ABSTRACT
Squamous cell carcinoma of the tonsil is one of the most frequent cancers of the oropharynx. The escalating rate of tonsil cancer during the last decades is associated with the increase of high risk-human papilloma virus (HR-HPV) infections. While the microbiome in oropharyngeal malignant diseases has been characterized to some extent, the microbial colonization of HR-HPV-associated tonsil cancer remains largely unknown. Using 16S rRNA gene amplicon sequencing, we have characterized the microbiome of human palatine tonsil crypts in patients suffering from HR-HPV-associated tonsil cancer in comparison to a control cohort of adult sleep apnea patients. We found an increased abundance of the phyla Firmicutes and Actinobacteria in tumor patients, whereas the abundance of Spirochetes and Synergistetes was significantly higher in the control cohort. Furthermore, the accumulation of several genera such as Veillonella, Streptococcus and Prevotella_7 in tonsillar crypts was associated with tonsil cancer. In contrast, Fusobacterium, Prevotella and Treponema_2 were enriched in sleep apnea patients. Machine learning-based bacterial species analysis indicated that a particular bacterial composition in tonsillar crypts is tumor-predictive. Species-specific PCR-based validation in extended patient cohorts confirmed that differential abundance of Filifactor alocis and Prevotella melaninogenica is a distinct trait of tonsil cancer. This study shows that tonsil cancer patients harbor a characteristic microbiome in the crypt environment that differs from the microbiome of sleep apnea patients on all phylogenetic levels. Moreover, our analysis indicates that profiling of microbial communities in distinct tonsillar niches provides microbiome-based avenues for the diagnosis of tonsil cancer.
Introduction
Oropharyngeal squamous cell carcinoma is one of the most prevalent tumor types within the group of head-and-neck cancers.Citation1 The stark rise in the incidence of high risk-human papilloma virus (HR-HPV) infections has led to a substantial increase of tonsil cancer making the tonsillar environment the most common site of oropharyngeal cancer.Citation2,Citation3 HPV preferentially infects keratinocyte progenitors located in the basal layer of the tonsillar crypt epithelium.Citation4,Citation5 While most infected individuals clear the virus within 18 months,Citation6 viral persistence and alterations in viral gene expression, along with enhanced production of the oncogenes E6 and E7, initiate HPV-mediated carcinogenesis.Citation5 Moreover, host resistance factors, cellular composition, and the general milieu of particular epithelial niches determine the outcome of HPV infection.Citation1,Citation4,Citation7 It is thus important to determine whether specific microenvironmental conditions in tonsillar crypts are associated with tonsil cancer.
All mucosal surfaces of the human body are colonized by a plethora of microbial organisms. Bacterial communities in the oral and oropharyngeal regions are particularly complex and contain, in addition to the gastrointestinal tract, one of the most diverse microbiomes.Citation8,Citation9 Oropharyngeal bacterial communities are dominated by the six major phyla Firmicutes, Bacteroidetes, Proteobacteria, Actinobacteria, Spirochetes, and Fusobacteria representing 96% of all taxa found in the oral cavity including the oropharynx.Citation8,Citation10 Moreover, different oral structures and tissues such as the hard and soft palates, sub- and supragingival surfaces, teeth, lips, cheeks and tonsils are colonized by distinct and dynamically changing microbial communities.Citation10–12 Hence, assessment of niche-specific differences of the oral and oropharyngeal microflora is crucial for understanding the role of bacteria in oropharyngeal diseases such as tonsil cancer.
The microbiota in human tonsils shows a distinct spatial distribution with enrichment of particular bacterial species in the lymphoid tissue, whereas other species are found mainly in layers adherent to the epithelium.Citation13 However, biased approaches using rRNA targeted oligonucleotide probes for specific bacterial speciesCitation13 may introduce a technical bias leading to overrepresentation of particular bacterial species. In contrast, culture-independent molecular profiling based on 16S rRNA gene amplicon sequencing revealed only minor differences in the bacterial composition when different sampling sites in human tonsils and adenoids were compared.Citation14 Noteworthy, the majority of studies elaborating on the composition of the tonsillar microbiome have analyzed tissues from pediatric patients.Citation14–21 Tonsil cancer occurs only in adults and the few available datasets on the adult microbiome in tonsil cancer have not considered the inclusion of control cohorts.Citation22,Citation23 Here, we have analyzed the tonsillar crypt microbiome from two different patient cohorts. We have enrolled patients with HR-HPV-positive squamous cell carcinoma of the tonsil and have established a control cohort of adult patients with obstructive sleep apnea (OSA). Sampling from different tonsillar compartments (epithelial surface, crypts and lymphoid tissue parenchyma) in OSA patients revealed a homogenous microbial composition in tonsillar niches across the oropharynx within individual patients. The microbiome composition in tonsillar crypts of tonsil cancer patients was significantly different when compared to OSA patients. Random forest-based classification of the crypt microbiome data and species-specific PCR-based validation of extended cohorts revealed that certain bacterial species combinations are tumor-predictive. In sum, the data presented here suggest that the analysis of bacterial communities in tonsillar crypts and the elaboration of tumor-predictive bacterial species pattern bear the potential to aid the detection of tonsil cancer.
Results
Tonsillar microbiome diversity in healthy individuals
As a first step for the assessment of microbial alterations in tonsil cancer, we have analyzed the tonsillar microbiome of a well-defined control cohort of patients that underwent tonsillectomy due to OSA. In total, 21 OSA patients were enrolled between May 2017 and May 2020. 16S rRNA gene amplicon sequencing has been performed on the samples of the first 14 recruited patients (), while recruitment continued to reach the final cohort size (Supplementary Table 1). To assess whether the crypt microbiome represents the global tonsillar bacterial composition, we acquired two punch biopsies each from the surface epithelium, the lymphoid tissue parenchyma and the crypts (). Data from the same patient and sampling site were regarded as technical replicates and were merged in downstream bioinformatics analyses. In total, 160 biopsies have been analyzed in the OSA cohort with 130 samples remaining after quality control. We found a uniform within-sample (α-)diversity across different collection sites with the mean number of observed species and Chao1 species richness estimators showing no significant differences between the three sampling sites (). Likewise, both Shannon and Simpson indices, which denote species richness and evenness, were not significantly different (). Principle coordinate analysis using Bray-Curtis (), UNIFRAC () and weighted UNIFRAC () distance metrics did not reveal significant differences in β-diversity between the tonsillar compartments. Bacteroidetes, Fusobacteria, and Firmicutes were the dominant phyla in the three different anatomical locations (). The abundance of the various phyla did not vary significantly between the sampling sites suggesting that microbial colonization occurs in a homogenous fashion across the different tonsillar niches.
