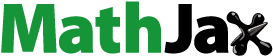
ABSTRACT
Chimeric antigen receptor (CAR) T-cell therapy combines antigen-specific properties of monoclonal antibodies with the lytic capacity of T cells. An effective and safe CAR-T cell therapy strategy relies on identifying an antigen that has high expression and is tumor specific. This strategy has been successfully used to treat patients with CD19+ B-cell acute lymphoblastic leukemia (B-ALL). Finding a suitable target antigen for other cancers such as acute myeloid leukemia (AML) has proven challenging, as the majority of currently targeted AML antigens are also expressed on hematopoietic progenitor cells (HPCs) or mature myeloid cells. Herein, we developed a computational method to perform a data transformation to enable the comparison of publicly available gene expression data across different datasets or assay platforms. The resulting transformed expression values (TEVs) were used in our antigen prediction algorithm to assess suitable tumor-associated antigens (TAAs) that could be targeted with CAR-T cells. We validated this method by identifying B-ALL antigens with known clinical effectiveness, such as CD19 and CD22. Our algorithm predicted TAAs being currently explored preclinically and in clinical CAR-T AML therapy trials, as well as novel TAAs in pediatric megakaryoblastic AML. Thus, this analytical approach presents a promising new strategy to mine diverse datasets for identifying TAAs suitable for immunotherapy.
Introduction
Pediatric acute myeloid leukemia (AML) is a challenging disease associated with high relapse and mortality rates.Citation1–3The reported overall survival (OS) of children with AML is 65%–70%, which lags behind that for pediatric acute lymphoblastic leukemia (ALL).Citation4 In recent years, improvement in outcomes of patients with AML has been largely attributed to a reduction in treatment-related mortality (TRM). Intensive chemotherapy regimens have resulted in increased TRM, leading to decreased OS. Also, patients with certain AML subtypes, such as megakaryoblastic AML (AMKL), carry an inherently poor prognosis.Citation5 Thus, there is an urgent need to develop novel targeted therapies for pediatric AMKL.
Genetically modified T cells that express chimeric antigen receptors (CARs) combine antigen-specific properties of monoclonal antibodies with the lytic capacity of T cells. CAR-T cells recognize and kill tumor cells in a major histocompatibility complex – independent manner by associating with a specific cell surface antigen, which induces T-cell activation and antigen-positive cellular lysis.Citation6–8 The ideal antigen target for immunotherapy is an antigen that is expressed on the cell surface of tumor cells, but has low to no expression on normal tissues. CD19, for example, is an antigen expressed on B-cell derived acute lymphoblastic leukemia (B-ALL), with its expression on normal tissues limited to B-cells, limiting its potential toxicities.Citation9 Because of this, immunotherapeutic strategies using CAR-T cells to target CD19+ malignancies have demonstrated clinical effectiveness with an acceptable toxicity profile.Citation10–12 Identifying an optimal antigen to target AML has proven difficult, given the marked overlap between antigens expressed on leukemic blasts and normal tissues.Citation13–16 Examples of antigens currently being targeted include CD33, CD123 and CLL-1. CD33 or siglec 3 is a transmembrane receptor that is expressed on AML blasts but is also expressed on normal HSPCs, lymphoid cells and in Leydig cells.Citation17 CD123 or interleukin 3 receptor alpha (IL3Ra), is an antigen highly expressed on AML blasts and leukemia stem cells (LSC) that also has low level expression on endothelial cells, and normal hematopoietic progenitor cells.Citation18 CLL1 or CLEC12A, belongs to the C-type lectin domain superfamily but has limited expression on mature myeloid cells.Citation19 However, clinical trials targeting CD33, CLL-1 or CD123 in adult and pediatric AML patients are ongoing. As myelotoxicity is a concern, CAR T cell therapy is used a bridge to bone marrow transplantation in certain instances.Citation20,Citation21
Publicly available expression data have been used to identify tumor-specific antigens in previous studies. For example, microarray data have been used to mine potential immunotherapy targets in pediatric cancers such as B-lineage ALL (B-ALL) and solid tumors.Citation22 Similarly, RNA-sequencing (RNA-seq) and proteomics data have been used to predict antigen targets in adult AML patients.Citation23 An integrative approach that can use data generated from microarray and RNA-seq can maximize the power of discovering immunotherapy targets by increasing the sample size, which is critical for pediatric cancer, a rare disease with limited number of patient samples. Such an approach has not yet been explored due to heterogeneities in sample acquisition and assay platforms. For example, data on the expression of non-disease tissues by transcriptome sequencing (RNA-seq) can be compiled from publicly available resources such as the Genotype-Tissue Expression (GTEx) project.Citation24 Data on disease tissues such as cancer in children, however, have been generated by RNA-seq through other initiatives such as the Pediatric Cancer Genome Project (PCGP) or the Therapeutically Applicable Research to Generate Effective Treatments (TARGET) project.Citation25,Citation26 Further, more than 3,000 microarray datasets have been made publicly available in the Gene Expression Omnibus in 2019 alone, which shows that microarray data remain a valuable resource for gene expression profiling despite the emergence of RNA-seq as the main platform for gene expression quantification in recent years. Significant challenges remain in unifying and normalizing these two data resources, given that microarray data measure relative expression whereas RNA-seq data measure absolute read counts.
To enable the comparative analysis of diverse expression datasets in the public domain, we developed a method to transform heterogeneous expression data to maximize the power of predicting tumor-associated antigens (TAAs). We show the application of this approach in identifying candidate TAAs in pediatric AMKL. Our approach to TAA prediction is generally applicable across cancer types and disease states.
