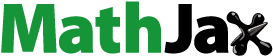
ABSTRACT
A substantial fraction of patients with stage I–III colorectal adenocarcinoma (CRC) experience disease relapse after surgery with curative intent. However, biomarkers for predicting the likelihood of CRC relapse have not been fully explored. Therefore, we assessed the association between tumor infiltration by a broad array of innate and adaptive immune cell types and CRC relapse risk. We implemented a discovery-validation design including a discovery dataset from Moffitt Cancer Center (MCC; Tampa, FL) and three independent validation datasets: (1) GSE41258 (2) the Molecular Epidemiology of Colorectal Cancer (MECC) study, and (3) GSE39582. Infiltration by 22 immune cell types was inferred from tumor gene expression data, and the association between immune infiltration by each cell type and relapse-free survival was assessed using Cox proportional hazards regression. Within each of the four independent cohorts, CD4+ memory activated T cell (HR: 0.93, 95% CI: 0.90–0.96; FDR = 0.0001) infiltration was associated with longer time to disease relapse, independent of stage, microsatellite instability, and adjuvant therapy. Based on our meta-analysis across the four datasets, 10 innate and adaptive immune cell types associated with disease relapse of which 2 were internally validated using multiplex immunofluorescence. Moreover, immune cell type infiltration was a better predictors of disease relapse than Consensus Molecular Subtype (CMS) and other expression-based biomarkers (Immune-AICMCC:238.1–238.9; CMS-AICMCC: 241.0). These data suggest that transcriptome-derived immune profiles are prognostic indicators of CRC relapse and quantification of both innate and adaptive immune cell types may serve as candidate biomarkers for predicting prognosis and guiding frequency and modality of disease surveillance.
Introduction
Of the patients with localized stage I–III colorectal cancer (CRC) who underwent surgical resection, 18% will typically experience distal relapse within the first three years following initial treatment with curative-intent.Citation1 Although post-operative surveillance is an important aspect of clinical care, variability exists in the follow-up strategies employed by physicians (e.g., modality and frequency) for localized cancers. Therefore, there is no consensus on the optimal intervals for disease surveillance with the goal of detecting disease relapse,Citation2–4 and improved approaches for assessing risk of disease relapse are needed.
Mounting evidence has implicated the tumor immune microenvironment (TME) in governing CRC relapse in early and advanced stages of disease.Citation5,Citation6 Tumor infiltration by tumor-infiltrating lymphocytes (TILs)Citation7 and specific T cell subsets, such as CD8+ cytotoxic T cells and CD45RO-marked CD4+ memory T cells, have been associated with an improved prognosis in CRC across multiple studies.Citation8,Citation9 Application of Immunoscore, an immunohistochemistry (IHC) based scoring system summarizing the density of CD3+ and CD8+ T cells within a CRC tumor and its invasive margins, suggests that the TME of primary tumors can estimate time to disease relapse.Citation10 Although Immunoscore and measures of TILs have both been inversely associated with disease relapse, opportunities exist to further refine our understanding of the TME and its role in cancer progression. First, most studies have examined the role of immune infiltration in predicting CRC relapse using traditional pathology and IHC techniques. These approaches are subject to pathologist variability, whereas transcriptomic profiles objectively measure genome-wide expression. Second, while previous studies have primarily focused on lymphocytes, a broader examination of diverse innate and adaptive immune cell subtypes, including myeloid cell lineages, is warranted.Citation11,Citation12 Specifically, innate immune cells, such as monocytes, can be reprogrammed by cancer cells to promote tumor cell invasion and growth.Citation13 Third, the predictive role of the TME has not been fully established in the setting of adjuvant therapy. Standard of care adjuvant chemotherapy and/or radiation treatment following surgical resection with curative intent can alter the TME and impact disease relapse. Thus, it is important to assess the prognostic value of the TME after adjusting for adjuvant therapy. Last, the predictive value of tumor immune infiltration should be compared to other molecular prognostic factors derived from transcriptomic profiles such as the consensus molecular subtype (CMS) classification of CRCCitation14 to fully assess the value of the TME at diagnosis as a prognostic biomarker.
Here, we investigated the association between infiltration by specific immune cell types and CRC relapse, adjusting for known prognostic indicators including receipt of adjuvant therapy. We also compared the predictive accuracy of immune infiltration scores of individual cell types and their combinations to other well-known transcriptome-derived prognostic indicators such as CMS classification for disease relapse.
Methods
Overview
Employing a computational deconvolution approach and a discovery-validation design, we quantified tumor infiltration of 22 immune cell types from CRC transcriptomic profiles across four independent studies. The discovery dataset included cases from the Total Cancer Care ProtocolCitation15 at Moffitt Cancer Center, referred to as the MCC dataset. Three datasets, GSE41258, the Molecular Epidemiology of Colorectal Cancer study (MECC), and GSE39582, provided independent validation of the utility of these biomarkers (). These studies were selected for validation purposes as they contain comprehensive clinical information on patients that allowed us to account for the effects of age, sex, stage at diagnosis, MSI status, and adjuvant therapy status when examining the association of 22 immune cell types with disease relapse. Baseline characteristics for all datasets are listed in . The primary outcome of interest is disease relapse. Disease relapse is defined as either a local (e.g. anastomosis site) or distal (e.g. lung, liver) disease relapse or CRC-specific death in patients with stage I–III tumors. Time to disease relapse was defined as the time from surgical resection with curative-intent to time of local or distal disease relapse or time to CRC-related death. For patients without a documented relapse or CRC-related death, survival times were censored at last follow-up or non-CRC specific death. Disease relapse information was available for the MCC and GSE41258 datasets. Disease-specific survival was available for the MECC dataset. Relapse-free survival was the primary outcome in the GSE39582 dataset.
Table 1. Baseline clinical characteristics for MCC, GSE41258, MECC, and GSE39582 participants
Discovery: Total Cancer Care MCC dataset
The discovery population (MCC) consisted of 134 stage I–III and 40 stage IV colorectal adenocarcinoma patients diagnosed at Moffitt Cancer Center between May 1994 and September 2010.Citation16 Primary analyses examining disease relapse were performed on stage I–III tumors, while CRC-specific death was the primary endpoint for analyses performed on stage IV-only tumor samples. Inclusion and exclusion criteria for the study sample are summarized in Supplementary Fig. S1.
