ABSTRACT
Tailored treatment for patients with rectal cancer requires clinically available markers to predict their response to neoadjuvant treatment. The quantity of tumor-infiltrating lymphocytes (TILs) in pre-operative tumor biopsies has been suggested to predict a favorable response, but opposing results exist. A biopsy-adapted Immunoscore (ISB) based on TILs has recently emerged as a promising predictor of tumor regression and prognosis in (colo)rectal cancer. We aimed to refine the ISB for prediction of response using multiplex immunofluorescence (mIF) on pre-operative rectal cancer biopsies. We combined the distribution and density of conventional T cell subsets and γδT cells with a type I Interferon (IFN)-driven response assessed using Myxovirus resistance protein A (MxA) expression. We found that pathological complete response (pCR) following neoadjuvant treatment was associated with type I IFN. Stratification of patients according to the density of CD8+ in the entire tumor tissue and MxA+ cells in tumor stroma, where equal weight was assigned to both parameters, resulted in improved predictive quality compared to the ISB. This novel stratification approach using these two independent parameters in pre-operative biopsies could potentially aid in identifying patients with a good chance of achieving a pCR following neoadjuvant treatment.
Introduction
Although surgery is the cornerstone for curative treatment in rectal cancer, neoadjuvant treatment reduces local recurrence and improves the chance of radical resection. Indeed, up to 20% of patients respond to neoadjuvant treatment with a clinical complete responseCitation1 defined as the absence of a clinically detectable tumor. However, neoadjuvant treatment increases the risk of post-operative complications and long-term side effects, and the risk-benefit trade-off for individual patients is important to consider. Unfortunately, few predictive markers for response to neoadjuvant treatment are available to discriminate between good and poor responders. Evaluating tumor-infiltrating lymphocyte (TILs) density in colorectal cancer (CRC) has led to the introduction of immunoscore (IS) as a prognostic indicator for survivalCitation2, perhaps even superior to the anatomically based TNM classificationCitation3–6. Still, whether pre-therapeutic density and distribution of TILs could be used to predict response to neoadjuvant treatment is uncertain, albeit reported in some studiesCitation7–10. In particular, the density of CD8+ TILs has been suggested as an independent predictor of complete responseCitation11. However, contradictory results have been reportedCitation12.
Granzyme B (GrzB) from cytotoxic T cells (CTLs) enters targeted cells through perforin-induced permeabilization and, via caspases, triggers apoptosis in tumor cellsCitation13. Increased numbers of intraepithelial GrzB+ CTLsCitation14 and upregulation of gene expression related to cytotoxic activity have been associated with higher tumor regression after neoadjuvant treatmentCitation7. Type I Interferon (IFN) can augment the priming of CTLs by promoting cross-presentation of antigens from dying target cellsCitation15. Tumor cell-derived DNA released from dying cells stimulates the cGAS-STING pathway, resulting in type I IFN production. Radiotherapy enhances this processCitation16,Citation17 Improved tumor antigen presentation via adaptive immune cells can then occur and fuel the cancer immune cycle leading to eradication of the the tumorCitation17,Citation18. Type I interferon binding to cell surface receptors triggers a signaling cascade inducing over 300 IFN-stimulated genes (ISGs). ISG15, ISG56 (IFIT1), myxovirus resistance protein A (MxA), and OAS are ISGs that serve as surrogate markers for type I interferon activity and are commonly used in experimental settings. Cells exposed to type I IFN express MxA through the JAK/STAT signaling pathwayCitation19. MxA upregulation is solely dependent on type I IFN in contrast to other ISGs that are also directly inducible by dsRNA and virusesCitation20,Citation21. In addition, immunofluorescence (IF) staining for MxA has been successfully used to detect the presence of a type I IFN response in the skin of humansCitation22. Hence, MxA can be used as a footprint for type I IFN in the tumor microenvironment (TME). Despite that γδT cells can quickly detect transformed cells, the contribution of this small population of cells, which are rare in the TME, remains controversialCitation23. Whether MxA-expressing cells and/or γδT cells in pre-operative cancer biopsies are indicative of a TME favorable to generating an effective anti-tumor response following neoadjuvant treatment has yet to be explored.
This study used multiplex immunofluorescence (mIF) to determine if the density and spatial distribution of T cell subsets in pre-operative biopsies from patients with rectal cancer could predict the response to neoadjuvant treatment. In addition, we investigated MxA expression, as a type I IFN footprint, to determine whether this could further improve the predictive capacity.
