ABSTRACT
Triple-negative breast cancer (TNBC) is characterized by broad genomic and transcriptional heterogeneity and results in a worse prognosis than other breast cancer types. Here, we integrated genomic and transcriptomic data combined with clinicopathologic information from 538 patients with TNBC and identified four novel significantly mutated genes (SMGs), namely, KDM6A, CD86, RBM47, and IFNGR1. A mutational signature (known as age-related signature 1) featured by enrichment of C > T mutations at NpCpG trinucleotides was associated with worse survival in TNBC (HR, 1.76 [95% CI, 1.07–2.90]; P = .025). We also analyzed gene transcriptomic profiles of TNBC samples and identified immune regulation-related gene pathways (e.g., antigen processing presentation and interferon signaling), and the cell cycle was significantly altered in samples with different signature 1 activity groups. The analysis further revealed that signature 1 was associated with decreased tumor immunogenicity and immunocyte infiltration in TNBC. This negative correlation was also observed in lung adenocarcinoma and prostate cancer samples. Furthermore, we found that patients with mutational signature 1 were markedly associated with decreased tumor mutation burden, even after controlling for age, grade, histological type, lymph node status, mutations in BRCA1/2 and ATR, and APOBEC and homologous recombination repair deficiency signatures (OR, 0.19 [95% CI, 0.11–0.32]; P < .001). Overall, this study identified previously unreported SMGs and re-annotated that age-related signature 1 was associated with a weakened immune microenvironment and predictive of poor survival in TNBC, offering opportunities to stratify patients into optimal treatment plans based on genomic subtyping.
Introduction
Triple-negative breast cancer (TNBC) accounts for 10–20% of breast carcinomas and is characterized by a lack of expression of the estrogen receptor and progesterone receptor and absence of ERBB2 (also known as HER2) amplification.Citation1,Citation2 TNBCs result in worse prognosis than other types of breast cancers (BCs), with an increased likelihood of early distant recurrence and death,Citation1 owing mainly to the extensive molecular heterogeneity of the disease.Citation3,Citation4
Recent next-generation sequencing has become a powerful tool to elucidate potential driver genetic aberrations underlying cancer development. TNBCs are significantly associated with BRCA1 germline mutations and high levels of genomic instability; TP53 and PIK3CA are the two most significantly mutated genes (SMGs).Citation5 Mutational signatures are the fingerprints of endogenous and exogenous factors that have acted over the course of tumorigenesis and heterogeneity. Age-related signature 1 was dominated by C > T mutation, which is associated with spontaneous deamination of 5-methylcytosine.Citation6 A recent study reported that younger Asian BCs harbor a more immune-active microenvironment than older Western BCs.Citation7 Signature 3, characterized by T > C mutations, was associated with a deficiency in genomic integrity maintenance (homologous recombination repair deficiency [HRD]) and strongly associated with BRCA1/2 mutations in breast cancer.Citation8
Clinical studies also found that patients with breast cancer with high immune infiltration, particularly TNBC and HER2-positive breast cancer, had better prognosis and treatment response.Citation9,Citation10 Recently, the correlation between the specific mutational signature process (e.g., HRD and APOBEC signatures) and antitumor immune activity has been observed across cancer types;Citation11-Citation13 however, the characteristics of immune infiltration and its association with mutational signatures in TNBC are still unclear. There is also evidence that immune-rich TNBCs may be under immune surveillance that continuously eliminates many immunogenic clones, resulting in lower clonal heterogeneity.Citation10
Since somatic mutations can be applied to clinical practice, the assessment of larger patient series is crucial in identifying tumor genomic aberrations that are potentially predictive responses and investigating whether patients have distinct clinical outcomes. Hence, the present study aimed to identify new SMGs and characterize mutational signatures that driving the molecular subtypes with genetic prognosticators and immune activity in patients with TNBC from published multi-omic studies. We believe that these findings may be applicable for prognostic prediction and therapeutic guidance for TNBC.
Results
SMGs in TNBC
Somatic mutational profiles of 538 patients with TNBC from previous whole-exome sequencing (WES) genomic studies were analyzed. A median of 118 mutations per sample (range, 1–2205) in a total of 93897 coding somatic mutations were collected from previously published studies. We combined MutSigCV and OncodriveFML algorithms to identify SMGs that met the criteria of positive accumulation, clustering at a hotspot, and functional importance. We identified a total of 16 SMGs in the pooled TNBC datasets (, Supplementary Table S1). Apart from well-known driver oncogenes (e.g., TP53, PIK3CA, FOXA1, KMT2 C/D, PTEN, RB1, BRCA1/2), we identified four new SMGs, namely, KDM6A (15 of 538, 2.8%), CD86 (8 of 538, 1.5%), RBM47 (7 of 538, 1.3%), and IFNGR1 (5 of 538, 0.9%), which were not previously implicated in TNBC. The mutation plots of these four novel SMGs are shown in Supplementary Figure S1.
Figure 1. Mutational landscape of significantly mutated genes in triple-negative breast cancer (TNBC) patients.