Results
Tumor-associated antigen prediction algorithm
Transformed expression values (TEVs) were analyzed using RNA-seq data from primary tumors to identify suitable TAAs: genes absent in physiologically critical tissues and highly expressed in 102 patients with AMKL enrolled in the Pediatric Cancer Genome Project (PCGP).Citation25 Six additional patients with AMKL enrolled in the NCI TARGET study were also included to confirm that tumor-specific expression of predicted TAAs was not cohort specific. RNA-seq and microarray data from normal human tissue and progenitor cells were used to assess undesirable genes for CAR-T therapy. Genes were implicated as potential antigens according to exposure to the extracellular matrix, which was identified using the definition in the Human Protein Reference Database and Ensembl (, Supplemental table 1).Citation27
Figure 1. Workflow for tumor-associated antigen (TAA) prediction
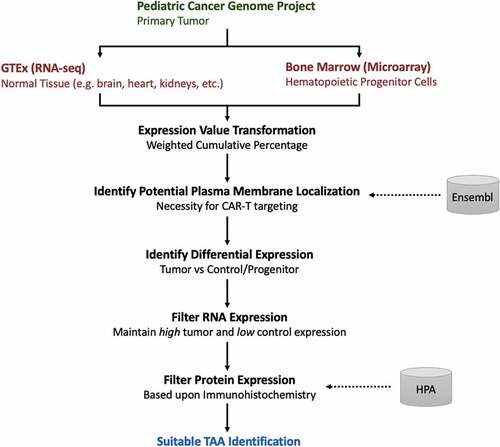
To identify genes with significantly higher expression in the tumor than in normal tissue, Wilcoxon–Mann–Whitney tests were performed using TEVs. Potential TAAs were initially ranked based on the degree of statistical significance associated with tumor-specific expression relative to control tissues. The presence or absence of expression for candidate TAAs was predicted in each primary tumor, provided by the unique detection calling predictions inferred from the control microarray data. However, this information was used only to estimate the percentage of tumors expressing the antigen rather than as a restrictive filter for declaring TAA candidates.
Protein expression was then assessed via immunohistochemistry data by using the following scale: not detected, low expression, medium expression, or high expression, as defined by the Human Protein Atlas.Citation28 TAAs were excluded as potentially suitable if protein expression was considered to be “low” or greater in more than seven normal tissues. Germline tissues were excluded from this filter, as cancer-testis antigens have been considered effective targets of immunotherapy since their function is generally not critical for survival.Citation29–32
Data transformation to improve heterogeneous data comparison
Representing gene expression values as FPKM is standard practice for RNA-seq analysis,Citation33 and their distribution can vary significantly across datasets such as TARGET and GTEx. However, microarray expression data are distinct from RNA-seq data in that they measure the hybridization value intensities accompanied by detection calls, which represent the probability of absence or presence of gene expression in each sample.Citation34,Citation35 By normalizing RNA-seq and microarray data such that they can be compared, additional information can be obtained, such as defining gene detection calls derived from microarray data, which can then be applied to RNA-seq TEVs.
We developed a method to transform gene expression values in each sample to a range of 0 to 1 values in both RNA-seq and microarray data to compare relative expression (). Transformed values in each sample were normalized by quantile normalization, using microarray TEVs as the target distribution, to make the distribution of TEVs equivalent between tumor and control samples (, Supplemental ). In doing so, TEVs and the associated microarray-derived gene detection calls could be compared across platforms.
Table 2. TAA candidate gene expression status prediction in RNA-seq data
Figure 2. Comparison of gene expression value distributions across data cohorts
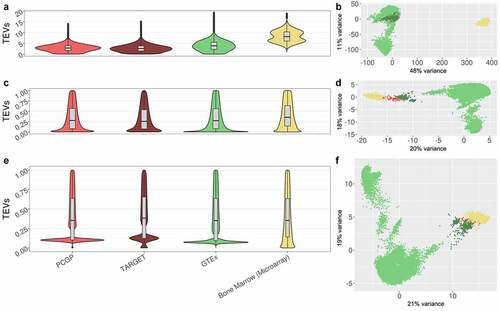
Model-based assessment of gene expression
To confirm our algorithm’s ability to infer gene detection calls strictly based upon TEVs, we built a logistic regression model to predict whether a gene is present or absent based on microarray TEVs. To assess the performance of this logistic regression model, we used a 10-fold cross-validation approach to test 648 bone marrow (BM) microarray samples.Citation37 Groups 1–9 included 65 samples per group (n = 585), and Group 10 included the remaining 63 samples. The logistic regression model predicted the presence or absence of the gene in the 10-fold cross validation with 90.8 ± 0.36% accuracy relative to microarray gene status prediction based on transformed probe hybridization values ().
Figure 3. Accuracy of gene expression status prediction based on transformed microarray hybridization values
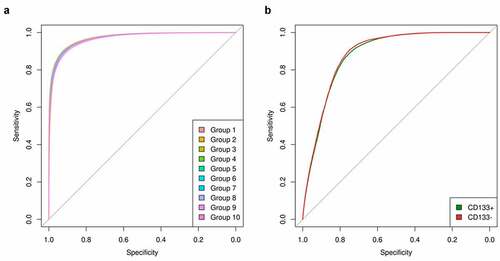
To further assess the validity of the logistic regression model, a distinct set of six samples derived from the same microarray platform was analyzed to assess the predictive ability of the logistic regression model across data cohorts. Samples were cell sorted into CD133+ and CD133 – cell populations.Citation38 The present or absent call for each gene from microarray was used as ground truth. The logistic regression model was applied to TEVs, and its prediction was 79.7 ± 1.76% accurate when using TEVs in CD133+ cells (). In CD133 – cells, gene status was predicted with 79.4 ± 0.79% accuracy (). The model correctly identified CD133+ and CD133 – subpopulations solely based on TEVs.
The logistic regression model built on microarray TEVs was then applied to RNA-seq TEVs derived from pediatric patients with B-ALL or AMKL. Common housekeeping genes, as well as those externally defined, were analyzed to assess the model’s ability to predict expected gene presence. The logistic regression model detected gene presence in housekeeping genes with 99.8% accuracy in 304 pediatric patients with B-ALL and 108 pediatric patients with AMKL. To assess the model’s ability to predict the expected absence of gene expression, we also checked the predicted status of tissue-specific antigens. Less than 1% of tissue-specific antigens – which were not expected to be expressed in pediatric B-ALL or AMKL – had predicted presence of gene expression based on TEVs ().
