The proinflammatory CD14+CD16+DR++ monocytes are a major source of TNF. J Immunol. 2002;168(7):3536–3542. doi:10.4049/jimmunol.168.7.3536.
- Skrzeczyńska-Moncznik J, Bzowska M, Loseke S, Grage-Griebenow E, Zembala M, Pryjma J. Peripheral blood CD14high CD16+ monocytes are main producers of IL-10. Scand J Immunol. 2008;67(2):152–159. doi:10.1111/j.1365-3083.2007.02051.x.
- Romano E, Kusio-Kobialka M, Foukas PG, Baumgaertner P, Meyer C, Ballabeni P, Michielin O, Weide B, Romero P, Speiser DE. Ipilimumab-dependent cell-mediated cytotoxicity of regulatory T cells ex vivo by nonclassical monocytes in melanoma patients. Proc Natl Acad Sci. 2015;112(19):6140–6145. doi:10.1073/pnas.1417320112.
- Lee J, Tam H, Adler L, Ilstad-Minnihan A, Macaubas C, Mellins ED. The MHC class II antigen presentation pathway in human monocytes differs by subset and is regulated by cytokines. PLoS One. 2017;12(8). doi:10.1371/journal.pone.0183594.
- Neo SY, Yang Y, Julien R, Ma R, Chen X, Chen Z, Tobin NP, Blake E, Seitz C, Thomas R, et al. CD73 immune checkpoint defines regulatory NK-cells within the tumor microenvironment. J Clin Invest. 2019 Nov 26. doi:10.1172/JCI128895.
- Trygg J, Wold S. Orthogonal projections to latent structures (O-PLS). J Chemom. 2002;16(3):119–128. doi:10.1002/cem.695.
- Dutta M, Subramani E, Taunk K, Gajbhiye A, Seal S, Pendharkar N, Dhali S, Ray CD, Lodh I, Chakravarty B, et al. Investigation of serum proteome alterations in human endometriosis. J Proteomics. 2015;114:182–196. doi:10.1016/J.JPROT.2014.10.021.
- Wheelock ÅM, Wheelock CE. Trials and tribulations of ‘omics data analysis: assessing quality of SIMCA-based multivariate models using examples from pulmonary medicine. Mol Biosyst. 2013;9(11):2589. doi:10.1039/c3mb70194h.
- Budczies J, Klauschen F, Sinn BV, Gyorffy B, Schmitt WD, Darb-Esfahani S, Denkert C. Cutoff Finder: a comprehensive and straightforward web application enabling rapid biomarker cutoff optimization. PLoS One. 2012;7(12):e51862. doi:10.1371/journal.pone.0051862.