Materials and methods
Population clinical variables and parameters
1774 patients treated for rectal cancer at Sahlgrenska University Hospital/Östra Sjukhuset, Gothenburg in Sweden between 2007 and 2019 were identified in the Swedish Colorectal Cancer Registry. Of these, 130 patients were included in the study as they were treated with neoadjuvant therapy with an interval to surgical resection >14 days that enables a possible tumor regression after treatment. Clinical data were retrieved from the registry. Reasons for exclusion are presented in a flow chart in Figure S1. Demographic characteristics of the study population and clinical parameters, including T-stage and type of neoadjuvant treatment that are possible factors that may predict response to neoadjuvant treatmentCitation24 are presented in .
Table 1. Demographic characteristics of the study population.
Immunofluorescence staining
Formalin-Fixed Paraffin-Embedded (FFPE) blocks of the pre-operative biopsies were sectioned with a Microtome into 4 μm sections and fixed on Superfrost Plus microscope slides (Thermo Scientific; Braunschweig, Germany). Slides were kept at room temperature overnight and then incubated at 60°C for 1 h. Deparaffinization prior to staining was performed by submerging the slides for 5 min in xylene (twice) and in ethanol [99.5% (twice), 95%, 70%] and distilled water. Deparaffinized slides were stained as previously describedCitation25. Antibodies and TSA reagents used for the mIF are specified in Table S1.
Hematoxylin and Eosin (H&E)
Deparaffinized slides were stained with Hematoxylin solution (Histolab; Gothenburg, Sweden) for 5 min, followed by a 3-min wash in water and then stained with Eosin (Histolab) for 30 s. The slides were then placed in ethanol [70%, 95% 99.5% (twice)] for 10 quick dips which followed by two 5-min incubations in xylene before mounting with Pertex (Histolab).
Image analyses
All pre-treatment biopsy specimens were stained with H&E and examined by a pathologist, who also marked the invasive tumor areas. The corresponding areas were then localized on immunofluorescence-stained sections (Figure S2a). Immunofluorescence-stained slides were scanned with TissueFAXS (TissueGnostics; Vienna, Austria) and analyzed with the StrataQuest Software (v. 7.0.1.189; TissueGnostics) as inCitation25. In addition, the intensity of the antibody fluorescently labeled markers was defined and used to identify the different cell types. To confirm the proper cutoff detection level, 10 samples were randomly selected in the cohort; several smaller areas in the sections were defined and the number of cells in these areas was counted separately by two investigators. The mean values of manually counted cells were then used to validate the numbers counted by the software. The staining intensity of CD3 varied slightly between sections. Therefore, three different groups were identified based on the mean intensity of CD3 staining: weak (<8000), intermediate (8000–10000), and strong (>10000). The cutoff value used for defining CD3 expressing cells was set accordingly.
Tumor regression
Tissue slides from the surgical specimens were retrieved for all patients, and the tumor regression grade (TRG) according to the AJCCCitation26 was determined by a pathologist (G.D.). TRG is graded from 0 to 3, where 0 denotes pathological complete response, and 3 denotes no response to the neoadjuvant treatment. Uncertainties regarding tumor regression were resolved by consulting a second, senior gastrointestinal pathologist. TRG served as a determinator of response to neoadjuvant treatment. Investigators involved in preparing and analyzing the pre-operative tissue slides were blinded to the TRG, until the point of statistical analysis when all mIF analyses were completed.
Heat-map based Immunoscore determination
The ranked percentiles of CD8+ T cell density in tumor tissue and MxA+ cells density in tumor stroma for each patient were first separately determined. Each patient was then assigned to one of the three categories: high (>70; pink), intermediate (25–70; white), or low (<25; blue) for the CD8 as well as the MxA values. The cutoff values (high, intermediate, and low) were based on the previously published biopsy-adapted Immunoscore (ISB)Citation7. Results were then color-coded in the heat-map as follows: dark red (if both CD8 and MxA values were high), red (if one value was high and the other was intermediate), light red (if one value was high and the other was low), white (if both values were intermediate), light blue (if one value was intermediate and the other was low), and dark blue (if both values were low). These categories formed the basis for the subsequent analyses.
Statistical analysis
Cell densities were compared using Wilcoxon’s rank sum test. Associations between markers were based on Pearson correlation coefficient analyses. Unilateral linear-by-linear association test was used to analyze the ordinal data. Logistic regression analyses were performed using the glm() function in R. Tests were considered significant at p < 0.05. All analyses were performed using GraphPad Prism software, v.9.0.2. (San Diego, CA, USA) and R language and environment for statistical computing, v.4.2.1 (Vienna, Austria).