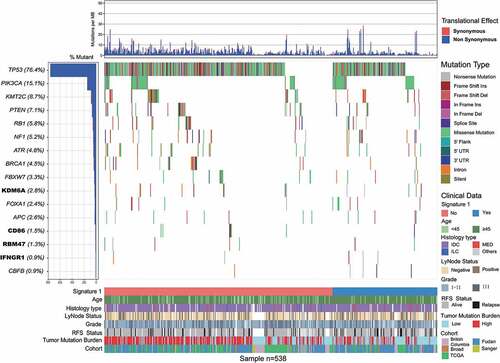
We also examined the mRNA expression levels of these four novel SMGs in tumor tissues versus matched adjacent normal control tissue in a separate microarray-based TNBC gene expression dataset.Citation14 The analyses showed that CD86 was significantly upregulated in tumor tissues, whereas KDM6A was significantly downregulated in tumor tissues (paired t-test, P < .05, Supplementary Figure S2). We also examined the association between SMG mutation status and survival prognosis. TNBC samples with RBM47 mutations were found to be associated with short survival outcomes in the aggregated TNBC cohort (log-rank test, P < .001; Supplementary Figure S3). A previous study also identified RBM47 as a suppressor of breast cancer progression and metastasis, and patients with low expression tended to have a poor clinical outcome.Citation15,Citation16
Mutational signatures operative in the aggregated TNBC cohort
To gain further insights into the mutational processes operative in patients with TNBC, we delineated the mutation signatures from the genomic mutational profile. We first counted the number of single-nucleotide variants in the matrix of 96 possible mutations occurring in a trinucleotide context in each TNBC sample and found that the predominant mutations were the C > T and C > G transitions at TpCpW trinucleotide sites (). Subsequently, we extracted three mutational signatures from the TNBC genomic data with varying mutational activities and annotated them against the Catalog of Somatic Mutations in Cancer (COSMIC) signature nomenclature (). Cosine similarity analysis demonstrated that the three signatures showed high similarity to known COSMIC signatures 1, 3, and 13 (cosine coefficients, 0.95, 0.91, and 0.86, respectively; Supplementary Figure S4). The proportion and activities of the three extracted signatures in every tumor sample (i.e., number of somatic mutations attribute to each signature) are presented in Supplementary Figure S5. We observed that signature 1 (mutational activity, 16270/82770, 19.4%) was dominated by C > T transitions at NpCpG trinucleotides (often termed CpG dinucleotides) that was associated with the age-related accumulation of spontaneous deamination of 5-methylcytosine in most cancer types. Signature 3, which was the most prevalent signature, accounting for 56899 in 82770 mutations (68.8%), was reported to have failure of DNA double-strand break repair by homologous recombination in breast, pancreatic, and ovarian cancers. Signature 13 (9601/82770, 11.8%), characterized by C > T mutations at TpCpW (where W = A or T) trinucleotide sequences, was attributed to the activity of the AID/APOBEC family of cytidine deaminases (APOBEC) in breast, bladder, cervical, and non-small cell lung cancers (NSCLCs)Citation17 ().
Figure 2. Mutational signatures extracted from the aggregated TNBC dataset.
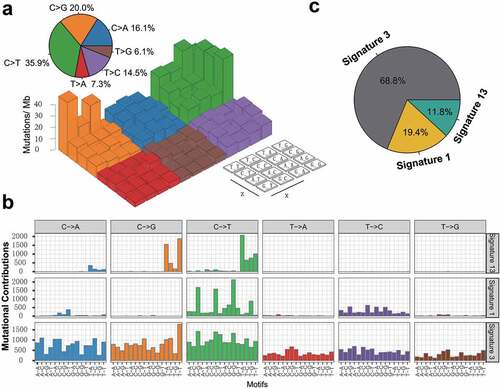
Mutational signature predictive of TNBC survival
To identify the extracted mutational signature activities associated with TNBC clinical features, we applied unsupervised hierarchical clustering for activities of signaturesCitation18 and identified two distinctive clusters, C1 and C2 (Supplementary Figure S6a). Survival analysis showed that their association with prognosis was marginally statistically significant (log-rank test, P = .075; Cox regression, HR, 0.57 [95% CI, 0.28–1.14]; Supplementary Figure S6b–c). Therefore, we followed the previously recommended method and criteria and stratified the extracted mutational signatures into binary variable (i.e., no and yes) model.Citation8,Citation19 We observed that patients with mutational signature 1 were markedly associated with worse survival outcomes (log-rank test, P = .025; HR, 1.76 [95% CI, 1.07–2.90]; ). To rule out the possibility that associations between signature 1 and prognosis were affected by confounding factors, we incorporated age, grade, histology type, and lymph node status into the multivariate Cox regression model. Associations between signature 1 and the survival of patients with TNBC remained statistically significant after controlling for these factors (HR, 1.85 [95% CI, 1.08–3.18]; P = .024; ). We also investigated the correlation between signature 1 activities and patient age and found a positive correlation using the Spearman regression model (Spearman r = 0.197, P < .001; Supplementary Figure S7), further confirming previous findings.Citation6 We also examined the difference between mutational activity and signature 1 with respect to the mutational status of these 16 SMGs. Increased mutational activities of signature 1 were observed in samples with mutations in CBFB, FOXA1, NF1, PIK3CA, and TP53 (Wilcoxon rank-sum test, all P < .05; Supplementary Figure S8).
Immune-related pathways and immunocyte infiltration associated with mutational signature 1
In this analysis, we investigated the potential mechanism behind mutational signature 1, which may regulate TNBC prognosis. Gene set enrichment analysis (GSEA) on REACTOME sets revealed that the enrichment of genes involved in the immune system, cell cycle, and class I MHC antigen processing presentation were significantly altered in samples with different signature 1 grouping (q < 0.001, , Supplementary Figure S9). We further analyzed the determinants of tumor immunogenicity with respect to mutational signature 1 using The Cancer Immunome Atlas (TCIA)-recommended immunophenoscore (IPS) scheme. A significant negative correlation between signature 1 activity and immunogenicity level was identified across the TNBC samples (Spearman r = −0.13, P = .001, ). We also conducted mutational signature analysis on other age-related tumor types from the TCGA dataset, including lung adenocarcinoma (LUAD) and prostate adenocarcinoma (PRAD) (Supplementary Figure S10a-b), and found that signature 1 was inversely correlated with the IPS (Spearman correlation test, LUAD, r = −0.23; PRAD, r = −0.14; P < .001 Supplementary Figure S10c-d).