Microarray gene expression data for all samples were curated by the MCC Biostatistics and Bioinformatics Shared Resource. All samples passed multiple standard quality controls, and all transcriptomic data analyzed were previously deposited to the Gene Expression Omnibus (GEO) site curated by the National Center for Bioinformatics (GSE131418), where associated clinical data can be found (see supplemental methods).
Validation datasets: GSE41258, MECC, GSE39582
The first validation dataset, GSE41258, is a publicly available dataset containing information on disease relapse, CRC-specific death, and corresponding clinical information including age, sex, stage at diagnosis, and microsatellite instability (MSI) status, for 169 patients with stage I–IV tumors.Citation17 The second validation set consists of gene expression microarray data from the Molecular Epidemiology of Colorectal Cancer (MECC) study, a population-based case-control study in northern Israel (GSE26682). This study was used to validate associations with CRC-specific survival.Citation18 The MECC gene expression dataset consists of 270 patients with stage I–IV tumors and corresponding clinical information, including MSI status. CRC-specific five-year survival in MECC was used as the outcome of interest (see Supplemental Methods). The third validation dataset, GSE39582, is also a publicly available dataset containing information on relapse-free survival and corresponding clinical information includes age, sex, stage at diagnosis, MSI status, and adjuvant therapy status for 507 patients with stage I–IV tumors.Citation19 Relapse-free survival in GSE39582 was used as the primary outcome of interest. Analyses of all four datasets were restricted to participants with stage I–III tumors, except where specifically noted for the exploration of disease-specific survival in patients with stage IV disease.
Quantification of tumor immune infiltration, consensus molecular subtype (CMS) classification, and pathway enrichment analysis
We applied the BASE deconvolution algorithmCitation20 implementing the LM22 matrix from CIBERSORTCitation21 to estimate the infiltration of 22 immune cell types from bulk tumor gene expression data (see Supplemental Methods). The 22 immune cell types examined are listed in Supplementary Table S1. We also implemented the MCP Counter method to compare the predictive performance of our BASE immune infiltration method with existing deconvolution approaches.Citation22 Furthermore, we measured relative T cell fraction using the DNA-based immunoSEQ assay (Adaptive Biotechnologies, Seattle, WA) on a subset of patients in the MECC validation dataset (n = 231; see Supplemental Methods).
CMS is a transcriptome-based classification of CRC with independent prognostic value.Citation14 Tumors were classified into CMS groups 1–4 or CMS_NA using the R package CMSclassifier, with a posterior probability of 0.5..Citation14 The CMS4 group was set as the reference CMS group for all comparative analyses.
Transcriptomic activity of pathways from the Hallmark and C2. Reactome Collections in the Molecular Signature Database (MSigDB V6.0)Citation23 was quantified for each tumor sample. Estimates of pathway activity were then used as inputs in downstream regression models examining the association between specific pathways and disease relapse adjusting for appropriate clinical variables. Using Spearman rank correlation, pathways that were associated with disease relapse in a multivariable Cox model (FDR <0.1) were then correlated with infiltration scores of individual immune cell types, which also independently associated with disease relapse. Pathways defined by genes that overlapped with the gene set defining the immune cell signatures were identified and the degree of shared overlap in genes was quantified for each pathway. We specifically focused on pathways that did not share genes with the immune-defining signature gene set. This allowed us to ensure that immune cell-pathway correlations were detecting true correlations between immune cell type infiltration and tumor pathway activity and that correlations were not driven by shared gene expression. Pathways enriched in the discovery MCC dataset were validated in the GSE41258 dataset (see Supplementary Methods).
Validation of transcriptome-derived tumor immune infiltration scores
Multiplex immunofluorescence (mIF) was performed on tumor cores embedded in tissue microarray (TMA)
blocks for a subset of patients in the MCC (N = 68) and MECC (N = 99) datasets to quantify and validate CD8+ T cell and memory T cell infiltration score estimates from bulk transcriptomic data. We calculated Spearman’s rank correlation coefficient between densities of CD3+/CD8+ cells and CD3+/CD45RO cells and CD8 T cell and memory T cell (resting and activated) transcriptome-derived immune infiltration scores, respectively. Additional details can be found in the Supplementary Methods.
Statistical analysis
Statistical significance testing of differences in infiltration score means was performed using the Wilcoxon rank sum test. Cox proportional hazards models were used to perform univariate and multivariate analyses to determine the association of immune cell types and pathways with time to disease relapse and CRC-specific survival in patients with stage I–III tumors. CMS group association with disease relapse was assessed to compare the performance of CMS groups and immune cell types in predicting disease relapse. All multivariable Cox-regression models adjusted for age, sex, and stage. For the MCC and GSE39582 datasets, we also adjusted for adjuvant therapy. Treatment information, including adjuvant therapy status was unavailable for the MECC and GSE41258 datasets. However, for the MECC, GSE41258, and GSE39582 datasets, we adjusted for MSI status. The MCC dataset did not have sufficient information for MSI adjustment. The association of CMS groups with disease relapse was assessed using both a logistic regression framework and Chi-Square test. In order to evaluate consistency of results across study cohorts, a meta-analysis was performed using results from all four datasets (see Supplemental Methods; Supplementary Table S2). For the MCC and GSE41258 datasets, which had the most sensitive measures of disease relapse, we compared the predictive performance of gene-expression derived estimates of infiltrating immune cells with CMS groups and single-gene (e.g., CD8A and CD3D) predictors using the Akaike Information Criterion (AIC). All regression models of interest were also compared to a null model, which examined the association between disease relapse and clinical variables only, using the likelihood ratio test (LRT). We also compared the predictive performance of gene-expression derived estimates of infiltrating immune cells with immunoSEQ T cell fraction for disease relapse on the subset of patients in the MECC validation dataset with both data sources. All p-values and confidence intervals are reported without adjustment for multiple hypothesis testing unless indicated otherwise. All statistical analyses were performed in R (Versions 3.4 & 3.5).