Results
Number and spatial distribution of tumor infiltrating lymphocytes and response to neoadjuvant treatment
Multiplex IF stainings were performed (Table S1; Figure S2) and cells of interest were counted digitally within the defined tumor border (Figure S2a-b, S3). To ensure the accurate quantification of conventional T cells, γδTCR-expressing cells were subtracted from CD3+ cells and analyzed separately. The median density of conventional CD3+ and CD3+CD8+T cells were 1065.43 (range: 102.51–3766.39) and 184.34 (range: 10.1–1633.8) cells/mm2 in the biopsy, respectively (Figure S3). The mean densities of the T cell subsets were plotted according to TRG (). There was no significant difference in the number of conventional T cells or γδT cells between complete responders and non-responders (). Similarly, when patients with TRG 0 were compared to the pooled TRG 1–3 group, no significant differences were observed.
Figure 1. Enumeration of T cells subsets in tumor tissue according to the effectiveness of treatment. (a) Bar charts show the mean density ±SEM of the CD3.+ T cells, CD3+CD8+ T cells, CD3+CD8+GrzB+ Cytotoxic T cells, and γδTCR+cells per mm2of the area according to the patients’ response to treatment in whole tumor tissue. (b) In the epithelium 50µm surrounding area (c) and only epithelium. (d) Bar charts show the mean density ±SEM of the T cells subsets per mm2 of the whole tumor tissue in complete responders v.s non-responders in whole tumor tissue. (e) In the epithelium and the area with 50µm width around it (f) and only epithelium. GrzB: Granzyme B, TRG: Tumor Regression Grade. Wilcoxon's rank sum test p <0.05 considered significant; n=130.
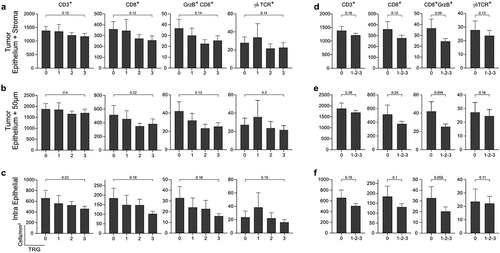
To assess the potential impact of spatial distribution of TILs in pre-operative biopsies, the tumor center (PanCK+ cells), tumor stroma (the remaining area within the tumor), and stromal area up to 50 µm from the tumor cells were annotated (Figure S2), and T cell subsets quantified digitally. No significant differences were detected in the number of conventional T cells or γδT cells, between complete responders (TRG 0) and non-responders (TRG 3). Similarly, there was no difference when TRG 0 was compared to a pooled TRG 1–3 group, regardless of whether cells were assessed in the tumor epithelium alone or together with 50 µm of surrounding stroma and only a trend was detected when assessing CD8+GrzB+ T cells, e.g. CTLs ().
Hence, the number of γδT cells, conventional T cells, and subsets thereof were not significantly different in the pre-operative tumor biopsies of complete responders (the total tumor area, the proximity, and/or the tumor epithelium) compared to other patients with lesser or no response to neoadjuvant treatment.
Extent of stromal type I interferon footprint and response to neoadjuvant treatment
MxA is expressed in cells exposed to type I or III IFN, but the capacity to respond to the latter is limited to intestinal epithelial cells, while cells in the lamina propria respond to type I IFNCitation27. We hypothesized that cells expressing MxA would indicate an environment permissive to mount a strong anti-tumor response following irradiation. The density of MxA+ cells in the tumor stroma was determined digitally and plotted against the TRG groups ( and Figure S2b). A significantly increased number of MxA+ cells were observed when TRG 0 was compared to TRG 3 or to the pooled group of TRG 1–3 (). However, no correlation between the number of MxA+ cells and either of the T cell subsets, including the CTLs, in the tumor was observed (Figure S4). Finally, a logistic regression model was constructed to explore the explanation of tumor regression based on cell densities, but the model did not reach significance neither for MxA nor CD8+ T cells (Table S2).
Figure 2. Enumeration of MxA+ cells in tumor stroma. (a) Bar charts show the mean density ±SEM of MxA+ Pan-CK− cells per mm2 of the tumor stroma according to the patients’ response to the treatment. (b) Bar charts show the mean density ±SEM of the MxA+ cells per mm2 of tumor stroma amongst complete responders and non-responders. TRG: Tumor Regression Grade. Wilcoxon's rank sum test; p <0.05 considered significant; n=130.
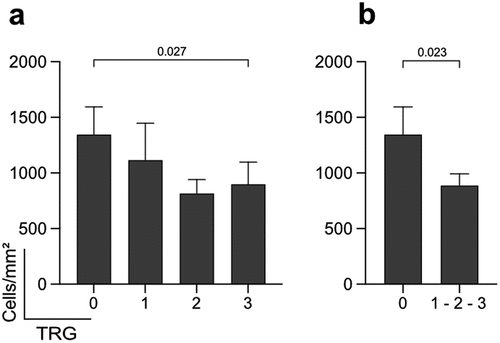
Collectively, this shows that quantification of MxA expression in the tumor stroma could aid in identifying patients with the capacity to mount a strong response to neoadjuvant treatment.