Figure 4. Significantly enriched pathways and immune infiltration alteration with signature 1 activities.
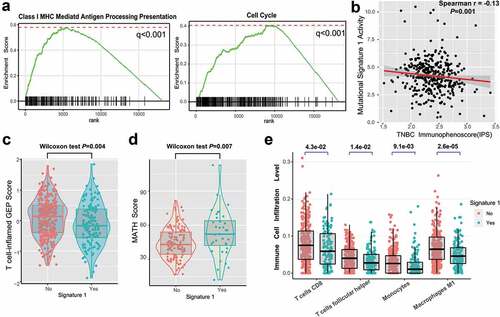
The T cell-inflamed gene expression profile (GEP) and immune cytolytic activity (CYT) were also analyzed, and a lower score was found in the signature 1 group (GEP scores, 0.25 vs −0.30, P = .004, ; CYT, 15.7 vs 11.2, P = .042, Supplementary Figure S11; Wilcoxon rank-sum test). We further compared clonal heterogeneity measured using the mutant allele tumor heterogeneity (MATH) score, which quantified the dispersion of variant allele frequencies in TNBC samples. Accordingly, patients with signature 1 had significantly higher MATH scores, indicating stronger clonal genomic heterogeneity (MATH scores, 43.6 vs 52.2, Wilcoxon rank-sum test, P = .007; ). Moreover, we evaluated (using the CIBERSORT algorithm) the abundance of tumor-infiltrating immunocytes in the TNBC microenvironment using the gene expression profile data. We found that CD8+ T cells, monocytes, macrophages, and antitumor leukocytes were less clustered in the mutational signature 1 group ().
Tumor mutation burden (TMB) associated with mutational signature 1
TNBC is genomically heterogeneous with varying TMBs, and its level is associated with the survival and immune infiltration of multiple cancer types.Citation20-Citation22 Therefore, we compared signature 1 with TMB and found a significantly lower TMB in patients with signature 1 (log2 TMB, median, 7.1 vs. 6.3, P < .001; Wilcoxon rank-sum test, ). Furthermore, MHC Class I-associated tumor neoantigen counts (burden) in the TCGA dataset also showed a similar tendency (log2 TNB, median, 6.7 vs 5.4, P < .001; ). TMB was largely attributed to genomic instability. To rule out the influence of confounding factors, we incorporated genomic integrity maintenance-associated genes (mutations in BRCA1/2, ATR, and SMGs in TNBC), extracted mutational signatures (1, 3, 13), and clinical features (age, grade, histology type, and lymph node status) into the Bayesian logistic regression model. The association between signature 1 and TMB remained statistically significant after adjusting for such factors (OR, 0.19 [95%CI, 0.11–0.32]; P < .001; ).
Figure 5. Association of signature 1 with tumor mutation burden in pooled TNBC cohort.
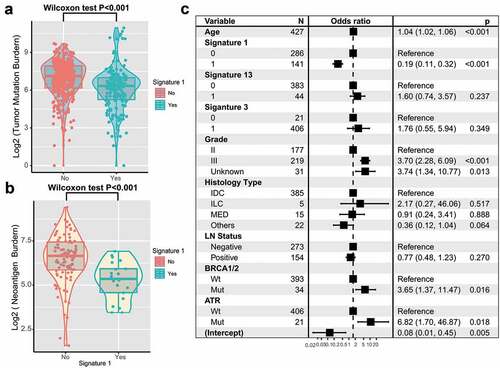
Discussion
In this study, a genomic meta-analysis of 538 TNBC samples from five published studies was performed and identified several novel less frequently mutated SMGs that were not recognized previously. We revealed that patients with age-related mutational signature 1 were associated with worse prognosis in TNBC and confirmed this finding in a multivariable Cox regression model. We further identified that this age-related signature was associated with lower immune activities and decreased TMB, suggesting that cancers with signature 1 probably escaped from immune surveillance.
Immune infiltration and surveillance in determining the prognosis of various types of cancers are increasingly recognized.Citation10,Citation23,Citation24 More than 70% of breast cancers contain at least some tumor-infiltrating lymphocytes and eliminate neoplastic cells by antitumor immunity. In this study, we identified that tumors with signature 1 were negatively associated with cytotoxic cell infiltration and antitumor immunogenicity, further supported by less enrichment among genes related to antigen processing presentation and interferon signaling. These findings suggest that the tumor immune escape mechanism of mutational signature 1 may predict the relapse-free survival of patients with TNBC. Moreover, signature 1 activities are negatively correlated with the anti-CTLA-4/PD-1 therapies immune response predictor IPS (determinant of tumor immunogenicity)Citation25 and neoantigen counts, suggesting that signature 1 may regulate treatment response to immunotherapy.