Results
Tumor infiltration by innate and adaptive immune cells is associated with disease relapse
We estimated tumor immune infiltration from gene expression profiles of tumors and examined the association between infiltration by each immune cell type and time to disease relapse (). In the MCC discovery dataset, high infiltration by several T cell subsets, including CD8+ T cells and CD4+ memory T cells (resting and activated), was associated with improved prognosis. Monocytic infiltration was associated with poor prognosis and disease relapse (Supplementary Fig. S2). B cell, T cell, macrophage, and dendritic cell subsets were all associated with stage at diagnosis, while only resting dendritic cells were associated with administration of adjuvant therapy (Supplementary Fig. S3–4). Tumor immune infiltration for all 22 cell types did not differ based on tumor anatomical origin (Supplementary Fig. S5). Therefore, we did not adjust for tumor anatomical origin when determining the association of the TME with disease relapse. In the discovery dataset (MCC), CD8+ T cell (HR: 0.92; 95% CI: 0.84–0.99; P =.04), CD4+ memory resting T cell (HR: 0.89; 95% CI: 0.80–0.99; P =.02), CD4+ memory activated T cell (HR: 0.91; 95% CI: 0.83–0.99; P =.03), and CD4+ Naïve T cell (HR: 0.91; 95% CI: 0.83–0.99; P =.04) infiltration scores were associated with a lower risk of CRC relapse. Monocyte (HR: 1.07; 95% CI: 1.01–1.15; P =.03) and neutrophil (HR: 1.07; 95% CI: 1.00–1.14; P =.04) infiltration was associated with an increased risk of disease relapse after adjusting for known prognostic clinical variables. However, given the strong association between CMS1 and MSI-high status, we also considered CMS1 as an imperfect proxy for MSI in sensitivity analyses and found that CD8+ T cells and CD4+ memory (resting and activated) T cells remained strong predictors of having a lower risk of disease relapse and fewer disease-relapse events. Monocytes and neutrophils remained strong predictors of worse prognosis and a shorter time to disease relapse (Supplementary Table S3). These results were replicated across three validation datasets, where MSI information was available and appropriately adjusted for in all regression analyses.
Figure 1. Immune infiltration and disease relapse association. The association between disease relapse and relative immune infiltration of 22 immune cell types in CRC samples across four datasets – MCC (n = 134), GSE41258 (n = 119), MECC (n = 257), GSE39582 (n = 464) – in stage I–III tumors was examined using multivariable Cox regression modeling. For all datasets, the association of each immune cell type with disease relapse was determined while adjusting for age, sex, adjuvant therapy treatment, microsatellite instability (MSI) status, and stage at diagnosis. For the GSE41258 and MECC datasets, adjuvant treatment status was not available while MSI information was not available for the MCC dataset. For the MECC dataset, the association between relative immune infiltrates and CRC-specific death was determined. Overall, 22 regression models were performed for each dataset. The coefficients for each immune cell type are displayed with their respective confidence intervals. A joint meta-analysis was performed based on results from across the four studies so that the collective association of immune cell infiltration on disease relapse could be examined. Meta-analysis is presented with the FDR-adjusted p-values for each immune cell type
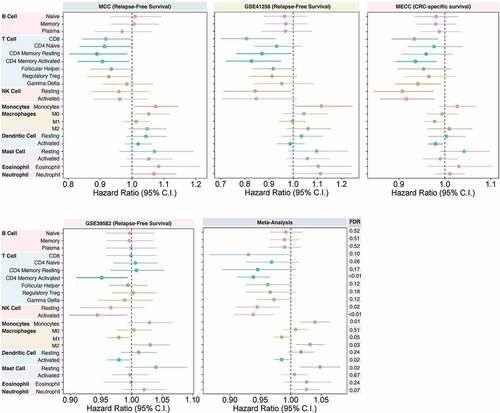
In the GSE41258 dataset, CD8+ T cell and CD4+ memory (resting and activated) T cells were associated with lower risk of disease relapse, while monocyte infiltration was associated with increased risk of disease relapse (). Notably, in the GSE41258 dataset, NK resting cells (HR: 0.84; 95% CI: 0.71–0.99; P =.04) were also associated with lower risk of disease relapse.
The association between CD8+ T cells, CD4+ memory activated T cells, and disease relapse in patients with stage I–III disease was replicated in the MECC dataset (). The associations for CD4+ memory resting T cells and monocytes with disease relapse were also trending in the same direction as observed for the MCC dataset (). In MECC, NK (resting and activated) cells, activated dendritic cells, and gamma-delta T cells were also strongly associated with improved CRC-specific five-year survival ().
In the GSE39582 dataset, where we were able to account for the effects of both adjuvant therapy and MSI status information on disease relapse, the association between CD4+ memory activated T cell (HR: 0.95; 95% CI: 0.91–0.99; P =.02) and disease relapse was replicated. Activated NK cell (HR: 0.94; 95% CI: 0.89–0.99; P =.03), activated dendritic cell (HR: 0.98; 95% CI: 0.96–0.99; P =.04), and M1 macrophage (HR: 0.98; 95% CI: 0.99–1.06; P =.05) infiltration was also associated with a lower risk of disease-relapse in this dataset.
Using a meta-analysis framework, we demonstrated that overall the strongest predictors of disease-relapse risk observed across all four datasets were CD4+ memory activated T cells (; HR: 0.93, 95% CI: 0.90–0.96; FDR = 0.0001), NK activated cells (; HR: 0.93; 95% CI: 0.90–0.97; FDR = 0.002), monocytes (; HR: 1.03; 95% CI: 1.01–1.06; FDR = 0.01), NK resting cells (; HR: 0.94; 95% CI: 0.89–0.98; FDR = 0.02), activated dendritic cells (; HR: 0.98; 95% CI: 0.97–0.99; FDR = 0.02), resting mast cells (; HR: 1.05; 95% CI: 1.01–1.08; FDR = 0.02), M2 macrophages (; HR: 1.03; 95% CI: 1.007–1.05; FDR = 0.03), M1 macrophages (; HR: 0.98; 95% CI: 0.97–0.99; FDR = 0.05) and neutrophils (; HR: 1.03; 95%CI:1.00–1.05; FDR = 0.07). While immune infiltration by CD8+ T cells was strongly associated with a lower disease relapse risk in some individual datasets, CD8+ T cell infiltration was less strongly associated with disease relapse in the meta-analysis (; HR: 0.93; 95% CI: 0.86–0.99; FDR = 0.10). This is likely due to the heterogeneity observed between datasets (heterogeneity p-value = 0.008; Supplementary Table S2). CD4+ memory activated T cell and CD8+ T cell immune infiltration scores were internally validated using multiplex immunofluorescence TMA cores from N = 68 MCC patients and N = 99 MECC patients using (see Supplementary Results; Supplementary Fig. S6).