Table 1. Characteristics of patients with 16S rRNA gene amplicon-based analysis of the tonsillar microbiome in the tonsillar carcinoma and obstructive sleep apnea cohorts
Figure 1. Microbiome diversity in different tonsillar compartments of obstructive sleep apnea patients. (a) Schematic representation of the human palatine tonsil. The different sampling sites within the tonsil are indicated in gray (crypt), dark blue (epithelium) and bright blue (lymphoid tissue). (b-c) α-diversity in different sampling sites of OSA patients (n = 14 patients, only crypt samples have been available for patient 1). (b) Species richness measured in terms of observed number of species and Chao1 index. (c) Shannon and Simpson indices estimating species richness and evenness. (d-f) Principle coordinate analysis of the tonsillar microbiome in distinct sampling sites calculated using Bray–Curtis (d), UNIFRAC (e) and weighted UNIFRAC (f) distance metrics. Dots represent crypt, epithelial and lymphoid tissue biopsies of individual patients (n = 14 patients). Dotted circles represent superimposed normal probability over datapoints. (g) Bacterial community composition in different sampling locations of OSA patients at the phylum level. Statistical analysis does not reach significance (p < .05). Statistical analysis was performed using Kruskal–Wallis (b-c, g) or PERMANOVA (d-f) tests
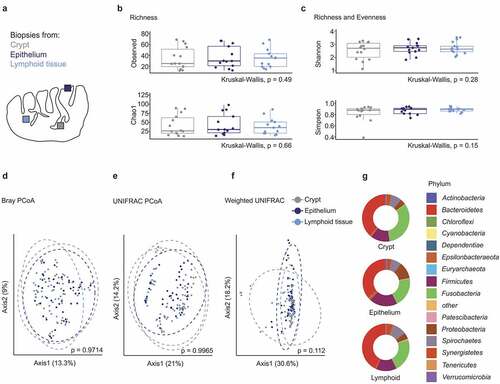
To further assess to what extent microbial community composition in tonsillar niches of individual patients is conserved, we compared the microbiome in ipsi- and contralateral tonsils of OSA patients (). Wilcoxon signed-rank testing did not indicate significant differences in species richness () or richness and evenness () in the microbiome of opposing tonsils. Analysis of β-diversity revealed a significant dissimilarity for paired PERMANOVA testing based on the Bray-Curtis distance (p = .0275) (), but not for UNIFRAC () or weighted UNIFRAC distances (). The assessment of individual patients in these analyses highlights the substantially higher variance between individual patients compared to the differences between ipsi- and contralateral tonsils (). In sum, these data indicate that tonsils of OSA patients harbor diverse bacterial communities and that the microbial composition in tonsillar niches of individual patients is similar across the oropharynx.
Figure 2. Microbial composition in ipsi- and contralateral tonsils of patients with obstructive sleep apnea. (a) Schematic representation of the oropharynx representing samples collected from right (dark blue) and left (bright blue) tonsils. (b-c) α-diversity displayed by observed number of species and Chao1 indices (b) and Shannon and Simpson indices (c). Dots represent crypt, epithelial and lymphoid tissue biopsies of individual patients (n = 14 patients). (d-f) Principle coordinate analysis calculated using Bray–Curtis (d), UNIFRAC (e) and weighted UNIFRAC (f) distance metrics. Samples of each patient are displayed by a distinct color. Dotted circles represent superimposed normal probability over data points. Statistical analysis was performed using Wilcoxon signed-rank test (b-c) or paired PERMANOVA (d-f) test
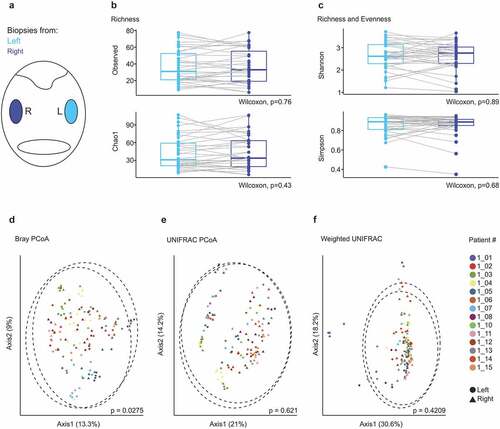
Tonsillar crypt microbiome composition in tonsil cancer
Tissue biopsies of 18 patients suffering from squamous cell carcinoma of the tonsil have been analyzed by 16S rRNA gene amplicon sequencing with almost 90% of the patients being HR-HPV-positive (). In addition to the HPV-status, age, sex, TNM tumor staging, nicotine and alcohol exposure and secondary carcinomas were documented (Supplementary Table 2). Two punch biopsies from the contralateral crypt were collected during the surgery and were included in the analysis (). The assessment of four α-diversity metrics did not reveal statistically significant differences between the crypt microbiome of tumor-affected and contralateral tonsils ( and c). Principle coordinate analysis using Bray-Curtis, UNIFRAC and weighted UNIFRAC estimators did not indicate statistically significant dissimilarities in the microbial community composition of tumor-affected vs. contralateral tonsils (). Extended analyses indicated that the severity of the disease here assessed as pathological tumor stage, had a significant effect on the β-diversity of the microbial communities in tumor patients (Supplementary Figure 1a-c). At phylum level, tumor-affected tonsils exhibited elevated (p = .056) relative abundance of Firmicutes (). The genera Fusobacterium, Prevotella, Prevoella_7 and Veillonella displayed the highest relative abundance in tonsillar crypts of tumor patients (). These data are indicative of a rather homogenous microbial colonization of the tonsillar crypts across the oropharynx in tumor patients; a finding that is in line with the results from OSA patients ().