Comprehensive knowledge of the oncogenes underlying human cancers is a critical foundation for cancer diagnostics, therapeutics, and selection of rational combination therapies.Citation26 Here, we combined MutSigCV and OncodriveFML algorithms, followed by filter criteria, to re-annotate mutations and identified four unreported novel SMGs in TNBC. KDM6A (lysine demethylase 6A, mutated in 3.0% of patients) is located in the X chromosome and catalyzed the demethylation of tri/dimethylated histone H3.Citation27 Mutations in KDM6A could promote carcinogenesis and confer drug resistance in tumors.Citation28,Citation29 T-lymphocyte activation antigen CD86 is a receptor involved in the costimulatory signal essential for T-lymphocyte proliferation and interleukin-2 productionCitation30 and plays a vital role in anti-CTLA-4 blockade therapy immune response.Citation31 The RNA-binding protein RBM47 has been shown to strongly inhibit tumor progression and metastasis and was found to be substantially downregulated during the epithelial-to-mesenchymal transition in breast cancer. Another study demonstrated that RBM47 functions as a regulator of the p53/p21-signaling axis and plays an important role in antitumor effects. In our study, patients with mutations in RBM47 were associated with poor clinical outcome, probably due to the non-synonymous functional mutation causing gene expression aberration. The ligand-binding chain (alpha) of the gamma interferon receptor encoding gene IFNGR1, acted in IFN-γ pathways, and its mutation was reported to regulate the immune response to PD-1 treatment.Citation13,Citation32
Mutations patterns of signature 1 were commonly found in most cancer types (BRCA, LUAD, PRAD, etc.), known as clock-like mutational process properties, which showed a correlation between the number of mutations and age at diagnosis.Citation6 In this meta-analysis, the age at diagnosis was also correlated with signature 1 mutation activity in TNBCs. Indeed, older patients with breast cancer harbored a less active immune environment than younger patients with breast cancer.Citation7 Recent studies also noted a cancer type-specific (BRCA, PRAD) negative correlation of immune cell infiltration and mutational signature 1,Citation33 and further investigation is required to understand how signature 1-related mutated clones escaped from immune recognition.
Recent advances have reported that genomic mutational signatures are associated with clinical prognosis and treatment response. Xing et al. revealed that mutational signature 18 was significantly enriched in tumors with cadherin 1 (CDH1) mutations and associated with poor prognoses in gastric cancer.Citation34 There is evidence that the APOBEC mutational signature is a potential predictive marker for PD-1 immunotherapy response in NSCLC.Citation13,Citation35 In our genomic meta-analysis, we identified that signature 1 was associated with shortened survival time in patients with TNBC and suggested a significant association with tumor escape from immunological surveillance. A recent study indicated that short-term chemotherapy (e.g., doxorubicin and cisplatin) may induce a more favorable tumor microenvironment and increase the likelihood of response to PD-1 blockade in TNBC.Citation36 Therefore, we speculated that patients with signature 1 after induction treatments of doxorubicin and cisplatin may improve the clinical benefit of immune checkpoint inhibitor therapy.
The main limitation of this study was the use of the public datasets from different cohorts, which introduced a heterogeneity in data processing and could have resulted in a batch effect in the final mutation lists. Although we utilized multiple genomic and transcriptomic datasets for analysis, the dataset with both WES and RNA sequencing was only available in TCGA and FUSCC cohorts. As a result, the association between mutational patterns and gene expression, including analysis of immune cell infiltration and oncogenic pathways, needs further validation. In addition, considering that SMG non-synonymous mutations causing amino acid changes in protein function are huge and complicated, we will focus on these areas in future studies.
To our knowledge, this is the largest number of samples of a genomic study that combined multiple WES and RNA-Seq datasets, with the ultimate goal of providing novel genomic-driven therapeutic strategies for TNBC. We identified a putative clock-like mutation signature and molecular biomarkers of response to TNBC treatment, demonstrating the complex interplay of host and tumor in immune surveillance. However, the mechanisms underlying the association between signature 1 and prognostic outcome remain unclear, and further studies are warranted.
Materials and methods
Genomic and clinical data
Somatic mutations and clinical data (a total of 93897 coding somatic mutations in 538 samples) of the aggregated TNBC cohort were curatedfrom recent publications, including 65 samples from BCCRC cohort,Citation37 13 from Broad cohort,Citation38 14 from Sanger cohort,Citation39 279 from FUSCC cohort,Citation40 and 167 from TCGA (https://portal.gdc.cancer.gov). All patients were female, and the extracted DNA and RNA for sequencing were obtained from primary untreated tumor tissues. We re-annotated all previously called somatic mutations using the OncotatorCitation41 against the hg19 reference genomics database. Transcriptomic data were obtained from TCGA and FUSCC cohorts. Both peptides resulting from wild-type and mutated sequences for predicted binding affinities scores below 500 nM to patient HLA are defined as neoantigens.Citation42 Somatic copy number alteration was obtained from Davoli et al.Citation43 The gene microarray data for 33 paired tumor-normal tissues were downloaded from GEO (ID: GSE76250) published by Jiang et al.Citation14 Detailed clinical information, including age, histological type, lymph node status, relapse-free survival, and status, was also collected from these studies and is presented in Supplementary Table S2. The overall clinical characteristics with specific signature grouping are summarized in Supplementary Table S3.
Identification of SMGs
We identified SMGs by combining MutSigCVCitation26 and OncodriveFMLCitation44 algorithms, followed by further filtering procedures. MutSigCV measures the significant enrichment of non-silent somatic mutations in a gene by addressing mutational context-specific background mutation rates. OncodriveFML was designed to identify genes with highly clustered and nonrandomly distributed functional mutations. Candidate novel SMGs were required to meet these criteria: statistically significance in two algorithms (MutSigCV and OncodriveFML, both q < 0.1) and expression in human Cancer Cell Line Encyclopedia (https://portals.broadinstitute.org/ccle)Citation45 and TCGA pan-cancer dataset.Citation46
Deciphering the mutational signature operative in the genome
We used the framework suggested by Kim et al.Citation47 to extract mutational signatures from aggregated TNBC samples’ (n = 538) genomic data. The method was based on Bayesian variant non-negative matrix factorization and can automatically determine the optimal number of mutational signatures. The mutation portrait matrix A was factorized into two nonnegative matrices W and H, where W represents mutational processes and H represents the corresponding mutational activities. Mutational activity in every sample refers to the number of somatic mutations attributed to the extracted relevant signature. The number of columns in matrix W is the number of mutational signatures. The rows of matrix A are the 96 mutational contexts, and its columns are the 538 TNBC samples. The 96 mutational contexts are derived from combinations of 6 mutational types (i.e., C > A, C > G, C > T, T > A, T > C, and T > G) and their 5′- and 3′-adjacent bases. The extracted mutational portrait of TNBC was compared and annotated by cosine similarity analysis against the COSMIC.Citation8,Citation46
Extracted signature with TMB
The extracted mutational signatures were stratified as binary variables (i.e., no and yes) in the multivariate model. The classification method is based on the previous method, in which a signature was considered significant if it contributed to more than 100 substitutions or more than 25% of the total mutation activities.Citation8 The mutational signature activities and designation of each tumor sample were also indicated in Supplementary Table S2. As mutations in BRCA1/2 and ATR and some mutational signatures increase mutation rates in the cancer genome, we recognized them as confounding factors and incorporated them into the generalized linear models and fit proportional hazards regression to analyze associations between mutation signatures and TMB.