IF-based quantification models and response to neoadjuvant treatment
Using the biopsy-adapted Immunoscore (ISB), determined within a cohort of patients by the mean value of the ranked percentile of CD3+ and CD8+ cell densities (Figure S5a), the cohort was divided into high (>70), intermediate (25–70) and low (<25) (Figure S5a). As previously reported,Citation7 we also found an accumulation of ISB high (46.1%) and few ISB low (7.7%) in the TRG 0 group (). In contrast, in the TRG 3 group ISB high constituted 20.9% and ISB low 27.9%. Dividing the cohort into three percentiles based on MxA densities in the tumor stroma yielded a very similar pattern as observed with the ISB score as well as with CD3 and CD8 separately (). The association between the MxA and TRG scores was determined to be significant using unilateral linear-by-linear association tests. The densities of CD8+ cells and MxA+ cells (Figure S4), as well as the ranked percentile of CD8+ and MxA+ cells (Figure S5b), were not correlated. The combination of these two parameters () did not result in improved separation. Thus, we used a heat-map approach to display the patients in the respective percentiles based on either CD3+CD8+ in tissue or MxA+ cells in tumor stroma (Figure S6). This revealed that among the patients with TRG 0, all but one were classified as high percentile of either CD8 or MxA ( and S6). This extended analysis based on both parameters also showed that none of the patients with TRG 0 were in the low percentiles of both parameters, while among TRGs 1–3 this group constituted approximately 10% ( and Figure S6). Furthermore, a pattern of gradual increase of patients that were in the high percentile of both parameters was noted as the effect of the neoadjuvant treatment increased – i.e., from TRG 3 toward TRG 0 (). The association between the heat-map approach and complete tumor regression was also tested using logistic regression (Table S2). Here, the heat-map approach did reach significance (p < 0.01). Including clinical parameters known to be associated with tumor regression (T-stage and type of neoadjuvant treatment) in a multivariable regression model diminished the significance of the heat map-based approach (p < 0.01). Neither of the clinical parameters were significant, which could be due to lack of power.
Figure 3. Classification of the patients according to the effectiveness of treatment. (a)The frequency of patients with CD3+ (Left-Upper), CD8+ (Middle –Upper) and MxA+ (Right-Upper), low (Blue), intermediate (White), and high (Red) according to tumor regression and the frequency of ISB (Left-Lower) and ISMxA+CD8+ (Right-Lower) low, intermediate, and high according to tumor regression. (b) The frequency of patients with either CD8+ or MxA+ high, intermediate and low according to the tumor regression. TRG: Tumor Regression Grade. Unilateral linear-by-linear association test; p <0.05 considered significant; n=130.
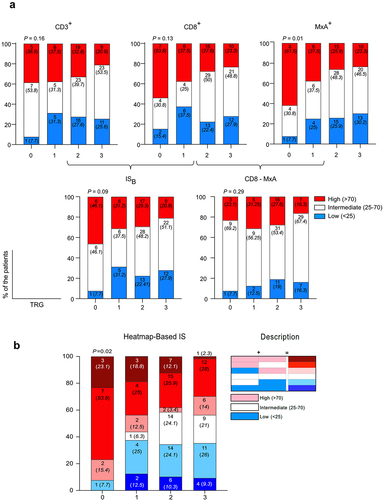
To statistically compare the quantifications using ranked percentiles of CD3+, CD8+, or MxA+ with ISB (CD3+ and CD8+), (CD8+ and MxA+) and the heat-map based (CD8+ or MxA+) models, the sensitivity, specificity, and diagnostic odds ratio were determined (). This revealed that the (CD8+ or MxA+) model had a slightly reduced specificity but increased sensitivity compared to the other single-cell quantifications or models and, importantly, resulted in the highest diagnostic odds ratio (). In conclusion, an intracohort scoring system based on equal weighing of two separate quantifications (total CD8+ T cells and stromal MxA) in the rectal cancer patient’s pre-operative biopsy can help to predict the extent of tumor regression ensuing the neoadjuvant treatment.
Table 2. Sensitivity, specificity, and diagnostic odds ratio of immune markers in prediction of response to treatment.
Discussion
Categorizing CRC patients using Immunoscore has previously been shown to have a strong prognostic power. Indeed, this may even surpass the anatomical-based TNM classification for predicting post-operative survivalCitation2,Citation28. A prediction tool designed for biopsies from rectal cancer patients (ISB) has recently shown considerable positive correlation between the density of TILs and neoadjuvant treatment responseCitation7. Here, we aimed to assess if improving the quality of predictability (sensitivity and/or specificity) by extending the parameters enumerated using mIF could be achieved.
