ABSTRACT
The purpose of this study was to identify novel autoantibodies against tumor-associated antigens (TAAbs) and explore the optimal diagnosis model based on the protein chip for detecting esophageal squamous cell carcinoma (ESCC). The human protein chip based on cancer-driving genes was customized to discover candidate TAAbs. Enzyme-linked immunosorbent assay was applied to verify and validate the expression levels of candidate TAAbs in the training cohort (130 ESCC and 130 normal controls) and the validation cohort (125 ESCC and 125 normal controls). Logistic regression analysis was adopted to construct the diagnostic model based on the expression levels of autoantibodies with diagnostic value. Twelve candidate autoantibodies were identified based on the protein chip according to the corresponding statistical methods. In both the training cohort and validation cohort, the expression levels of 10 TAAbs (GNA11, PTEN, P53, SRSF2, GNAS, ACVR1B, CASP8, DAXX, PDGFRA, and MEN1) in ESCC patients were higher than that in normal controls. The panel consisting of GNA11, ACVR1B and P53 demonstrated favorable diagnostic power. The sensitivity, specificity and accuracy of the model in the train cohort and the validation cohort were 71.5%, 93.8%, 79.6% and 77.6%, 81.6%, 70.8%, respectively. In either cohort, there was no correlation between positive rate of the autoantibody panel and clinicopathologic features for ESCC patients. Protein chip technology is an effective method to identify novel TAAbs, and the panel of 3 TAAbs (GNA11, ACVR1B, and P53) is promising for distinguishing ESCC patients from normal individuals.
1 Introduction
Esophageal cancer (EC) ranked sixth among the leading causes of cancer deaths with an estimated 509 000 deaths, and seventh in terms of incidence with an estimated 572 000 new cases in 2018 worldwide.Citation1 In 2015, there were estimated 188 000 deaths and 246 000 new cases of EC according to the latest report of cancer epidemiology in China.Citation2 In China, the large majority of EC patients were esophageal squamous cell carcinoma (ESCC) patients.Citation3 EC has a dreadful 5-year survival rate of 19%, because a multitude of patients are diagnosed at late stage.Citation4 The 5-year survival rate of patients with stage I is as high as 80%, which indicates early detection is critical for reducing the mortality of EC patients.Citation5,Citation6 Endoscopy examination and mucosa biopsy are common diagnostic methods for EC patients. Nevertheless, sampling error, invasiveness and high cost limit their wide applications in screening practice.Citation7 The commonly used auxiliary diagnostic markers in clinical practice, such as squamous cell carcinoma antigen (SCCA), carcinoembryonic antigen (CEA), and cytokeratin 19 fragment (CYFRA21-1), lack sufficient diagnostic ability for EC.Citation8 Tumor-associated antigens (TAAs) are the abnormally expressed proteins that are able to trigger immune response due to the occurrence and development of cancers, and the produced autoantibodies are called autoantibodies against tumor-associated antigens (TAAbs).Citation9 TAAbs can be detected before the patient’s clinical symptoms and present higher stability over time.Citation10 In recent years, many studies have demonstrated that TAAbs may be a promising tool of detecting cancers, such as hepatocellular carcinoma,Citation11,Citation12 ovarian cancer,Citation13 lung cancer,Citation14 colorectal cancer,Citation15 breast cancer,Citation16 and esophageal cancer.Citation17
Recently, protein chip technology accelerates the research process of tumor biomarkers. Yang et al. used a human proteome microarray to discover potential serum biomarkers and identify a promising panel for detecting gastric cancer.Citation18 However, Huprot™ Human proteomic chip is expensive and needs a lot of human and material resources. The occurrence of tumor is a complex process involving multiple genes and factors. Carcinogens are able to influence normal cells and cause gene mutations. It has been reported that the function or structure of the proteins encoded by the mutated genes will change, and these abnormal proteins may in turn induce immune response to produce corresponding autoantibodies.Citation19,Citation20 The mutated genes that promote the growth and proliferation of cancer cells are called cancer-driving genes, which can cause selective growth of tumor cells.Citation21 Bert Vogelstein et al. reported 138 cancer-driving genes by analyzing the result of high-throughput gene sequencing of cancer genome.Citation22 Therefore, we put forward a hypothesis: autoantibodies against tumor-associated antigens encoded by cancer-driving genes may be potential biomarkers for detecting ESCC. In this study, we customized the protein chip based on 154 human proteins mainly encoded by the cancer-driving genes to identify TAAbs for detection of ESCC. The design of the present study is illustrated in .