Figure 3. The tonsillar microbiome composition in tonsil cancer patients. (a) Schematic representation of the oropharynx illustrating crypt biopsies collected from tumor-affected and non-affected contralateral tonsils. (b-c) Comparison of α-diversity between tumor-affected and contralateral tonsils. Observed number of species, Chao1, Simpson and Shannon indices are shown. (d-f) Principle coordinate analysis using Bray–Curtis (d), UNIFRAC (e) and weighted UNIFRAC (f) distance metrics. Dotted circles represent superimposed normal probability over data points. (g) Taxonomic characterization of the microbiome in tonsil cancer patients on the phylum level. Statistical analysis does not reach significance (p < .05). (h) Relative abundance of the top eight highest abundant genera detected in tumor and contralateral tonsils. Statistical analysis was performed using Wilcoxon-Mann-Whitney test (b-c, g-h) or PERMANOVA test (d-f)
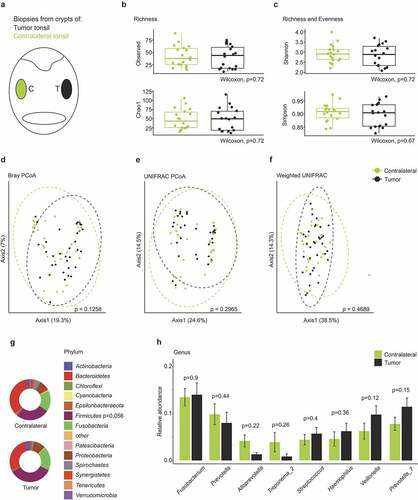
Distinct tonsillar crypt microbiome composition in tonsil cancer
Next, we compared the crypt microbiome of ipsi- and contralateral tonsils of tumor patient with the crypt microbiome of OSA patients. α-diversity measures revealed equal richness () and richness and evenness () in the microbial communities. In contrast, principle coordinate analysis revealed a significant shift of the microbiome composition in tumor patients compared to OSA patients for all distance metrics ( and Supplementary Figure 2a-c). In particular, we found a substantial dissimilarity in the microbial composition on the phylum level with significantly elevated abundance of Firmicutes and Actinobacteria and significantly reduced abundance of Spirochetes, Synergistetes and Fusobacteria in tumor patients ( and Supplementary Figure 2d). These results were further corroborated using projection of taxa abundance onto the phylogenetic tree. As shown in Supplementary Figure 3, consistent patterns emerged along tree branches with significant differences between tumor and OSA patients and substantially higher abundance of the phylum Firmicutes and its genera Veillonella, Streptococcus and Megasphaera in tumor patients. The microbial composition analysis on the genus level revealed differential abundance of several genera in tonsillar crypts of tumor vs. OSA patients ( and Supplementary Figure 4). Fusobacterium was the most abundant in OSA tonsils, whereas Veillonella and Prevotella_7 were highly abundant in tumor tonsils (). The crypt microbiome of contralateral tonsils of tumor patients consistently showed an intermediate relative abundance on the genus level ( and Supplementary Figure 4a). The observed shift in relative genera abundance was coherent across patients in both cohorts (Supplementary Figure 4b). The analysis of patient metadata revealed that tumor-specific changes were independent of sex (Supplementary Figure 5a-c), alcohol consumption (data not shown) and smoking habits (Supplementary Figure 5d-g). Principle coordinate analysis did not indicate compositional dissimilarity based on smoking status (Supplementary Figure 5d-g). A highly similar microbial composition could be observed on the phylum level in smokers and non-smokers (Supplementary Figure 5g). Together, these data unveil a distinct tonsillar crypt microbiome composition in patients suffering from tonsil cancer.
Figure 4. Comparison of the crypt microbiome in tonsil cancer and obstructive sleep apnea (OSA) patients. (a-b) α-diversity in crypt biopsies of OSA and tonsil cancer patients with (a) species richness assessed by the observed number of species and Chao1 index and (b) Shannon and Simpson indices indicating species richness and evenness. (c-e) Principle coordinate analysis using Bray–Curtis (c), UNIFRAC (d) and weighted UNIFRAC (e) distance metrics. Dotted circles represent superimposed normal probability over data points. (f) Bacterial community composition in tumor and OSA patient samples on the phylum level. Significant p-values from the statistical analysis are indicated. (g) Comparison of the top eight genera with the highest overall relative abundance. Statistical analysis was performed using Kruskall-Wallis (a-b, f), PERMANOVA test (c–e), or Wilcoxon-Mann-Whitney test (g) with *, p < .05; **, p < .01; ***, p < .001; ns, not significant
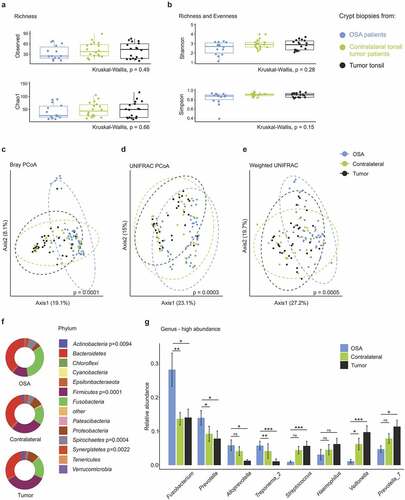
Identification of tonsil cancer patients based on predictive microbiome pattern
To further elaborate a potential relationship between the microbiota and tonsil cancer, we compared the crypt microbiome of tumor-affected and OSA tonsils at the species level using highly resolved taxonomic classification based on the human oral microbiome database.Citation24 Since bacterial operational taxonomic units typically do not follow a Gaussian distribution, differences on the species level were assessed using the DESeq2 method,Citation25 which permits the analysis of count data based on the negative binomial distribution. The heatmap shown in lists the top 45 differentially abundant bacterial species in a pattern that clearly separates tumor from OSA patients. To assess whether the abundance of certain bacterial species in tumor tonsils could be used as a predictor for the presence of tonsil cancer, we trained a machine-learning algorithm using the available data from OSA and tonsil cancer patients. The applied random forest classification method was shown previously to outperform other supervised classifiers on microbiome data.Citation26 Based on the complete 16S rRNA gene amplicon datasets from tumor and OSA patients, the trained model was able to detect a tumor-affected patient with high accuracy (0.89), sensitivity (0.89) and specificity (0.88) (). The top ten tumor-predictive species included Treponema denticola, Fusobacterium periodonticum, Filifactor alocis, Fusobacterium nucleatum subsp.vincentii, Megasphaera micronuciformis, Prevotella melaninogenica and Veillonella atypica (), which showed all significantly different abundances in tumor vs. OSA patients (). To validate these results, we performed quantitative PCR analysis using biopsy DNA and published primers that allow for the reliable detection of bacterial species. For the ten potentially predictive bacterial species, the published 16S rRNA gene PCR primers for F. alocisCitation27 and P. melaninogenicaCitation28 produced the most robust abundance measures. Using crypt DNA samples from the extended cohorts (21 OSA patients and 28 tonsil tumor patients, Supplementary Tables 1 and 2), we found a significantly higher relative abundance of F. alocis in crypt biopsies of OSA compared to tumor patients (). As expected from the random forest analysis, P. melaninogenica could be detected by PCR in all samples with significantly higher relative abundance in tumor-affected tonsils compared to OSA tonsils (). Collectively, these data reveal that the assessment of the composition of microbial communities in tonsillar crypts can serve as diagnostic means for the detection of tonsil cancer.
