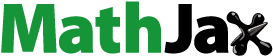
ABSTRACT
Prostate cancer (PCa) immunotherapy has shown limited efficacy so far, even in advanced-stage cancers. The success rate of PCa immunotherapy might be improved by approaches more adapted to the immunobiology of the disease. The objective of this study was to perform a multi-omics analysis to identify immune genes associated with PCa progression to better characterize PCa immunobiology and propose new immunotherapeutic targets. mRNA, miRNA, methylation, copy number aberration, and single nucleotide variant datasets from The Cancer Genome Atlas PRAD cohort were analyzed after filtering for genes associated with immunity. Sparse partial least squares-discriminant analyses were performed to identify features associated with biochemical recurrence (BCR) in each type of omics data. Selected features predicted BCR with a balanced error rate (BER) of 0.20 to 0.51 in single-omics and of 0.05 in multi-omics analyses. Amongst features associated with BCR were genes from the Immunoglobulin Ig-like Receptor (LILR) family which are immune checkpoints with immunotherapeutic potential. Using Multivariate INTegrative (MINT) analysis, the association of five LILR genes with BCR was quantified in a combination of three RNA-seq datasets and confirmed with Kaplan-Meier analysis in both these and in an independent RNA-seq dataset. Finally, immunohistochemistry showed that a high number of LILRB1 positive cells within the tumors predicted long-term adverse outcomes. Thus, tumors characterized by abnormal expression of LILR genes have an elevated risk of recurring after definitive local therapy. The immunotherapeutic potential of these regulators to stimulate the immune response against PCa should be evaluated in pre-clinical models.
Introduction
Prostate cancer (PCa) immunotherapy has been mostly attempted with therapeutic anti-cancer vaccines using either dendritic cell-based, whole cell-based, or vector-based vaccines as well as with some other approaches but always with limited efficacy.Citation1–3 In recent years, the development of immune checkpoint inhibitors has revolutionized cancer immunotherapy. Immune checkpoints are a series of receptors/ligands that either inhibit or activate the function of immune cells.Citation4 CTLA-4 and PD-1 or its ligand PD-L1 are the most well-known immune checkpoints but several others have been identified.Citation5,Citation6 The inhibition of CTLA-4 and PD-1/PD-L1 has shown impressive anti-tumor activity in cancers such as melanoma, lung, kidney, and bladder cancers and efforts are being made to improve their efficacy, notably through the identification of biomarkers for the selection of patients more likely to respond or through combination with other drugs or therapies.Citation7
Initial attempts of PCa immunotherapy using immune checkpoint inhibitors have been however rather disappointing. Two Phase III trials testing Ipilimumab (anti-CTLA-4) for the treatment of metastatic castrate-resistant prostate cancer (CRPC) showed no effect on overall survival compared to placebo although it had some positive impact on progression-free survival.Citation8,Citation9 Phase I and II trials testing PD-1 or PD-L1 inhibitors have also been conducted. These studies showed that a higher anti-tumor activity could be observed in subsets of patients with tumors showing DNA repair abnormalities, inactivating CDK12 mutations or when the inhibitors were used in combination with other drugs such as enzalutamide or olaparib.Citation10–12 Although these results are encouraging, PCa immunotherapy is still suboptimal, and more effective approaches must be identified. A better understanding of the antitumor immune defects associated with PCa progression will help to develop immunotherapies more adapted to this disease.
To fill this gap, we performed a multi-omics analysis using The Cancer Genome Atlas (TCGA) PCa datasets to identify immune-related features associated with biochemical recurrence (BCR; i.e a rise in PSA level after local therapy) with the presumption that the identification of features common to different types of omics data would support their relevance and importance in the immunobiology of PCa. These analyses led us to identify some leukocyte immunoglobulin-like receptors (LILR) as candidate biomarkers of BCR.
LILR is a family of immune receptors that either activate (LILRA members) or suppress (LILRB members) immune cell functions. These Type 1 transmembrane glycoproteins are composed of two or four extracellular Ig-like domains that bind ligands and either a short cytoplasmic tail with an immunoreceptor tyrosine-based activation motif (ITAM), for the LILRA members of the family, or a long cytoplasmic tail with an immunoreceptor tyrosine-based inhibitory motif (ITIM), for the LILRB members of the family.Citation13,Citation14 These receptors are widely expressed in hematopoietic-lineage cells but their exact function is still not well understood. LILRA and LILRB have been shown to bind to various ligands including membrane-bound proteins such as MHC class I molecules (most strongly with HLA-G molecules) and soluble proteins such as angiopoietin-like proteins (ANGPTLs), myelin inhibitors, and S100A8/9 proteins.Citation14 LILR up- or downregulation was shown to impact the response to bacterial and viral infections as well as to influence the outcomes in diseases such as autoimmunity, inflammatory diseases, and cancer.Citation15
We report here the details of our multi-omics analyses and discuss the potential of LILR and especially LILRB1 as targets for PCa immunotherapy.
Results
Eligibility and preparation of data
The TCGA PRAD project comprises 498 men treated with radical prostatectomy. From these cases a large number are inadequate in terms of quality of RNA sequencing as indicated by the TCGA PRAD team.Citation16 As our objective was to identify features associated with BCR which may happen several years after radical prostatectomy, we selected cases with a minimum of 5 years of clinical follow-up and combined them with those cases where BCR happened before 5 years. We, therefore, discarded several cases that did not encounter our eligibility criteria (see details in Material and Methods). This imposed an important selection as only 45 cases were conserved for this analysis (Supplementary Figure S1). The resulting cohort is enriched in high-risk PCa which may help to identify features associated with BCR (Supplementary Table S1).
mRNA, miRNA, methylation, CNA, and SNV datasets from the TCGA PRAD project were downloaded and curated. Non-informative data in each dataset were discarded. RNA-seq data were completely reanalyzed. The numbers of CNA and SNV data were considerably reduced by the selection of features. Following this first step, the number of RNA, miRNA, methylation, CNA, and SNV features were 29,820, 1,211, 20,112, 13,925, and 928, respectively. We next applied our filter to select features associated with a set of 812 immune genes. After filtering for immune genes the resulting number of RNA, miRNA, methylation, CNV, and SNV features were 768, 1,211, 768, 138, and 6, respectively (Supplementary Figure S2).