2 Materials and methods
2.1 Collection of serum samples
This study consisted of 646 subjects, including 341 ESCC patients and 305 healthy individuals. From June 2013 to December 2017, serums from ESCC patients were collected from a third-level grade-A hospital (Zhengzhou, China). All patients were confirmed by histopathology and had no other malignancies and had not received any treatments. The normal controls, without digestive tract related diseases, and autoimmune diseases, were selected from the biological sample bank of Henan Key Laboratory of Tumor Epidemiology. In verification and validation phase, 510 subjects were selected, including 255 ESCC patients and 255 normal controls. The case group and control group were matched 1:1 according to the gender and age (± 3 years). The serums were collected according to the standard protocol. Approval for this study was obtained from the Medical Ethics Committee of Zhengzhou University and informed consent was signed by all participants.
2.2 Customization of protein microarray
This study was commissioned to the BCBIO Biotechnology Corporation (Guangzhou, China) to customize the HuProtTM protein chip based on cancer-driving genes. The protein chip contained 154 human recombinant proteins (more than one different protein fragment for the same gene),including 143 proteins encoded by cancer driving genes and 11 proteins (IMP1, IMP2, IMP3, CyclinB1, c-Myc, CIP2A/p90, RalA, YWHAZ, RBM39, and two fragments of Survivin) with high diagnostic value in the previous research in our laboratory. The layout of 154 recombinant proteins on the protein microarray was presented in Table S1. The autoantibodies of corresponding proteins in serums from 86 ESCC patients and 50 normal controls were detected by protein chip technology to discover candidate autoantibodies for detecting ESCC. The experimental process was carried out in accordance with the standard protocol.
2.3 Enzyme-linked immunosorbent assay (ELISA)
ELISA was used to detect the expression levels of autoantibodies discovered by protein chip. The coated concentrations of the 12 recombinant proteins (SRSF2, ACVR1B, MEN1, CASP8, FBXW7, PDGFRA, GNAS, PTEN, DAXX, P53, GNA11, and EGFR) were 0.125, 0.125, 0.125, 0.125, 0.125, 0.25, 0.25, 0.25, 0.25, 0.5, 0.5, and 0.5 ng/ml, respectively. The last column of each 96-well plate was coated with human IgG protein to provide the standard curve. The corresponding step of ELISA have been described in our previous study.Citation23The expression levels of autoantibodies were converted into the concentration according to the standard curve of each plate, and the concentrations were defined as unit (U).
2.4 Statistical analysis
Genepix Pro 6.0, IBM SPSS Statistics software (Version 21.0) and GraphPad Prism 6.0 were applied to analyze the data of this study. For the extracted data of IgG-responsive proteins, the signal-to-noise ratio (SNR) was defined as the ratio of the foreground to background median intensity in order to eliminate the deviation caused by the inconsistency of background intensity between different samples. The differences in clinical characteristics of participant in each group were analyzed by Fisher’s test or t test. The nonparametric test was used to compare the difference between patient group and control group. The receiver operating characteristic (ROC) curve was used to evaluate the diagnostic value of autoantibodies. The sensitivity and specificity were determined by maximum Yoden Index (YI). Logistic regression analysis was used to explore the optimal panel for distinguishing ESCC from healthy individuals. All P values were determined based on two-tailed and P < .05 was defined to be significant.
3 Results
3.1 Characteristics of study population
The whole study consisted of three phases, including discovery phase, verification phase and validation phase. In the discovery phase, the serum samples from 86 ESCC patients and 50 normal individuals were detected by the protein chip technology. The basic characteristics of 136 participants were described in Table S2. In verification phase and validation phase, ELISA was applied to test the expression level of 12 candidate TAAbs in serums from 255 ESCC patients and 255 normal controls. Clinical characteristics of ESCC patients and controls were shown in . There was no significant difference in age, histological grade, site of tumor, depth of tumor invasion, and distant metastasis status in the two cohorts. All patients were staged according to the TNM staging standard of the Union for International Cancer Control (UICC).Citation24
Table 1. Clinical characteristics of ESCC patients and controls in verification and validation phase.
