ABSTRACT
Objectives: Clinical benefits of immune-checkpoint blockade (ICB) versus standard chemotherapy have been established in unselected non-small cell lung cancer (NSCLC). However, the response to ICB therapy among patients is heterogeneous in clinical practice.
Materials and Methods: We retrospectively assessed the predicitive effect of the primary and metastatic lesion spectrum (baseline sum of the longest diameters [SLD], number of metastatic sites and specific organ metastases) on the efficacy of atezolizumab over docetaxel in OAK and POPLAR trial cohorts. A decision model, termed DSO (Diameter-Site-Organ), based on the spectrum was developed and validated for guiding ICB.
Results: Higher SLD (>38 mm) and more metastatic sites (≥2) were characterized with pronounced overall survival (OS) benefits from atezolizumab versus docetaxel. Specifically, adrenal gland and brain metastases were identified as favorable predictors of atezolizumab treatment. The DSO model was developed in the discovery cohort to integrate the directive effect of the primary and metastatic lesion spectrum. Remarkably, a general pattern of enhanced efficacy of atezolizumab versus docetaxel was observed along with the increase of the DSO score. For patients with DSO score > 0, atezolizumab yielded a significantly prolonged OS than docetaxel, whereas OS was generally similar between two treatments in patients with DSO score ≤ 0. Equivalent findings were also seen in the internal and external validation cohorts.
Conclusions: The response to anti-PD-L1 therapy among patients varied with the primary and metastatic lesion spectrum. The DSO-based system might provide promising medication guidance for ICB treatment in NSCLC patients.
Introduction
Recent advancements in cancer immunotherapies have revolutionized the treatment of non-small cell lung cancer (NSCLC), such as the development of anti-programmed cell death 1 (PD-1) and anti-programmed cell death ligand 1 (PD-L1) antibodies as immune-checkpoint inhibitors (ICI). While there is a significant increase in the likelihood of achieving durable clinical benefit, outcomes are still relatively poor for patients with previously treated, advanced, or metastatic NSCLC. Food and Drug Administration (FDA) licenses for nivolumab, pembrolizumab, and atezolizumab in the second-line treatment of advanced-stage NSCLC have been granted based on improvements in overall survival (OS) versus that observed with docetaxel.Citation1 Whereas only the trial of pembrolizumab required a PD-L1 tumor proportional score (TPS) ≥ 1%, with the highest proportion of responders of 20%.Citation2 The trials of the other agents demonstrated superior outcomes in unselected patient populations, though with a dismal proportion of responders.Citation3,Citation4 Thus, numerous parameters predicting ideal candidates for second-line ICI treatment have been identified as potential markers,Citation5 encompassing body mass index (BMI),Citation6 neutrophil and lymphocyte count number (LIPI),Citation7,Citation8 C-reactive protein (CRP),Citation9 patient-reported physical function.Citation10 Still, efforts to establish effective markers from other dimensions, specially from image examinations,Citation11 are warranted to complement the field and promote precision medicine.
Patients with advanced NSCLC represented increasing tumor heterogeneity and gene profile alteration, leading to a discrepant response to standard treatment. Docetaxel chemotherapy was generally considered to benefit those with good performance status, lower tumor burden and good-tolerance to cytotoxic drugs,Citation12 while immune-checkpoint blockade (ICB) therapy was mostly favorable to those with an activated immune microenvironment and continuous antigen exposure.Citation13 Notably, a recent study has demonstrated tumor size was positively associated with antigen burden and T-cell reinvigoration, which reflected the anti-tumor immunity.Citation14 Nevertheless, tumor size was also regarded as a negative prognostic factor of survival outcomes in patients with advanced NSCLC.Citation15 These findings indicated that baseline tumor size may serve as a compounding predictor for the prognostic survival and the efficacy of treatment.
Generally, a primary and metastatic lesion spectrum can be characterized by the tumor size of the primary and metastatic lesions (sum of the longest diameters, SLD), the number of lesions, and the specific metastatic status of each organ. Differences in anatomical location of metastases have been reported to affect immunotherapeutic efficacy.Citation16,Citation17 Just as cancers arise from different organs with different genetic features, each organ has its own immune system with different immunologic features; how cancers interact within their respective immune contextures could ultimately help to personalize ICB for patients. Previous studies suggested that patients with poor prognostic factors, including bone and liver metastases, appeared to have poor outcomes in ICB monotherapy.Citation18,Citation19 Nonetheless, these reports generally focused on a particular metastatic organ and thus presented limited information on clinical practice.
In the present study, we investigated the predictive effect of the primary and metastatic lesion spectrum on the efficacy of atezolizumab over docetaxel therapy, and furtherly developed a decision model based on the spectrum to screen the best beneficiaries in advanced NSCLC patients across randomized OAK and POPLAR trials, with the aim of identifying and validating a signature that could predict long-term survival benefits of immunotherapy over standard-of-care chemotherapy.
Materials and methods
Patient population
Patients with a diagnosis of advanced NSCLC enrolled in the phase III OAK (NCT02008227)Citation4 and phase II POPLAR (NCT01903993)Citation3 trials were included in the present study for secondary analysis. These two studies were randomized second-line clinical trials of atezolizumab, 1200 mg, vs docetaxel, 75 mg/m2, with both administered intravenously every 3 weeks for patients with advanced NSCLC in whom platinum-containing therapy had failed.Citation3,Citation4 Both the OAK and the POPLAR trials were done in full accordance with the guidelines for Good Clinical Practice in a manner aligned with the Declaration of Helsinki.Citation3,Citation4,Citation20 The study was approved by the Institutional Ethical Review Boards of Nanfang Hospital (NFEC-2021-003). Informed consent was not required for our present study because of the retrospective character.