Figure 5. Characterization of tonsil cancer and obstructive sleep apnea (OSA) patient microbiomes at species level. (a) Heatmap showing top 45 differentially abundant species in crypts of tumor patients compared to OSA patients. (b) Mean decrease in accuracy of top ten tumor-predictive species calculated by means of random forest analysis. Bar color indicates phylum affiliation as used in Figure 4F of respective species. (c) Relative abundance of top ten predictive species plotted for tumor-bearing and contralateral tonsils of tumor and OSA patients. (d-e) Species specific quantitative PCR. Relative abundance of F. alocis (d) and P. melaninogenica (e) in samples from OSA and tumor patients. Statistical analysis was performed using Wilcoxon-Mann-Whitney test (c-e) with *, p < .05; **, p < .01; ***, p < .001; ns, not significant
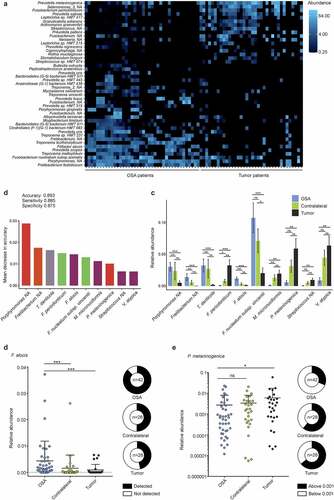
Discussion
According to the recent WHO classification, tonsillar squamous cell carcinomas are now subdivided into distinct morphological groups with HPV-positive cancer arising from the tonsillar crypts and exhibiting a non-keratinizing morphology.Citation29 The tonsil cancer patients recruited in this study suffered from squamous cell carcinoma and the majority of the patients showed infection with the high-risk strain HPV16, which is consistent with the global pattern.Citation30 Since the oral cavity and the oropharynx provide heterogeneous niches for bacterial colonization, we have focused on the microbiome in tonsillar crypts as the most critical region for HPV-induced carcinogenesis. Since the tonsillar microbiome in tonsil tumor patients was significantly different from the microbiome of adult sleep apnea patients on all phylogenetic levels, we conclude that HPV-induced carcinogenesis profoundly alters the conditions for bacterial growth in tonsillar crypts. It appears that the dysbiosis in tumor tonsils diffused to the contralateral, non-affected tonsils. Our data thus suggest that bacterial communities change and adapt to altered conditions in the tonsillar environment due to the tumor growth and that these changes exert a dominant effect on the oropharyngeal microbiome in remote locations.
Localized chronic inflammation is thought to be one of the major drivers of carcinogenesis.Citation31 The Gram-negative and invasive bacteria Fusobacterium (F.) nucleatum and Porphyromonas (P.) gingivalis are frequently discussed to be causally involved in the induction of squamous cell carcinoma in the oropharynx.Citation32,Citation33 F. nucleatum and P. gingivalis have been shown to promote tumor progression in an oropharyngeal squamous cell carcinoma mouse modelCitation34 and F. nucleatum subspecies polymorphum has been found to be significantly increased in oral squamous cell carcinoma biopsies.Citation35 Moreover, an elevated abundance in oral wash and surface swabs of oral squamous cell carcinoma has been described for the genus Fusobacterium.Citation36,Citation37 However, in our study with the comparison of HPV-associated tonsillar squamous cell carcinoma and sleep apnea patient, the abundance of Fusobacterium in crypts of tumor tonsils was reduced. Moreover, at the species level, we found that an increased abundance of F. periodonticum and a decreased abundance of F. nucleatum subspecies vincentii were part of the tumor-predictive microbiome signature according to the random forest analysis. It is conceivable that these partially contradictory results are due to the distinct bacterial colonization patterns in the tonsillar crypt microenvironment in a diverse array of oral and oropharyngeal cancers.
The particular structure of tonsillar crypts is determined by the reticulated lymphoepithelial layer that separates the oropharynx from the underlying lymphoid tissue.Citation38 It is most likely that the reticulated epithelium that is interspersed with lymphocytes and myeloid cells, favors virus-induced malignant transformation due to direct access of the virus to epithelial stem cells. Furthermore, it is possible that particular bacteria foster the access of HPV particles to the basal epithelial cell layer. Indeed, Streptococcus-derived furin like endopeptidases have been shown to promote infection of epithelial cells by HPV16 pseudovirus suggesting that carcinogenesis could be enhanced by particular bacterial products.Citation39 It will be important to assess in future studies whether the bacterial species that have been identified here can alter the crypt microenvironment and thereby change HR-HPV infectivity and carcinoma induction.
It is most likely that the composition of bacterial communities in the tonsillar crypt not only affects tumor growth, but that once the carcinogenesis is progressing, the altered microenvironmental conditions in and around the tumor affect the local microbiome. Indeed, in this study tonsillar crypts of tumor-affected and contralateral, non-affected tonsils of cancer patients harbored highly similar microbial communities. These results are in line with the study of Wang et al.Citation23 who found more similarities than differences between the overall microbiomes from frozen tumor and adjacent normal tissues in a heterogeneous patient cohort on head and neck squamous cell carcinoma. Moreover, it appears that bacterial communities change during oral cancer progression in generalCitation36 and in tonsil cancer as described here. Some overarching trends in oral and tonsil cancer-associated microbiome alterations emerge: elevated Rothia and lowered Fusobacterium abundance,Citation40 elevated Streptococcus and Veillonella,Citation41 reduced Porphyromonas.Citation36,Citation42 Clearly, tumor-associated and -predictive changes in the microbial composition need to be detailed and validated on the species level to facilitate further delineation of pathogenic principles and the elaboration of diagnostic procedures.
Current conclusions about the association of the microbiome with oralpharyngeal cancer have been mainly drawn from studies using saliva or oral rinse microbiomes.Citation41,Citation43,Citation44 Using tonsillar crypt biopsies, our study has identified a set of bacterial species that appears to be tonsil cancer-predictive. The elevated abundance of P. melaninogenica in tonsil cancer is consistent with the heightened presence of this bacterial species in saliva of oral cancer patients.Citation45 The latter study of Mager et al.Citation45 has used DNA hybridization to detect 40 distinct bacterial species in saliva specimen. A later study based on 16S rRNA gene amplicon sequencing has found a higher abundance of F. nucleatum subspecies polymorphum and Porphyromonas aeruginosa in archived frozen tissue of oral squamous cell carcinoma patients, whereas bacterial species such as Streptococcus mitis, Rothia mucilaginosa and Haemophilus parainfluenzae were more frequent in epithelial swabs of healthy controls.Citation35 These previous studies, together with the data presented here indicate that the assessment of bacterial dysbiosis with the quantification of differentially abundant bacterial “indicator” species could guide the diagnosis of oropharyngeal malignancies. Clearly, future studies will be required to further validate the set of bacterial species for diagnosis of tonsil cancer elaborated here and to define niche-specific microbiome alterations in other oropharyngeal cancer entities.