3.2 Candidate TAAbs based on protein microarray in discovery phase
Based on the SNRs of 136 serum samples in the protein chip, 12 candidate TAAbs (SRSF2, ACVR1B, MEN1, CASP8, FBXW7, PDGFRA, GNAS, PTEN, DAXX, P53, GNA11, and EGFR) were screened by different statistical methods. The candidate TAAbs obtained by nonparametric test and ROC curve analysis were consistent, including GNA11, PTEN, P53, SRSF2, and GNAS. The top ten candidate TAAbs in area under ROC (AUC) were GNA11, PTEN, P53, SRSF2, GNAS, ACVR1B, CASP8, DAXX, PDGFRA, and FBXW7. In addition, five candidate TAAbs (P53, GNAS, MEN1, EGFR, and CASP8) were determined when the difference of positive rate (cut off = mean+2 standard error) of SNR between case group and control group was more than 10%. Table S3 illustrated the diagnostic value of 12 candidate TAAbs. The AUCs ranged from 0.54 to 0.68. GNA11 demonstrated the greatest diagnostic performance for ESCC with an AUC of 0.68 (95%CI: 0.59–0.78), sensitivity of 48.8%, specificity of 85.7% and accuracy of 66.7% among 12 TAAbs. P53 and MEN1 achieved the favorable specificity of 93.9%, and PDGFRA presented the outstanding sensitivity of 70.9%. Although the sensitivity of EGFR and CASP8 were less impressive (22.1% and 26.7%, respectively), the specificities were promising (91.8% and 87.8%, respectively). Table S4 exhibited the basic characteristics of screened candidate TAAbs.
3.3 Autoantibodies in verification phase and validation phase
The expression levels of the 12 anti-TAAs autoantibodies (SRSF2, ACVR1B, MEN1, CASP8, FBXW7, PDGFRA, GNAS, PTEN, DAXX, P53, GNA11, and EGFR) were calculated according to the standard curve of each plate. ) and ) displayed the expression levels of 12 anti-TAAs autoantibodies in training cohort and validation cohort, respectively. In the training cohort, except for EGFR and FBXW7, the levels of ten anti-TAAs autoantibodies were significantly higher in ESCC group than that in control group, and the results were true in the validation cohort. and presented separately the ROC curve analysis of 12 autoantibodies in two cohorts. The diagnostic value of single autoantibody for ESCC detection was illuminated in . In the training cohort, the AUCs ranged from 0.50 to 0.84, and the ranges of the sensitivity and specificity were 24.6%–88.8% and 26.9%–96.9%, respectively. GNA11 manifested the highest diagnostic accuracy of 75.8% with an AUC of 0.84 (95%CI: 0.80–0.89), sensitivity of 59.2% and specificity of 96.9% among 12 anti-TAAs autoantibodies. In the validation cohort, the AUCs ranged from 0.55 to 0.82, and the ranges of the sensitivity and specificity were 36.8%–96.8% and 28.8%–92.8%, respectively.
Table 2. Diagnostic value of 12 anti-TAAs autoantibodies in training and validation cohort for ESCC detection.
Figure 2. The expression levels of 12 anti-TAAs autoantibodies in ESCC patients and healthy controls. (a) Scatter plots of the expression levels of autoantibodies in training cohort, (b) scatter plots of the expression levels of autoantibodies in validation cohort. Lines represented median and quartile range; E, ESCC patients; N normal controls.
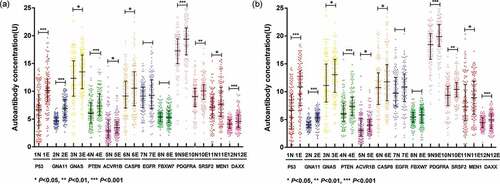
3.4 Establishment and validation of diagnostic model of autoantibodies
Logistic regression analysis was adopted to generate diagnostic model based on the expression levels of ten significant TAAbs (GNA11, PTEN, P53, SRSF2, GNAS, ACVR1B, CASP8, DAXX, PDGFRA, and MEN1) in training cohort (130 ESCC and 130 NC). The diagnostic model constructed by the backward or forward logistic regression methods were completely consistent. The predicted possibility for diagnosis as ESCC was PRE (P = ESCC, 3 TAAbs) = 1/(1+ EXP (-(-6.822-0.388 × ACVR1B + 1.155 × GNA11 + 0.185 × P53))). The diagnostic model consisting of ACVR1B, GNA11 and P53 was able to distinguish ESCC patients from healthy individuals, with an AUC of 0.88 (95%CI: 0.84–0.92), the sensitivity of 71.5%, the specificity of 93.8% and the accuracy rate of 79.6% (). The formula of predictive probability attained from the training cohort was substituted into the validation cohort (125 ESCC and 125 NC). The diagnostic value of the prediction model in the validation cohort was similar to that in the training cohort, with an AUC of 0.85 (95%CI: 0.80–0.90), the sensitivity of 77.6%, the specificity of 81.6% and the concordance rate of 70.8% (). There was no significant difference in AUC between the two cohorts (P = .885), which indicated that the model was stable.