Deidentified patient-level clinical data, as well as variant calls for blood-based tumor mutation burden (bTMB), of the OAK and POPLAR trials were obtained from a previously published study and from the F. Hoff mann-La Roche Ltd, Genentech, Inc. according to Roche’s policy and process for clinical study data sharing.Citation21,Citation22 The sum of the longest diameters (SLD) in baseline was measured based on Response Evaluation Criteria in Solid Tumors (RECIST, version 1.1) guidelines.Citation3,Citation4,Citation23 The number of metastases and the specific metastatic sites (including liver, brain, adrenal gland, mediastinum, pleura, and pleural effusion) per patient was recorded in baseline. PD-L1 expression was prospectively measured using the SP142 immunohistochemistry assay, and PD-L1 strong expression was defined as the percentage of PD-L1 expression of ≥50% on tumor cells (TC3) or ≥10% on immune cells (IC3).Citation3,Citation4 bTMB was evaluated using the FoundationOne assay, with a panel comprising 1.1 Mb of coding region in the genome, as is described in the previous study.Citation21
Study design
The primary and metastatic lesion spectrum of a patient encompasses SLD, number of metastases, and organ-specific metastatic status in baseline. We first evaluated the predictive effect of the primary and metastatic lesion spectrum on the efficacy of atezolizumab versus docetaxel, from the perspectives of its three components. Next, we proposed a machine-learning based decision system, termed DSO (Diameter-Site-Organ), integrating the instructive value of the primary and metastatic lesion spectrum to guide treatment strategy of NSCLC patients, i.e. atezolizuamb or docetaxel (). The DSO model was initially developed in the OAK discovery cohort, and its generalization capacity was then evaluated in the OAK internal validation cohort and the POPLAR external validation cohort (). The overall survival (OS) was defined as the primary outcome of the study.
Figure 1. Flow Chart of the study design
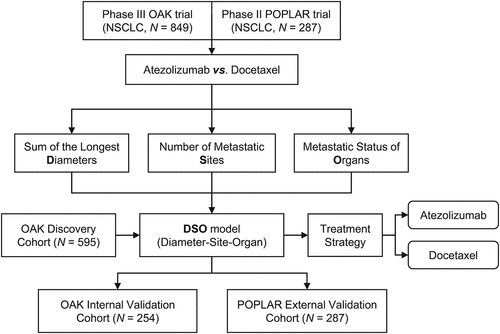
Integrative modeling with machine learning
The baseline spectrum of primary and metastatic lesions was used as the input indicator for the machine learning-based model. Before the development of the model, the OAK trial data were randomly partitioned into discovery and internal validation cohorts at a 7:3 ratio using stratified sampling; the POPLAR trial data were assigned as the external validation cohort. Demographic and clinicopathologic characteristics of the three cohorts were provided (Table S1).
Extreme Gradient Boosting (XGBoost)Citation24 was implemented to set up regressors in predicting OS of atezolizumab (termed atezolizumab regressor) and docetaxel (termed docetaxel regressor). The atezolizumab regressor and the docetaxel regressor were trained in the atezolizumab-treated subgroup and the docetaxel-treated subgroup of the discovery cohort respectively; the predictive value of the two regressors was confirmed in the atezolizumab-treated subgroup and docetaxel-treated subgroup of the three cohorts successively (Supplementary Methods, Figure S1-3, and Table S2). The final model, termed DSO (sum of the longest Diameter-number of metastatic Sites-metastatic Organs), integrated the results from the two regressors (denoted as Atezo score and Doce score respectively) by subtraction operation, and thus outputted the DSO score. The directive capacity of the DSO model for medication guidance was first evaluated in the discorvery cohort and then validated in the internal and external validation cohorts, during which the association between DSO score and the efficacy of atezolizumab versus docetaxel was explored.
Statistical analysis
The Kaplan-Meier method with the log-rank test were conducted to compare survival probabilities between subgroups. Hazard ratios (HR) with 95% confidence intervals (CI) were reported. GraphPad Prism (version 8.0.1) was utilized to draw survival curves, and Review Manager version 5.3 (RevMan, Cochrane Collaboration, Oxford, England) for forest plots. Stratified sampling, data preprocessing, and the development of the DSO model were performed in R (version 3.6.1) unless otherwise specified; XGBoost regressors were developed with R package xgboost. The Fisher’s exact test and the Wilcoxon rank-sum test were used to examine the between-group differences per organ-specific metastatic status for the percentage of PD-L1 strong expression and the distribution of bTMB, respectively. A comparison of DSO score between subgroups was performed using the unpaired t test and visualized by GraphPad Prism. Cox proportional regression was performed to calculate the P value of the treatment-by-biomarker interaction term in the intention-to-treat (ITT) population using R package survival. The relationship between clinical characteristics and DSO score was assessed using the t-test or the Fisher’s exact test. All P values were based on a two-tailed test and P ≤ 0.05 was considered statistically significant.
Results
Baseline tumor burden for the evaluation of atezolizumab treatment
A total of 1136 individuals were pooled across OAK and POPLAR trials to explore the impact of baseline tumor burden of primary and metastatic lesions on the efficacy of atezolizumab versus docetaxel in the second-line setting of NSCLC.
Although higher SLD was identified as an unfavorable predictive factor for both atezolizumab (P < .001; ) and docetaxel (P < .001; ), the OS in atezolizumab-treated patients was generally similar compared to those receiving docetaxel regimen, among patients with SLD in the lowest quartile (1st quartile: HR 0.88, 95% CI 0.63–1.23; ). As a contrast, atezolizumab consistently demonstrated an OS benefit versus docetaxel in individuals with higher SLD (2nd quartile: HR 0.65, 95% CI 0.49–0.86; 3rd quartile: HR 0.65, 95% CI 0.49–0.86; 4th quartile: HR 0.71, 95% CI 0.54–0.93; ).