Features associated with BCR
To identify immune gene-related features associated with BCR, prediction modeling was performed using sparse partial least squares-discriminant analysis (sPLS-DA). These analyses were first performed on each data type separately. Supplementary Table 2 provides the list of features identified by sPLS-DA in each omics dataset. Overall, 51 mRNA, 44 miRNA, 36 methylation loci, 32 CNA, and 6 SNV were identified. The selected mRNA, miRNA, and methylation loci predicted well occurrence of a BCR with balanced error rate (BER) of 0.20, 0.23, and 0.26, respectively, while the selected CNA and SNV, with BER of 0.46 and 0.51, respectively, did not predict as well BCR (). We next merged all those features into one single set of data and performed a general sPLS-DA analysis. This resulted in an almost perfect prediction of BCR with a BER of 0.05 ().
Figure 1. Results of sPLS-DA in individual omics datasets from TCGA PRAD. To identify immune-related features associated with BCR, prediction modeling was performed using sPLS-DA. Overall, 51 mRNA, 44, miRNA, 36 methylation loci, 32 CNA and 6 SNV were identified. The selected mRNA, miRNA and methylation loci predicted well BCR with BER of 0.20, 0.23 and 0.26, respectively. With BER of 0.46 and 0.51, the selected CNA and SNV, respectively, did not predict well BCR
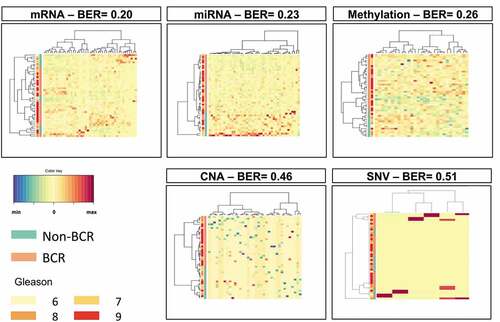
Figure 2. Results of sPLS-DA of the combined omics datasets from TCGA PRAD. Following the sPLS-DA of individual omics datasets, we merged those features into one single set of data and performed a general sPLS-DA analysis. This resulted in an almost perfect prediction of BCR with a BER of 0.05
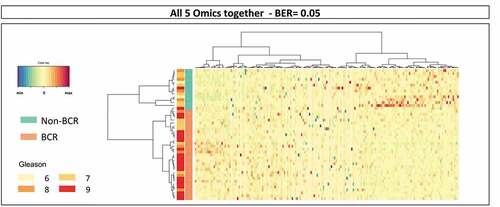
Analysis of mRNA selection showed that many features were associated with leukocyte activation, cell activation, regulation of catalytic activity, immune system process, intracellular signal transduction, etc. (Supplementary Figure S3). Among these were some features associated with antigen processing and presentation that were also retrieved in the other types of omics data. These comprised predominantly HLA molecules, killer-cell immunoglobulin-like receptor (KIR), and leukocyte immunoglobulin-like receptor (LILR) genes (Supplementary Table S2). Since LILR are a family of immune regulators that are showing some potential as targets for cancer immunotherapy, we further analyzed the association between 30 LILR gene-associated features and BCRCitation17,Citation18 (Supplementary Table S3). As shown in , the 30 LILR gene-associated features alone could predict BCR in sPLS-DA analysis with a BER of 0.34 suggesting a role of this family of receptors in the progression of PCa. Similar analyses were performed with features associated with the KIR genes or the HLA genes, alone or in combination with those of LILR genes. These sPLS-DA resulted in BER that were higher than 0.34 indicating that genes of the LILR family alone were the most strongly associated with BCR (results not shown).
Figure 3. Results of sPLS-DA of LILR gene-related features in the combined omics dataset of TCGA PRAD. The analysis of the 30 LILR gene-related features in the combined dataset resulted in the prediction of BCR with a BER of 0.34
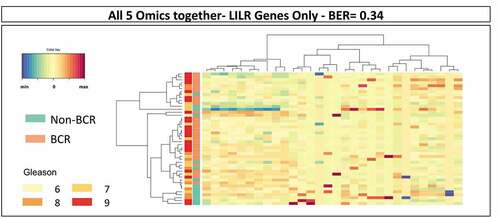
To further validate the association of LILR genes with BCR and because of the paucity of PCa multiomics datasets besides TCGA, we sought to validate this association in a combination of RNA-seq datasets of PCa that would represent more than 150 patient tumors to ensure statistical power. Therefore, we selected the GSE54460 RNA-Seq dataset from Long et al.Citation19 and an RNA-seq dataset from VPCCCitation20 with the objective to combine them with RNA-seq from the 52 selected cases of the TCGA PRAD project. We reanalyzed these new data in the same way to ensure proper assembly of the datasets (Supplementary Figure S4). Thereafter, we used the Multivariate INTegrative (MINT) approach to assess whether the LILR genes were associated with BCR in the combined RNA-seq dataset of 171 tumors (Supplementary Table S4). shows that five genes from the family of LILR genes (LILRB1, LILRB2, LILRB3, LILRB5, and LILRA3) were associated with BCR with a BER of 0.34 confirming an association between LILR genes and BCR.