Table 3. Diagnostic value of the anti-TAAs autoantibodies panel for ESCC patients with different stages.
3.5 Evaluation of the diagnostic value of the model in different stages
The diagnostic performance of the model for ESCC patients with different stages was illuminated in and . In training cohort, the prediction model provided an enhanced AUC of 0.90 (95%CI: 0.85–0.95), with the sensitivity of 73.1%, the specificity of 93.9% and the accuracy rate of 81.3% for early detection of ESCC (stage 0–II). The diagnostic value of this panel for early cancer patients was higher than that for late cancer patients, but the difference in AUCs was not significant (P = .066). The ability of the anti-TAAs autoantibodies panel to distinguish early-stage cancer patients from healthy controls was confirmed in an independent validation cohort. The autoantibodies panel exhibited almost the same diagnostic value for early ESCC in the validation cohort, with an AUC of 0.87 (95%CI: 0.83–0.92), with the sensitivity of 81.5%, the specificity of 81.6% and the consistency rate of 76.5% (). Besides, there was no significant difference in the ability of the model to distinguish the ESCC patients of different depth of tumor invasion, the presence of lymph node metastasis and the presence of distant metastasis (P > .05) ().
3.6 Effect of clinicopathological features on the sensitivity and specificity
The impact of cancer patients of clinicpathologic feature on the specificity/sensitivity variation was denoted in Table S5 and Figure S1. ESCC patients were classified according to sex, age, histological grade, site of tumor, TNM stage, depth of tumor invasion, lymph node metastasis status, and distant metastasis status. Table S5 demonstrated there was no relationship between positive rate of the autoantibody panel and clinicopathologic features in both the training cohort and validation cohort (P > .05). Figure S1 presented the sensitivity at a fixed specificity of 95% by patient demographics and tumor characteristics. There was seemingly a higher incidence of the autoantibodies panel in patients with early stage than later stage, negative distant metastasis than positive distant metastasis.
4 Discussion
In this study, we discovered 12 candidate autoantibodies for diagnosis of ESCC by customized protein chip based on cancer-driving genes in 136 serum samples and employed ELISA to detect the expression levels of 12 TAAbs in 510 individual samples. We constructed a diagnostic model consisting of P53, ACVR1B and GNA11 that achieved great diagnostic value, with an AUC of 0.88 (95%CI: 0.84–0.92), the sensitivity, the specificity and the accuracy rate of 71.5%, 93.8%, and 79.6%, respectively in the training cohort. The AUC in the training cohort was similar to that in the validation cohort (P = .885), which indicated that the model had rosy stability. The results demonstrated that the ability of the model to differentiate ESCC was higher than that of reported autoantibodies combinations,Citation25,Citation26 and it had a promising ability to differentiate ESCC cancer from normal individuals.
There are a lot of methods for screening candidate TAA for early diagnosis of cancer, and the most commonly used techniques are serological analysis of expression cDNA libraries (SEREX) and serological proteome analysis (SERPA). However, the false positive rate of SEREX technique is too high and unable to screen post-translational modified proteins.Citation27 Due to the limitation of the loading amount of sample and detection sensitivity, SERPA can only identify relatively high abundance proteins, and its ability to isolate polar acids and alkaloids is limited.Citation28 With the development of human genome sequencing, high-throughput protein chip technology is also used for screening TAAb for detecting cancer. Nevertheless, its wide application in screening TAA is limited by its high economic cost. We applied the protein chip consisting of 154 recombinant human proteins encoded by cancer-driving genes to detect autoantibodies in ESCC and normal serums to discover candidate biomarkers. It not only retained the advantages of protein chip technology and greatly reduced the cost of research, but also improved the probability that specific autoantibodies were screened out.