Figure 2. Efficacy of atezolizumab versus docetaxel according to baseline tumor size and number of metastases
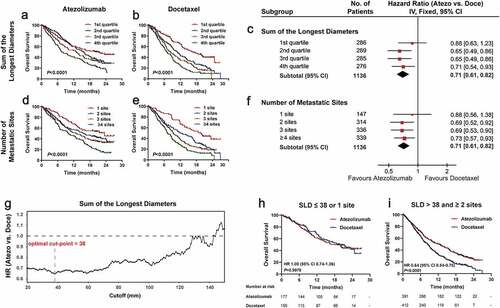
Likewise, for the number of metastatic sites, more metastatic sites were inversely correlated with clinical outcomes for both atezolizumab (P < .001; ) and docetaxel (P < .001; ). However, no significant difference in OS was observed between treatments in patients with one metastatic site (1 site: HR 0.88, 95% CI 0.56–1.38; ), whereas an OS benefit derived from atezolizumab versus docetaxel was seen in patients with at least two metastatic sites (2 sites: HR 0.69, 95% CI 0.52–0.92; 3 sites: HR 0.69, 95% CI 0.53–0.90; ≥4 sites: HR 0.73, 95% CI 0.57–0.93; ).
To determine an exact cut-point of SLD for the comparison of atezolizumab and docetaxel, we calculated the hazard ratios of atezolizumab versus docetaxel with a series of optional cutoff values; the best clinical benefit of atezolizumab over docetaxel was achieved when the cutoff value was equal to 38 mm (). Accordingly, exploratory analysis was performed based on the combination of the number of metastatic sites (1 site or ≥2 sites) and SLD (≤38 or >38) (Figure S4); there was no significant difference of OS between atezolizumab and docetaxel in the group with relatively lower tumor burden (SLD ≤ 38 or 1 site: HR 1.00, 95% CI 0.74–1.36, P = .9978; ), but a predominant OS advantage of atezolizumab over docetaxel was found in the group with higher tumor burden (SLD > 38 and ≥2 sites: HR 0.64, 95% CI 0.54–0.75, P < .0001; ).
Metastatic organs for the outcome of atezolizumab treatment
Given the critical roles of metastatic sites in advanced NSCLC, we further explored the predictive value of the metastatic spectrum on long-term survival benefits of atezolizumab over docetaxel. It is noteworthy that patients harboring different organ metastases showed different efficacy tendencies between the two treatments.
Specifically, adrenal gland metastasis exerted no obvious influence on the survival benefits of patients treated with atezolizumab (HR 0.92, 95% CI 0.67–1.26, P = .5995; ). In parallel, OS was shorter among docetaxel-treated patients with adrenal gland metastasis relative to those without adrenal gland metastasis (HR 1.46, 95% CI 1.06–2.00, P = .0082; ). Intriguingly, a direct comparison of the efficacy of the two treatments showed that atezolizumab resulted in a significantly greater OS benefit relative to docetaxel (HR 0.53, 95% CI 0.36–0.79, P = .0012; ). Additonally, OS benefit was similar based on the presence or absence of brain metastasis regardless of atezolizumab (HR 0.87, 95% CI 0.61–1.25, P = .4816; ) or docetaxel (HR 1.16, 95% CI 0.83–1.61, P = .3574; ). Yet it’s worth noting that favorable long-term survival prospects of atezolizumab over docetaxel were demonstrated in the overall population with brain metastasis (HR 0.55, 95% CI 0.35–0.88, P = .0115; ).
Figure 3. Efficacy of atezolizumab versus docetaxel according to specific metastatic organs
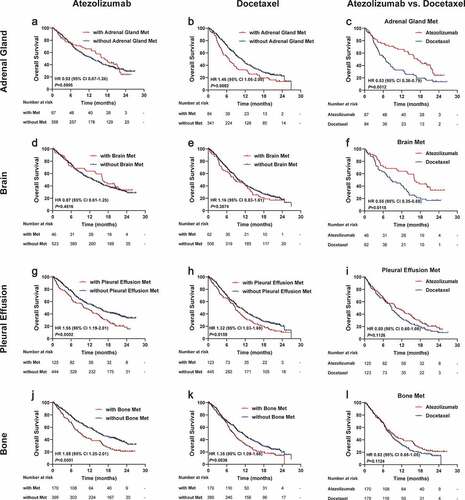
As a contrast, the presence of malignant pleural effusion (), bone metastasis (), and liver metastasis (Figure S5a-b) yielded unfavorable survival prospects irrespective of receiving atezolizumab or docetaxel treatment. But OS only trended longer in the atezolizumab arm compared with the docetaxel arm, without reaching a conventional level of statistical significance (, and Figure S5c). With respect to pleural metastasis and mediastinum metastasis, OS trended shorter in patients with either the two metastases (Figure S5d-e and S5g-h), and both displayed a nonsignificant trend favoring atezolizumab relative to docetaxel (Figure S5f and S5i).
Development of the Diameter-Site-Organ (DSO) model
In virtue of the instructional significance of the primary and metastatic lesion spectrum to therapeutic decision-making of advanced NSCLC in the second-line setting, we thus set out to propose a machine learning-based model incorporating baseline SLD, the number of metastatic sites, and metastatic organs (DSO model) for assisting medication guidance. To begin with, the OAK trial data were divided into a discovery cohort (N = 595) and an internal validation cohort (N = 254) with stratified sampling, while the POPLAR trial data were reserved as an external validation cohort (N = 287) (Table S1). The DSO model was developed in the discovery cohort (Supplementary Methods).