Table 1. Modeling of gene expression using TEVs
When the logistic regression model is applied to primary tumor TEVs, presence or absence of candidate TAAs can be assessed on a patient-by-patient basis. Assessing the presence of a TAA in a cohort of patients may provide insights into the range of patients who may be suitable for receiving CAR-T therapy targeting a specific TAA (). Conversely, gene status information can exclude potential target antigens or identify a set of patients for whom a particular antigen is not recommended. For example, a TAA that has demonstrated widespread clinical effectiveness as a CAR-T target might not be present in one patient or a small subset of patients, perhaps due to antigen escape in a patient who has relapsed.Citation45 Our algorithm can detect the presence or absence of a single gene in a single patient, which allows us to detect this type of case before administering treatment.
Performance assessment of suitable antigen targets in pediatric B-ALL
Examples of suitable TAAs for B-cell malignancies include CD19 and CD22.Citation10,Citation12,Citation46,Citation47 Therefore, our TAA prediction algorithm was first applied to data derived from 196 pediatric B-ALL patients for performance assessment. TAAs that have already demonstrated clinical effectiveness, CD19 and CD22, ranked seventh and sixth, respectively, among the 28 total TAAs predicted by our algorithm.Citation11,Citation12,Citation47 Several other known B-cell surface markers – CD11a, CD38, CD45, CD69, CD72, CD79a, CD79b, CD132, and CD179b – were also identified as potential antigen targets due to their overexpression in B-ALL.Citation48–55 Identification of TAAs demonstrating clinical effectiveness in B-ALL validated of our algorithm and provided the proof of concept necessary to apply the algorithm to other diseases ( and ).
Figure 4. Gene expression profiles of predicted TAAs in B-ALL and AMKL
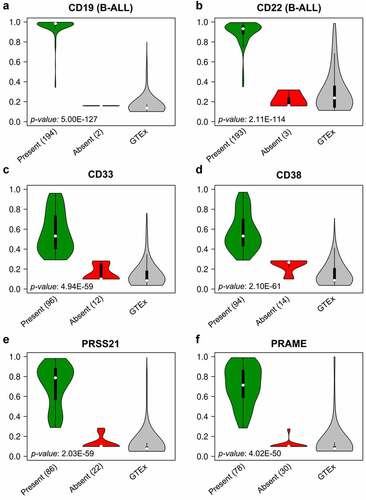
Identification of tumor-associated antigens in pediatric AMKL
Clinically effective antigen targets with a minimal adverse-effects profile have not yet been identified in pediatric patients with AMKL. We focused on identifying TAAs in 108 patients with AMKL in the PCGP and TARGET databases, given that patients with AMKL tend to have a worse prognosis.Citation13,Citation14,Citation56,Citation57 Gene expression in normal tissue was tested using 11,688 RNA-seq samples across 53 normal human tissues in the GTEx database.Citation24 Further, gene expression was tested in progenitor cells using 648 normal human BM samples generated from the Microarray Innovations in Leukemia study, as antigens presented on progenitor cells are not desirable targets for CAR-T therapy.Citation37
Our algorithm identified 41 potential TAAs in patients with AMKL (, Supplemental table 3), and their profiles were similar to those of clinically effective antigen targets for B-ALL (). After a manual review of the 41 identified targets, 14 genes were removed as they were expressed in vital tissues or not expressed on the cell surface. Of the remaining 27 AMKL TAAs, 15 were members of cluster of the differentiation (CD) family.Citation58 We observed a similar distribution of TEVs of established antigens in CAR-T therapy, CD19 and CD22, as well as antigens that have demonstrated promise in AML, CD33 and CD38. Further, we identified a similar, advantageous expression profile in two cancer-testis antigens, PRSS21 and PRAME ().Citation12,Citation17,Citation59–61
Discussion
As the number and diversity of publicly available gene expression data continue to increase, it is critical to develop analytical approaches that can effectively normalize datasets. Having the capability to compare heterogeneous data can increase the potential and improve the quality of studies such as biomarker discovery. To enable the comparison of gene expression data generated from different datasets/batches and platforms, we reasoned that absolute values may be different among batches or platforms but relative values are conserved between different batches or platforms. We developed an expression value transformation method to reduce data heterogeneity and use it to predict suitable TAAs in pediatric AMKL.
We show that apart from comparisons of TEVs, information on gene presence or absence derived from microarray data is also an effective metric to assess the degree of gene expression in RNA-seq data. We generated a logistic regression model to predict the status of gene expression strictly on the basis of TEVs. This model predicted gene expression status with consistent accuracy by using microarray TEVs in a 10-fold cross-validation as well as assessment in an independent microarray dataset (). We then applied this model to determine gene status using RNA-seq TEVs of TAAs for each patient. Our predicted AMKL TAAs had high gene expression in AMKL blasts and low gene expression in control samples, which is similar to what is seen in clinically effective antigen targets in B-ALL ( and ). The AMKL TAAs identified had strong expression in at least 72% of patients with AMKL (). An objective assessment of sample-specific gene expression, as described herein, by using microarray TEVs and the corresponding prediction of gene expression status could be useful to confirm experimental conditions, define an empirical threshold to filter out low gene expression before gene expression analysis, or even select patient cohorts for targeted therapy.
This study demonstrates an application of the transformation methods that can be used to compare gene expression values across data cohorts and even experiment types. We assessed TAAs that could serve as suitable therapeutic candidates for CAR-T cell therapy in B-ALL to confirm that our algorithm can effectively identify clinically effective antigen targets such as CD19 and CD22. We then applied this algorithm to data from pediatric patients with AMKL to identify potentially suitable antigen targets.
Most early phase clinical trials for AML have focused on adult patients and targeted genes in the CD family. Our algorithm successfully identified three genes – CD7, CD33 and CD38 – that are currently being tested in AML clinical trials for CAR-T therapy.Citation62–65 CD32 and CD123, which are being explored preclinically in AML, were excluded due to the stringency of parameters described herein.Citation66,Citation67 CD32 was excluded as a candidate TAA due to detectable protein expression in 14 control tissues. CD123 exceeded the maximum median RNA expression value cutoff (0.35) in GTEx control tissues and exhibited detectable protein expression in 45 control tissues.