Specifically, CD4+ memory activated T cells, NK cells (resting and activated), M1 macrophages, and activated dendritic cells were all associated with a lower risk of disease relapse and having fewer disease relapse events. In contrast, monocyte, M2 macrophage, neutrophil, and resting mast cell infiltration was associated with a higher risk of disease relapse and having more disease relapse events (; FDR < 0.1). Interestingly, although not significantly associated with disease relapse in any of the individual datasets, joint statistical analysis of the discovery and validation datasets was able to demonstrate the association of resting mast cell infiltration with shorter time to disease relapse (FDR < 0.05; ; Supplementary Table S2).
Immune-immune interactions between regulatory T cells and eosinophils are significant predictors of disease relapse
Immune–immune interactions have known biologically relevance including modulating the TME. Therefore, we aimed to examine immune-immune interactions in the TME in order to determine if statistical interactions between immune cells have predictive value for estimating disease relapse. Therefore, we examined immune interactions using risk modeling. Using lasso penalized regression on the MCC dataset, the following immune cells and their combinations with non-zero coefficients at the minimum lambda were selected for risk modeling: CD4+ memory resting T cells, monocytes, regulatory T cells, eosinophils, and the interaction between regulatory T cells and eosinophils ()). A risk score, dubbed the “immune + clinical model”, was developed using these immune cell combinations and clinical variables of interest as predictors. Patients with high-risk scores were then compared to patients with low-risk scores in order to determine if the “immune + clinical model” based risk score was predictive of disease relapse. The “immune + clinical model” risk score was developed using the MCC dataset and validated on the GSE41258 dataset ()). Similarly, a risk score was developed using only clinical variables, dubbed the “clinical model” risk score ()), which was also trained on the MCC dataset and validated on the GSE41258 dataset. This allowed us to compare the performance of the immune-based risk score with the clinical covariate-based risk score in predicting disease relapse. High-low dichotomization of the clinical-based risk scores and the immune-based risk scores showed that the immune-based risk scores best-predicted disease relapse in the GSE41258 dataset ()). These findings highlight the relevance of examining combinations of immune cell types and their collective impact on disease relapse rather than examining one immunological cell type at a time.
Figure 2. Immune infiltration and risk model prediction. Using least absolute shrinkage and selection operator (Lasso) regression modeling, immune predictors and their combinations that associate with disease relapse were selected for risk modeling. All models were trained on the MCC dataset and applied to the GSE41258 validation dataset. The immune model was developed using the Lasso selected immune variable and includes the clinical variables age, sex, and stage (a). The clinical model was developed only using the clinical variables age, sex, and stage (b). The outputs from the immune and clinical models were dichotomized into high-risk and low-risk groups and their association with disease relapse was examined in both datasets and displayed in Kaplan-Meier (KM) curves with confidence bands (c). Differences between survival curves in the KM plots were determined using log-rank test. Meaningful p-values are shown for the GSE41258 validation dataset
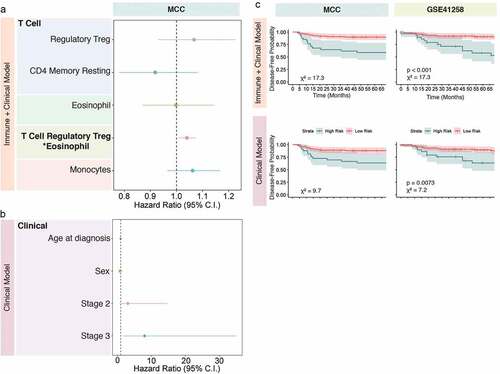
Tumor immune infiltration is a better predictor of time to CRC relapse than other transcriptome-based biomarkers
Other molecular stratification schemes, such as CMS and CD8A and CD3D gene expression, have previously been shown to be predictive of clinical outcomes in CRC. Therefore, we aimed to determine if tumor immune infiltration estimates inferred from the transcriptome are better predictors of disease relapse than these known transcriptomic signatures or clinical variables alone. Specifically, we compared the performance of CD4+ memory (resting and activated) T cells, CD8+ T cell, CD4+ naïve T cell, monocyte, and neutrophil infiltration with these other molecular stratification schemes, as these cell types were strongly associated with disease relapse in stage I–III tumors in our discovery MCC dataset. CD8+ T cell (AICMCC: 239.5; AICGSE41258: 172.4), monocyte (AICMCC: 238.9; AICGSE41258: 178.4), CD4+ memory resting T cell (AICMCC: 238.1; AICGSE41258: 178.2), and CD4+ memory activated T cell (AICMCC: 239.1; AICGSE41258: 175.1) infiltration were better predictors of disease relapse than CMS (AICMCC: 241.0; AICGSE41258: 184.6), clinical variables alone (), CD8A expression (AICMCC: 243.9; AICGSE41258: 182.2), and CD3D expression (AICMCC: 243.0; AICGSE41258: 181.4) in both MCC and GSE41258 where disease relapse in stage I–III tumors were directly assessed (). Last, no single CMS group was consistently associated with time to disease relapse using multivariable modeling in either the MCC or GSE41258 datasets. However, the proportion of CMS4 tumors was higher in patients with a disease relapse event compared to patients without relapse ()) in the MCC dataset (MCC; χ2 test p-value = 0.03). CMS group did not predict CRC relapse better than any model examining immune infiltration or clinical variables alone, thereby further highlighting the prognostic value of expression-derived immune infiltration over CMS classification.