Based on the customized protein chip of cancer-driving genes and ELISA, ten autoantibodies (GNA11, SRSF2, GNAS, PDGFRA, PTEN, P53, ACVR1B, CASP8, DAXX, and MEN1) related to the diagnosis of ESCC were identified in this study. The genes encoding the first three proteins are carcinogenic genes, and the last six genes are tumor suppressor genes. GNA11 belongs to the family of guanine nucleotide-binding proteins (G proteins), which function as modulators or transducers in various transmembrane signaling systems.Citation29 SRSF2 is a member of the serine/arginine (SR)-rich family of pre-mRNA splicing factors and involved in regulating cell proliferation and maintaining genomic stability.Citation30 GNAS is a complex imprinted expression gene and an important component of many signal transduction pathways, such as APC, PI3K, TGF-b, and RAS pathway.Citation31 PDGFRA is closely related to the invasion and malignant transformation of tumors. Immunochemical analysis showed that PDGFRA was positive in a rare case of primary small cell carcinoma of the esophagus.Citation32 PTEN is involved in a variety of cell signal transduction pathways, including regulation of cell growth, migration, apoptosis, and maintenance of chromosomal integrity.Citation33,Citation34 The mutation of TP53 can acquire carcinogenic characteristics and promote the invasion, metastasis, proliferation, and survival of cancer cells. Wu et al. reported that the positive rate of anti-P53 autoantibody in serum of cancer patients was significantly higher than that of healthy controls (14.59% vs 1.02%), which suggested that anti-P53 autoantibody can be used to detect malignant tumors.Citation35 ACVR1B, an activin sensor, belongs to the transforming growth factor beta (TGF-beta) superfamily and is involved in regulating muscle growth.Citation36 CASP8 is involved in exogenous apoptotic signaling pathway, and its genetic variation can affect cancer susceptibility.Citation37 DAXX can bind to the death domain of Fas death receptor and enhance Fas-mediated apoptosis.Citation38 MEN1 is known as multiple endocrine neoplasia type 1 and regulates several pathways and processes by altering chromatin structure through the modification of histones.Citation39 Except for P53, there was no report on the expression level of nine autoantibodies in serum from ESCC patients.
A host of studies have shown that autoantibodies in serum of cancer patients can be used for detecting cancers. This study included two independent cohorts (training cohort and validation cohort) to verify discovered autoantibodies and evaluated the diagnostic value of single autoantibody for ESCC patients in training cohort. The results indicated that the ranges of AUC, sensitivity and specificity of 10 meaningful autoantibodies (GNA11, SRSF2, GNAS, PDGFRA, PTEN, P53, ACVR1B, CASP8, DAXX, and MEN1) were 0.58–0.84, 33.8%–88.5%, and 26.9%–96.9%, respectively. Accordingly, the diagnostic performance of single autoantibody was not sufficient for cancers, which is consistent with prior reports.Citation25,Citation40 Studies have reported that combined autoantibodies can improve the sensitivity of detecting cancers.Citation41 A lot of researchers had poured attention into exploring the optimal panel of autoantibodies for the diagnosis of ESCC patients. Zhou et al. reported great diagnostic accuracy of 6 autoantibodies combination (c-Myc, P53, P62, P16, cyclin B1, and IMP1) in detection of ESCC, with an AUC of 0.78, the sensitivity of 64% and the specificity of 94%.Citation42 However, this study did not establish a prediction model and lacked further validation. Xu et al. showed that a panel consisting of Hsp70, P53, Prx-VI, and NY-ESO-1 attained the sensitivity of 55%, the specificity of 98 and the sensitivity of 48%, the specificity of 96% for ESCC detection in the test and validation cohorts.Citation25 In two studies, the selected autoantibodies were not the biomarkers based on esophageal cancer, and lack of specificity for cancer. In addition, the sensitivity and specificity are unable to meet the clinical requirement. In the current study, we used ESCC patients and normal controls to screen out 12 candidate autoantibodies. In the verification phase and validation phase, logistic regression analysis was employed to generate prediction model including P53, ACVR1B and GNA11 (AUC = 0.88, sensitivity = 71.5%, specificity = 93.8%), and the diagnostic value of this panel was confirmed in validation cohort. Logistic regression analysis is a traditional statistical method for screening related variables and has been widely adopted to classify cancers.Citation43
Our study was superior compared with studies on identifying serum autoantibodies for diagnosis of ESCC. Firstly, this study was the first to customize the protein microarray based on the cancer-driving genes to discover autoantibodies associated with ESCC and had yielded promising results. Secondly, logistic regression analysis was applied to establish the diagnostic model in the training cohort, and the diagnostic value of the panel was validated in an independent cohort. However, this study also has some limitations. Although the customized protein chip encoded by cancer-driving genes saved cost, there was also the possibility of missing the proteins that were not customized on the chip.