We identified two novel, potentially suitable cancer-testis antigens, PRSS21 and PRAME, in pediatric AMKL which have a similar expression profile to successfully targeted antigens using CAR-T cell therapy. These genes were overexpressed in primary tumor samples from patients with AMKL, and their RNA and protein expression was almost exclusively limited to testes in normal tissues.Citation28 Therapies targeting cancer/testis antigens have demonstrated anti-tumor efficacy.Citation29 Previous research supports that PRSS21 and PRAME are targetable antigens on the plasma membrane.Citation68,Citation69 Both PRSS21 and PRAME have been associated with tumor progression in testicular tumors and melanomas, respectively, which may explain their AMKL-specific presence.Citation70 Further, PRAME is known to be recognized by cytolytic T lymphocytes, which is a critical checkpoint in defining antigen potential in CAR-T therapy.Citation71 Another candidate identified by our algorithm that has proven therapeutic success, potassium-activated channel KCNN4, is currently a Food and Drug Administration–approved drug target in sickle cell anemia.Citation72
In conclusion, we successfully generated a data transformation method that improves the ability to probe datasets from multiple gene expression platforms. We identified relatively tumor-specific overexpression of 28 genes in pediatric AMKL (). Our outlined strategy can also be extrapolated to identify suitable surface antigens as immunotherapy targets in other contexts.
Materials and methods
Data sources
RNA-seq data from 102 patients with AMKL in the PCGP and 6 patients with AMKL in the TARGET data cohort were analyzed to represent the primary tumor population.Citation56,Citation57 To consider the potential off-target effects of TAAs, 11,688 RNA-seq samples were considered across 53 normal human tissues from the GTEx database.Citation24 Also, 648 Affymetrix Human Genome U133 Plus 2.0 Array samples generated from the Microarray Innovations in Leukemia (MILE) study in human BM tissue were also used as controls and in building the logistic regression model to predict gene presence in RNA-seq data.Citation37 Six CD133 cell-sorted Affymetrix Human Genome U133 Plus 2.0 Array samples from GSE7181 were used to evaluate the performance of the logistic regression model when analyzing microarray TEVs and gene expression status.Citation38
Potential membrane association was assigned to genes via Gene Ontology terms specified in Ensembl.Citation27 Relative protein expression data were downloaded from the Human Protein Atlas.
Expression value transformations
Gene expression value transformations were performed on the largest common gene subset among data cohorts being compared. For microarray expression data wherein multiple probes were associated with the same gene, the largest expression value was considered. The transformation methods described below can be applied to gene expression values across platforms to assess relative gene expression. We describe the efficacy of transformations in normalizing heterogeneous gene expression comparisons between data cohorts, as well as between RNA-seq and microarray gene expression data.
Weighted Cumulative Percentage (WCP)
The WCP value transformation method is a fine discriminator of relative gene expression intensity within a sample. The method involves transformation of non-negative log2 raw expression values of descending intensity into a continuous value with the range 0–1, with 0 being the least expression and 1 being the highest expression assigned. The WCP transformation method can be given as
where gi represents the cumulative non-negative log2 raw expression value and g’i represents the transformed value calculated at each iteration. Genes with the same non-negative log2 raw expression value are assigned the same transformed value without increasing gi. If not already, the minimum quantile normalized WCP values are set to 0.
Parameter Tuning
Given that CD19 and CD22 have demonstrated clinical effectiveness in B-ALL, we benchmarked transformed values and TAA prediction metrics from these examples to help predict suitable TAAs in AMKL. Thresholds for RNA expression were set to consider only genes with a minimum median TEV of 0.5 in primary cancer samples. In addition, a threshold was set for a maximum median value of 0.3 in normal tissue from the GTEx database. If the gene under consideration is expressed on any tissues that are considered vital for survival, such as the brain or heart, the gene is flagged so that this information is easily accessible upon manual review. However, a strict maximum expression value threshold was not set for the BM dataset, since CD19 expression was high in the BM, likely due to the known role of CD19 in B-cell development, but this did not diminish the suitability of this antigen.Citation12,Citation73 However, consideration of BM gene expression data was critical in filtering out TAAs such as CCR7 that are not expressed in GTEx normal tissues but are highly expressed in the BM, which contain critical hematopoietic progenitor cells.
Expert Review
After detecting gene targets by using our algorithm, potential TAAs were further analyzed by expert review to confirm their suitability as targets for CAR-T therapy. The default ranking for each gene is defined by an aggregate of negative log10 p-values associated with the degree of significance of tumor expression relative to control tissue, which were multiplied by the difference in median transformed expression value on primary tumors versus controls. However, this order is not a definitive ranking for TAA suitability.
Candidate TAAs were required to be present on the plasma membrane of the intended target cell for recognition by CAR-T cells. Confirmation of extracellular exposure involved extensive literature review and data mining. TAAs were excluded in this analysis if their localization on the plasma membrane was questionable.
Further, literature review helped exclude genes that would likely not serve well as TAAs due to their association with the critical tissue that should not be targeted by CAR-T therapy. For example, RHAG was excluded as a suitable TAA because even though its RNA and protein expression are confined, expression on erythrocytes would likely yield off-target effects that are not clinically tolerable.Citation28 Another TAA ANK1 initially identified by our algorithm was excluded because direct assay suggested that it was localized on the cytoplasmic side of the membrane, which would likely not be suitable for recognition via CAR-T cells.Citation74
Data analyses
Data were analyzed and visualized by using self-developed bash and R scripts. BiocParallel, MASS, pheatmap, and plyr R packages were used within the script for parallel processing, logistic regression modeling, visualization, and object manipulation, respectively.Citation75–78
Code Availability
Author contributions
P.V., S.G., and Y.F. conceived the project. Y.F. devised the methodology. P.S. performed the data analysis and generated the tables and figures. P.S. wrote the original draft, and all authors contributed to the editing and finalizing of the manuscript.