Table 2. The performance of nine models for predicting CRC relapse was compared in two datasets – MCC and GSE41258. All multivariable Cox proportional hazards regression models for a given dataset were compared to one another using the Akaike Information Criterion (AIC) and the likelihood ratio test (LRT)
In a subset of patients (n = 231) with stage, I–IV disease in the MECC validation dataset, both gene expression-derived immune infiltration estimates for 22 immune cell types and T cell fraction from immunoSEQ were available. Of the 22 expression-derived immune cell infiltration scores, only CD8+ T cell immune infiltration considered alone performed slightly better (model AIC: 765.6) than T cell fraction (model AIC: 766.2) in predicting CRC-specific survival in multivariable models adjusting for known prognostic clinical variables. While both expression-based immune infiltration scores and immunoSEQ T cell fraction are independent predictors of CRC disease relapse, T cell fraction has a larger effect size when predicting disease relapse (Supplementary Table S4–S5).
Compared to other deconvolution methods, such as MCP counter, our method (BASE) for estimating immune infiltration using transcriptomic profiles was able to replicate known associations between lymphocyte infiltration (T cells) and disease relapse (Supplementary Figure S7). Using the meta-analysis approach for the BASE-derived immune infiltration estimates, we demonstrated the association of at least 9 immune cell types with disease relapse. Applying the same meta-analysis framework to the MCP Counter-derived immune infiltration estimates, revealed that only endothelial cells were predictive of disease relapse risk (HR: 1.77; 95%CI:1.20–2.62; FDR:0.04).
CMS3 tumors are enriched in T cell subtypes and depleted in monocytic and macrophage infiltrates compared to CMS4
Given that CMS4 is defined by stromal infiltration with fibroblasts and macrophages and CMS1 tumors are characterized by broad lymphocytic infiltration, we examined the distribution of the 22 immune cell infiltration scores across CMS groups to determine if each CMS group exhibits a unique immune profile (, Supplementary Fig. S8). Consistently across all datasets, higher T cell infiltration and lower monocyte and macrophage activity were observed in CMS3 tumors compared to CMS4 tumors, while CMS1 and CMS_NA tumors were characterized by broad immune infiltration by all 22 immune cell types compared to CMS4 tumors. Notably, CMS1 tumors also exhibited higher NK cell infiltration than other CMS groups across all datasets (). The immune profile of CMS3 and CMS1 tumors may also explain their association with lower disease relapse risk. Using logistic regression, the association of CMS group with having a disease relapse event was assessed collectively across all three datasets revealing CMS1 (OR: −0.72; p-value = 0.01) and CMS3 (OR = −0.62; p-value = 0.02) tumors are associated with a lower odds of having a disease relapse event.
Figure 3. Consensus Molecular Subtype (CMS) association with immune infiltration and disease relapse. Relative immune infiltration across CMS groups was determined by examining the median difference between the immune infiltration in each CMS and the reference CMS4 (left). High immune infiltration is shown in red and low immune infiltration is shown in blue. Distribution of CMS tumors in patients who either did or did not exhibit disease relapse (a and b) or who either did nor did not die from CRC (c) was examined in four independent datasets for stage I–III tumors and is displayed in tables (right). Using a logistic regression framework, we assessed the collective effect of CMS distribution on disease relapse in stage I–III tumors across all four datasets, while adjusting for age, sex, stage, and dataset attributes
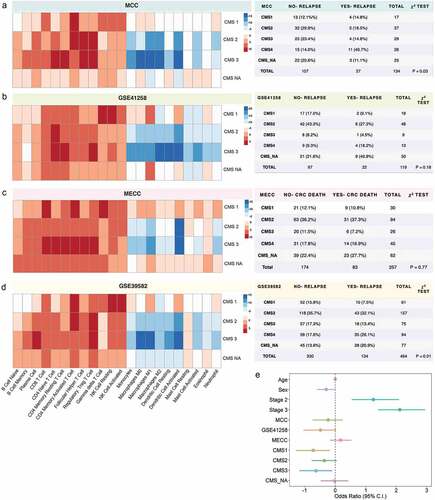
The VEGF ligand-receptor pathway correlates positively with memory T cell infiltration and negatively with monocytic infiltration
Finally, we aimed to discover potential interactions between immune cell types and tumor cells in the microenvironment that influence disease relapse. We first determined the association between pathway (gene sets) activity and disease relapse using multivariable Cox regression adjusting for clinical variables. Next, we examined the correlation between pathways and immune infiltration estimates for pathways and immune cell types which were independently found to associate with disease relapse (, Table S6–S11, Supplementary Fig. S9–S10). This approach allowed us to identify three key pathways in both the MCC and GSE41258 datasets that negatively correlated with CD4+ memory resting T cell and CD8+ T cell infiltration and positively correlated with monocyte infiltration (Supplementary Fig. S10). These pathways were: SIGNALING_BY_HIPPO, VEGF_LIGAND_RECEPTOR_INTERACTIONS, and RECEPTOR_LIGAND_BINDING_INITIATES_THE_SECOND_PROTEOLYTIC_CLEAVAGE_OF_NOTCH_RECEPTOR (Supplementary Fig. S9–S10). Overall, these results suggest tumor pathway activity and tumor immune infiltration jointly influence disease relapse risk, and potential tumor-immune interactions that together may influence relapse can be determined from transcriptomic data.