GSEA and network analysis
The R package limmaCitation48 was used to evaluate the differential expression of each gene in TNBC samples with different signature 1 activities (no vs yes). Specifically, gene expression data were normalized by voom and then fed to lmFit and eBayes functions in the R limma package. Differential expression statistics were used as input to perform GSEACitation49 based on the REACTOME gene set (downloaded from the MSigDB database version 6.3). The fast GSEA algorithm implemented in the Bioconductor R package fgsea was used.
Tumor infiltrating lymphocyte cell analysis
The deconvolution approach CIBERSORT (http://cibersort.stanford.edu/) was used to estimate the abundance of 22 distinct leukocyte subsetsCitation50 with the gene expression profile in TNBC.
Tumor immunogenicity analysis
IPS was used to determine the tumor immunogenicity, which was based on TCIA-recommended method.Citation25 The scoring scheme was developed from a panel of immune-related genes belonging to four classes: MHC-related molecules, checkpoints or immunomodulators, effector cells (activated CD8 + T cells and CD4 + T cells, Tem CD8+ and Tem CD4+ cells), and suppressor cells (Tregs and MDSCs). For each class, a sample-wise Z score from the gene expression data was calculated. Then, the weighted averaged Z score was calculated by averaging the Z scores within the respective category leading to four values and the sum of the weighted averaged Z score of the four categories was recognized as the IPS.
Quantifying the T cell-inflamed gene expression profiling (GEP) and CYT
T cell-inflamed gene expression profiling (GEP) proposed by Ayers et al.Citation51 were applied to assess T cell infiltration and quantify the GEP scores. GEP is composed of 18 inflammatory genes associated with chemokine expression, antigen presentation, and adaptive immune resistance. T cell-inflamed GEP scores were calculated as a weighted mean of normalized expression values for these genes. The CYT was calculated as the geometric mean of GZMA and PRF1 transcript levels.
MATH score
The MATH score was calculated as the median absolute deviation of each somatic mutation’s allelic fraction from the median allelic fraction of all mutations in the tumor, divided by the median variant allelic fraction (VAF).Citation10 We calculated MATH values for TCGA samples from mutant variant allele frequencies (VAF) of all genes.
Statistical analysis
The statistical analysis in this study was generated using R 3.6.1. Quantitative data were presented as median. Continuous variables between groups were compared using the Wilcoxon rank-sum test or paired t-test depending on data distribution. The Spearman correlation coefficient was used to analyze the correlation between two quantitative variables. Kaplan–Meier survival analysis and Cox proportional hazards model were used to analyze the association between mutational signatures and prognosis using the R survival package (survminer 0.4.6). We used stan_lm from the R package rstanarm (version 2.19.1) to perform multivariate Bayesian logistic regression analyses. All comparisons were two-sided with an alpha level of 0.05, and the Benjamini–Hochberg method was applied to control the false discovery rate for multiple hypothesis testing.Citation52
List of abbreviations
TCGA | = | the Cancer Genome Atlas |
HR | = | Hazard Ratio |
OR | = | Odds Ratio |
TCIA | = | The Cancer Immunome Atlas |
LUAD | = | Lung Adenocarcinoma |
PRAD | = | Prostate Adenocarcinoma |
FUSCC | = | Fudan University Shanghai Cancer Center |
BCCRC | = | British Columbia Cancer Research Center |
SMG | = | Significantly Mutated Gene |
HRD | = | Homologous Recombination Repair Deficiency |
TMB | = | Tumor Mutation Burden |
NB | = | Neoantigen Burden |
NMF | = | Nonnegative matrix factorization |
GSEA | = | Gene Sets Enrichment Analysis |
GEP | = | T cell-inflamed Gene Expression Profiling |
CYT | = | Cytolytic activity |
IPS | = | Immunophenoscore |
SNVs | = | Single-nucleotide Variants |
MDSCs | = | Myeloid-derived suppressor cells |
Availability of data and materials
All relevant data and materials within this work are made available in this manuscript and TCGA databases.
Disclosure of potential conflicts of interest
The authors report no conflict of interest.
Author contribution
All authors reviewed the manuscript and agreed to submission. H.C. designed the project. H.C., W.C., X.R.Y., Y.Z., S.W.S., X.C.L. performed administrative, technical, or material support. H.C. and W.C. performed Statistical analysis. H.C. wrote the manuscript. H.C, M.L. revised the paper.
Ethics approval and consent to participate
All studies have been approved by the Institutional Research Board.
Acknowledgments
The authors sincerely thanks Prof. Zhimin Shao, Yi-Zhou Jiang and Ding Ma from Fudan University Shanghai Cancer Center (FUSCC) for provided the genomic and transcriptomic sequencing data. We also thank Dr.Yichen Yang and Hongru Shen from Tianjin Medical University Cancer Institute and Hospital for helpful discussions and advice.