Supplemental Material
Download ()Supplemental Material
Download ()Supplemental Material
Download ()Supplemental Material
Download ()Supplemental Material
Download ()Supplemental Material
Download ()Supplemental Material
Download ()Supplemental Material
Download ()Supplemental Material
Download ()Disclosure statement
P.V., S.G., P.S., and Y.F. have patent applications and/or patents in the field of cell and gene therapy for cancer. S.G. consults for ViraCyte, is a member of the DSMB of Immatics, and receives research support unrelated to this project from Tessa Therapeutics.
Funding. This study was supported by the American Lebanese Syrian Associated Charities (ALSAC).
This study was also in part supported by the National Cancer Institute grant P30 CA021765. The content is solely the responsibility of the authors and does not recessarily represent the official views of the National Institute of Health.
Acknowledgments. We thank David Finkelstein, Hongjian Jin, Beisi Xu, Tim Shaw, and Lance Palmer for their helpful discussions. We also thank Vani Shanker for her help in manuscript preparation.
Supplementary material
Supplemental data for this article can be accessed on the publisher’s website
Correction Statement
This article has been republished with minor changes. These changes do not impact the academic content of the article.
Additional information
Funding
References
- Sr L, Mk D, Ahmedin J. Cancer statistics. CA Cancer J Clin. 2018;68(1):7–10. doi:10.3322/caac.21442.
- As G, Ta A, Jp P, Meshinchi S, Cogaml C. Children’s Oncology Group’s 2013 blueprint for research: acute myeloid leukemia. Pediatr Blood Cancer. 2013;60(6):964–971. doi:10.1002/pbc.24432.
- Zwaan CM, Kolb EA, Reinhardt D, Abrahamsson J, Adachi S, Aplenc R, Esjm DB, De Moerloose B, Dworzak M, Gibson BES, et al. Collaborative efforts driving progress in pediatric acute myeloid leukemia. J Clin Oncol. 2015;33(27):2949. doi:10.1200/JCO.2015.62.8289.
- Rubnitz JE. Current management of childhood acute myeloid leukemia. Pediatr Drugs. 2017;19(1):1–10. doi:10.1007/s40272-016-0200-6.
- Vardiman JW, Thiele J, Arber DA, Brunning RD, Borowitz MJ, Porwit A, Harris NL, Le Beau MM, Hellström-Lindberg E, Tefferi A, et al. The 2008 revision of the World Health Organization (WHO) classification of myeloid neoplasms and acute leukemia: rationale and important changes. Blood. 2009;114(5):937–951.
- Alvarez-Vallina L, Hawkins RE. Antigen-specific targeting of CD28-mediated T cell co-stimulation using chimeric single-chain antibody variable fragment-CD28 receptors. Eur J Immunol. 1996;26:2304–2309.
- Song D-G, Ye Q, Poussin M, Harms GM, Figini M, and Powell DJ. CD27 costimulation augments the survival and anti-tumor activity of redirected human T cells in vivo. Blood, The Journal of the American Society of Hematology. 2012;119(3): ;696–706.
- Quintarelli C, Orlando D, Boffa I, Guercio M, Polito VA, Petretto A, Lavarello C, Sinibaldi M, Weber G, Del Bufalo F, et al. Choice of costimulatory domains and of cytokines determines CAR T-cell activity in neuroblastoma. Oncoimmunology. 2018;7(6):e1433518.
- Alexander TB, Wang L, Inaba H, Triplett BM, Pounds S, Ribeiro RC, Pui C-H, Rubnitz JE. Decreased relapsed rate and treatment-related mortality contribute to improved outcomes for pediatric acute myeloid leukemia in successive clinical trials. Cancer. 2017;123:3791–3798.
- Kochenderfer JN, Dudley ME, Feldman SA, Wilson WH, Spaner DE, Maric I, Stetler-Stevenson M, Phan GQ, Hughes MS, Sherry RM, et al. B-cell depletion and remissions of malignancy along with cytokine-associated toxicity in a clinical trial of anti-CD19 chimeric-antigen-receptor–transduced T cells. Blood. 2011;118(21):167. blood–2011. doi:10.1182/blood.V118.21.167.167.
- Davila ML, Riviere I, Wang X, Bartido S, Park J, Curran K, Chung SS, Stefanski J, Borquez-Ojeda O, Olszewska M, et al. Efficacy and toxicity management of 19-28z CAR T cell therapy in B cell acute lymphoblastic leukemia. Sci Transl Med. 2014;6(224):224ra25–224ra25. doi:10.1126/scitranslmed.3008226.
- Maude SL, Teachey DT, Porter DL, and Grupp SA. CD19-targeted chimeric antigen receptor T cell therapy for acute lymphoblastic leukemia. Blood, The Journal of the American Society of Hematology 125 no. 26 . 2015;4017–4023.
- Creutzig U, Zimmermann M, Ritter J, Henze G, Graf N, Löffler H, Schellong G. Definition of a standard-risk group in children with AML. Br J Haematol. 1999;104(3):630–639. doi:10.1046/j.1365-2141.1999.01304.x.
- Creutzig U, Van Den Heuvel-Eibrink MM, Gibson B, Dworzak MN, Adachi S, De Bont E, Harbott J, Hasle H, Johnston D, and Kinoshita A, et al. Diagnosis and management of acute myeloid leukemia in children and adolescents: recommendations from an international expert panel, on behalf of the AML Committee of the International BFM Study Group. Blood, The Journal of the American Society of Hematology 120 no. 16 . 2012;3187–3205.
- Muñoz L, Nomdedéu JF, López O, Carnicer MJ, Bellido M, Avent A, Brunet S, Sierra J. Interleukin-3 receptor alpha chain (CD123) is widely expressed in hematologic malignancies. Haematologica. 2001;86:1261–1269.
- Ehninger A, Kramer M, Röllig C, Thiede C, Bornhäuser M, Von Bonin M, Wermke M, Feldmann A, Bachmann M, Ehninger G, et al. Distribution and levels of cell surface expression of CD33 and CD123 in acute myeloid leukemia. Blood Cancer J. 2014;4(6):e218. doi:10.1038/bcj.2014.39.