Table 3. CD4+ Memory Resting T Cell infiltration and tumor pathway enrichment correlation in the MCC cohort. Spearman rank correlation was used to determine the association between tumor pathway activity and CD4+ Memory Resting T Cell infiltration scores. Tumor pathways from the C2. Reactome collection in MSigDB were selected if they associated with disease relapse in a multivariable regression model (FDR < 0.1). The gene set size for each pathway of interest is noted. The overlap between gene sets defining a given tumor pathway and genes in the LM22 signature gene set is also shown. In addition, the specific overlap with CD4+ Memory Resting T cell defining genes is also noted
Discussion
In summary, we demonstrated that gene expression-derived estimates of adaptive and innate immune cell type in the TME have strong prognostic value beyond current expression-based biomarkers. By combining transcriptomic data with bioinformatic deconvolution methods, we inferred tumor immune infiltration by 22 immune cell types and showed that transcriptome-based inferences about the TME are predictive of disease relapse. Specifically, in a joint meta-analysis of four cohorts, CD4+ memory activated T cell tumor infiltration was the strongest predictor of disease relapse even after accounting for known prognostic indicators including adjuvant therapy. Other immune cell types significantly associated with disease relapse based on the results from our meta-analysis include NK resting and activated cells, monocytes, resting mast cells, neutrophils, M1 and M2 macrophages, activated dendritic cells, and CD8+ T cells. Our results on the association of M2 macrophage and neutrophil infiltration with poor disease prognosis align well with previous studies and demonstrate the utility of examining both adaptive and immune cell infiltration.Citation24 We also demonstrated that transcriptome-derived immune infiltration estimates of CD4+ memory T cells, CD8+ T cells, and monocytes are better predictors of disease relapse than CMS group classification, CD8A expression, and CD3D expression. Our results also suggest that both expression-based immune cell estimates and immunoSEQ-derived relative T cell fraction estimates are independent predictors of disease relapse. However, it appears that each method detects different underlying biology (Supplementary Table S5) given the strong independent performance of each predictor in joint modeling analyses. In comparison to other deconvolution approaches, such as MCP counter, our transcriptome-derived immune infiltration method (BASE) was able to replicate known associations of lymphocytic and myeloid-lineage cell infiltration with disease relapse. We also highlighted the importance of examining both adaptive and innate immune cell subtypes in the TME. Monocytic subpopulations in the TME are known potent immune suppressors that can facilitate tumor dissemination by inducing EMT/cancer stem cell phenotypes and promote M2 macrophage polarization.Citation25 Memory T cells targeting oncogenic mutations can be detected in peripheral blood of patients with metastatic CRC making these antigen-experienced CD4+ memory T cells highly suitable for adoptive T cell therapy.Citation26 Therefore, selecting candidates with high CD4+ memory T cell infiltration for adoptive T cell therapy would be highly favorable.
Overall, our study underscores the use of transcriptome-derived tumor immune infiltration estimates as a biomarker for predicting disease relapse in CRC patients with stage I–III disease. This is particularly valuable given the lack of biomarkers, with the exception of CEA at the time of diagnosis, for predicting disease relapse in high-risk patients.
CMS3 tumors demonstrate T cell enrichment and macrophage and monocyte depletion compared to CMS4 tumors
We showed that high T cell infiltration, particularly by the CD8+ and CD4+ memory T cell subsets and low macrophage and monocyte infiltration was consistently observed in the CMS1 and CMS3 groups compared to CMS4 tumors (, Supplementary Fig. S9). Our findings on high lymphocytic and NK cell activity in CMS1 tumors compared to CMS4 tumors align well with previous studies that have demonstrated high lymphocytic infiltration in CMS1 tumors and high monocyte and myeloid cell infiltration in CMS4 tumors.Citation27 Moreover, our logistic regression results suggest CMS1 and CMS3 tumors are associated with not having a disease relapse event. These findings align with our previous work demonstrating that the CMS3 phenotype is selectively excluded in CRC metastases compared to primary tumors, such that CMS3 tumors were never observed in lung and liver CRC metastases.Citation16 Our observation of high T cell activity and low monocyte activity in CMS3 tumors is supported by previous observationsCitation28 and may explain their potential exclusion from metastases as well as why patients with CMS3 primary tumors in the GSE41258, MCC, and GSE39582 datasets exhibited fewer disease relapse events. This has potential implications for assessing disease prognosis based on the CMS phenotypes. Last, while the focus of this study is the relationship between disease relapse and immune infiltration, we did examine the association of 22 specific immune cell types with disease-specific survival in patients with stage IV disease from all four datasets as well as in patients from the two GSE datasets with stage IV microsatellite stable (MSS) disease (Supplementary Fig. S11) using our meta-analysis framework. As these cohorts were relatively small and most likely underpowered, no specific immune cell type was found to be significantly associated with CRC-specific survival. However, interestingly, we did note that unlike in the disease relapse analyses, NK activated cells may be associated with shorter CRC-specific survival in patients with stage IV disease and in patients with stage IV MSS disease.
Potential interactions between VEGF-tumor pathway activity and T cells may have therapeutic value
Understanding tumor immune interactions is essential as was demonstrated by studies examining the joint utility of tumor-inflamed gene expression profile (GEP) and tumor mutation burden in predicting PD-1 checkpoint blockade response across multiple tumor types.Citation29 Therefore, we aimed to discover potential tumor immune interactions by examining if specific immune cell subtypes that are known to associate with disease relapse are also associated with specific oncogenic pathways. As such, we examined correlations between tumor immune infiltration estimates and tumor pathway activity. We found 3 main pathways which were independently associated with disease relapse and correlated with T cell and monocyte infiltration of which the VEGF_LIGAND_RECEPTOR_INTERACTIONS pathway was the most clinically relevant. VEGF ligand receptors are required for the recruitment and migration of monocytes, macrophages, and hematopoietic precursors. Moreover, tumor angiogenesis is directly regulated by VEGF-A and VEGFR2, and VEGF inhibitor therapy with Bevacizumab is a first-line regimen for metastatic CRC, albeit the gain in survival made with Bevacizumab has been modest.Citation30 Given the association of the VEGF ligand-receptor pathway with monocyte and T cell infiltration, our studies suggest that combining anti-angiogenic drug targets with immunotherapy agents may be promising, and that VEGF inhibitor therapies may yield better results if used in a subset of patients with high monocyte and low T cell infiltration prior to metastatic progression.
Study limitations and future directions
We examined the relationship between molecular and clinical variables, most notably, adjuvant therapy receipt, CMS groups, and MSI, and gene-expression derived tumor immune infiltration to comprehensively determine the independent association of gene-expression derived immune infiltration on disease relapse. This is particularly valuable in the setting of immunotherapy, such as adoptive T cell transfer therapy, where selecting patients with high CD4+ memory T cell populations would be advantageous.Citation26 Although gene expression panels examining molecular profiles such as CMS and tumor immune profiles are not part of the standard repertoire of molecular assays available in a clinical setting, such profiling of tumors is becoming increasingly common in clinical research settingsCitation31 and have utility for stratifying patients into high and low-risk groups in the setting of clinical trials. However, this study should be considered in the context of its limitations. Although adjuvant therapy information was abstracted, information on the type and duration of treatment was incomplete. Moreover, the accuracy of distinguishing between resting and activated cell states is limited. Therefore, establishing immune signatures for distinct cell states at the bulk tumor level would immensely benefit from future work at the single-cell level, where individual cell states can be better delineated.