Conclusions
The incidence of HR-HPV-associated tonsil cancer has been steadily increasing in the recent decades. The comparison of the tonsillar crypt microbiome of patients suffering from tonsil cancer with an OSA patient control cohort shows that the microbial ecosystem in the crypt environment changes during cancer development. Characteristic changes in the microbial composition could be detected on all phylogenetic levels. Machine learning-based analysis of the 16S rRNA gene amplicon sequencing data and PCR-based validation indicated that particular bacterial species combinations in the tonsillar crypt microenvironment can aid the diagnosis of tonsil cancer.
Methods
Study population and sample collection
The study protocol has been reviewed and approved by the Ethikkommission Ostschweiz (EKOS), permission number 2017–00051. All study participants provided written informed consent in accordance with the Declaration of Helsinki and the International Conference on Harmonization Guidelines for Good Clinical Practice. All regulations were followed according to the Swiss authorities and according to the clinical protocols. The sample size calculation for the study was not performed because of the exploratory nature of the study design.
Patients suffering from obstructive sleep apnea (OSA) were prospectively enrolled in control cohort 1 with a total of 21 participants that underwent tonsillectomy between May 2017 and May 2020. Exclusion criteria were as follows: (a) acute or chronic tonsillitis, (b) any disease with immunosuppression, (c) immunosuppressive treatment, and (d) intake of antibiotics up to 4 weeks before surgery. After excision, tonsils were placed on a sterile surface and 4 mm punch biopsies (Stiefel® Biopsy punch) were taken using a sterile single-use stainless-steel instrument immediately in the operation theater using separate sterile pairs of scissors and forceps to avoid cross-contamination between samples. Two biopsies were punched out of the tonsil surface epithelium and two biopsies were taken from tonsillar crypts. Next, tonsils were cut in half with a sterile surgical blade and two lymphoid tissue samples were harvested by punch biopsy.
Patients diagnosed with a primary, untreated and histologically confirmed squamous cell carcinoma of the tonsil (n = 28) were prospectively included into the study cohort 2 between August 2017 and February 2020. Patients with previous radiotherapy or surgery due to oropharyngeal cancer were excluded. Two punch biopsies from tonsillar crypts were taken directly after removal of the tumor-affected tonsil and two crypt biopsies were acquired from the contralateral tonsil during surgery. In cases when tumor tonsils were not resected, crypt biopsies were acquired in situ by punch biopsy. Each biopsy was acquired using a fresh sterile biopsy punch and a separate sterile pair of scissors and forceps. Tissue biopsies were placed in sterile tubes on ice and stored at −80°C within 30 min until further analysis.
DNA extraction and 16S rRNA gene amplicon sequencing
Bacterial genomic DNA was extracted and purified using QIAamp DNA Stool Mini Kit (Qiagen) with minor modifications. Lysing Matrix D tubes (MPBio) were used for tissue disruption and an additional extraction step was introduced after incubation of bacteria with a lysis buffer (20 mg/ml lysozyme – Sigma 62970–5 G-F, 2 mM EDTA, 1.2% Triton, 20 mM Tris-HCL) for an optimal yield of bacterial DNA. PCR amplification of the V4 region of bacterial 16S rRNA gene was performed with barcoded primers following a dual-index paired-end sequencing approach.Citation46 PCR was performed using KAPA HiFi polymerase (Roche) under the following cycling conditions: initial denaturation at 98°C (2 min), 25–40 cycles of 98°C (30 sec), 55°C (30 sec), 72°C (20 sec) and final elongation at 72°C (7 min). PCR products including a negative control were electrophoresed on a 1% agarose gel to ensure high-fidelity amplification. NucleoMag® NGS (Macherey-Nagel) was used for PCR clean up and size selection. Individual libraries were normalized with SequalPrep™ Normalization Plate Kit (ThermoFisher) according to the manufacturer’s instruction and combined into a pooled library. The combined library was qualitatively and quantitatively assessed with a High Sensitivity D1000 ScreenTape device (Agilent) and on a Qubit fluorometer (ThermoFisher). 16S rRNA V4 amplicon sequencing was performed on the Illumina MiSeq v2 platform. Miseq data was converted to fastq format and demultiplexed using bcl2fastq software (Illumina).
Read processing and taxonomic analysis
Cutadapt was used for quality trimming and removal of the primer and adaptor sequences.Citation47 Further processing and analysis were run in R version 3.6.1. Trimmed reads were filtered and truncated based on quality scores and read length using the R/bioconductor package dada2 v1.14.0Citation48 with 230 bp as length for forward reads and 210 bp for reverse reads. After dereplication error rates were estimated based on a parametric error model as implemented in the dada2 package and sequence variants were generated using the ‘dada’ function. Chimeric reads were removed using the ‘removeBimeraDenovo’ function. Finally, taxonomy was assigned based on the RDP trainset 16/release 11.5Citation49 and the HOMD v.15.1Citation24 database by applying the ‚assignTaxonomy’ function from the dada2 package that utilizes the RDP Naive Bayesian Classifier described by Wang et al,Citation50 with kmer size = 8, and 100 bootstrap replicates. In order to further infer phylogenetic relationships R/bioconductor packages msa v.1.18.0Citation51 and phangorn v.2.5.5Citation52 were used to build multiple sequence alignments and construct a phylogenetic tree. Before downstream analysis samples were rarefied to an even sampling depth of 500 reads using the R/bioconductor package phyloseq v.1.32.0.Citation53 Samples with less than 500 reads were removed from further analysis resulting in a final dataset of 192 samples. The observed low read counts compared to typical feces samples were expected, as the DNA was isolated from tissue biopsies.
Statistical analysis
Rarefied samples were analyzed for alpha diversity based on shannon, simpson and inverse simpson metrics and beta diversity based on Bray-Curtis, UNIFRAC, and weighted UNIFRAC distances. All diversity measures were computed running the ‘diversity’ function from the R/Bioconductor package vegan v.2.5–7Citation54 and Kruskal-Wallis and Wilcoxon tests were used to test for significant differences in α- diversity measures. β-diversity was visualized by principal coordinate analysis and permutational ANOVA was run using the ‘adonis’ function from R/bioconductor package vegan v.2.5–7Citation54 to test for significant differences between patient and sample groups. Samples that were collected from the same patient and location were regarded as technical replicates and all statistical tests were run on mean values from technical replicates. Differences in relative abundances between groups on phylum, family, and genus level were tested using Kruskal–Wallis and Wilcoxon-Mann-Whitney tests to compute statistics. In order to further examine the hierarchical relationship of taxa with differences in their relative abundance, a tree-based visualization technique was used by running the ‘heat_tree’ function from the metacoder R packages v.0.3.3.Citation55 Finally, differentially abundant taxa between groups were identified utilizing the Bioconductor packages phyloseq v.1.32.0Citation53 and DESeq2 v.1.28.1Citation25 following standard protocols for differential abundance analysis. Statistical analyses of quantitative PCR data were performed with Prism 8.4.3 (GraphPad). Graphs depict mean ± s.d. and differences between two groups were evaluated using Wilcoxon-Mann-Whitney tests. Results were considered statistically significant with p < .05.