- Kenderian SS, Ruella M, Shestova O, Klichinsky M, Aikawa V, Morrissette JJD, Scholler J, Song D, Porter DL, Carroll M, et al. CD33-specific chimeric antigen receptor T cells exhibit potent preclinical activity against human acute myeloid leukemia. Leukemia. 2015;29(8):1637–1647. doi:10.1038/leu.2015.52.
- Bras AE, de Haas V, van Stigt A, Jongen-Lavrencic M, Beverloo HB, Te Marvelde JG, Zwaan CM, Jjm VD, Leusen JHW, Velden VHJ. van der Velden VHJ. CD123 expression levels in 846 acute leukemia patients based on standardized immunophenotyping. Cytom Part B Clin Cytom. 2019;96(2):134–142. doi:10.1002/cyto.b.21745.
- Tashiro H, Sauer T, Shum T, Parikh K, Mamonkin M, Omer B, Rouce RH, Lulla P, Rooney CM, Gottschalk S, et al. Treatment of acute myeloid leukemia with T cells expressing chimeric antigen receptors directed to C-type lectin-like molecule 1. Mol Ther. 2017;25(9):2202–2213. doi:10.1016/j.ymthe.2017.05.024.
- Hills RK, Castaigne S, Appelbaum FR, Delaunay J, Petersdorf S, Othus M, Estey EH, Dombret H, Chevret S, Ifrah N, et al. Addition of gemtuzumab ozogamicin to induction chemotherapy in adult patients with acute myeloid leukaemia: a meta-analysis of individual patient data from randomised controlled trials. Lancet Oncol. 2014;15(9):986–996. doi:10.1016/S1470-2045(14)70281-5.
- Thokala R, Olivares S, Mi T, Maiti S, Deniger D, Huls H, Torikai H, Singh H, Champlin RE, Laskowski T, et al. Redirecting specificity of T cells using the sleeping beauty system to express chimeric antigen receptors by mix-and-matching of VL and VH domains targeting CD123+ tumors. PLoS One. 2016;11(8):8. doi:10.1371/journal.pone.0159477.
- Orentas RJ, Yang JJ, Wen X, Wei JS, Mackall CL, Khan J. Identification of cell surface proteins as potential immunotherapy targets in 12 pediatric cancers. Front Oncol. 2012;2:194. doi:10.3389/fonc.2012.00194.
- Perna F, Berman SH, Soni RK, Mansilla-Soto J, Eyquem J, Hamieh M, Hendrickson RC, Brennan CW, Sadelain M. Integrating proteomics and transcriptomics for systematic combinatorial chimeric antigen receptor therapy of AML. Cancer Cell. 2017;32(4):506–519. doi:10.1016/j.ccell.2017.09.004.
- Lonsdale J, Thomas J, Salvatore M, Phillips R, Lo E, Shad S, Hasz R, Walters G, Garcia F, Young N, et al. The genotype-tissue expression (GTEx) project. Nat Genet. 2013;45(6):580. doi:10.1038/ng.2653.
- Jde DR, Branstetter C, Ma J, Li Y, Walsh MP, Cheng J, Obulkasim A, Dang J, Easton J, Verboon LJ, et al. Pediatric non–Down syndrome acute megakaryoblastic leukemia is characterized by distinct genomic subsets with varying outcomes. Nat Genet. 2017;49(3):451–456. doi:10.1038/ng.3772.
- Bolouri H, Farrar JE, Triche JT, Ries RE, Lim EL, Alonzo TA, Ma Y, Moore R, Mungall AJ, Marra MA, et al. The molecular landscape of pediatric acute myeloid leukemia reveals recurrent structural alterations and age-specific mutational interactions. Nat Med. 2018;24(1):103. doi:10.1038/nm.4439.
- Hubbard T, Barker D, Birney E, Cameron G, Chen Y, Clark L, Cox T, Cuff J, Curwen V, Down T, et al. The Ensembl genome database project. Nucleic Acids Res. 2002;30(1):38–41. doi:10.1093/nar/30.1.38.
- Uhlen M, Oksvold P, Fagerberg L, Lundberg E, Jonasson K, Forsberg M, Zwahlen M, Kampf C, Wester K, Hober S, et al. Towards a knowledge-based human protein atlas. Nat Biotechnol. 2010;28(12):1248. doi:10.1038/nbt1210-1248.
- Scanlan MJ, Gure AO, Jungbluth AA, Old LJ, Chen Y-T. Cancer/testis antigens: an expanding family of targets for cancer immunotherapy. Immunol Rev. 2002;188(1):22–32. doi:10.1034/j.1600-065X.2002.18803.x.
- Simpson AJG, Caballero OL, Jungbluth A, Chen Y-T, Old LJ. Cancer/testis antigens, gametogenesis and cancer. Nat Rev Cancer. 2005;5(8):615. doi:10.1038/nrc1669.
- Rapoport AP, Stadtmauer EA, Binder-Scholl GK, Goloubeva O, Vogl DT, Lacey SF, Badros AZ, Garfall A, Weiss B, Finklestein J, et al. NY-ESO-1–specific TCR–engineered T cells mediate sustained antigen-specific antitumor effects in myeloma. Nat Med. 2015;21(8):914. doi:10.1038/nm.3910.
- Wang C, Gu Y, Zhang K, Xie K, Zhu M, Dai N, Jiang Y, Guo X, Liu M, Dai J, et al. Systematic identification of genes with a cancer-testis expression pattern in 19 cancer types. Nat Commun. 2016;7(1):1–12.
- Trapnell C, Williams BA, Pertea G, Mortazavi A, Kwan G, van Baren MJ, Salzberg SL, Wold BJ, Pachter L. Transcript assembly and abundance estimation from RNA-Seq reveals thousands of new transcripts and switching among isoforms. Nat Biotechnol. 2010;28(5):511. doi:10.1038/nbt.1621.
- Lockhart DJ, Dong H, Byrne MC, Follettie MT, Gallo MV, Chee MS, Mittmann M, Wang C, Kobayashi M, Norton H, et al. Expression monitoring by hybridization to high-density oligonucleotide arrays. Nat Biotechnol. 1996;14(13):1675. doi:10.1038/nbt1296-1675.