In the DSO-based system, patients initially received imaging evaluation to acquire the primary and metastatic lesion spectrum of tumors, including the information of SLD, metastatic sites and metastatic organs, which was used as input for two independent OS regressors to predict an Atezo score and a Doce score for each patient. By subtracting the Atezo score and the Doce score, a final DSO score was generated as the expected clinical benefit of atezolizumab versus docetaxel for the patient; therefore, patients with DSO score ≤0 (Atezo score ≤ Doce score) would prefer docetaxel, while those with DSO score > 0 (Atezo score > Doce score) would prefer atezolizumab ().
Figure 4. Development of the DSO model for medication guidance for patients with NSCLC in the discovery cohort
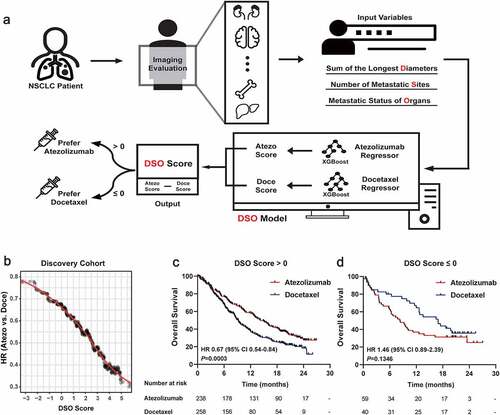
The instructional significance of the DSO model was first evaluated in the discovery cohort (N = 595). Critically, as the DSO score increased, the HR value of atezolizumab versus docetaxel decreased gradually (), suggesting that the DSO score, to a certain extent, could reflect the degree to which atezolizumab was superior to docetaxel for the patient. Subgroup analyses were performed with Kaplan-Meier estimates of OS categorized by the DSO score; long-term clinical benefit with atezolizumab compared to docetaxel was demonstrated in patients with DSO score > 0 (HR 0.67, 95% CI 0.54–0.84, P = .0003; ). Meanwhile, although no significant difference was observed between treatments in patients with DSO score ≤ 0, there even appeared a trend that docetaxel yielded better survival than atezolizumab in these patients according to the hazard ratios (HR 1.46, 95% CI 0.89–2.39, P = .1346; ).
Generalization performance of the DSO model in the validation cohorts
To verify the generalization of the model, we further evaluated the predictive effect of the DSO model on the efficacy of atezolizumab versus docetaxel, successively in the internal validation cohort (N = 254) and the external validation cohort (N = 287).
In a similar vein, the HR of atezolizumab versus docetaxel decreased in magnitude as the DSO socre increased, indicative of a positive association of the DSO score with the long-term survival benefits of atezolizumab over docetaxel in the internal validation cohort (). Significantly, among patients with DSO score > 0, atezolizumab resulted in a greater OS benefit as compared to docetaxel (HR 0.58, 95% CI 0.42–0.81, P = .0015; ). Concurrently, survival prospects in atezolizumab-treated patients with DSO score ≤ 0 were similar to those in the docetaxel-treated patients (HR 1.08, 95% CI 0.52–2.24, P = .8402; ).
Figure 5. Internal and external validation of the DSO model for guiding immune checkpoint therapy
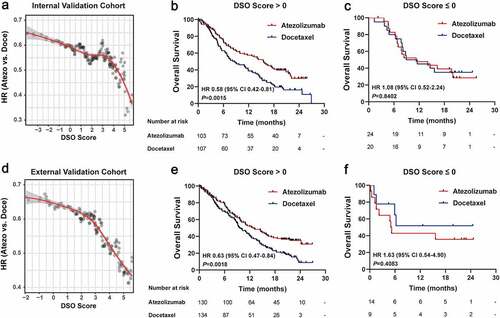
In analogy, the findings were replicated in the external validation cohort. It is notable that the DSO score was aslo positively associated with the efficacy of atezolizumab versus docetaxel (). Within the exteranl cohort, OS consistently favored atezolizumab over docetaxel in patients with DSO score > 0 (HR 0.63, 95% CI 0.47–0.84, P = .0018; ), whereas patients with DSO score ≤ 0 presented a nonsignificant trend favoring docetaxel (HR 1.63, 95% CI 0.54–4.90, P = .4083; ).
Moreover, we also investigated the efficacy of atezolizumab versus docetaxel in subgroups defined by different DSO score, with a continuous cut-points of DSO score from 1 to 5. Results showed that the DSO-high group demonstrated more superiority of atezolizumab over docetaxel along with the increase of cut-point (Figure S6).
Reverse engineering and interpretation of the DSO model
The association analysis was conducted to explore the interpretability of the DSO model from the perspective of the primary and metastatic lesion spectrum. Within the subgroup with SLD ≤ 38 and 1 metastatic site, almost all of the patients obtained a DSO score < 0; by comparison, a majority of patients with SLD > 38 or ≥ 2 sites obtained a DSO score > 0 (). In addition, the dual positive subgroup (SLD > 38 and ≥2 sites) obtained a significantly higher DSO score in average than either the single positive (SLD ≤ 38 or 1 site) (P < .0001) or the dual negative subgroup (SLD ≤ 38 and 1 site) (P < .0001) (). Simultaneously, DSO scores were compared among subgroups categorized by specific organ metastases. Overall, a general pattern of a higher DSO score in average was observed in patients harboring adrenal gland metastasis or brain metastasis, compared to those with other organ metastases ().