Random forest analysis
Random Forest analysis was performed on species abundance data from control and tumor crypt samples using the randomForest R package v.4.6–14.Citation56 Data pre-processing included removal of rare OTUs based on mean relative abundances followed by re-normalization and scaling of the remaining 405 OTUs. The random forest model was fitted by running the ‘randomForest’ function with ntree = 5001 and default values for mtry. Model significance was assessed by running the ‘rf.significance’ function from the rfUtilities R package v.2.1–5Citation57 with 1,000 permutations. Model performance was tested by running a leave-one-out cross-validation utilizing the ‘train’ function from the caret R package v.6.0–86Citation58 with identical mtry and ntree parameters as before. Finally, the mean decrease in accuracy (MDA) was computed as a measure for the importance of each taxa for the overall model and used to rank the taxa.
Quantitative PCR
Extracted DNA was used as a template for bacterial qPCR amplification and quantification using a real-time PCR machine (Quant Studio 5) and SYBR Green PCR technology (Applied Biosystems, Thermo Fisher Scientific). A pair of ubiquitous bacterial 16S rDNA-directed primers (forward 5’-ACTCCTACGGGAGGCAGCAGT-3’ and reverse 5’-ATTACCGCGGCTGCTGGC-3’) was used to assess the total amount of bacteria in the samples.Citation59 Cycling conditions have included a denaturation step at 95°C for 10 min followed by 30 cycles of 95°C (10 sec), 63°C (20 sec), and 72°C (20 sec). Using the CT-values of eubacteria abundances of F.alocis and P.melaninogenica were calculated. F.alocis was quantified using species-specific primers targeting the 16S rRNA gene that have been established and validated.Citation27 16S rRNA gene‐directed forward 5’‐CAG GTG GTT TAA CAA GTT AGT GG‐3’ and reverse 5’‐CTA AGT TGT CCT TAG CTG TCT CG‐3’ primers and a cycling protocol of denaturation at 95°C (2 min) followed by 40 cycles of 95°C (15 sec), 60°C (1 min) and 72°C (1 min) were applied. P.melaninogenica specific 16S rRNA gene directed forward 5’-GTGGGATAACCTGCCGAAAG-3’ and reverse 5’-CCCATCCATTACCGATAAATCTTTA-3’ primers have been described and tested before.Citation28 The cycling protocol used was 95°C (10 min) and 40 cycles of 95°C (15 sec), 60°C (1 min) and 72°C (1 min). PCR amplification of each sample was performed in duplicates and the 20 ul qPCR mixture consisted of 10 ul of 2X SYBR Green MasterMix (Applied Biosystems, Thermo Fisher Scientific) 1 ul of each primer, 7 ul PCR-grade water and 1ul of extracted DNA.
Short summary
Tonsil cancer patients harbor a characteristic tonsillar crypt microbiome that is significantly different from the microbiome of adult sleep apnea patients on all phylogenetic levels.
Consent for publication
Not applicable.
Data and materials availability
The datasets generated and analysed during the current study are available in the SRA repository, with accession number PRJNA699728. Further information and requests for resources and reagents should be directed to and will be fulfilled by the lead contact, Burkhard Ludewig ([email protected]).
Author contributions
B.L., S.J.S. and M.A.B. designed the study; B.L., A.DM. and M.L. discussed data and wrote the paper; A.DM., M.L., Y.S., C.E., J.C., and I.D. conducted experiments and discussed data; M.L., K.B. performed bioinformatics analyses and discussed data; K.D.MC. discussed data and provided reagents; M.B.G. discussed data; S.J.S., W.J., M.A.B., discussed data and provided patient material.
Supplemental Material
Download ()Acknowledgments
The authors want to thank Sonja Caviezel-Firner for excellent technical support.
Supplementary material
Supplemental data for this article can be accessed on the publisher’s website.
Disclosure statement
The author(s) declares no competing financial interests.
Additional information
Funding
References
- Johnson DE, Burtness B, Leemans CR, Lui VWY, Bauman JE, Grandis JR. Head and neck squamous cell carcinoma. Nature Reviews Disease Primers. 2020;6:92.
- Leemans CR, Snijders PJF, Brakenhoff RH. The molecular landscape of head and neck cancer. Nat Rev Cancer. 2018;18(5):269–12. doi:10.1038/nrc.2018.11.
- Weatherspoon DJ, Chattopadhyay A, Boroumand S, Garcia I. Oral cavity and oropharyngeal cancer incidence trends and disparities in the United States: 2000–2010. Cancer Epidemiol. 2015;39(4):497–504. doi:10.1016/j.canep.2015.04.007.
- Egawa N, Egawa K, Griffin H, Doorbar J. Human papillomaviruses; epithelial tropisms, and the development of Neoplasia. Viruses. 2015;7(7):3863–3890. doi:10.3390/v7072802.
- Faraji F, Zaidi M, Fakhry C, Gaykalova DA. Molecular mechanisms of human papillomavirus-related carcinogenesis in head and neck cancer. Microbes Infect. 2017;19(9–10):464–475. doi:10.1016/j.micinf.2017.06.001.
- Giuliano AR, Lu B, Nielson CM, Flores R, Papenfuss MR, Lee J-H, Abrahamsen M, Harris RB. Age-specific prevalence, incidence, and duration of human papillomavirus infections in a cohort of 290 us men. J Infect Dis. 2008;198(6):827–835. doi:10.1086/591095.
- Wakabayashi R, Nakahama Y, Nguyen V, Espinoza JL. The host-microbe interplay in human papillomavirus-induced carcinogenesis. Microorganisms. 2019;7(7):7. doi:10.3390/microorganisms7070199.
- Dewhirst FE, Chen T, Izard J, Paster BJ, Tanner ACR, Yu W-H, Lakshmanan A, Wade WG. The human oral microbiome. J Bacteriol. 2010;192(19):5002–5017. doi:10.1128/JB.00542-10.
- The Human Microbiome Project Consortium. Structure, function and diversity of the healthy human microbiome. Nature. 2012;486(7402):207–214. doi:10.1038/nature11234.
- Huse SM, Ye Y, Zhou Y, Fodor AA, Ahmed N. A core human microbiome as viewed through 16S rRNA sequence clusters. PLoS One. 2012 e34242-e34242;7(6):e34242. doi:10.1371/journal.pone.0034242.
- Martinez-Guryn K, Leone V, Chang EB. Regional diversity of the gastrointestinal microbiome. Cell Host Microbe. 2019;26(3):314–324. doi:10.1016/j.chom.2019.08.011.