Disclosure of potential conflicts of interest
Stephen Gruber reports research funding from Myriad Genetics, consulting to Fulgent Genetics, and is co-founder and equity shareholder of Brogent International LLC. Victor Moreno reports he is a consultant to Bioiberica S.A. U. and Grupo Ferrer S.A., and has received research funds from Universal DX, and is a co-investigator in grants with Aniling.
Role of the funder/sponsor
The funders had no role in the design, collection, management, analysis, and interpretation of the study, preparation or approval of the manuscript and decision to submit the manuscript for publication.
Supplemental Material
Download ()Acknowledgments
We appreciate the contributions of the Advanced Analytical and Digital Pathology Laboratory in the Pathology Department at Moffitt Cancer Center led by medical director, Daryoush Saeed-Vafa, MD. Specifically, we acknowledge Neale Lopez-Blanco for tissue preparation and staining; Carlos Moran Segura for tissue preparation and staining, antibody panel design, and multi-spectral scanning; and Jonathan Nguyen for image quantification. We would also like to thank Dr. Eric A. Welsh for his assistance in the acquisition, curation, and cleaning of the datasets used in this manuscript.
Supplementary material
Supplemental data for this article can be accessed on the publisher’s website.
Additional information
Funding
References
- van Gestel YRBM, de Hingh IHJT, van Herk-sukel MPP, van Erning FN, Beerepoot LV, Wijsman JH, Slooter GD, Rutten HJT, Creemers GJM, Lemmens VEPP. Patterns of metachronous metastases after curative treatment of colorectal cancer. Cancer Epidemiol. Elsevier Ltd. 2014;38(4):448–12. doi:10.1016/j.canep.2014.04.004.
- Sadahiro S, Suzuki T, Ishikawa K, Nakamura T, Tanaka Y, et al. Recurrence patterns after curative resection of colorectal cancer in patients followed for a minimum of ten years. Hepatogastroenterology. 2003;50:1362–1366. http://www.ncbi.nlm.nih.gov/pubmed/14571738.
- Eisenberg B, Decosse JJ, Harford F, Michalek J. Carcinoma of the colon and rectum: the natural history reviewed in 1704 patients. Cancer. 1982;49:1131–1134. doi:10.1002/1097-0142(19820315)49:6<1131::AID-CNCR2820490611>3.0.CO;2-T.
- Secco GB, Fardelli R, Gianquinto D, Bonfante P, Baldi E, Ravera G, Derchi L, Ferraris R. Efficacy and cost of risk-adapted follow-up in patients after colorectal cancer surgery: a prospective, randomized and controlled trial. Eur J Surg Oncol. 2002;28:418–423. doi:10.1053/ejso.2001.1250.
- Pagès F, Berger A, Camus M, Sanchez-Cabo F, Costes A, Molidor R, Mlecnik B, Kirilovsky A, Nilsson M, Damotte D. Effector memory T cells, early metastasis, and survival in colorectal cancer. N Engl J Med. 2005;353:2654–2666. doi:10.1056/NEJMoa051424.
- Mlecnik B, Van Den Eynde M, Bindea G, Church SE, Vasaturo A, et al. Comprehensive intrametastatic immune quantification and major impact of immunoscore on survival. 2017 [accessed 2019 Jan 10]. https://academic.oup.com/jnci/article-abstract/110/1/97/4093937
- Rozek LS, Schmit SL, Greenson JK, Tomsho LP, Rennert HS, Rennert G, Gruber SB. Tumor-infiltrating lymphocytes, Crohn’s-like lymphoid reaction, and survival from colorectal cancer. JNCI J Natl Cancer Inst. Oxford University Press. 2016;108. doi:10.1093/jnci/djw027.
- Prizment AE, Vierkant RA, Smyrk TC, Tillmans LS, Nelson HH, Lynch CF, Pengo T, Thibodeau SN, Church TR, Cerhan JR. Cytotoxic T cells and granzyme B associated with improved colorectal cancer survival in a prospective cohort of older women. Cancer Epidemiol Biomarkers Prev. 2017;26:622–631. doi:10.1158/1055-9965.EPI-16-0641.
- Nosho K, Baba Y, Tanaka N, Shima K, Hayashi M, Meyerhardt JA, Giovannucci E, Dranoff G, Fuchs CS, Ogino S. Tumour-infiltrating T-cell subsets, molecular changes in colorectal cancer, and prognosis: cohort study and literature review. J Pathol. 2010;222:350–366. doi:10.1002/path.2774.
- Pagès F, Mlecnik B, Marliot F, Bindea G, Ou F-S, Bifulco C, Lugli A, Zlobec I, Rau TT, Berger MD, et al. International validation of the consensus Immunoscore for the classification of colon cancer: a prognostic and accuracy study. Lancet. 2018;391(10135):2128–2139. doi:10.1016/S0140-6736(18)30789-X.
- Pollard JW. Tumour-educated macrophages promote tumour progression and metastasis. Nat Rev Cancer. 2004;4:71–78. doi:10.1038/nrc1256.
- Gordon SR, Maute RL, Dulken BW, Hutter G, George BM, McCracken MN, Gupta R, Tsai JM, Sinha R, Corey D, et al. PD-1 expression by tumour-associated macrophages inhibits phagocytosis and tumour immunity. Nature. 2017;545(7655):495–499. doi:10.1038/nature22396.
- Cassetta L, Fragkogianni S, Sims AH, Swierczak A, Forrester LM, Zhang H, Soong DYH, Cotechini T, Anur P, Lin EY, et al. Human tumor-associated macrophage and monocyte transcriptional landscapes reveal cancer-specific reprogramming, biomarkers, and therapeutic targets. Cancer Cell Cell Press. 2019;35(4):588–602.e10. doi:10.1016/j.ccell.2019.02.009.