Supplementary material
Supplemental data for this article can be accessed on the publisher’s website.
Additional information
Funding
References
- Bauer KR, Brown M, Cress RD, Parise CA, Caggiano V. Descriptive analysis of estrogen receptor (ER)-negative, progesterone receptor (PR)-negative, and HER2-negative invasive breast cancer, the so-called triple-negative phenotype: a population-based study from the California cancer registry. Cancer. 2007;109(9):1721–10. doi:10.1002/cncr.22618.
- Dent R, Trudeau M, Pritchard KI, Hanna WM, Kahn HK, Sawka CA, Lickley LA, Rawlinson E, Sun P, Narod SA, et al. Triple-negative breast cancer: clinical features and patterns of recurrence. Clin Cancer Res. 2007;13(15):4429–4434. doi:10.1158/1078-0432.CCR-06-3045.
- Jiang T, Shi W, Natowicz R, Ononye SN, Wali VB, Kluger Y, Pusztai L, Hatzis C. Statistical measures of transcriptional diversity capture genomic heterogeneity of cancer. BMC Genomics. 2014;15(1):876. doi:10.1186/1471-2164-15-876.
- Bareche Y, Venet D, Ignatiadis M, Aftimos P, Piccart M, Rothe F, Sotiriou C. Unravelling triple-negative breast cancer molecular heterogeneity using an integrative multiomic analysis. Ann Oncol. 2018;29(4):895–902. doi:10.1093/annonc/mdy024.
- Lehmann BD, Pietenpol JA. Clinical implications of molecular heterogeneity in triple negative breast cancer. Breast. 2015;24(Suppl 2):S36–40. doi:10.1016/j.breast.2015.07.009.
- Alexandrov LB, Jones PH, Wedge DC, Sale JE, Campbell PJ, Nik-Zainal S, Stratton MR. Clock-like mutational processes in human somatic cells. Nat Genet. 2015;47(12):1402–1407. doi:10.1038/ng.3441.
- Kan Z, Ding Y, Kim J, Jung HH, Chung W, Lal S, Cho S, Fernandez-Banet J, Lee SK, Kim SW, Kan Z, Ding Y, Kim J, Jung HH, Chung W, Lal S. Multi-omics profiling of younger Asian breast cancers reveals distinctive molecular signatures. Nat Commun. 2018;9(1):1725. doi:10.1038/s41467-018-04129-4.
- Alexandrov LB, Nik-Zainal S, Wedge DC, Aparicio SA, Behjati S, Biankin AV, Bignell GR, Bolli N, Borg A, Børresen-Dale A-L, et al. Signatures of mutational processes in human cancer. Nature. 2013;500(7463):415–421. doi:10.1038/nature12477.
- Bianchini G, Qi Y, Alvarez RH, Iwamoto T, Coutant C, Ibrahim NK, Valero V, Cristofanilli M, Green MC, Radvanyi L, et al. Molecular anatomy of breast cancer stroma and its prognostic value in estrogen receptor-positive and -negative cancers. J Clin Oncol. 2010;28(28):4316–4323. doi:10.1200/JCO.2009.27.2419.
- Karn T, Jiang T, Hatzis C, Sanger N, El-Balat A, Rody A, Holtrich U, Becker S, Bianchini G, Pusztai L, et al. Association between genomic metrics and immune infiltration in triple-negative breast cancer. JAMA Oncol. 2017;3(12):1707–1711. doi:10.1001/jamaoncol.2017.2140.
- Connor AA, Denroche RE, Jang GH, Timms L, Kalimuthu SN, Selander I, McPherson T, Wilson GW, Chan-Seng-Yue MA, Borozan I, et al. Association of distinct mutational signatures with correlates of increased immune activity in pancreatic ductal adenocarcinoma. JAMA Oncol. 2017;3(6):774–783. doi:10.1001/jamaoncol.2016.3916.
- Desrichard A, Kuo F, Chowell D, Lee KW, Riaz N, Wong RJ, Chan TA, Morris LGT. Tobacco smoking-associated alterations in the immune microenvironment of squamous cell carcinomas. J Natl Cancer Inst. 2018;110(12):1386–1392. doi:10.1093/jnci/djy060.
- Chen H, Chong W, Teng C, Yao Y, Wang X, Li X. The immune response-related mutational signatures and driver genes in non-small-cell lung cancer. Cancer Sci. 2019;110(8):2348–2356. doi:10.1111/cas.14113.
- Jiang YZ, Liu YR, Xu XE, Jin X, Hu X, Yu KD, Shao ZM. Transcriptome analysis of triple-negative breast cancer reveals an integrated mRNA-lncRNA signature with predictive and prognostic value. Cancer Res. 2016;76(8):2105–2114. doi:10.1158/0008-5472.CAN-15-3284.
- Vanharanta S, Marney CB, Shu W, Valiente M, Zou Y, Mele A, Darnell RB, Massague J. Loss of the multifunctional RNA-binding protein RBM47 as a source of selectable metastatic traits in breast cancer. eLife. 2014;3. doi:10.7554/eLife.02734.
- Radine C, Peters D, Reese A, Neuwahl J, Budach W, Janicke RU, Sohn D. The RNA-binding protein RBM47 is a novel regulator of cell fate decisions by transcriptionally controlling the p53-p21-axis. Cell Death Differ. 2019. doi:10.1038/s41418-019-0414-6.
- Roberts SA, Lawrence MS, Klimczak LJ, Grimm SA, Fargo D, Stojanov P, Kiezun A, Kryukov GV, Carter SL, Saksena G, et al. An APOBEC cytidine deaminase mutagenesis pattern is widespread in human cancers. Nat Genet. 2013;45(9):970–976. doi:10.1038/ng.2702.