Using mIF, we found similar densities of CD3 and CD8-expressing cells in biopsies as previously describedCitation7, but there was no significant difference according to TRG. The trend of increased TILs and pathological complete response pCR was slightly improved when the expression of GrzB was included, but even when CD8+GrzB+ TILs, i.e., CTLs in the tumor center were quantified, the prediction was not sufficient. Some studies have found a strong correlation between T cell infiltration in the tumor and response to neoadjuvant treatment in rectal cancerCitation7,Citation9 and other types of cancersCitation29,Citation30. Yang et al. observed that a high baseline influx of CD8+ T cells in rectal cancers was significantly associated with pCRCitation31. This has also been reported in studies of CRCCitation10,Citation11,Citation32–34. In contrast, other studies have not found a significant correlation between TIL density in pre-operative biopsies and tumor shrinkage after neoadjuvant treatmentCitation12,Citation35. This discrepancy may be due to the different evaluation systems used for TRG, cutoff values set for high and low influx of TILs, size of the cohorts, and/or intracohort dependency in the quantification model. It is also possible that our mIF approach could result in slightly increased variability compared to a colorimetric analysis of single parameters. Gene expression analyses of biopsies from patients with rectal cancer have revealed upregulation of GrzB in patients showing complete or partial response to neoadjuvant treatmentCitation7,Citation36. These findings are in line with the slight increase in the capacity to separate pCR from other patients we observed when the GrzB parameter was included. We found no association between γδTILs and tumor regression after neoadjuvant treatment. However, these unconventional T cells are emerging as a double-edged sword in the TMECitation37 with both cytotoxic and immunosuppressive effectsCitation38. Indeed, an imbalance between the subtypes of γδ TILs with these opposing roles has been proposed in rectal cancerCitation39. Therefore, evaluating the predictive capacity of γδTILs for neoadjuvant treatment response in rectal cancer might require subset analyses, which was not possible here due to the limitation of cell markers in multiplex staining.
We assessed the response to type I IFN by quantification of MxA-expressing cells selectively in the tumor stroma. This revealed a significantly higher density of MxA-expressing cells in tumor stroma of patients with pCR compared to patients with a partial or no response, suggesting that patients with pCR are exposed to higher levels of type I IFN. The importance of type I IFN in the cancer immune cycle has been ascribed to promoting a vigorous anti-tumor response by fostering cross-priming of CTLsCitation17. A recent study using murine colon carcinoma cells determined that during the anti-tumor response, type I IFN controls the effector function of CTLs by triggering the STAT3-GrzB axis resulting in GrzB transcription in immune cellsCitation40. However, only a very weak correlation between the density of cells exposed to type I IFN and TIL subsets, including CTLs, was detected in our cohort. One could speculate that CTLs have already migrated from the stroma under the influence of type I IFN and entered the tumor epithelium to perform effector functions. However, no significant association was found at this location either. When we used an approach similar to that used to create the ISB tool (dividing the cohort into low, high, and intermediate ranked quartiles), but instead used the density of MxA-expressing cells in the stroma, we found comparable – actually slightly improved – sensitivity compared to ISB. This supports that a type I IFN footprint could be an independent factor in identifying rectal cancer patients in whom pCR could be achieved after neoadjuvant treatment. Although MxA is widely used as a marker when measuring type I IFN biological activity, it does not show the direct presence of the cytokine, which subtype of type I interferon that is secreted or which cell that is the actual producer. Hence, additional studies are required to address this in the TME of rectal cancers.
Although our attempts to use CD3 and/or CD8 densities to predict the response to neoadjuvant treatment did not reach statistical significance, our use of the ISB tool resulted in similar results to those previously publishedCitation7. Our qualitative heat-map approach stratified the patients into six groups according to either CD8+ cell count in the tumor and/or MxA+ cells in tumor stroma. This quantification method found a significant association with tumor regression in response to neoadjuvant treatment. Furthermore, our method achieved a higher diagnostic odds ratio, compared to either ISB (CD3 and CD8) or MxA and CD8 as single parameters implying improved ability to classify patients’ response. Our approach also revealed that all patients except one (7.7%) in the pCR group were in the high quartile of either CD8 or MxA and that 23.1% were high in both, while none were low in both. In contrast in the group with no detectable response (TRG 3) only one patient (2.3%) was in both high quartiles. The stronger response to neoadjuvant treatment in patients with high numbers of TILs prior to treatment could be due to the fact that, although productive priming of tumor-specific CTLs has occurred, the level of activation is insufficient to overcome the suppressive TME to enable tumor eradication. However, following neoadjuvant treatment, tumor antigens are released from dying cells and taken up by professional antigen presenting cells (pAPCs) in tumor tissues that, with sufficient co-stimulation, can reinvigorate and unleash the CTLs in patients with higher numbers of TILs. Indeed, tumors with type I IFN driven-inflammation can create a TME permissive for TIL-mediated tumor eradicationCitation41. Such tumors would be identified by increased density of MxA-expressing cells. Neoadjuvant treatment could, through the induction of tumor cell death, release sufficient antigens to allow pAPCs under the influence of type I IFN to ignite the cancer immune cycle and activate tumor-specific T cells. This may explain why a TME with both a high number of CTLs and a type I IFN footprint would be the most proficient in generating an anti-tumor response following neoadjuvant treatment, while either could suffice on their own.