Figure 6. Correlational analyses of DSO score with tumor size, metastatic lesion number and metastatic organs
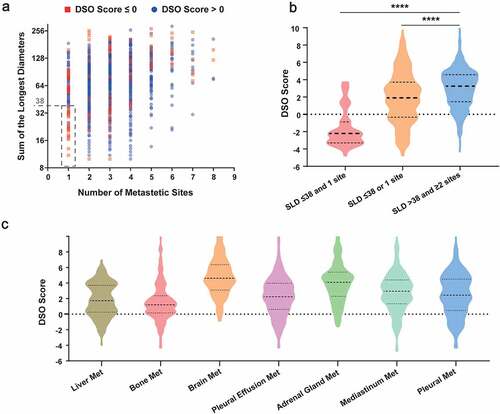
Furthermore, the interation analysis revealed that the OS benefit of atezolizumab versus docetaxel was significantly influenced by tumor burden (P [interaction] = 0.0123; Table S3); the interaction between treatment and metastatic organs was also significant (P [interaction] = 0.0292; Table S4). Similar analysis was performed between treatment and the output of the DSO model, where a significant heterogeneity of treatment effect was observed as expected (DSO score: P [interaction] < 0.0001, Table S5; DSO group: P [interaction] = 0.0005, Table S6).
Discussion
Recently, considerable successes have been witnessed in advanced nonselective NSCLC patients receiving ICB therapy, but still, a large proportion of patients cannot derive durable benefits from it; effective and easily accessible biomarkers that can offer clinical guidance are thus highly needed.
Baseline tumor burden has been demonstrated as a predictor of poor survival for immunotherapy in the previous studies.Citation15,Citation25 However, this conclusion was not derived from the direct comparison between immunotherapy and chemotherapy, and therefore overlooked its prognostic effect.Citation15 Herein, we for the first time proposed that patients with higher tumor burden tend to derive more benefits from immunotherapy compared with chemotherapy. The somewhat surprising finding may be justified by multiple reasons. First, patients harboring higher tumor burden are often accompanied with compromised performance statusCitation12 and cancer-associated cachexia,Citation26,Citation27 both of which markedly increased toxic adverse events and decreased tolerance when undergoing chemotherapy.Citation12,Citation28,Citation29 As a contrast, immunotherapy provides a more favorable safety and tolerability profile even in patients with poor performance status.Citation30,Citation31 Besides, instead of a direct cytotoxicity on tumor tissue, immune checkpoint inhibitors exert an indirect effect in immune regulation and pertinently provide durable benefits for patients harboring high tumor burden, who are characterized with an immunosuppressive microenvironment induced by the upregulation of PD-1/PD-L1.Citation32 Moreover, previous research has put forward a positive correlation between baseline tumor burden and CD8+ T-cell reinvigoration after ICB therapy, suggesting that the anti-tumor effect induced by immunotherapy could be augmented along with the increase of tumor burden.Citation14
Researches probing into the seed (cancer cell) versus the soil (invaded organ) have illustrated that the immune landscape differs greatly in different organs,Citation33,Citation34 which will no doubt influence anti-tumor immunity.Citation35 Unraveling organ-specific immunity holds great promise in promoting the development of precise immunotherapeutic strategy. Specifically for brain metastasis, the limited access for cytotoxic agents to penetrate the blood brain barrier substantially restrict the effect of chemotherapy;Citation36 apart from that, the microenvironment landscape of brain metastasis characterized with an accumulation of tumor-infiltrating lymphocytesCitation37 determines a pronounced response to immunotherapy, while at the same time inducing an astrocyte-mediated resistance to chemotherapy through the upregulation of GSTA5, BCL2L1, and TWIST1;Citation36,Citation38 therefore, the presence of brain metastasis predicts a significant benefit from immunotherapy versus chemotherapy. As regards adrenal gland metastasis, patients harboring this kind of organ metastasis yields prolonged survival with immunotherapy compared to chemotherapy, which might be attributed to the higher proportion of PD-L1 strong expression (metastasis vs. non-metastasis: 23.33% vs. 14.74%, P = .0142; Figure S7b) and the upregulated bTMB (metastasis vs. non-metastasis: 11 vs. 7 mutations/Mb in median, P = .0001; Figure S7b). On the contrary, for those whose tumors metastasized to bone, pleura, or pleural effusion, the relatively low levels of bTMB and PD-L1 (Figure S7a-b), which are responsible for the innate immune suppression, lend a potential mechanistic basis to the limited benefit derived from immune checkpoint inhibitors in these patients. Collectively, this study demonstrated that the occurrence of specific organ metastases could provide medication guidance for advanced NSCLC, and offered new clues for translational researches into underlying mechanisms.
One of the novelties of the present study manifests in the innovation of the model. To the best of our knowledge, this is the first machine-learning based model capable to measure the degree of the survival benefits derived from atezolizumab over docetaxel through integrative evaluation of the primary and metastatic lesion spectrum. Of note, the DSO model was an assistant decision-making model for medication guidance rather than a prediction model for a particular treatment, thus distinguishing it from others as well as enabling it to resolve the defects of traditional prediction models. The traditional prediction model that developed merely in the atezolizumab-treated population might neglect the prognosis of patients themselves and caused misunderstandings. To resolve this defect, the DSO model was developed based on two prediction model, namely the atezolizumab regressor and the docetaxel regressor; by subtracting the predicted scores from the two regressors, we can thus have a direct comparison of atezolizumab versus docetaxel, and tell which treatment is more appropriate for each patient according to the DSO score. To further demonstrate the advantage of the DSO model over classical indicators, the instructive values of blood-based TMB (bTMB) and PD-L1 expression level were investigated. Interestingly, there was no evidence that the efficacy of atezolizumab versus docetaxel promoted with the increase of bTMB (Figure S8b) or PD-L1 expression level (Figure S8c); as a contrast, a general pattern of enhanced efficacy of atezolizumab versus docetaxel was observed, along with the increase of DSO score (Figure S8a). Last but not least, the association of DSO score with tumor burden and metastatic organs, as well as their interaction effects with treatment were analyzed for reverse-engineering and interpretation, explaining the instructive value of the machine learning-based model for medication guidance; meanwhile, the distribution of other clinical characteristics was generally balanced between groups categorized by DSO score (Table S7).