Figure 4. Results of the MINT analysis. Using the combined TCGA-GSE54460-VPCC RNA-seq dataset, five LILR genes were found to be associated together with BCR. The five LILR genes were associated with BCR with a BER of 0.34
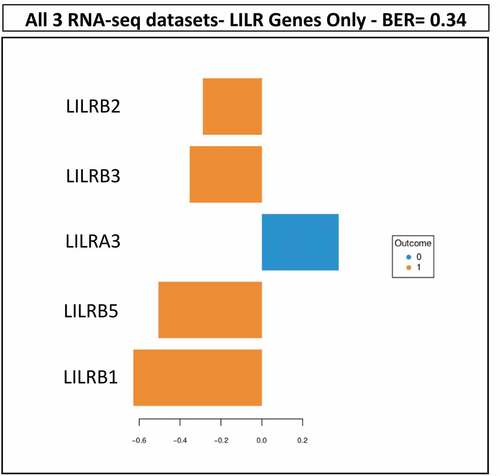
To further characterize the association of these LILR genes with BCR, we performed Kaplan-Meier analyses. shows that, as revealed by the MINT analysis, LILRB1 is a gene that is strongly associated with BCR as a high level of LILRB1 mRNA is associated with shorter BCR-free survival (); log-rank p < .0001). High level of LILRB2 mRNA is also associated with shorter BCR-free survival but this association is less significant (); log-rank p = .04). A high level of LILRB5 mRNA is nearly significantly associated with a shorter BCR-free survival (); log-rank p = .06) while that of LILRB3 mRNA alone is not associated with BCR-free survival (); log-rank p = .27). When the mRNA levels of these four genes are summed, the association with BCR-free survival is not better (); log-rank p = .008) than that of LILRB1 mRNA alone indicating that LILRB1 is the main driver of the association. Moreover, removing LILRB1 from the combination greatly affects the significance of the association (not shown), supporting the importance of LILRB1. At the opposite, a high level of LILRA3 mRNA was significantly associated with better BCR-free survival (); log-rank p = .003).
Figure 5. Kaplan-Meier analysis of dichotomized LILRB1 (a), LILRB2 (b), LILRB3 (c), LILRB5 (d) and LILRA3 (f) mRNA levels in the combined TCGA-GSE54460-VPCC RNA-seq dataset. High levels of LILRB mRNA have a tendency to be associated with shorter BCR-free survival but only LILRB1 and LILRB2 can predict BCR with a significant p value. The sum of the levels of these mRNA (e) was also associated with a significantly shorter BCR-free survival. At the opposite, higher level of LILRA3 mRNA (f) is associated with a higher BCR-free survival
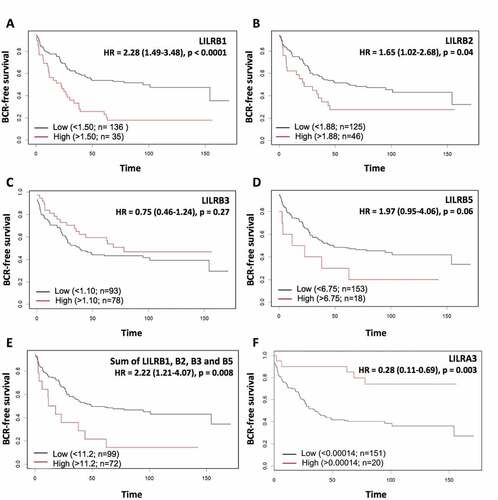
As the combined TCGA-GSE54460-VPC cohort is composed of high-risk tumors, we next sought to determine whether the association of these genes with BCR was maintained in a cohort of intermediate-risk PCa samples. We, therefore, performed new Kaplan-Meier analyses using a sub-cohort of the CPC-GENE projectCitation21 comprising 144 men operated at our institution (Supplementary Table S5). shows that high levels of LILRB2, LILRB3, and LILRB5 mRNA were significantly associated with shorter BCR-free survival. However, LILRB1 mRNA level was not significantly associated with BCR in this cohort although a trend can be observed. LILRA3 mRNA level was not quantified in the CPC-GENE dataset; therefore, the association of this gene with BCR could not be assessed. When taken together, the sum of the levels of LILRB1, LILRB2, LILRB3, and LILRB5 mRNA was associated with BCR (); log-rank p = .009).
Figure 6. Kaplan-Meier analysis of dichotomized LILRB1 (a), LILRB2 (b), LILRB3 (c), and LILRB5 (d) mRNA levels in the CPC-GENE RNA-seq dataset. High mRNA levels of LILRB2, LILRB3, LILRB5 genes, but not that of LILRB1, were significantly associated with shorter BCR-free survival. The sum of these mRNA levels (e) was also associated with a significantly shorter BCR-free survival (HR = 2.51, p = 0,009). The association of LILRA3 mRNA level with BCR could not be analyzed as there was no LILRA3 mRNA data in the CPC-GENE dataset
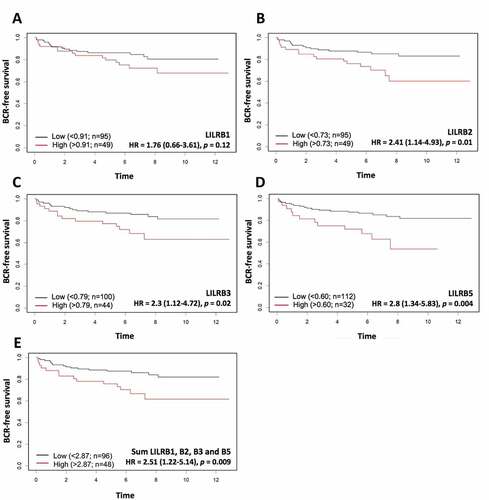
The absence of a statistically significant association of LILRB1 mRNA level with BCR in the intermediate-risk cohort suggests that LILRB1 gene expression could be associated with grade. Spearman correlation analysis showed indeed that LILRB1 mRNA level was correlated with grade in the TCGA-GSE54460-VPCC cohort (rs = 0.53, p = .01; Supplementary Table S6), while the mRNA levels of LILRB2, LILRB3, LILRB5, and LILRA3 were not associated with grade in the combined cohort. To further assess the association of the LILRB1 gene with BCR, we analyzed the expression of LILRB1 protein in a series of 20 high-risk prostate tumors by immunohistochemistry. LILRB1 protein was found on immune cells scattered between tumor glands (Supplementary Figure S5 and Table S7). No tumor cells or stromal cells expressed the protein. In Kaplan-Meier analyses, a high level of LILRB1+ cells infiltrating the tumor was found to be associated with poor clinical outcomes such as the need for definitive androgen deprivation therapy (); log-rank 0 = 0.009) and having lethal PCa defined as PCa that has already led to death or metastatic castration-resistant PCa that will eventually lead to death by PCa.