- Aas JA, Paster BJ, Stokes LN, Olsen I, Dewhirst FE. Defining the normal bacterial flora of the oral cavity. J Clin Microbiol. 2005;43(11):5721–5732. doi:10.1128/JCM.43.11.5721-5732.2005.
- Swidsinski A, Göktas O, Bessler C, Loening-Baucke V, Hale LP, Andree H, Weizenegger M, Hölzl M, Scherer H, Lochs H. Spatial organisation of microbiota in quiescent adenoiditis and tonsillitis. J Clin Pathol. 2007;60(3):253–260. doi:10.1136/jcp.2006.037309.
- Johnston J, Hoggard M, Biswas K, Astudillo-García C, Radcliff FJ, Mahadevan M, Douglas RG. Paired analysis of the microbiota between surface tissue swabs and biopsies from pediatric patients undergoing adenotonsillectomy. Int J Pediatr Otorhinolaryngol. 2018;113:51–57. doi:10.1016/j.ijporl.2018.07.024.
- Johnston J, Hoggard M, Biswas K, Astudillo-García C, Waldvogel-Thurlow S, Radcliff FJ, Mahadevan M, Douglas RG. The bacterial community and local lymphocyte response are markedly different in patients with recurrent tonsillitis compared to obstructive sleep apnoea. Int J Pediatr Otorhinolaryngol. 2018;113:281–288. doi:10.1016/j.ijporl.2018.07.041.
- Johnston J, Hoggard M, Biswas K, Astudillo-García C, Radcliff FJ, Mahadevan M, Douglas RG. Pathogen reservoir hypothesis investigated by analyses of the adenotonsillar and middle ear microbiota. Int J Pediatr Otorhinolaryngol. 2019;118:103–109. doi:10.1016/j.ijporl.2018.12.030.
- Choi DH, Park J, Choi JK, Lee KE, Lee WH, Yang J, Lee JY, Park YJ, Oh C, Won H-R. et al: association between the microbiomes of tonsil and saliva samples isolated from pediatric patients subjected to tonsillectomy for the treatment of tonsillar hyperplasia. Exp Mol Med. 2020;52(9):1564–1573. doi:10.1038/s12276-020-00487-6.
- Galli J, Calò L, Posteraro B, Rossi G, Sterbini FP, Paludetti G, Sanguinetti M. Pediatric oropharyngeal microbiome: mapping in chronic tonsillitis and tonsillar hypertrophy. Int J Pediatr Otorhinolaryngol. 2020;139:110478. doi:10.1016/j.ijporl.2020.110478.
- Jensen A, Fagö-Olsen H, Sørensen CH, Kilian M, Xu P. Molecular mapping to species level of the tonsillar crypt microbiota associated with health and recurrent tonsillitis. PLoS One. 2013;8(2):e56418. doi:10.1371/journal.pone.0056418.
- Kim KS, Min HJ. Correlation between adenotonsillar microbiome and clinical characteristics of pediatrics with snoring. Clin Exp Otorhinolaryngol. 2020. doi:10.21053/ceo.2020.01634.
- Watanabe H, Goto S, Mori H, Higashi K, Hosomichi K, Aizawa N, Takahashi N, Tsuchida M, Suzuki Y, Yamada T, et al. Comprehensive microbiome analysis of tonsillar crypts in IgA nephropathy. Nephrology Dialysis Transplantation. 2017;32(12):2072–2079.
- Bahig H, Fuller CD, Mitra A, Yoshida-Court K, Solley T, Ping Ng S, Abu-Gheida I, Elgohari B, Delgado A, Rosenthal DI et al. Longitudinal characterization of the tumoral microbiome during radiotherapy in HPV-associated oropharynx cancer. Clinical and Translational Radiation Oncology. 2021;26:98–103. doi:10.1016/j.ctro.2020.11.007.
- Wang H, Funchain P, Bebek G, Altemus J, Zhang H, Niazi F, Peterson C, Lee WT, Burkey BB, Eng C. Microbiomic differences in tumor and paired-normal tissue in head and neck squamous cell carcinomas. Genome Med. 2017;9(1):14. doi:10.1186/s13073-017-0405-5.
- Escapa F. I, Huang Y, Chen T, Lin M, Kokaras A, Dewhirst FE, Lemon KP: construction of habitat-specific training sets to achieve species-level assignment in 16S rRNA gene amplicon datasets. Microbiome. 2020;8(1):65. doi:10.1186/s40168-020-00841-w.
- Love MI, Huber W, Anders S. Moderated estimation of fold change and dispersion for RNA-seq data with DESeq2. Genome Biol. 2014;15(12):550. doi:10.1186/s13059-014-0550-8.
- Knights D, Costello EK, Knight R. Supervised classification of human microbiota. FEMS Microbiol Rev. 2011;35(2):343–359. doi:10.1111/j.1574-6976.2010.00251.x.
- Siqueira JF Jr., Rôças IN. Detection of Filifactor alocis in endodontic infections associated with different forms of periradicular diseases. Oral Microbiol Immunol. 2003;18(4):263–265. doi:10.1034/j.1399-302X.2003.00073.x.
- Martin FE, Nadkarni MA, Jacques NA, Hunter N. Quantitative microbiological study of human carious dentine by culture and real-time PCR: association of anaerobes with histopathological changes in chronic pulpitis. J Clin Microbiol. 2002;40(5):1698–1704. doi:10.1128/JCM.40.5.1698-1704.2002.
- Westra WH, Lewis JS Jr. Update from the 4th Edition of the World Health Organization Classification of Head and Neck Tumours: oropharynx. Head Neck Pathol. 2017;11(1):41–47. doi:10.1007/s12105-017-0793-2.
- Näsman A, Du J, Dalianis T. A global epidemic increase of an HPV-induced tonsil and tongue base cancer - potential benefit from a pan-gender use of HPV vaccine. J Intern Med. 2020;287(2):134–152. doi:10.1111/joim.13010.
- Hanahan D, Weinberg RA. Hallmarks of cancer: the next generation. Cell. 2011;144(5):646–674. doi:10.1016/j.cell.2011.02.013.
- Sami A, Elimairi I, Stanton C, Ross RP, Ryan CA. The role of the microbiome in oral squamous cell carcinoma with insight into the microbiome-treatment axis. Int J Mol Sci. 2020;21:21. doi:10.3390/ijms21218061.
- Tuominen H, Rautava J. Oral microbiota and cancer development.Pathobiology. 2021;88:116–126. doi:10.1159/000510979.
- Binder Gallimidi A, Fischman S, Revach B, Bulvik R, Maliutina A, Rubinstein AM, Nussbaum G, Elkin M. Periodontal pathogens porphyromonas gingivalis and Fusobacterium nucleatum promote tumor progression in an oral-specific chemical carcinogenesis model. Oncotarget. 2015;6(26):22613–22623. doi:10.18632/oncotarget.4209.