- Liu W, Mei R, Di X, Ryder TB, Hubbell E, Dee S, Webster TA, Harrington CA, Ho M, Baid J, et al. Analysis of high density expression microarrays with signed-rank call algorithms. Bioinformatics. 2002;18(12):1593–1599. doi:10.1093/bioinformatics/18.12.1593.
- Bolstad BM, Irizarry RA, Åstrand M, Speed TP. A comparison of normalization methods for high density oligonucleotide array data based on variance and bias. Bioinformatics. 2003;19:185–193.
- Haferlach T, Kohlmann A, Wieczorek L, Basso G, Te KG, M-c B, De JV, Hernández JM, Hofmann W-K, Mills KI, et al. Clinical utility of microarray-based gene expression profiling in the diagnosis and subclassification of leukemia: report from the International Microarray Innovations in Leukemia Study Group. J Clin Oncol Off J Am Soc Clin Oncol. 2010;28(15):2529–2537. doi:10.1200/JCO.2009.23.4732.
- Beier D, Hau P, Proescholdt M, Lohmeier A, Wischhusen J, Oefner PJ, Aigner L, Brawanski A, Bogdahn U, Beier CP. CD133+ and CD133- glioblastoma-derived cancer stem cells show differential growth characteristics and molecular profiles. Cancer Res. 2007;67(9):4010–4015. doi:10.1158/0008-5472.CAN-06-4180.
- Markman B, Javier Ramos F, Capdevila J, Tabernero J. EGFR and KRAS in colorectal cancer. Adv Clin Chem. 2010;51:72.
- Han J, Chu J, Chan WK, Zhang J, Wang Y, Cohen JB, Victor A, Meisen WH, Kim S, Grandi P, et al. CAR-engineered NK cells targeting wild-type EGFR and EGFRvIII enhance killing of glioblastoma and patient-derived glioblastoma stem cells. Sci Rep. 2015;5:11483.
- Davies JR, Kirkham S, Svitacheva N, Thornton DJ, Carlstedt I. MUC16 is produced in tracheal surface epithelium and submucosal glands and is present in secretions from normal human airway and cultured bronchial epithelial cells. Int J Biochem Cell Biol. 2007;39:1943–1954.
- Blumenthal RD, Leon E, Hansen HJ, Goldenberg DM. Expression patterns of CEACAM5 and CEACAM6 in primary and metastatic cancers. BMC Cancer. 2007;7:2.
- Zheng C, Feng J, Lu D, Wang P, Xing S, Coll J-L, Yang D, Yan X. A novel anti-CEACAM5 monoclonal antibody, CC4, suppresses colorectal tumor growth and enhances NK cells-mediated tumor immunity. PLoS One. 2011;6:e21146.
- Eisenberg E, Levanon EY. Human housekeeping genes, revisited. Trends Genet. 2013;29:569–574.
- Majzner RG, Mackall CL. Tumor antigen escape from CAR T-cell therapy. Cancer Discov. 2018;8:1219–1226.
- Kochenderfer JN, Wilson WH, Janik JE, Dudley ME, Stetler-Stevenson M, Feldman SA, Maric I, Raffeld M, Nathan D-AN, and Lanier BJ, et al. Eradication of B-lineage cells and regression of lymphoma in a patient treated with autologous T cells genetically-engineered to recognize CD19. Blood, The Journal of the American Society of Hematology 116 no. 20 . 2010;4099–4102.
- Qin H, Nguyen SM, Ramakrishna S, Tarun S, Yang L, Verdini NP, Haso W, Fry TJ. Novel CD19/CD22 Bicistronic Chimeric Antigen Receptors Outperform Single or Bivalent Cars in Eradicating CD19+ CD22+, CD19-, and CD22-Pre-B Leukemia. 2017.
- Bose TO, Colpitts SL, Pham Q-M, Puddington L, and Lefrançois L. CD11a is essential for normal development of hematopoietic intermediates. The Journal of Immunology 193 no. 6 . 2014;2863–2872.
- Hathcock KS, Hirano H, Murakami S, Hodes RJ. CD45 expression by B cells. Expression of different CD45 isoforms by subpopulations of activated B cells. J Immunol. 1992;149:2286–2294.
- Vazquez BN, Laguna T, Carabana J, Krangel MS, Lauzurica P. CD69 gene is differentially regulated in T and B cells by evolutionarily conserved promoter-distal elements. J Immunol. 2009 jimmunol–0900839;183(10):6513–6521. doi:10.4049/jimmunol.0900839.
- Parnes JR, Pan C. CD72, a negative regulator of B-cell responsiveness. Immunol Rev. 2000;176:75–85.
- Vela JAG, Delgado I, Benito L, Monteserin MC, Alonso LG, Somolinos N, Andreu MA, Ona F. CD79b expression in B cell chronic lymphocytic leukemia: its implication for minimal residual disease detection. Leukemia. 1999;13(10):1501. doi:10.1038/sj.leu.2401511.
- Kiyokawa N, Sekino T, Matsui T, Takenouchi H, Mimori K, Tang W, Matsui J, Taguchi T, Katagiri YU, Okita H, et al. Diagnostic importance of CD179a/b as markers of precursor B-cell lymphoblastic lymphoma. Mod Pathol. 2004;17(4):423.
- Tangye SG, Avery DT, Deenick EK, Hodgkin PD. Intrinsic differences in the proliferation of naive and memory human B cells as a mechanism for enhanced secondary immune responses. J Immunol. 2003;170(2):686–694. doi:10.4049/jimmunol.170.2.686.
- Chillemi A, Zaccarello G, Quarona V, Lazzaretti M, Martella E, Giuliani N, Ferracini R, Pistoia V, Horenstein AL, Malavasi F. CD38 and bone marrow microenvironment. 2014.
- Jr D, Rk W, Zhang J, Er M, C-h P, Ding L, Tj L, We E. The pediatric cancer genome project. Nat Genet. 2012;44(6):619. doi:10.1038/ng.2287.