Figure 7. Kaplan-Meier curves of BCR-free (a), ADT-free (b) and lethal PCa-free (c) survival according to high (level 3) vs low (levels 1–2) levels of LILRB1+ cells in the tumor area of high-risk PCa samples. The number of LILRB1+ cells in the tumor area of 20 formalin-fixed and paraffin-embedded T2-T3 stage prostate tumor samples with long clinical follow-up was analyzed using immunohistochemistry. Expression of LILRB1 was classified as level 1, 2 and 3 corresponding to low, intermediate and high number of positive cells. Statistical significance was determined by the log-rank test
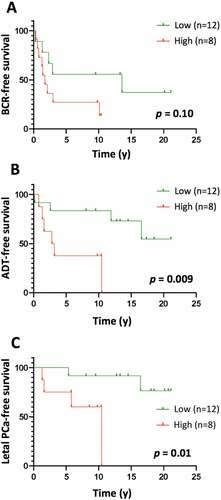
Discussion
PCa immunotherapy using the first-generation immune checkpoint inhibitors (i.e. against CTLA-4 and PD-1/PD-L1) has shown poor success in initial clinical trials. Some explanations for this might be the limited immunogenicity of PCa cells or immunosuppressive mechanisms other than those involving these major immune checkpoints.Citation22 To explore the immunobiology of PCa, we performed a bioinformatic analysis using different types of omics data to identify immune-related features associated with the first event of PCa progression, i.e. the BCR consisting in an elevation of serum PSA after local therapy. We hypothesized that the identification of biological features selected in different types of omics would support their biological relevance and might provide candidate molecular targets for immunotherapeutic intervention.
To perform these multi-omics analyses involving large datasets we used for variable selection sPLS-DA, a multivariate exploratory approach, that provides more insight into cell biology, biological pathways, or complex traits than other commonly used approaches such as machine learning approaches.Citation23 We first used this approach on the TCGA PRAD data within each type of omics to select features related to BCR and then we merged the selected features and applied the same approach on all the selected features. BCR was used as the clinical outcome of PCa progression instead of late outcomes associated with aggressive cancer such as detection of metastases or PCa-specific death. A major limitation of our approach is the size of the cohort available after the selection based on quality criteria. As reported, the TCGA PRAD cohort has a very short median follow-up which limits the correlation analyses between genomic features and clinical outcomes, especially the late ones. In order to increase the accuracy of the association between features and BCR, we selected 5 years as a minimal follow-up knowing that it would considerably reduce the size of the final cohort.
The sPLS-DA led to the identification of an association between a series of genes involved in antigen presentation and regulation of immune cell activity and PCa progression. HLA antigens, KIR, and LILR receptors form a complex system of molecules involved in the recognition of self/non-self antigens which can have an impact on various immunological responses and impact, for example the outcome of viral infections, and diseases such as autoimmunity and cancer.Citation13,Citation24 Amongst these, LILRs were those that had the smallest BER associated with BCR. The association was further demonstrated in the combined RNA-seq datasets of 171 tumors using the MINT method which revealed an association between the sum of LILRB1, LILRB2, LILRB3, LILRB5, and LILRA3 mRNA levels and BCR. Kaplan-Meier analyses further confirmed this association and identified LILRB1 as the gene with the strongest association with BCR. However, validation in a cohort of intermediate-risk PCa with very few high-grade tumors showed no significant association of LILRB1 mRNA level with BCR while an association was observed for LILRB2, LILRB3, and LILRB5 mRNA levels. The absence of a significant association of LILRB1 mRNA level with BCR in this cohort is concordant with the association of LILRB1 mRNA level with Gleason grade observed in the combined TCGA-GSE54460-VPCC cohort (Supplementary Table S5). This positive relationship with grade might be explained by the fact that LIlRB1 is known to be predominantly expressed in macrophages and higher levels of M2 macrophages have been shown to be positively associated with Gleason grade and worst outcome.Citation25,Citation26 Immunohistochemistry analysis of 20 high-risk tumors supported this association with adverse long-term outcomes. Multi-parametric analyses would be needed to confirm whether the immune cells expressing LILRB1 are indeed macrophages.
In cancer, LILRBs and especially LILRB1 immunosuppressive activity have been shown to contribute to cancer evasion. For example, Raji cells proliferation was proportionally inhibited by increasing amounts of HLA-G aggregated on nanoparticles and this inhibition was reversed when LILRB1 expression was inhibited using small interfering RNA or antagonistic mAb demonstrating that HLA-G inhibition is depending on LILRB1 expression.Citation14 The immunosuppressive function of the HLA-G/LILRB1 signaling pathway has led to the identification of LILRB1 and HLA-G as new immune checkpoints that are potential targets for immunotherapy.Citation27 More recently the LILRB1/MHC-I signaling pathway was identified as a second “Don’t Eat Me” signal in tumor-associated macrophages.Citation17 Studies of the primary “Don’t Eat Me” signal, the CD47/SIRP-- signaling pathway, showed that inhibition of LILRB1 or MHC-I molecules potentiates the phagocytosis of tumor cells by macrophages in a manner that is independent of the inhibition of the CD47/SIRP-
axis.Citation17 Further analysis of the LILRB1/MHC-1 pathway may lead to the development of therapies to help restore macrophage function in the tumor microenvironment. Such therapies could complement the CD47/SIRP-
-based therapies that are already showing great potential in pre-clinical and early clinical studiesCitation28,Citation29.
In conclusion, we performed a multi-omics analysis using PCa datasets that led us to identify a series of immune features that all together were strongly associated with BCR. Further analysis of these features allowed us to identify some candidate molecular targets that could be prioritized for immunotherapeutic intervention in PCa. Our data point toward a role for LILRB molecules and especially LILRB1 and suggest that these receptors could play a role in the resistance of PCa to anti-tumor immune response. Immunotherapeutic interventions aiming at the inhibition of the LILRB1/MHC-I pathway alone or in combination with therapies targeting complementary pathways (e.g. CD47/SIRP-; PD-1/PD-L1, etc.) may provide a more adapted immunotherapeutic treatment to PCa immunobiology and would hopefully lead to better clinical response. Testing this approach in pre-clinical models to assess the immunotherapeutic potential of LILRB1 inhibition to stimulate an immune response against PCa may be of significant promise.