- Li X, Wu WK, Xing R, Wong SH, Liu Y, Fang X, Zhang Y, Wang M, Wang J, Li L, et al. Distinct subtypes of gastric cancer defined by molecular characterization include novel mutational signatures with prognostic capability. Cancer Res. 2016;76(7):1724–1732. doi:10.1158/0008-5472.CAN-15-2443.
- Chen H, Chong W, Wu Q, Yao Y, Mao M, Wang X. Association of LRP1b mutation with tumor mutation burden and outcomes in melanoma and non-small cell lung cancer patients treated with immune check-point blockades. Front Immunol. 2019;10:1113. doi:10.3389/fimmu.2019.01113.
- Jiang T, Shi W, Wali VB, Pongor LS, Li C, Lau R, Győrffy B, Lifton RP, Symmans WF, Pusztai L, et al. Predictors of chemosensitivity in triple negative breast cancer: an integrated genomic analysis. PLoS Med. 2016;13(12):e1002193. doi:10.1371/journal.pmed.1002193.
- Li X, Pasche B, Zhang W, Chen K. Association of MUC16 mutation with tumor mutation load and outcomes in patients with gastric cancer. JAMA Oncol. 2018;4(12):1691–1698. doi:10.1001/jamaoncol.2018.2805.
- Devarakonda S, Rotolo F, Tsao MS, Lanc I, Brambilla E, Masood A, Olaussen KA, Fulton R, Sakashita S, McLeer-Florin A, et al. Tumor mutation burden as a biomarker in resected non-small-cell lung cancer. J Clin Oncol. 2018;36(30):2995–3006. doi:10.1200/JCO.2018.78.1963.
- Efstathiou JA, Mouw KW, Gibb EA, Liu Y, Wu C-L, Drumm MR, da Costa JB, Du Plessis M, Wang NQ, Davicioni E, et al. Impact of immune and stromal infiltration on outcomes following bladder-sparing trimodality therapy for muscle-invasive bladder cancer. Eur Urol. 2019;76(1):59–68. doi:10.1016/j.eururo.2019.01.011.
- Mahajan UM, Langhoff E, Goni E, Costello E, Greenhalf W, Halloran C, Ormanns S, Kruger S, Boeck S, Ribback S, et al. Immune cell and stromal signature associated with progression-free survival of patients with resected pancreatic ductal adenocarcinoma. Gastroenterology. 2018;155(5):1625–1639 e1622. doi:10.1053/j.gastro.2018.08.009.
- Charoentong P, Finotello F, Angelova M, Mayer C, Efremova M, Rieder D, Hackl H, Trajanoski Z. Pan-cancer immunogenomic analyses reveal genotype-immunophenotype relationships and predictors of response to checkpoint blockade. Cell Rep. 2017;18(1):248–262. doi:10.1016/j.celrep.2016.12.019.
- Lawrence MS, Stojanov P, Polak P, Kryukov GV, Cibulskis K, Sivachenko A, Carter SL, Stewart C, Mermel CH, Roberts SA, et al. Mutational heterogeneity in cancer and the search for new cancer-associated genes. Nature. 2013;499(7457):214–218. doi:10.1038/nature12213.
- Chakraborty AA, Laukka T, Myllykoski M, Ringel AE, Booker MA, Tolstorukov MY, Meng YJ, Meier SR, Jennings RB, Creech AL, et al. Histone demethylase KDM6A directly senses oxygen to control chromatin and cell fate. Science. 2019;363(6432):1217–1222. doi:10.1126/science.aaw1026.
- Andricovich J, Perkail S, Kai Y, Casasanta N, Peng W, Tzatsos A. Loss of KDM6A activates super-enhancers to induce gender-specific squamous-like pancreatic cancer and confers sensitivity to BET inhibitors. Cancer Cell. 2018;33(3):512–526 e518. doi:10.1016/j.ccell.2018.02.003.
- Stief SM, Hanneforth AL, Weser S, Mattes R, Carlet M, Liu WH, Bartoschek MD, Dominguez MH, Oettle M, Kempf J, et al. Loss of KDM6A confers drug resistance in acute myeloid leukemia. Leukemia. 2019. doi:10.1038/s41375-019-0497-6.
- Chen L, Flies DB. Molecular mechanisms of T cell co-stimulation and co-inhibition. Nat Rev Immunol. 2013;13(4):227–242. doi:10.1038/nri3405.
- Wing JB, Ise W, Kurosaki T, Sakaguchi S. Regulatory T cells control antigen-specific expansion of Tfh cell number and humoral immune responses via the coreceptor CTLA-4. Immunity. 2014;41(6):1013–1025. doi:10.1016/j.immuni.2014.12.006.
- Gao J, Shi LZ, Zhao H, Chen J, Xiong L, He Q, Chen T, Roszik J, Bernatchez C, Woodman SE, et al. Loss of IFN-γ pathway genes in tumor cells as a mechanism of resistance to Anti-CTLA-4 therapy. Cell. 2016;167(2):397–404 e399. doi:10.1016/j.cell.2016.08.069.
- Budczies J, Seidel A, Christopoulos P, Endris V, Kloor M, Gyorffy B, Seliger B, Schirmacher P, Stenzinger A, Denkert C, et al. Integrated analysis of the immunological and genetic status in and across cancer types: impact of mutational signatures beyond tumor mutational burden. Oncoimmunology. 2018;7(12):e1526613. doi:10.1080/2162402X.2018.1526613.
- Xing R, Zhou Y, Yu J, Yu Y, Nie Y, Luo W, Yang C, Xiong T, Wu WKK, Li Z, et al. Whole-genome sequencing reveals novel tandem-duplication hotspots and a prognostic mutational signature in gastric cancer. Nat Commun. 2019;10(1):2037. doi:10.1038/s41467-019-09644-6.