Using protein microarray to identify cancer-related autoantibodies is a feasible method for detection of cancer. The diagnostic model consisting of P53, ACVR1B and GNA11 may be useful for differentiation between ESCC patients and healthy persons.
Declaration of interest statement
The authors report no conflict of interest.
Supplemental Material
Download ()Supplementary material
Supplemental data for this article can be accessed on the publisher’s website
Additional information
Funding
References
- Ferlay J, Colombet M, Soerjomataram I, Mathers C, Parkin DM, Piñeros M, Znaor A, Bray F. Estimating the global cancer incidence and mortality in 2018: GLOBOCAN sources and methods[J]. Int J Cancer. 2019;144(8):1941–10. doi:10.1002/ijc.31937.
- Zheng RS, Sun KX, Zhang SW. Report of cancer epidemiology in China, 2015[J]. Chin J Oncol. 2019;41:19–28.
- Cao XQ, Sun XB. Incidence and trend of esophageal cancer[J]. Chin J Clin Oncol. 2016;43(21):932–936. doi:10.3969/j..1000-8179.2016.21.984.
- Siegel RL, Miller KD, Jemal A. Cancer statistics, 2018[J]. CA Cancer J Clin. 2018;68(1):7–30. doi:10.3322/caac.21442.
- Zhang L, Li W, Lyu X, Song Y, Mao Y, Wang S, Huang J. Adjuvant chemotherapy with paclitaxel and cisplatin in lymph node-positive thoracic esophageal squamous cell carcinoma[J]. Chin J Cancer Res. 2017;29(2):149–155. doi:10.21147/j..1000-9604.2017.02.08.
- Tachimori Y, Ozawa S, Numasaki H, Ishihara R, Matsubara H, Muro K, Oyama T, Toh Y, Udagawa H, Uno T, et al. Comprehensive registry of esophageal cancer in Japan, 2012[J]. Esophagus. 2019;16(3):221–245. doi:10.1007/s10388-019-00674-z.
- Zhang C, Wang C, Chen X, Yang C, Li K, Wang J, Dai J, Hu Z, Zhou X, Chen L, et al. Expression profile of microRNAs in serum: a fingerprint for esophageal squamous cell carcinoma[J]. Clin Chem. 2010;56(12):1871–1879. doi:10.1373/clinchem.2010.147553.
- Hirajima S, Komatsu S, Ichikawa D, Takeshita H, Konishi H, Shiozaki A, Morimura R, Tsujiura M, Nagata H, Kawaguchi T, et al. Clinical impact of circulating miR-18a in plasma of patients with oesophageal squamous cell carcinoma[J]. Br J Cancer. 2013;108(9):1822–1829. doi:10.1038/bjc.2013.148.
- Tan EM, Zhang J. Autoantibodies to tumor-associated antigens: reporters from the immune system[J]. Immunol Rev. 2008;222(1):328–340. doi:10.1111/j.1600-065X.2008.00611.x.
- Liu W, Peng B, Lu Y, Xu W, Qian W, Zhang J-Y. Autoantibodies to tumor-associated antigens as biomarkers in cancer immunodiagnosis[J]. Autoimmun Rev. 2011;10:331–335.
- Zheng SX, Xiang BD, Long JM, Qu C, Mo ZJ, Li K, Zhuang Y, Lv ZL, Zhou SF. Diagnostic value of serum SMP30 and Anti-SMP30 antibody in hepatocellular carcinoma[J]. Lab Med. 2018;1–8. doi:10.1093/labmed/lmy004.
- Zhang JY, Tan EM. Autoantibodies to tumor-associated antigens as diagnostic biomarkers in hepatocellular carcinoma and other solid tumors[J]. Expert Rev Mol Diagn. 2010;10:321–328. doi:10.1586/erm.10.12.
- Katchman BA, Chowell D, Wallstrom G, Vitonis AF, LaBaer J, Cramer DW, Anderson KS. Autoantibody biomarkers for the detection of serous ovarian cancer[J]. Gynecol Oncol. 2017;146(1):129–136. doi:10.1016/j.ygyno.2017.04.005.
- Dai L, Li J, Tsay JJ, Yie T-A, Munger JS, Pass H, Rom WN, Tan EM, Zhang J-Y. Identification of autoantibodies to ECH1 and HNRNPA2B1 as potential biomarkers in the early detection of lung cancer[J]. Oncoimmunol. 2017;6:e1310359. doi:10.1080/2162402x.2017.1310359.