Material and methods
Patients and datasets
This study was approved by the Research Ethics Committee of the CHU de Québec-Université Laval (Project 2018–3670). Next-generation sequencing (NGS) RNA-seq, miRNA, methylation, copy number aberration (CNA), and single nucleotide variants (SNV) data from the TCGA PRAD project (498 samples) along with their associated clinical data were downloaded from the Genomic Data Commons (GDC) portal (https://portal.gdc.cancer.gov/). The GSE54460 RNA-Seq and clinical data (106 samples) published by Long et al.Citation19 were downloaded from the Gene Expression Omnibus (GEO) website (https://www.ncbi.nlm.nih.gov/geo/query/acc.cgi?acc=GSE54460). The Vancouver Prostate Cancer Center (VPCC) RNA-Seq and clinical data (85 samples) were provided by C. Collins (University of British Columbia, Vancouver, BC, Canada).Citation20 A sub-cohort from the Canadian Prostate Cancer Genome Network (CPC-GENE) (n = 144) was used as a validation cohort.Citation19 This cohort corresponds to men that were operated in our institution. Data were downloaded from https://ega-archive.org/. All patients had localized disease and were treated by radical prostatectomy. For each patient, available clinical data comprised at least the pathological characteristics of the tumor (grade and stage), PSA level at diagnosis, the occurrence of BCR, the time between radical prostatectomy and BCR, the occurrence of death, and date of death as well as the date of the last follow-up.
Eligibility criteria
Eligibility was set by criteria of minimal quality. The TCGA PRAD project comprised of 498 participants. However, according to the TCGA Research Network, 131 participants must be omitted because of excessive RNA degradation.Citation16 The TCGA cohort is also characterized by a short follow-up. Patients with less than 60 months of follow-up were discarded. We also ignored tumors with less than 40% of the tumor cell content. Patients treated with neoadjuvant or concomitant hormonal therapy were not conserved for the study. The same selection criteria were applied to GSE54460, VPCC, and CPC-GENE cohorts except for the selection based on the percentage of tumor cell content as this information was not provided.
OMIC data processing
RNA-Seq data
The RNA-Seq data were completely re-analyzed to avoid variability in the data processing. The use of a common pipeline of analysis ensures the accuracy of integrative analyses of transcriptomic datasets. The quality of the raw FASTQ files was assessed using FastQCCitation30 (v0.11.5) and trimmed with TrimmomaticCitation31 (v0.32). A threshold quality per base of 30 (based on Phred 33) and a minimal length of 40 bases was necessary otherwise the read was not conserved for analysis. The sequences were then mapped and quantified (pseudo-alignment) on GRCh38.p7 using Kallisto (v0.43.0, default parameters, provided index).Citation32 Kallisto provides isoform counts, adjusted for the amount of bias in the experiment to ensure a coherent non-naive mapping; consequently, gene counts were computed with tximport.Citation33 The Ensembl Gene ID was converted with Biomart toolsCitation34,Citation35 from transcript ID to gene ID. The RNA-seq counts were then normalized to negative control genes (housekeeping genes) using the RUVg method.Citation36,Citation37 In order to perform this normalization, we selected from the literature a series of six housekeeping genes that could be candidates for control reference genes in PCa experiments.Citation38–41 These genes were: RRN18S, ACTB, PPIA, GAPDH, PGK1, and GUSB. The expression of these genes was tested by RT-qPCR in a series of 50 prostate tumors and they were shown to be stably expressed between tumor samples (data not shown). However, we excluded from the final list the ribosomal gene RRN18S because ribosomal RNAs were removed from our RNA-seq datasets. We also excluded PGK1 as it was shown that hypoxia in PCa alters the RNA abundance of this gene.Citation38 Therefore, we finally used GUSB, PPIA, GAPDH, and ACTB as negative control genes for the normalization of the counts. The same process was applied to GSE554460 and VPCC RNA-seq datasets. The dataset corresponding to 144 tumors from the CPC-GENE cohort was used for validation. The mRNA expression data were not processed as were the data from TCGA, GSE54460, and VPCC. FASTQ files were downloaded and directly used for statistical analyses.
miRNA data
The level 3 NGS miRNA data from the TCGA PRAD project were provided as normalized counts in reads-per-million-miRNA-mapped. miRNAs with a normalized count of zero or with no value were removed from the miRNA dataset. mirWalk 2.0,Citation42 which relies on different databases (mirTarBase, mirDB, and TargetS), was used to assign genes to miRNA according to predicted target genes.
Methylation data
Genome-wide methylation data from the TCGA PRAD project were generated using the Illumina Infinium HumanMethylation27 and HumanMethylation450 BeadChip platform (https://docs.gdc.cancer.gov/Data/Bioinformatics_Pipelines/Methylation_LO_Pipeline/). The level 3 methylation data were used and Beta (ß) values selected. ß values (0 for an unmethylated allele to 1 for fully methylated allele) are the estimate of methylation level using the ratio of intensities between methylated and unmethylated alleles. Genes with no ß values were removed from the dataset.
CNA data
The level 3 CNA data from the TCGA PRAD project were preprocessed using BirdsuiteCitation23 from the Broad Institute and the R package DNAcopy (v1.44).Citation43 The data were cleaned, normalized, segmented, and log transformed. From these data, we created an index with all unique regions found. These regions were annotated with ChipSeekerCitation44 which associates the closest genomic object to the region’s coordinates.
SNV data
The level 3 SNV data from the TCGA were retrieved and processed with the VCFtools suite.Citation45 An index with the mutation found in all patients at the base level was created and all unique mutations were kept as final variables. For each mutation, we kept the information of location (chromosome and coordinates) and mutation type.