Another novelty manifests in the advantages of the primary and metastatic lesion spectrum for medication guidance compared to other metrics. To be specific, regarding accessibility, baseline tumor burden and metastatic sites are readily available at diagnosis through image examinations without additional and subsequent tests, whereas early tumor shrinkage should be assessed at the six-week visit.Citation11 As for stability, the primary and metastatic lesion spectrum would not be disturbed by the short-term physical condition, whilst hematological markers (e.g. LIPICitation7,Citation8 and CRPCitation10) might be affected by infection, trauma, and the usage of glucocorticoids or antibiotics, etc. As regards objectivity, the baseline metastatic lesion number and the metastatic sites would not be interfered by subjective judgment, as compared with patient-reported outcomes.Citation10 Even so, we believe that the combination of multi-dimensional information would be the mainstay of future precision medicine, and the primary and metastatic lesion spectrum might serve as another dimension to complement with the identified markers.
There are several limitations in this study. Firstly, baseline metastatic status of adrenal gland, pleura and mediastinum, as well as PD-L1 expression level were not available in the POPLAR trial data because of data missing; on the other hand, however, the external validation cohort could, to some extent, better simulate and reflect the clinical practice, where not every patient could receive a thorough imaging evaluation to assess the systemic cancer spread. In addition, the present study did not evaluate the effect of the primary and metastatic lesion spectrum on safety and tolerability profile of atezolizumab versus docetaxel because of insufficient data, which deserved efforts in ongoing researches in the OAK and POPLAR data and also real-world data. Besides, the difference in the selection of target lesions for measuring SLD might lead to the between-scorer variability.Citation15 Lastly, the role of ICB treatment in NSCLC is currently moved to the first-line setting;Citation39 nonetheless, the conclusions on the primary and metastatic lesion spectrum drew from the study might also provide implications for improving the understanding of first-line regimens, and the approach for developing a decision model for screening beneficiaries of ICB treatment could also be applied in the first-line setting.
Conclusions
This study revealed the predictive and instructional capacity of the baseline SLD, the number of metastatic sites, and specific organ metastases, through a direct comparison between atezolizumab and docetaxel in NSCLC. The DSO model based on the primary and metastatic lesion spectrum might provide medication guidance for ICB in second-line NSCLC patients, and might as well improve the understanding of first-line immune checkpoint therapy.
Abbreviations
Competing interests:
The authors have no actual or potential conflicts of interest to declare.
Ethics approval and consent to participate
The study was approved by the Institutional Ethical Review Boards of Nanfang Hospital. All patients enrolled in OAK and POPLAR provided signed informed consent in accordance with the protocols of the corresponding studies.
Supplemental Material
Download ()Acknowledgments
We would like to thank Professor David S. Shames and Dr Jing-Yu Zhang from Genentech, Inc. for helping us with signing the data-sharing agreement, which enabled us to get the individual clinical data of the OAK and POPLAR clinical trials.
Availability of data and materials
Patient-level clinical variables (including the SLD, the number of metastatic sites, and PD-L1 expression level), survival outcome, and bTMB in OAK and POPLAR studies were accessible from a previously published study as describe in the Materials and Methods section. Data concerning the metastatic status of each specific organ were applied from the F. Hoff mann-La Roche Ltd, Genentech, Inc. after signing a data-sharing agreement, according to Roche’s policy and process for clinical study data sharing.
Supplementary material
Supplemental data for this article can be accessed on the publisher’s website
Additional information
Funding
References
- Camidge DR, Doebele RC, Kerr KM. Comparing and contrasting predictive biomarkers for immunotherapy and targeted therapy of NSCLC. Nat Rev Clin Oncol. 2019;16(6):341–11. doi:10.1038/s41571-019-0173-9.
- Herbst RS, Baas P, Kim DW, Felip E, Perez-Gracia JL, Han JY, Molina J, Kim J-H, Arvis CD, Ahn M-J, et al. Pembrolizumab versus docetaxel for previously treated, PD-L1-positive, advanced non-small-cell lung cancer (KEYNOTE-010): a randomised controlled trial. Lancet. 2016;387(10027):1540–1550. doi:10.1016/S0140-6736(15)01281-7.
- Fehrenbacher L, Spira A, Ballinger M, Kowanetz M, Vansteenkiste J, Mazieres J, Park K, Smith D, Artal-Cortes A, Lewanski C, et al. Atezolizumab versus docetaxel for patients with previously treated non-small-cell lung cancer (POPLAR): a multicentre, open-label, phase 2 randomised controlled trial. Lancet. 2016;387(10030):1837–1846. doi:10.1016/S0140-6736(16)00587-0.
- Rittmeyer A, Barlesi F, Waterkamp D, Park K, Ciardiello F, Von Pawel J, Gadgeel SM, Hida T, Kowalski DM, Dols MC, et al. Atezolizumab versus docetaxel in patients with previously treated non-small-cell lung cancer (OAK): a phase 3, open-label, multicentre randomised controlled trial. Lancet. 2017;389(10066):255–265. doi:10.1016/S0140-6736(16)32517-X.
- Havel JJ, Chowell D, Chan TA. The evolving landscape of biomarkers for checkpoint inhibitor immunotherapy. Nat Rev Cancer. 2019;19(3):133–150. doi:10.1038/s41568-019-0116-x.
- Kichenadasse G, Miners JO, Mangoni AA, Rowland A, Hopkins AM, Sorich MJ. Association between body mass index and overall survival with immune checkpoint inhibitor therapy for advanced non-small cell lung Cancer. JAMA Oncol. 2020;6(4):512–518. doi:10.1001/jamaoncol.2019.5241.