In this study, we explored the correlations between infiltration of T cell subsets, type I IFN response, and tumor regression following neoadjuvant treatment. We stratified the patients according to the density of CD8+ and MxA+ cells in the entire tumor tissue and tumor stroma, respectively, with a heat-map approach. We also added clinical data previously suggested to predict tumor response, and still our model provided better correlation with treatment response. The lack of a validation of the heat-map approach in an independent cohort is a limitation of the study. Ongoing studies will hopefully validate these data in the future. Following a successful verification, this novel approach could aid in identifying patients with a good chance of achieving a pCR. The stratification could potentially also be used to identify patients who would benefit from intratumoral immune cell reinvigoration preceding, or in combination with, neoadjuvant treatment to replace surgery.
Ethical approval
Swedish Ethical Review Authority, Dnr 2019–04748
Supplemental Material
Download PDF (2.8 MB)Disclosure statement
No potential conflict of interest was reported by the author(s).
Data availability statement
Data are available within the article and supplementary material.
Supplementary material
Supplemental data for this article can be accessed online at https://doi.org/10.1080/2162402X.2023.2209473
Correction Statement
This article has been republished with minor changes. These changes do not impact the academic content of the article.
Additional information
Funding
References
- Dossa F, Chesney TR, Acuna SA, Baxter NN. A watch-and-wait approach for locally advanced rectal cancer after a clinical complete response following neoadjuvant chemoradiation: a systematic review and meta-analysis. Lancet Gastroenterol Hepatol. 2017;2(7):501–8. doi:10.1016/S2468-1253(17)30074-2.
- Galon J, Type, density, and location of immune cells within human colorectal tumors predict clinical outcome. Science. 2006;313(5795):1960–1964. doi:10.1126/science.1129139.
- Galon J, Lanzi A. Immunoscore and its introduction in clinical practice. Q J Nucl Med Mol Imaging. 2020;64(2):152–161. doi:10.23736/S1824-4785.20.03249-5.
- Marliot F, Chen X, Kirilovsky A, Sbarrato T, El Sissy C, Batista L, Van den Eynde M, Haicheur-Adjouri N, Anitei M-G, Musina A-M, et al. Analytical validation of the Immunoscore and its associated prognostic value in patients with colon cancer. J ImmunoTher Cancer. 2020;8:(1). doi:10.1136/jitc-2019-000272.
- Pagès F, International validation of the consensus Immunoscore for the classification of colon cancer: a prognostic and accuracy study. Lancet. 2018;391(10135):2128–2139. doi:10.1016/S0140-6736(18)30789-X.
- Mlecnik B, Tosolini M, Kirilovsky A, Berger A, Bindea G, Meatchi T, Bruneval P, Trajanoski Z, Fridman W-H, Pagès F, et al. Histopathologic-based prognostic factors of colorectal cancers are associated with the state of the local immune reaction. J Clin Oncol. 2011;29(6):610–618. doi:10.1200/JCO.2010.30.5425.
- El Sissy C, Kirilovsky A, Van den Eynde M, Muşină A-M, Anitei M-G, Romero A, Marliot F, Junca A, Doyen J, Mlecnik B, et al. A diagnostic biopsy-adapted immunoscore predicts response to neoadjuvant treatment and selects patients with rectal cancer eligible for a watch-and-wait strategy. Clin Cancer Res. 2020;26(19):5198–5207. doi:10.1158/1078-0432.CCR-20-0337.
- Huang Y, Lou Y, Zhu X, Wang YC, Zhang L, Liu HL. Local environment in biopsy better predict the pathological response to neoadjuvant chemoradiotherapy in rectal cancer. Biosci Rep. 2019:39(3). doi:10.1042/BSR20190003
- Xu Y, Lou X, Liang Y, Zhang S, Yang S, Chen Q, Xu Z, Zhao M, Li Z, Zhao K, et al. Predicting neoadjuvant chemoradiotherapy response in locally advanced rectal cancer using tumor-infiltrating lymphocytes density. . 2021;14:5891–5899. doi:10.2147/JIR.S342214.
- Yasuda K, Nirei T, Sunami E, Nagawa H, Kitayama J. Density of CD4(+) and CD8(+) T lymphocytes in biopsy samples can be a predictor of pathological response to chemoradiotherapy (CRT) for rectal cancer. Radiat Oncol. 2011;6:49. doi:10.1186/1748-717X-6-49.