- Al-hebshi NN, Nasher AT, Maryoud MY, Homeida HE, Chen T, Idris AM, Johnson NW. Inflammatory bacteriome featuring fusobacterium nucleatum and pseudomonas aeruginosa identified in association with oral squamous cell carcinoma. Sci Rep. 2017;7(1):1834. doi:10.1038/s41598-017-02079-3.
- Yang C-Y, Yeh Y-M, Yu H-Y, Chin C-Y, Hsu C-W, Liu H, Huang P-J, Hu S-N, Liao C-T, Chang K-P. et al: oral microbiota community dynamics associated with oral squamous cell carcinoma staging. Front Microbiol. 2018;9:862. doi:10.3389/fmicb.2018.00862.
- Zhao H, Chu M, Huang Z, Yang X, Ran S, Hu B, Zhang C, Liang J. Variations in oral microbiota associated with oral cancer. Sci Rep. 2017;7(1):11773. doi:10.1038/s41598-017-11779-9.
- Nave H, Gebert A, Pabst R. Morphology and immunology of the human palatine tonsil. Anat Embryol (Berl). 2001;204(5):367–373. doi:10.1007/s004290100210.
- Pavlova SI, Wilkening RV, Federle MJ, Lu Y, Schwartz J, Tao L. Streptococcus endopeptidases promote HPV infection in vitro. Microbiologyopen. 2019;8(1):e00628. doi:10.1002/mbo3.628.
- Mukherjee PK, Wang H, Retuerto M, Zhang H, Burkey B, Ghannoum MA, Eng C. Bacteriome and mycobiome associations in oral tongue cancer. Oncotarget. 2017;8(57):97273–97289. doi:10.18632/oncotarget.21921.
- Guerrero-Preston R, Godoy-Vitorino F, Jedlicka A, Rodríguez-Hilario A, González H, Bondy J, Lawson F, Folawiyo O, Michailidi C, Dziedzic A, et al. 16S rRNA amplicon sequencing identifies microbiota associated with oral cancer, human papilloma virus infection and surgical treatment. Oncotarget. 2016;7(32):51320–51334. doi:10.18632/oncotarget.9710.
- Banerjee S, Tian T, Wei Z, Peck KN, Shih N, Chalian AA, O’Malley BW, Weinstein GS, Feldman MD, Alwine J, et al. Microbial signatures associated with oropharyngeal and oral squamous cell carcinomas. Sci Rep. 2017;7(1):4036. doi:10.1038/s41598-017-03466-6.
- Börnigen D, Ren B, Pickard R, Li J, Ozer E, Hartmann EM, Xiao W, Tickle T, Rider J, Gevers D, et al. Alterations in oral bacterial communities are associated with risk factors for oral and oropharyngeal cancer. Sci Rep. 2017;7(1):17686. doi:10.1038/s41598-017-17795-z.
- Lim Y, Fukuma N, Totsika M, Kenny L, Morrison M, Punyadeera C. The performance of an oral microbiome biomarker panel in predicting oral cavity and oropharyngeal cancers. Front Cell Infect Microbiol. 2018;8:267. doi:10.3389/fcimb.2018.00267.
- Mager DL, Haffajee AD, Devlin PM, Norris CM, Posner MR, Goodson JM. The salivary microbiota as a diagnostic indicator of oral cancer: a descriptive, non-randomized study of cancer-free and oral squamous cell carcinoma subjects. J Transl Med. 2005;3:27. doi:10.1186/1479-5876-3-27.
- Kozich JJ, Westcott SL, Baxter NT, Highlander SK, Schloss PD. Development of a dual-index sequencing strategy and curation pipeline for analyzing amplicon sequence data on the MiSeq Illumina sequencing platform. Appl Environ Microbiol. 2013;79(17):5112–5120. doi:10.1128/AEM.01043-13.
- Martin M. Cutadapt removes adapter sequences from high-throughput sequencing reads.EMBnet.journal. 2011;17(1):3.
- Callahan BJ, McMurdie PJ, Rosen MJ, Han AW, Johnson AJA, Holmes SP. DADA2: high-resolution sample inference from Illumina amplicon data. Nat Methods. 2016;13(7):581–583. doi:10.1038/nmeth.3869.
- Cole JR, Wang Q, Fish JA, Chai B, McGarrell DM, Sun Y, Brown CT, Porras-Alfaro A, Kuske CR, Tiedje JM. Ribosomal database project: data and tools for high throughput rRNA analysis. Nucleic Acids Res. 2014;42(D1):D633–642. doi:10.1093/nar/gkt1244.
- Wang Q, Garrity GM, Tiedje JM, Cole JR. Naive bayesian classifier for rapid assignment of rrna sequences into the new bacterial taxonomy. Appl Environ Microbiol. 2007;73(16):5261–5267. doi:10.1128/AEM.00062-07.
- Bodenhofer U, Bonatesta E, Horejš-Kainrath C, Hochreiter S. msa: an R package for multiple sequence alignment. Bioinformatics. 2015;31(24):3997–3999. doi:10.1093/bioinformatics/btv494.
- Patino LH, Muñoz M, Cruz-Saavedra L, Muskus C, Ramírez JD: Genomic Diversification, Structural Plasticity, and. Hybridization in leishmania (Viannia) braziliensis. Front Cell Infect Microbiol 2020;10:635.
- McMurdie PJ, Holmes S. phyloseq: an R package for reproducible interactive analysis and graphics of microbiome census data. PLoS One. 2014;42:e61217. doi:10.1371/journal.pone.0061217.
- Oksanen J, Blanchet FG, Friendly M, Kindt R, Legendre P, McGlinn D, Minchin PR, O’Hara RB, Simpson GL, Solymos P, et al. vegan: community ecology package. R Package Version. 2018;2.5–7.
- Foster ZSL, Sharpton TJ, Grünwald NJ, Poisot T. Metacoder: an R package for visualization and manipulation of community taxonomic diversity data. PLoS Comput Biol. 2017;13(2):e1005404. doi:10.1371/journal.pcbi.1005404.
- Liaw A, Wiener M. Classification and Regression by randomForest. R News. 2002;2:18–22.
- Evans JS, Murphy MA: rfUtilities. R package version 2.1-5, https://cran.r-project.org/package=rfUtilities. 2018.
- Kuhn M: caret: classification and regression training. R package version 6.0-86. https://CRAN.R-project.org/package=caret. 2020.
- Amann RI, Binder BJ, Olson RJ, Chisholm SW, Devereux R, Stahl DA. Combination of 16S rRNA-targeted oligonucleotide probes with flow cytometry for analyzing mixed microbial populations. Appl Environ Microbiol. 1990;56(6):1919–1925. doi:10.1128/aem.56.6.1919-1925.1990.