Set of immune genes
The multivariate analyses were focused on immune genes. The set of selected immune genes for these analyses is composed of 812 Ensembl genes. This set of genes was derived from a meta-analysis that targeted the anti-genome of tumor cells in interaction with the immune system.Citation46
Statistical analyses
To identify features associated with BCR in every set of omics, sparse partial least square-discriminant analysis (sPLS-DA) models were calculated using the mixOmics package.Citation23 In each sPLS-DA analysis, the BCR was defined as the Y response. The Mfold validation strategy with a fold of 5 and 200 repetitions was used to ensure the stability of the model. To assess the predictive potential of mixed omics data, the selected omics features were merged and again sPLS-DA were calculated as above. The association between selected features and BCR was further analyzed by multivariate regression analysis in three RNA-seq datasets (i.e. TCGA, GSE54460, and VPCC) using the Multivariate INTegrative (MINT)Citation47 method from the mixOmics package. Again, the Mfold validation strategy with a fold of 5 and 200 repetitions was used as in the sPLS-DA analyses. Within all R (v3.3 to 3.6) analyses, the seed parameter was defined as 2543 for reproducibility. Code used for the PLS-DA model can be found here http://mixomics.org/methods/pls-da/and the one for the MINT model can be found here http://mixomics.org/mixmint/stemcells-example/.
The balanced error rate (BER = 1–0.5*(sensitivity + specificity)) measured at the centroid distance was used in sPLS-DA and MINT analyses to assess the quality of the association between the BCR and the omic features. A BER of 0 means a perfect classification while a BER over 0.5 means no association with the response variable for binary classification problems. We considered a BER<0.4 as a good score value.
To perform Kaplan-Meier survival analysis, the expression data for each mRNA of interest were optimally dichotomized using the Cutoff finder tool.Citation48 The method fitting Cox proportional hazard models to dichotomize variable was used to define a threshold abundance value. Then the survival (v2.41–3) and survminer (v0.4.1) packages were used to perform the survival analysis within R.
Immunohistochemistry
Analysis of LILRB1 expression was performed on prostate tumors obtained from our local biobank URO-1. This analysis was approved by the Research Ethics Committee of the CHU de Québec-Université Laval (Project 2012–1059). Briefly, 5-µm-thick sections of formalin-fixed and paraffin-embedded tumors were deparaffinized and submitted to heat-induced antigen retrieval (97°C, 20 min) in Tris/EDTA, pH 9 (Dako Code K8004: EnVision™ FLEX, High pH buffer) using a PT Link, Pre-Treatment Module for Tissue Specimens (Dako, Burlington, ON, Canada). Endogenous peroxidases were blocked by incubation in 3% peroxide solution for 10 min. Bound antibodies were revealed using the IDetect super stain HRP polymer kit (ID labs, London, Ontario, Canada) as follows. Slides were initially incubated for 10 minutes at room temperature with Super block solution to avoid nonspecific background staining and then with anti-LILRB1 rabbit monoclonal antibody (mAb)(clone EPR21007, dilution 1:500, Abcam, Toronto, ON) during 1 h at room temperature. After washes, slides were incubated for 30 min with HRP-Polymer Conjugate according to manufacturer’s recommendations. Final revelation was performed by a 5 min of incubation with DAB. Finally, slides were rinsed, counterstained with hematoxylin, dehydrated, and mounted with a coverslip using MM 24 low viscosity mounting medium (Leica Microsystems, Durham, USA). Slides were digitized using a Nanozoomer (Hamamatsu Photonics, Bidgewater NJ, USA) and visualized using the NDP.view2 software (Hamamatsu Photonics). Scoring of the relative number of positive cells in the tumor area was performed by a trained technician and was reported on a scale from 1 to 3.
Author contribution
BV, YF, AB, ML, and AD conceived the study. YF, AB, and AD supervised the study. BV performed all bioinformatics and statistical analyses. JL and PCB performed some bioinformatic analyses. OEM performed the analysis of housekeeping gene expression. OEM, XPL, HH, and VP were involved in the immunohistochemistry analyses. AB, ML, MLMM, PB, CC, YF, and AD interpreted data and provided advice. BV, AB, and ML wrote the manuscript. All authors critically reviewed the manuscript.
Supplemental Material
Download ()Acknowledgments
The authors would like to thank Fan Mo and Antonio Hurtado-Coll for the preparation of the data from VPCC.
Disclosure statement
No potential conflicts of interest were disclosed.
Supplementary material
Supplemental data for this article can be accessed on the publisher’s website.
Additional information
Funding
References
- Comiskey MC, Dallos MC, Drake CG. Immunotherapy in prostate cancer: teaching an old dog new tricks. Curr Oncol Rep. 2018;20:75. doi: 10.1007/s11912-018-0712-z.
- Bilusic M, Madan RA, Gulley JL. Immunotherapy of prostate cancer: facts and hopes. Clin Cancer Res Off J Am Assoc Cancer Res. 2017;23:6764–11. doi:10.1158/1078-0432.CCR-17-0019.
- Scheid E, Major P, Bergeron A, Finn OJ, Salter RD, Eady R, Yassine-Diab B, Favre D, Peretz Y, Landry C, et al. Tn-MUC1 DC vaccination of rhesus macaques and a phase I/II trial in patients with nonmetastatic castrate-resistant prostate cancer. Cancer Immunol Res. 2016;4:881–892. doi:10.1158/2326-6066.CIR-15-0189.
- Wei SC, Duffy CR, Allison JP. Fundamental mechanisms of immune checkpoint blockade therapy. Cancer Discov. 2018;8:1069–1086. doi:10.1158/2159-8290.CD-18-0367.
- Dempke WCM, Fenchel K, Uciechowski P, Dale SP. Second- and third-generation drugs for immuno-oncology treatment-The more the better? Eur J Cancer Oxf Engl 1990. 2017;74:55–72. doi: 10.1016/j.ejca.2017.01.001.
- Marin-Acevedo JA, Dholaria B, Soyano AE, Knutson KL, Chumsri S, Lou Y. Next generation of immune checkpoint therapy in cancer: new developments and challenges. J Hematol Oncol J Hematol Oncol. 2018;11:39. doi:10.1186/s13045-018-0582-8.