- Pedersen JW, Gentry-Maharaj A, Nostdal A, Fourkala E-O, Dawnay A, Burnell M, Zaikin A, Burchell J, Papadimitriou JT, Clausen H, et al. Cancer-associated autoantibodies to MUC1 and MUC4–a blinded case-control study of colorectal cancer in UK collaborative trial of ovarian cancer screening[J]. Int J Cancer. 2014;134:2180–2188. doi:10.1002/ijc.28538.
- Loke SY, Lee ASG. The future of blood-based biomarkers for the early detection of breast cancer[J]. Eur J Cancer. 2018;92:54–68. doi:10.1016/j.ejca.2017.12.025.
- Looi K, Megliorino R, Shi FD, Peng XX, Chen Y, Zhang JY. Humoral immune response to p16, a cyclin-dependent kinase inhibitor in human malignancies[J]. Oncol Rep. 2006;16:1105–1110.
- Yang L, Wang J, Li J, Zhang H, Guo S, Yan M, Zhu Z, Lan B, Ding Y, Xu M, et al. Identification of serum biomarkers for gastric cancer diagnosis using a human proteome microarray[J]. Mol Cell Proteomics. 2016;15(2):614–623. doi:10.1074/mcp.M115.051250.
- Tan EM. Autoantibodies as reporters identifying aberrant cellular mechanisms in tumorigenesis[J]. J Clin Invest. 2001;108(10):1411–1415. doi:10.1172/JCI14451.
- Schwitalle Y, Kloor M, Eiermann S, Linnebacher M, Kienle P, Knaebel HP, Tariverdian M, Benner A, von Knebel Doeberitz M. Immune response against frameshift-induced neopeptides in HNPCC patients and healthy HNPCC mutation carriers[J]. Gastroenterology. 2008;134(4):988–997. doi:10.1053/j.gastro.2008.01.015.
- Greenman C, Stephens P, Smith R, Dalgliesh GL, Hunter C, Bignell G, Davies H, Teague J, Butler A, Stevens C, et al. Patterns of somatic mutation in human cancer genomes[J]. Nature. 2007;446(7132):153–158. doi:10.1038/nature05610.
- Vogelstein B, Papadopoulos N, Velculescu VE, Zhou S, Diaz LA, Kinzler KW. Cancer genome landscapes[J]. Science. 2013;339(6127):1546–1558. doi:10.1126/science.1235122.
- Wang S, Qin J, Ye H, Wang K, Shi J, Ma Y, Duan Y, Song C, Wang X, Dai L, et al. Using a panel of multiple tumor-associated antigens to enhance autoantibody detection for immunodiagnosis of gastric cancer[J]. Oncoimmunol. 2018;7:e1452582. doi:10.1080/2162402x.2018.1452582.
- Sobin LH, Gospodarowicz MK, Christian W. TNM classification of malignant tumors. 7th. Oxford, USA: Wiley-Blackwell; 2009.
- Xu YW, Peng YH, Chen B, Wu Z-Y, Wu J-Y, Shen J-H, Zheng C-P, Wang S-H, Guo H-P, Li E-M, et al. Autoantibodies as potential biomarkers for the early detection of esophageal squamous cell carcinoma[J]. Am J Gastroenterol. 2014;109:36–45. doi:10.1038/ajg.2013.384.
- Zhang HF, Qin JJ, Ren PF, Shi J-X, Xia J-F, Ye H, Wang P, Song C-H, Wang K-J, Zhang J-Y, et al. A panel of autoantibodies against multiple tumor-associated antigens in the immunodiagnosis of esophageal squamous cell cancer[J]. Cancer Immunol Immunother. 2016;65:1233–1242. doi:10.1007/s00262-016-1886-6.
- Le XXN, Chunyang S, Guoli Y. Application of SEREX method in the identification of tumor-associated antigens[J]. Science and Technology of West China, 2009, 8: 56–57
- Gao Hongjun Zhao Xiaohang. SERPA strategy and its application in tumour-associated antigens[J]. Medical Recapitulate, 2010, 16: 2853–2854
- Kimple AJ, Bosch DE, Giguere PM, Siderovski DP. Regulators of G-protein signaling and their Galpha substrates: promises and challenges in their use as drug discovery targets[J]. Pharmacol Rev. 2011;63:728–749. doi:10.1124/pr.110.003038.