- Sorich MJ, Rowland A, Karapetis CS, Hopkins AM. Evaluation of the lung immune prognostic index for prediction of survival and response in patients treated with atezolizumab for NSCLC: pooled analysis of clinical trials. J Thorac Oncol. 2019;14(8):1440–1446. doi:10.1016/j.jtho.2019.04.006.
- Mezquita L, Auclin E, Ferrara R, Charrier M, Remon J, Planchard D, Ponce S, Ares LP, Leroy L, Audigier-Valette C, et al. Association of the lung immune prognostic index with immune checkpoint inhibitor outcomes in patients with advanced non-small cell lung cancer. JAMA Oncol. 2018;4(3):351–357. doi:10.1001/jamaoncol.2017.4771.
- Hopkins AM, Kichenadasse G, Garrett-Mayer E, Karapetis CS, Rowland A, Sorich MJ. Development and validation of a prognostic model for patients with advanced lung cancer treated with the immune checkpoint inhibitor atezolizumab. Clin Cancer Res. 2020;26(13):3280–3286. doi:10.1158/1078-0432.CCR-19-2968.
- Hopkins AM, Wagner J, Kichenadasse G, Modi N, Rowland A, Sorich MJ. Patient-reported outcomes as a prognostic marker of survival in patients with advanced nonsmall cell lung cancer treated with immunotherapy. Int J Cancer. 2020;147(11):3085–3089. doi:10.1002/ijc.33133.
- Hopkins AM, Kichenadasse G, Karapetis CS, Rowland A, Sorich MJ. Early tumor shrinkage identifies long-term disease control and survival in patients with lung cancer treated with atezolizumab. J Immunother Cancer. 2020;8(1):e000500. doi:10.1136/jitc-2019-000500.
- Clinical practice guidelines for the treatment of unresectable non-small-cell lung cancer. Adopted on May. 16, 1997. by the American society of clinical oncology. J Clin Oncol. 15(8):2996–3018. Doi:10.1200/JCO.1997.15.8.2996.
- Galluzzi L, Chan TA, Kroemer G, Wolchok JD, Lopez-Soto A. The hallmarks of successful anticancer immunotherapy. Sci Transl Med. 2018;10(459):eaat7807. doi:10.1126/scitranslmed.aat7807.
- Huang AC, Postow MA, Orlowski RJ, Mick R, Bengsch B, Manne S, Xu W, Harmon S, Giles JR, Wenz B, et al. T-cell invigoration to tumour burden ratio associated with anti-PD-1 response. Nature. 2017;545(7652):60–65. doi:10.1038/nature22079.
- Hopkins AM, Kichenadasse G, McKinnon RA, Rowland A, Sorich MJ. Baseline tumor size and survival outcomes in lung cancer patients treated with immune checkpoint inhibitors. Semin Oncol. 2019;46(4–5):380–384. doi:10.1053/j.seminoncol.2019.10.002.
- Tamiya M, Tamiya A, Inoue T, Kimura M, Kunimasa K, Nakahama K, Taniguchi Y, Shiroyama K, Isa SI, Nishino K, et al. Metastatic site as a predictor of nivolumab efficacy in patients with advanced non-small cell lung cancer: a retrospective multicenter trial. PLoS One. 2018;13(2):e0192227. doi:10.1371/journal.pone.0192227.
- Gadgeel SM, Lukas RV, Goldschmidt J, Conkling P, Park K, Cortinovis D, de Marinis F, Rittmeyer A, Patel JD, Von Pawel J, et al. Atezolizumab in patients with advanced non-small cell lung cancer and history of asymptomatic, treated brain metastases: exploratory analyses of the phase III OAK study. Lung Cancer. 2019;128:105–112. doi:10.1016/j.lungcan.2018.12.017.
- Tumeh PC, Hellmann MD, Hamid O, Tsai KK, Loo KL, Gubens MA, Rosenblum M, Harview CL, Taube JM, Handley N, et al. Liver metastasis and treatment outcome with Anti-PD-1 monoclonal antibody in patients with melanoma and NSCLC. Cancer Immunol Res. 2017;5(5):417–424. doi:10.1158/2326-6066.CIR-16-0325.
- Vokes EE, Ready N, Felip E, Horn L, Burgio MA, Antonia SJ, Arén Frontera O, Gettinger S, Holgado E, Spigel D, et al. Nivolumab versus docetaxel in previously treated advanced non-small-cell lung cancer (CheckMate 017 and CheckMate 057): 3-year update and outcomes in patients with liver metastases. Ann Oncol. 2018;29(4):959–965. doi:10.1093/annonc/mdy041.
- Mentz RJ, Hernandez AF, Berdan LG, Rorick T, O’Brien EC, Ibarra JC, Curtis LH, Peterson ED. Good clinical practice guidance and pragmatic clinical trials: balancing the best of both worlds. Circulation. 2016;133(9):872–880. doi:10.1161/CIRCULATIONAHA.115.019902.
- Gandara DR, Paul SM, Kowanetz M, Schleifman E, Zou W, Li Y, Rittmeyer A, Fehrenbacher L, Otto G, Malboeuf C, et al. Blood-based tumor mutational burden as a predictor of clinical benefit in non-small-cell lung cancer patients treated with atezolizumab. Nat Med. 2018;24(9):1441–1448. doi:10.1038/s41591-018-0134-3.
- Strom BL, Buyse M, Hughes J, Knoppers BM. Data sharing, year 1–access to data from industry-sponsored clinical trials. N Engl J Med. 2014;371(22):2052–2054. doi:10.1056/NEJMp1411794.