- Ma X, Liu Y, Liu Y, Alexandrov LB, Edmonson MN, Gawad C, Zhou X, Li Y, Rusch MC, Easton J, et al. Pan-cancer genome and transcriptome analyses of 1,699 paediatric leukaemias and solid tumours. Nature. 2018;555(7696):371. doi:10.1038/nature25795.
- Povey S, Lovering R, Bruford E, Wright M, Lush M, The WH. HUGO gene nomenclature committee (HGNC). Hum Genet. 2001;109(6):678–680. doi:10.1007/s00439-001-0615-0.
- Haso W, Lee DW, Shah NN, Stetler-Stevenson M, Yuan CM, Pastan IH, Dimitrov DS, Morgan RA, FitzGerald DJ, Barrett DM, et al. Anti-CD22–chimeric antigen receptors targeting B-cell precursor acute lymphoblastic leukemia. Blood. 2013;121(7):1165–1174. doi:10.1182/blood-2012-06-438002.
- Cui Q, Qian C, Xu N, Kang L, Dai H, Cui W, Song B, Yin J, Li Z, Zhu X, et al. CD38-directed CAR-T cell therapy: a novel immunotherapy strategy for relapsed acute myeloid leukemia after allogeneic hematopoietic stem cell transplantation. J Hematol & Oncol. 2021;14(1):1–5.
- Tambaro FP, Singh H, Jones E, Rytting M, Mahadeo KM, Thompson P, Daver N, DiNardo C, Kadia T, and Garcia-Manero G, et al. Autologous CD33-CAR-T cells for treatment of relapsed/refractory acute myelogenous leukemia. Leukemia . 2021;1–5.
- Burnett AK, Russell NH, Hills RK, Kell J, Freeman S, Kjeldsen L, Hunter AE, Yin J, Craddock CF, Dufva IH, et al. Addition of gemtuzumab ozogamicin to induction chemotherapy improves survival in older patients with acute myeloid leukemia. J Clin Oncol. 2012;30(32):3924–3931.
- Krejcik J, Casneuf T, Nijhof IS, Verbist B, Bald J, Plesner T, Syed K, Liu K, Nwcj VDD, Weiss BM, et al. Daratumumab depletes CD38+ immune regulatory cells, promotes T-cell expansion, and skews T-cell repertoire in multiple myeloma. Blood. 2016;128(3):384–394.
- Tasian SK. Acute myeloid leukemia chimeric antigen receptor T-cell immunotherapy: how far up the road have we traveled? Ther. Adv Hematol. 2018;9:135–148.
- Gomes-Silva D, Atilla E, Atilla PA, Mo F, Tashiro H, Srinivasan M, Lulla P, Rouce RH, Cabral JMS, Ramos CA, et al. CD7 CAR T cells for the therapy of acute myeloid leukemia. Mol Ther. 2019;27(1):272–280.
- Uy GL, Godwin J, Rettig MP, Vey N, Foster M, Arellano ML, Rizzieri DA, Topp MS, Huls G, Lowenberg B, et al. Preliminary results of a phase 1 study of flotetuzumab, a CD123 x CD3 bispecific Dart®protein, in patients with relapsed/refractory acute myeloid leukemia and myelodysplastic syndrome. 2017.
- Saito Y, Kitamura H, Hijikata A, Tomizawa-Murasawa M, Tanaka S, Takagi S, Uchida N, Suzuki N, Sone A, Najima Y, et al. Identification of therapeutic targets for quiescent, chemotherapy-resistant human leukemia stem cells. Sci Transl Med. 2010;2(17):17ra9–17ra9.
- Martin EW, Buzza MS, Driesbaugh KH, Liu S, Fortenberry YM, Leppla SH, Antalis TM. Targeting the membrane-anchored serine protease testisin with a novel engineered anthrax toxin prodrug to kill tumor cells and reduce tumor burden. Oncotarget. 2015;6:33534.
- Proto-Siqueira R, Figueiredo-Pontes LL, Panepucci RA, Garcia AB, Rizzatti EG, Nascimento FM, Ishikawa HCF, Larson RE, Falcao RP, Simpson AJ, et al. PRAME is a membrane and cytoplasmic protein aberrantly expressed in chronic lymphocytic leukemia and mantle cell lymphoma. Leuk Res. 2006;30(11):1333–1339.
- Tang T, Kmet M, Corral L, Vartanian S, Tobler A, Papkoff J. Testisin, a Glycosyl-Phosphatidylinositol–Linked Serine Protease, Promotes Malignant Transformation In vitro and In vivo. Cancer Res. 2005;65:868–878.
- Baren V. PRAME, a gene encoding an antigen recognized on a human melanoma by cytolytic T cells, is expressed in acute leukaemia cells. Br J Haematol. 1998;102:1376–1379.
- Ataga KI, Smith WR, De Castro LM, Swerdlow P, Saunthararajah Y, Castro O, Vichinsky E, Kutlar A, Orringer EP, Rigdon GC, et al. Efficacy and safety of the Gardos channel blocker, senicapoc (ICA-17043), in patients with sickle cell anemia. Blood. 2008;111(8):3991–3997.
- Alizadeh AA, Eisen MB, Davis RE, Ma C, Lossos IS, Rosenwald A, Boldrick JC, Sabet H, Tran T, Yu X, et al. Distinct types of diffuse large B-cell lymphoma identified by gene expression profiling. Nature. 2000;403(6769):503.
- Pradhan D, Morrow JS. The spectrin-ankyrin skeleton controls CD45 surface display and interleukin-2 production. Immunity. 2002;17:303–315.
- Kolde R, and Kolde, M. Package 'pheatmap'. R Package 1 7 (:) . 2012. 790.
- Morgan M, Carey V, BiocParallel: LM. Bioconductor facilities for parallel evaluation. R Packag Version. 2014;0.4:1.
- Wickham H. Data manipulation with dplyr. In: R user conference. Vol. 30. 2014.
- Venables WN, and Ripley BD. Modern Applied Statistics with S. Fourth. New York: Springer; 2002.