- Longo V, Brunetti O, Azzariti A, Galetta D, Nardulli P, Leonetti F, Silvestris N. Strategies to improve cancer immune checkpoint inhibitors efficacy, other than abscopal effect: a systematic review. Cancers. 2019;11(4):539. doi:10.3390/cancers11040539.
- Kwon ED, Drake CG, Scher HI, Fizazi K, Bossi A, van den Eertwegh AJ, Krainer M, Houede N, Santos R, Mahammedi H, et al. Ipilimumab versus placebo after radiotherapy in patients with metastatic castration-resistant prostate cancer that had progressed after docetaxel chemotherapy (CA184-043): a multicentre, randomised, double-blind, phase 3 trial. Lancet Oncol. 2014;15:700–712. doi:10.1016/S1470-2045(14)70189-5.
- Beer TM, Kwon ED, Drake CG, Fizazi K, Logothetis C, Gravis G, Ganju V, Polikoff J, Saad F, Humanski P, et al. Randomized, double-blind, phase III trial of ipilimumab versus placebo in asymptomatic or minimally symptomatic patients with metastatic chemotherapy-naive castration-resistant prostate cancer. J Clin Oncol Off J Am Soc Clin Oncol. 2017;Jan;35(1):40–47. doi:10.1200/JCO.2016.69.1584.
- Jafari S, Molavi O, Kahroba H, Hejazi MS, Maleki-Dizaji N, Barghi S, Kiaie SH, Jadidi-Niaragh F. Clinical application of immune checkpoints in targeted immunotherapy of prostate cancer. Cell Mol Life Sci CMLS. 2020 Oct;77(19):3693-3710. doi: 10.1007/s00018-020-03459-1.
- Markowski MC, Shenderov E, Eisenberger MA, Kachhap S, Pardoll DM, Denmeade SR, Antonarakis ES. Extreme responses to immune checkpoint blockade following bipolar androgen therapy and enzalutamide in patients with metastatic castration resistant prostate cancer. The Prostate. 2020;80(5):407–411. doi:10.1002/pros.23955.
- Isaacsson Velho P, Antonarakis ES. PD-1/PD-L1 pathway inhibitors in advanced prostate cancer. Expert Rev Clin Pharmacol. 2018;11:475–486. doi:10.1080/17512433.2018.1464388.
- Hudson LE, Allen RL. Leukocyte Ig-like receptors - a model for MHC class i disease associations. Front Immunol. 2016;7:281. doi: 10.3389/fimmu.2016.00281.
- Naji A, Menier C, Maki G, Carosella ED, Rouas-Freiss N. Neoplastic B-cell growth is impaired by HLA-G/ILT2 interaction. Leukemia. 2012;26:1889–1892. doi:10.1038/leu.2012.62.
- Brown D, Trowsdale J, Allen R. The LILR family: modulators of innate and adaptive immune pathways in health and disease. Tissue Antigens. 2004;64:215–225. doi: 10.1111/j.0001-2815.2004.00290.x.
- Abeshouse A, Ahn J, Akbani R, Ally A, Amin S, Andry C, Annala M, Aprikian A, Armenia J, Arora A, et al. The molecular taxonomy of primary prostate cancer. Cell. 2015;163(4):1011–1025. doi:10.1016/j.cell.2015.10.025.
- Barkal AA, Weiskopf K, Kao KS, Gordon SR, Rosental B, Yiu YY, George BM, Markovic M, Ring NG, Tsai JM, et al. Engagement of MHC class I by the inhibitory receptor LILRB1 suppresses macrophages and is a target of cancer immunotherapy. Nat Immunol. 2018;19:76–84. doi:10.1038/s41590-017-0004-z.
- Zhao J, Zhong S, Niu X, Jiang J, Zhang R, Li Q. The MHC class I-LILRB1 signalling axis as a promising target in cancer therapy. Scand J Immunol. 2019;90:e12804. doi:10.1111/sji.12804.
- Long Q, Xu J, Osunkoya AO, Sannigrahi S, Johnson BA, Zhou W, Gillespie T, Park JY, Nam RK, Sugar L, et al. Global transcriptome analysis of formalin-fixed prostate cancer specimens identifies biomarkers of disease recurrence. Cancer Res. 2014;74(12):3228–3237. doi:10.1158/0008-5472.CAN-13-2699.
- Wyatt AW, Mo F, Wang K, McConeghy B, Brahmbhatt S, Jong L, Mitchell DM, Johnston RL, Haegert A, Li E, et al. Heterogeneity in the inter-tumor transcriptome of high risk prostate cancer. Genome Biol. 2014;15(8):426. doi:10.1186/s13059-014-0426-y.
- Chen S, Huang V, Xu X, Livingstone J, Soares F, Jeon J, Zeng Y, Hua JT, Petricca J, Guo H, et al. Widespread and functional RNA circularization in localized prostate cancer. Cell. 2019;176(4):831–843.e22. doi:10.1016/j.cell.2019.01.025.
- Bryant G, Wang L, Mulholland DJ. Overcoming oncogenic mediated tumor immunity in prostate cancer. Int J Mol Sci. 2017;18(7):1542. doi:10.3390/ijms18071542.
- Rohart F, Gautier B, Singh A, Cao K-A, Schneidman D. L. mixOmics: an R package for ‘omics feature selection and multiple data integration. PLOS Comput Biol. 2017;13:e1005752. doi:10.1371/journal.pcbi.1005752.
- Ivarsson MA, Michaëlsson J, Fauriat C. Activating killer cell Ig-like receptors in health and disease. Front Immunol. 2014;5:184. doi: 10.3389/fimmu.2014.00184.
- Shimura S, Yang G, Ebara S, Wheeler TM, Frolov A, Thompson TC. Reduced infiltration of tumor-associated macrophages in human prostate cancer: association with cancer progression. Cancer Res. 2000;60:5857–5861.
- Erlandsson A, Carlsson J, Lundholm M, Fält A, Andersson S-O, Andrén O, Davidsson S. M2 macrophages and regulatory T cells in lethal prostate cancer. The Prostate. 2019;79(4):363–369. doi:10.1002/pros.23742.