- Eisenhauer EA, Therasse P, Bogaerts J, Schwartz LH, Sargent D, Ford R, Dancey J, Arbuck S, Gwyther S, Mooney M, et al. New response evaluation criteria in solid tumours: revised RECIST guideline (version 1.1). Eur J Cancer. 2009;45(2):228–247. doi:10.1016/j.ejca.2008.10.026.
- Chen T, Guestrin C XGBoost: a scalable tree boosting system. Proceedings of the 22nd ACM SIGKDD International Conference on Knowledge Discovery and Data Mining. San Francisco, California, USA: Association for Computing Machinery; 2016. p 785–794.
- Sasaki K, Morioka D, Conci S, Margonis GA, Sawada Y, Ruzzenente A, Kumamoto T, Iacono C, Andreatos N, Guglielmi A, et al. The tumor burden score: a new “metro-ticket” prognostic tool for colorectal liver metastases based on tumor size and number of tumors. Ann Surg. 2018;267(1):132–141. doi:10.1097/SLA.0000000000002064.
- de Lerma Barbaro A. The complex liaison between cachexia and tumor burden (review). Oncol Rep. 2015;34(4):1635–1649. doi:10.3892/or.2015.4164.
- Salazar-Degracia A, Granado-Martínez P, Millán-Sánchez A, Tang J, Pons-Carreto A, Barreiro E. Reduced lung cancer burden by selective immunomodulators elicits improvements in muscle proteolysis and strength in cachectic mice. J Cell Physiol. 2019;234(10):18041–18052. doi:10.1002/jcp.28437.
- Fearon K, Arends J, Baracos V. Understanding the mechanisms and treatment options in cancer cachexia. Nat Rev Clin Oncol. 2013;10(2):90–99. doi:10.1038/nrclinonc.2012.209.
- Prado CM, Baracos VE, McCargar LJ, Mourtzakis M, Mulder KE, Reiman T, Butts CA, Scarfe AG, Sawyer MB. Body composition as an independent determinant of 5-fluorouracil-based chemotherapy toxicity. Clin Cancer Res. 2007;13(11):3264–3268. doi:10.1158/1078-0432.CCR-06-3067.
- Middleton G, Brock K, Savage J, Mant R, Summers Y, Connibear J, Shah R, Ottensmeier C, Shaw P, Lee S-M, et al. Pembrolizumab in patients with non-small-cell lung cancer of performance status 2 (PePS2): a single arm, phase 2 trial. Lancet Respir Med. 2020;8(9):895–904. doi:10.1016/S2213-2600(20)30033-3.
- Spigel DR, McCleod M, Jotte RM, Einhorn L, Horn L, Waterhouse DM, Creelan B, Babu S, Leighl NB, Chandler JC; Spigel DR, McCleod M, Jotte RM, Einhorn L, Horn L, Waterhouse DM, et al. Safety, efficacy, and patient-reported health-related quality of life and symptom burden with nivolumab in patients with advanced non-small cell lung cancer, including patients aged 70 years or older or with poor performance status (checkmate 153). J Thorac Oncol. 2019;14(9):1628–1639. doi:10.1016/j.jtho.2019.05.010.
- Wu CT, Huang YC, Chen WC, Chen MF. Effect of tumor burden on tumor aggressiveness and immune modulation in prostate cancer: association with IL-6 signaling. Cancers (Basel). 2019;11(7):992. doi:10.3390/cancers11070992.
- Jiménez-Sánchez A, Memon D, Pourpe S, Veeraraghavan H, Li Y, Vargas HA, Gill MB, Park KJ, Zivanovic O, Konner J, et al. Heterogeneous tumor-immune microenvironments among differentially growing metastases in an ovarian cancer patient. Cell. 2017;170(5):927–38.e20. doi:10.1016/j.cell.2017.07.025.
- Lehmann B, Biburger M, Brückner C, Ipsen-Escobedo A, Gordan S, Lehmann C, Voehringer D, Winkler T, Schaft N, Dudziak D, et al. Tumor location determines tissue-specific recruitment of tumor-associated macrophages and antibody-dependent immunotherapy response. Sci Immunol. 2017;2(7):eaah6413. doi:10.1126/sciimmunol.aah6413.
- Salmon H, Remark R, Gnjatic S, Merad M. Host tissue determinants of tumour immunity. Nat Rev Cancer. 2019;19(4):215–227. doi:10.1038/s41568-019-0125-9.
- Quail DF, Joyce JA. The microenvironmental landscape of brain tumors. Cancer Cell. 2017;31(3):326–341. doi:10.1016/j.ccell.2017.02.009.
- Klemm F, Maas RR, Bowman RL, Kornete M, Soukup K, Nassiri S, Brouland J-P, Iacobuzio-Donahue CA, Brennan C, Tabar V, et al. Interrogation of the microenvironmental landscape in brain tumors reveals disease-specific alterations of immune cells. Cell. 2020;181(7):1643–1660.e17. doi:10.1016/j.cell.2020.05.007.
- Lin Q, Balasubramanian K, Fan D, Kim SJ, Guo L, Wang H, Bar-Eli M, Aldape KD, Fidler IJ. Reactive astrocytes protect melanoma cells from chemotherapy by sequestering intracellular calcium through gap junction communication channels. Neoplasia. 2010;12(9):748–754. doi:10.1593/neo.10602.
- Ettinger DS, Wood DE, Aggarwal C, Aisner DL, Akerley W, Bauman JR, Bharat A, Bruno DS, Chang JY, Chirieac LR, et al. NCCN guidelines insights: non-small cell lung cancer, version 1.2020. J Natl Compr Canc Netw. 2019;17(12):1464–1472. doi:10.6004/jnccn.2019.0059.