- Farchoukh L, Intratumoral budding and automated CD8-positive T-cell density in pretreatment biopsies can predict response to neoadjuvant therapy in rectal adenocarcinoma. Mod Pathol. 2021;34(1):171–183.
- McCoy MJ, Hemmings C, Anyaegbu CC, Austin SJ, Lee-Pullen TF, Miller TJ, Bulsara MK, Zeps N, Nowak AK, Lake RA, et al. Tumour-infiltrating regulatory T cell density before neoadjuvant chemoradiotherapy for rectal cancer does not predict treatment response. Oncotarget. 2017;8(12):19803–19813. doi:10.18632/oncotarget.15048
- Golstein P, Griffiths GM. An early history of T cell-mediated cytotoxicity. Nat Rev Immunol. 2018;18(8):527–535. doi:10.1038/s41577-018-0009-3.
- Jarosch A, Neoadjuvant radiochemotherapy decreases the total amount of tumor infiltrating lymphocytes, but increases the number of CD8+/Granzyme B+ (GrzB) cytotoxic T-cells in rectal cancer. Oncoimmunology. 2018;7(2):e1393133. doi:10.1080/2162402X.2017.1393133.
- Corrales L, McWhirter SM, Dubensky TW, Gajewski TF. The host STING pathway at the interface of cancer and immunity. J Clin Invest. 2016;126(7):2404–2411. doi:10.1172/JCI86892.
- Deng L, Liang H, Xu M, Yang X, Burnette B, Arina A, Li X-D, Mauceri H, Beckett M, Darga T, et al. STING-Dependent cytosolic DNA sensing promotes radiation-induced type i interferon-dependent antitumor immunity in Immunogenic tumors. Immunity. 2014;41(5):843–852. doi:10.1016/j.immuni.2014.10.019
- Zhu Y, An X, Zhang X, Qiao Y, Zheng T, Li X. STING: a master regulator in the cancer-immunity cycle. Mol Cancer. 2019;18(1):152. doi:10.1186/s12943-019-1087-y.
- Chen DS, Mellman I. Oncology meets immunology: the cancer-immunity cycle. Immunity. 2013;39(1):1–10. doi:10.1016/j.immuni.2013.07.012.
- Davis D, Yuan H, Yang Y-M, Liang F-X, Sehgal PB. Interferon-α-induced cytoplasmic MxA structures in hepatoma Huh7 and primary endothelial cells. Współczesna Onkologia. 2018;22(2):86–94. doi:10.5114/wo.2018.76149.
- Holzinger D, Jorns C, Stertz S, Boisson-Dupuis S, Thimme R, Weidmann M, Casanova J-L, Haller O, Kochs G. Induction of MxA gene expression by influenza a virus requires type I or type III interferon signaling. J Virol. 2007;81(14):7776–7785. doi:10.1128/JVI.00546-06.
- Haller O, Kochs G. Human MxA protein: an interferon-induced dynamin-like GTPase with broad antiviral activity. J Interferon Cytokine Res: The Official J Int Soc Interferon Cytokine Res. 2011;31:79–87.
- Bond E, Liang F, Sandgren KJ, Smed-Sörensen A, Bergman P, Brighenti S, Adams WC, Betemariam SA, Rangaka MX, Lange C, et al. Plasmacytoid dendritic cells infiltrate the skin in positive tuberculin skin test indurations. J Invest Dermatol. 2012;132(1):114–123. doi:10.1038/jid.2011.246.
- Ma R, Yuan D, Guo Y, Yan R, Li K. Immune effects of γδ T cells in colorectal cancer: a review. Front Immunol. 2020;11:1600. doi:10.3389/fimmu.2020.01600.
- Hammarström K, Imam I, Mezheyeuski A, Ekström J, Sjöblom T, Glimelius B. A comprehensive evaluation of associations between routinely Collected Staging Information and the Response to (Chemo)radiotherapy in Rectal Cancer. Cancers (Basel). 2020;13(1). doi:10.3390/cancers13010016.
- Liang F, Nilsson LM, Byvald F, Rezapour A, Taflin H, Nilsson JA, Yrlid U. A fraction of CD8+ T cells from colorectal liver metastases preferentially repopulate autologous patient-derived xenograft tumors as tissue-resident memory T cells. Cancers (Basel). 2022;14:14(12). doi:10.3390/cancers14122882.