- Wang S, Jia M, He Z, Liu X-S. APOBEC3B and APOBEC mutational signature as potential predictive markers for immunotherapy response in non-small cell lung cancer. Oncogene. 2018;37(29):3924–3936. doi:10.1038/s41388-018-0245-9.
- Voorwerk L, Slagter M, Horlings HM, Sikorska K, van de Vijver KK, de Maaker M. Immune induction strategies in metastatic triple-negative breast cancer to enhance the sensitivity to PD-1 blockade: the TONIC trial. Nat Med. 2019;25(6):920–928. doi:10.1038/s41591-019-0432-4.
- Shah SP, Roth A, Goya R, Oloumi A, Ha G, Zhao Y, Turashvili G, Ding J, Tse K, Haffari G, et al. The clonal and mutational evolution spectrum of primary triple-negative breast cancers. Nature. 2012;486(7403):395–399. doi:10.1038/nature10933.
- Banerji S, Cibulskis K, Rangel-Escareno C, Brown KK, Carter SL, Frederick AM, Lawrence MS, Sivachenko AY, Sougnez C, Zou L, et al. Sequence analysis of mutations and translocations across breast cancer subtypes. Nature. 2012;486(7403):405–409. doi:10.1038/nature11154.
- Stephens PJ, Tarpey PS, Davies H, Van Loo P, Greenman C, Wedge DC, Nik-Zainal S, Martin S, Varela I, Bignell GR, et al. The landscape of cancer genes and mutational processes in breast cancer. Nature. 2012;486(7403):400–404. doi:10.1038/nature11017.
- Jiang Y-Z, Ma D, Suo C, Shi J, Xue M, Hu X, Xiao Y, Yu K-D, Liu Y-R, Yu Y, et al. Genomic and transcriptomic landscape of triple-negative breast cancers: subtypes and treatment strategies. Cancer Cell. 2019;35(3):428–440 e425. doi:10.1016/j.ccell.2019.02.001.
- Ramos AH, Lichtenstein L, Gupta M, Lawrence MS, Pugh TJ, Saksena G, Meyerson M, Getz G. Oncotator: cancer variant annotation tool. Hum Mutat. 2015;36(4):E2423–2429. doi:10.1002/humu.22771.
- Rooney MS, Shukla SA, Wu CJ, Getz G, Hacohen N. Molecular and genetic properties of tumors associated with local immune cytolytic activity. Cell. 2015;160(1–2):48–61. doi:10.1016/j.cell.2014.12.033.
- Davoli T, Uno H, Wooten EC, Elledge SJ. Tumor aneuploidy correlates with markers of immune evasion and with reduced response to immunotherapy. Science. 2017;355(6322):eaaf8399. doi:10.1126/science.aaf8399.
- Mularoni L, Sabarinathan R, Deu-Pons J, Gonzalez-Perez A, Lopez-Bigas N. OncodriveFML: a general framework to identify coding and non-coding regions with cancer driver mutations. Genome Biol. 2016;17(1):128. doi:10.1186/s13059-016-0994-0.
- Ghandi M, Huang FW, Jane-Valbuena J, Kryukov GV, Lo CC, McDonald ER 3rd, Barretina J, Gelfand ET, Bielski CM, Li H, et al. Next-generation characterization of the cancer cell line encyclopedia. Nature. 2019;569(7757):503–508. doi:10.1038/s41586-019-1186-3.
- Kandoth C, McLellan MD, Vandin F, Ye K, Niu B, Lu C, Xie M, Zhang Q, McMichael JF, Wyczalkowski MA, et al. Mutational landscape and significance across 12 major cancer types. Nature. 2013;502(7471):333–339. doi:10.1038/nature12634.
- Kim J, Mouw KW, Polak P, Braunstein LZ, Kamburov A, Kwiatkowski DJ, Kwiatkowski DJ, Rosenberg JE, Van Allen EM, D’Andrea AD, et al. Somatic ERCC2 mutations are associated with a distinct genomic signature in urothelial tumors. Nat Genet. 2016;48(6):600–606. doi:10.1038/ng.3557.
- Ritchie ME, Phipson B, Wu D, Hu Y, Law CW, Shi W, Smyth GK. limma powers differential expression analyses for RNA-sequencing and microarray studies. Nucleic Acids Res. 2015;43(7):e47. doi:10.1093/nar/gkv007.
- Subramanian A, Tamayo P, Mootha VK, Mukherjee S, Ebert BL, Gillette MA, Paulovich A, Pomeroy SL, Golub TR, Lander ES, et al. Gene set enrichment analysis: a knowledge-based approach for interpreting genome-wide expression profiles. Proc Natl Acad Sci U S A. 2005;102(43):15545–15550. doi:10.1073/pnas.0506580102.
- Newman AM, Liu CL, Green MR, Gentles AJ, Feng W, Xu Y, Hoang CD, Diehn M, Alizadeh AA. Robust enumeration of cell subsets from tissue expression profiles. Nat Methods. 2015;12(5):453–457. doi:10.1038/nmeth.3337.
- Ayers M, Lunceford J, Nebozhyn M, Murphy E, Loboda A, Kaufman DR, Albright A, Cheng JD, Kang SP, Shankaran V, et al. IFN-gamma-related mRNA profile predicts clinical response to PD-1 blockade. J Clin Invest. 2017;127(8):2930–2940. doi:10.1172/JCI91190.
- Love MI, Huber W, Anders S. Moderated estimation of fold change and dispersion for RNA-seq data with DESeq2. Genome Biol. 2014;15(12):550. doi:10.1186/s13059-014-0550-8.