- Xiao R, Sun Y, Ding JH, Lin S, Rose DW, Rosenfeld MG, Fu X-D, Li X. Splicing regulator SC35 is essential for genomic stability and cell proliferation during mammalian organogenesis[J]. Mol Cell Biol. 2007;27:5393–5402. doi:10.1128/mcb.00288-07.
- Parish AJ, Nguyen V, Goodman AM, Murugesan K, Frampton GM, Kurzrock R. GNAS, GNAQ, and GNA11 alterations in patients with diverse cancers[J]. 2018, 124: 4080–4089.doi:10.1002/cncr.31724
- Terada T. Primary esophageal small cell carcinoma with brain metastasis and with CD56, KIT, and PDGFRA expressions[J]. Pathol Oncol Res. 2012;18(4):1091–1093. doi:10.1007/s12253-011-9374-y.
- Gong L, Govan JM, Evans EB, Dai H, Wang E, Lee S-W, Lin H-K, Lazar AJ, Mills GB, Lin S-Y, et al. Nuclear PTEN tumor-suppressor functions through maintaining heterochromatin structure[J]. Cell Cycle. 2015;14(14):2323–2332. doi:10.1080/15384101.2015.1044174.
- Shen WH, Balajee AS, Wang J, Wu H, Eng C, Pandolfi PP, Yin Y. Essential role for nuclear PTEN in maintaining chromosomal integrity[J]. Cell. 2007;128(1):157–170. doi:10.1016/j.cell.2006.11.042.
- Wu M, Mao C, Chen Q, Cu X-W, Zhang W-S. Serum p53 protein and anti-p53 antibodies are associated with increased cancer risk: a case-control study of 569 patients and 879 healthy controls[J]. Mol Biol Rep. 2010;37:339–343. doi:10.1007/s11033-009-9744-7.
- Kollias HD, McDermott JC. Transforming growth factor-beta and myostatin signaling in skeletal muscle[J]. J Appl Physiol. 2008;104(1985):579–587. doi:10.1152/japplphysiol.01091.2007.
- Chen D, Ma T, Liu XW, Liu Z. CASP-8-652 6N ins/del polymorphism and cancer risk: A literature-based systematic HuGE review and meta-analysis[J]. Exp Ther Med. 2012;4:762–770. doi:10.3892/etm.2012.661.
- Horvilleur E, Sbarrato T, Hill K, Spriggs RV, Screen M, Goodrem PJ, Sawicka K, Chaplin LC, Touriol C, Packham G, et al. A role for eukaryotic initiation factor 4B overexpression in the pathogenesis of diffuse large B-cell lymphoma[J]. Leukemia. 2014;28(5):1092–1102. doi:10.1038/leu.2013.295.
- Chandrasekharappa SC, Guru SC, Manickam P, Olufemi SE, Collins FS, Emmert-Buck MR, Debelenko LV, Zhuang Z, Lubensky IA, Liotta LA, et al. Positional cloning of the gene for multiple endocrine neoplasia-type 1[J]. Science. 1997;276:404–407. doi:10.1126/science.276.5311.404.
- Dai L, Lei N, Liu M, Zhang J-Y. Autoantibodies to tumor-associated antigens as biomarkers in human hepatocellular carcinoma (HCC)[J]. Exp Hematol Oncol. 2013:2. doi:10.1186/2162-3619-2-15.
- Dai L, Tsay JC, Li J, Yie T-A, Munger JS, Pass H, Rom WN, Zhang Y, Tan EM, Zhang J-Y, et al. Autoantibodies against tumor-associated antigens in the early detection of lung cancer[J]. Lung Cancer. 2016;99:172–179. doi:10.1016/j.lungcan.2016.07.018.
- Zhou SL, Yue WB, Fan ZM, Du F, Liu BC, Li B, Han XN, Ku JW, Zhao XK, Zhang P, et al. Autoantibody detection to tumor-associated antigens of P53, IMP1, P16, cyclin B1, P62, C-myc, Survivn, and Koc for the screening of high-risk subjects and early detection of esophageal squamous cell carcinoma[J]. Dis Esophagus. 2014;27:790–797. doi:10.1111/dote.12145.
- Timmerman D, Testa AC, Bourne T, Ferrazzi E, Ameye L, Konstantinovic ML, Calster BV, Collins WP, Vergote I, Huffel SV, et al. Logistic regression model to distinguish between the benign and malignant adnexal mass before surgery: a multicenter study by the International ovarian tumor analysis group[J]. J Clin Oncol. 2005;23:8794–8801. doi:10.1200/jco.2005.01.7632.