- Guinney J, Dienstmann R, Wang X, de Reyniès A, Schlicker A, Soneson C, Marisa L, Roepman P, Nyamundanda G, Angelino P, et al. The consensus molecular subtypes of colorectal cancer. Nat Med. 2015;21:1350–1356. doi:10.1038/nm.3967.
- Fenstermacher DA, Wenham RM, Rollison DE, Dalton WS. Implementing personalized medicine in a cancer center. Cancer J. NIH Public Access. 2011;17:528–536. doi:10.1097/PPO.0b013e318238216e.
- Kamal Y, Schmit SL, Hoehn HJ, Amos CI, Frost HR. Transcriptomic differences between primary colorectal adenocarcinomas and distant metastases reveal metastatic colorectal cancer subtypes. Cancer Res. 2019 [cited 2019 Jun 27]:canres.3945.2018. http://www.ncbi.nlm.nih.gov/pubmed/31239274
- Sheffer M, Bacolod MD, Zuk O, Giardina SF, Pincas H, Barany F, Paty PB, Gerald WL, Notterman DA, Domany E, et al. Association of survival and disease progression with chromosomal instability: A genomic exploration of colorectal cancer. Proc Natl Acad Sci U S A. 2009;106:7131–7136. doi:10.1073/pnas.0902232106.
- Sanz-Pamplona R, Cordero D, Berenguer A, Lejbkowicz F, Rennert H, Salazar R, Biondo S, Sanjuan X, Pujana MA, Rozek L, et al. Gene expression differences between colon and rectum tumors. Clin Cancer Res. 2011;17:7303–7312. doi:10.1158/1078-0432.CCR-11-1570.
- Marisa L, de Reyniès A, Duval A, Selves J, Gaub MP, Vescovo L, Etienne-Grimaldi M-C, Schiappa R, Guenot D, Ayadi M, et al. Gene expression classification of colon cancer into molecular subtypes: characterization, validation, and prognostic value. PLoS Med. Public Library of Science. 2013;10:e1001453. doi:10.1371/journal.pmed.1001453.
- Varn FS, Andrews EH, Mullins DW, Cheng C. Integrative analysis of breast cancer reveals prognostic haematopoietic activity and patient-specific immune response profiles. Nat Commun. 2016;7:10248. doi:10.1038/ncomms10248.
- Newman AM, Liu CL, Green MR, Gentles AJ, Feng W, Xu Y, Hoang CD, Diehn M, Alizadeh AA. Robust enumeration of cell subsets from tissue expression profiles. Nat Methods. 2015;12:453–457. doi:10.1038/nmeth.3337.
- Becht E, Giraldo NA, Lacroix L, Buttard B, Elarouci N, Petitprez F, Selves J, Laurent-Puig P, Sautès-Fridman C, Fridman WH. Estimating the population abundance of tissue-infiltrating immune and stromal cell populations using gene expression. Genome Biol. BioMed Central Ltd. 2016;17:218. doi:10.1186/s13059-016-1070-5.
- Liberzon A, Birger C, Thorvaldsdó H, Ghandi M, Mesirov JP, et al. The molecular signatures database hallmark gene set collection. Cell Syst. 2015;1:417–425. doi:10.1016/j.cels.2015.12.004.
- Ye L, Zhang T, Kang Z, Guo G, Sun Y, Lin K, Huang Q, Shi X, Ni Z, Ding N, et al. Tumor-infiltrating immune cells act as a marker for prognosis in colorectal cancer. Front Immunol. Frontiers Media S.A. 2019;10:2368. PMID: 31681276; PMCID: PMC6811516. doi: 10.3389/fimmu.2019.02368..
- Ouzounova M, Lee E, Piranlioglu R, El Andaloussi A, Kolhe R, Demirci MF, Marasco D, Asm I, Chadli A, Hassan KA, et al. Monocytic and granulocytic myeloid derived suppressor cells differentially regulate spatiotemporal tumour plasticity during metastatic cascade. Nat Commun. 2017;8:14979. doi:10.1038/ncomms14979.
- Cafri G, Yossef R, Pasetto A, Deniger DC, Lu Y-C, Parkhurst M, Gartner JJ, Jia L, Ray S, Ngo LT, et al. Memory T cells targeting oncogenic mutations detected in peripheral blood of epithelial cancer patients. Nat Commun. 2019;10:449. doi:10.1038/s41467-019-08304-z.
- Becht E, De Reyniès A, Giraldo NA, Pilati C, Buttard B, Lacroix L, Selves J, Sautès-Fridman C, Laurent-Puig P, Fridman WH, et al. Immune and stromal classification of colorectal cancer is associated with molecular subtypes and relevant for precision immunotherapy. Clin Cancer Res. American Association for Cancer Research Inc. 2016;22:4057–4066. doi:10.1158/1078-0432.CCR-15-2879.
- Karpinski P, Rossowska J, Sasiadek MM. Immunological landscape of consensus clusters in colorectal cancer. Oncotarget. 2017;8:105299–105311. doi:10.18632/oncotarget.22169.
- Cristescu R, Mogg R, Ayers M, Albright A, Murphy E, Yearley J, Sher X, Liu XQ, Lu H, Nebozhyn M, et al. Pan-tumor genomic biomarkers for PD-1 checkpoint blockade–based immunotherapy. Science. 2018;362:eaar3593. doi:10.1126/science.aar3593.
- Baraniskin A, Buchberger B, Pox C, Graeven U, Holch JW, Schmiegel W, Heinemann V. Efficacy of bevacizumab in first-line treatment of metastatic colorectal cancer: A systematic review and meta-analysis. Eur J Cancer. 2019;106:37–44. doi:10.1016/j.ejca.2018.10.009.
- Butterfield LH, Disis ML, Fox BA, Kaufman DR, Khleif SN, Wang E. SITC 2018 workshop report: immuno-oncology biomarkers: state of the art. J Immunother Cancer. BioMed Central. 2018;6:138. doi:10.1186/s40425-018-0453-4.