- Carosella ED, Ploussard G, LeMaoult J, Desgrandchamps F, Systematic A. Review of immunotherapy in urologic cancer: evolving roles for targeting of CTLA-4, PD-1/PD-L1, and HLA-G. Eur Urol. 2015;68:267–279. doi:10.1016/j.eururo.2015.02.032.
- Hayat SMG, Bianconi V, Pirro M, Jaafari MR, Hatamipour M, Sahebkar A. CD47: role in the immune system and application to cancer therapy. Cell Oncol Dordr. 2019;43:19-30. doi:10.1007/s13402-019-00469-5.
- Sikic BI, Lakhani N, Patnaik A, Shah SA, Chandana SR, Rasco D, Colevas AD, O’Rourke T, Narayanan S, Papadopoulos K, et al. First-in-human, first-in-class phase i trial of the anti-CD47 antibody Hu5F9-G4 in patients with advanced cancers. J Clin Oncol Off J Am Soc Clin Oncol. 2019;37:946–953. doi:10.1200/JCO.18.02018.
- Andrews S. 2010. FastQC: a quality control tool for high throughput sequence data. Available online at:http://www.bioinformatics.babraham.ac.uk/projects/fastqc
- Bolger AM, Lohse M, Usadel B. Trimmomatic: a flexible trimmer for Illumina sequence data. Bioinformatics. 2014;30:2114–2120. doi:10.1093/bioinformatics/btu170.
- Bray NL, Pimentel H, Melsted P, Pachter L. Near-optimal probabilistic RNA-seq quantification. Nat Biotechnol. 2016;34:525–527. doi:10.1038/nbt.3519.
- Soneson C, Love MI, Robinson MD. Differential analyses for RNA-seq: transcript-level estimates improve gene-level inferences. F1000Research. 2015;4:1521. doi:10.12688/f1000research.7563.1.
- Smedley D, Haider S, Durinck S, Pandini L, Provero P, Allen J, Arnaiz O, Awedh MH, Baldock R, Barbiera G, et al. The BioMart community portal: an innovative alternative to large, centralized data repositories. Nucleic Acids Res. 2015;43(W1):W589–W598. doi:10.1093/nar/gkv350.
- Kinsella RJ, Kähäri A, Haider S, Zamora J, Proctor G, Spudich G, Almeida-King J, Staines D, Derwent P, Kerhornou A, et al. Ensembl BioMarts: a hub for data retrieval across taxonomic space. Database (Oxford). 2011 Jul 23;2011:bar030. doi: 10.1093/database/bar030.
- Risso D, Ngai J, Speed TP, Dudoit S. Normalization of RNA-seq data using factor analysis of control genes or samples. Nat Biotechnol. 2014;32:896–902. doi:10.1038/nbt.2931.
- Gagnon-Bartsch JA, Speed TP. Using control genes to correct for unwanted variation in microarray data. Biostatistics. 2012;13:539–552. doi:10.1093/biostatistics/kxr034.
- Vajda A, Marignol L, Barrett C, Madden SF, Lynch TH, Hollywood D, Perry AS. Gene expression analysis in prostate cancer: the importance of the endogenous control. The Prostate. 2013;73:382–390. doi:10.1002/pros.22578.
- Chua SL, See Too WC, Khoo BY, Few LL. UBC and YWHAZ as suitable reference genes for accurate normalisation of gene expression using MCF7, HCT116 and HepG2 cell lines. Cytotechnology. 2011;63:645–654. doi:10.1007/s10616-011-9383-4.
- de Kok JB, Roelofs RW, Giesendorf BA, Pennings JL, Waas ET, Feuth T, Swinkels DW, Span PN. Normalization of gene expression measurements in tumor tissues: comparison of 13 endogenous control genes. Lab Invest. 2005;85:154–159. doi:10.1038/labinvest.3700208.
- Ohl F, Jung M, Xu C, Stephan C, Rabien A, Burkhardt M, Nitsche A, Kristiansen G, Loening SA, Radonić A, et al. Gene expression studies in prostate cancer tissue: which reference gene should be selected for normalization? J Mol Med. 2005;83:1014–1024. doi:10.1007/s00109-005-0703-z.
- Dweep H, Gretz N. miRWalk2.0: a comprehensive atlas of microRNA-target interactions. Nat Methods. 2015;12: 697–697. doi:10.1038/nmeth.3485.
- Seshan VE, Olshen A DNAcopy: DNA copy number data analysis. (Bioconductor version: Release (3.10), 2020). doi:10.18129/B9.bioc.DNAcopy.
- Yu G, Wang L-G, He Q-Y. ChIPseeker: an R/Bioconductor package for ChIP peak annotation, comparison and visualization. Bioinformatics. 2015;31:2382–2383. doi:10.1093/bioinformatics/btv145.
- Danecek P, Auton A, Abecasis G, Albers CA, Banks E, DePristo MA, Handsaker RE, Lunter G, Marth GT, Sherry ST, et al. The variant call format and VCFtools. Bioinformatics. 2011;27:2156–2158. doi:10.1093/bioinformatics/btr330.
- Angelova M, Charoentong P, Hackl H, Fischer ML, Snajder R, Krogsdam AM, Waldner MJ, Bindea G, Mlecnik B, Galon J, et al. Characterization of the immunophenotypes and antigenomes of colorectal cancers reveals distinct tumor escape mechanisms and novel targets for immunotherapy. Genome Biol. 2015;16(1). doi:10.1186/s13059-015-0620-6.
- Rohart F, Eslami A, Matigian N, Bougeard S, Lê Cao K-A. MINT: a multivariate integrative method to identify reproducible molecular signatures across independent experiments and platforms. BMC Bioinform. 2017;18:128. doi: 10.1186/s12859-017-1553-8.
- Budczies J, Klauschen F, Sinn BV, Győrffy B, Schmitt WD, Darb-Esfahani S, Denkert C. Cutoff finder: a comprehensive and straightforward web application enabling rapid biomarker cutoff optimization. PLoS ONE. 2012;7(12):e51862. doi:10.1371/journal.pone.0051862.