- Langer R, Becker K. Tumor regression grading of gastrointestinal cancers after neoadjuvant therapy. Virchows Arch. 2018;472(2):175–186.10.1007/s00428-017-2232-x
- Mahlakoiv T, Hernandez P, Gronke K, Diefenbach A, Staeheli P. Leukocyte-derived IFN-α/β and epithelial IFN-λ constitute a compartmentalized mucosal defense system that restricts enteric virus infections. PLoS Pathog. 2015;11(4):e1004782.10.1371/journal.ppat.1004782
- Galon J, Bruni D. Approaches to treat immune hot, altered and cold tumours with combination immunotherapies. Nat Rev Drug Discov. 2019;18(3):197–218. doi:10.1038/s41573-018-0007-y.
- Denkert C, Loibl S, Noske A, Roller M, Müller BM, Komor M, Budczies J, Darb-Esfahani S, Kronenwett R, Hanusch C, et al. Tumor-associated lymphocytes as an independent predictor of response to neoadjuvant chemotherapy in breast cancer. J Clin Oncol. 2010;28(1):105–113. doi:10.1200/JCO.2009.23.7370.
- Hwang HW, Jung H, Hyeon J, Park YH, Ahn JS, Im Y-H, Nam SJ, Kim SW, Lee JE, Yu J-H, et al. A nomogram to predict pathologic complete response (pCR) and the value of tumor-infiltrating lymphocytes (TILs) for prediction of response to neoadjuvant chemotherapy (NAC) in breast cancer patients. Breast Cancer Res Treat. 2019;173(2):255–266. doi:10.1007/s10549-018-4981-x
- Yang Y, Tian W, Su L, Li P, Gong X, Shi L, Zhang Q, Zhao B, Zhao H. Tumor-infiltrating cytotoxic T cells and tumor-associated macrophages correlate with the outcomes of neoadjuvant chemoradiotherapy for locally advanced rectal cancer. Front Oncol. 2021;11:743540. doi:10.3389/fonc.2021.743540.
- Zaghloul H, Abbas A, Abdulah D. Tumor microenvironment mediators CD8(+)- and FOXP3(+)-labeled T lymphocytes are prospective prognosticators in curatively treated rectal cancer patients. J Gastrointest Cancer. 2021;52(1):177–186. doi:10.1007/s12029-020-00376-1.
- Shinto E, Omata J, Sikina A, Sekizawa A, Kajiwara Y, Hayashi K, Hashiguchi Y, Hase K, Ueno H. Predictive immunohistochemical features for tumour response to chemoradiotherapy in rectal cancer. BJS Open. 2020;4(2):301–309.10.1002/bjs5.50251
- Lai S, Lou X, Fan X, Sun W, Deng Y, Wang J, Huang Y, Dou R. Density and distribution of lymphocytes in pretherapeutic rectal cancer and response to neoadjuvant therapy. Gastroenterol Rep (Oxf). 2020;8(6):445–452. doi:10.1093/gastro/goaa016.
- Miyakita H, Tumor-infiltrating lymphocytes in biopsy specimens obtained 7 days after starting chemoradiotherapy for rectal cancer are predictors of the response to chemoradiotherapy. Oncology. 2020;98(12):869–875. doi:10.1159/000508922.
- Akiyoshi T, Tanaka N, Kiyotani K, Gotoh O, Yamamoto N, Oba K, Fukunaga Y, Ueno M, Mori S. Immunogenomic profiles associated with response to neoadjuvant chemoradiotherapy in patients with rectal cancer. Br J Surg. 2019;106(10):1381–1392. doi:10.1002/bjs.11179.
- Li Y, Li G, Zhang J, Wu X, Chen X. The dual roles of human γδ T cells: anti-tumor or tumor-promoting. Front Immunol. 2020;11:619954. doi:10.3389/fimmu.2020.619954.
- Hu G, Wu P, Cheng P, Zhang Z, Wang Z, Yu X, Shao X, Wu D, Ye J, Zhang T, et al. Tumor-infiltrating CD39 + γ δ Tregs are novel immunosuppressive T cells in human colorectal cancer. Oncoimmunology. 2017;6(2):e1277305. doi:10.1080/2162402X.2016.1277305.
- Rong L, Li K, Li R, Liu H-M, Sun R, Liu X-Y. Analysis of tumor-infiltrating gamma delta T cells in rectal cancer. World J Gastroenterol. 2016;22(13):3573–3580. doi:10.3748/wjg.v22.i13.3573.
- Lu C, Type I interferon suppresses tumor growth through activating the STAT3-granzyme B pathway in tumor-infiltrating cytotoxic T lymphocytes. J Immunother Cancer. 2019;7(1):157. doi:10.1186/s40425-019-0635-8.
- Bonavita E, Bromley CP, Jonsson G, Pelly VS, Sahoo S, Walwyn-Brown K, Mensurado S, Moeini A, Flanagan E, Bell CR, et al. Antagonistic inflammatory phenotypes dictate tumor fate and response to immune checkpoint blockade. Immunity. 2020;53(6):1215–1229.e8. doi:10.1016/j.immuni.2020.10.020